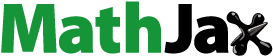
Abstract
This study reports the application of inverse virtual screening (iVS) methodologies to identify cellular proteins as suitable targets for a library of heterocyclic small-molecules, with potential pharmacological implications. Standard synthetic procedures allow facile generation of these ligands showing a high degree of core scaffold diversity. Specifically, we have computationally investigated the binding efficacy of the new series for target proteins which are involved in cancer pathogenesis. As a result, nine macromolecules demonstrated efficient binding interactions for the molecular dataset, in comparison to the co-crystallised ligand for each target. Moreover, the iVS analysis led us to confirm that 27 analogues have high affinity for one or more examined cellular proteins. The additional evaluation of ADME and drug score for selected hits also highlights their capability as drug candidates, demonstrating valuable leads for further structure optimisation and biological studies.
Graphical Abstract
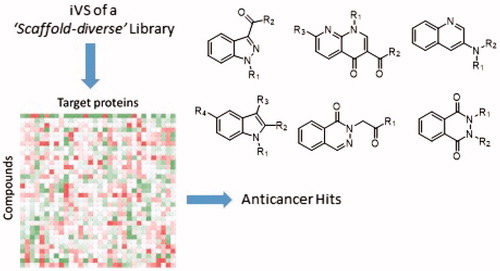
1. Introduction
Heterocyclic chemistry is one of the most valuable sources of novel molecules with a wide range of biological activities, mainly due to the unique ability of the resulting compounds to mimic the structure of endogenous ligands and reversibly bind to various targets of interestCitation1–3. In medicinal chemistry, the main advantage of heterocyclic structures possibly rely on the capability of synthesising one such a library based on a specific core, allowing screening protocols against a variety of different targetsCitation4. Fused heterocycles can also be designed with almost unlimited combinations, resulting in novel bi- or polycyclic scaffolds with diverse physical, chemical, and biological properties. Overall, the fusion of rings leads to sterically well-defined and rigid structures, holding the promise for high functional specialisation which results from the ability to orient substituents in three-dimensional space as required by the biological targetsCitation5. From cancer therapy to the treatment of infectious, parasitic, and metabolic diseases, the drugs employed are often based on biologically active heterocyclic templates that interfere with the functioning of enzymes, the transmission of nerve impulses or the action of hormones on receptors, to name a few actions.
Scaffold diversity (i.e. variation of the nature of core scaffolds), appendage diversity (or building-block diversity, i.e. variation in structural moieties around a common scaffold), functional group diversity (i.e. variation in the functional groups present in the molecules) and stereochemical diversity (i.e. variation in the three-dimensional orientation of macromolecule-interacting residues) are the main determinants for late stage chemical diversificationCitation6–8. Indeed, it is generally recognised that the more structurally diverse a molecule is, the more likely it can interact with a particular biological macromolecule in a selective and specific mannerCitation6,Citation7,Citation9–12. In this context, the exploitation of scaffold diversity has a strategic role to reach amplified structural variation and explore new areas of the chemical space in biological investigationsCitation9,Citation13–15. Moreover, it has been reported that the overall shape diversity of small-molecules is primarily dependent on the nature of the specific molecular scaffold (being the latter also intrinsically linked to functional diversity)Citation16, with the peripheral substituents having a lower impactCitation17,Citation18. Therefore, there is a widespread consensus that the increase of scaffold diversity in a small-molecule library is one of the most effective means to implement its whole structural diversityCitation6,Citation7,Citation9,Citation13–15.
Computer-aided molecular screening has become a crucial tool in drug design and discovery and computational techniques represent a valid resource for the rapid evaluation of new compounds with potential biological activity. Currently, approaches such as structure-based, ligand-based and virtual screening are widely used in various drug discovery contexts, spanning from hit identification to lead optimisation stagesCitation19–22. The recognition of biological targets by synthetic molecules is of primary importance, as well as the possibility to analyse a big database of compounds by evaluating their binding mode with targets of pharmacological interest (i.e. virtual screening)Citation23,Citation24. In contrast, the inverse virtual screening (iVS) is a computational approach that focuses on the in silico evaluation of a panel of biological targets typically involved in diseasesCitation25–28. Specifically, multiple cellular proteins (from appropriately built databases) are screened by iVS in order to identify potential targets for suitable ligands of interest. This methodology allows the rapid analysis of crucial features in the process of hit identification, including target validation, drug repurposing and side effects/toxicity prediction. Moreover, iVS demonstrates a valuable tool to preliminary explore possible biological activities towards a selection of protein targets having pharmacological interest.
Herein we report the in silico investigation of 32 new heterocyclic small-molecules through iVS, in order to validate a scaffold-guided structural diversity approach for future biological tests. This compound dataset shows high variation in the nature of the core molecular scaffolds (i.e. indole, indazole, quinoline, naphtyridone, phthalazinone and phthalhydrazide). iVS analysis has been conducted through a panel of 32 selected proteins implicated in cancer progression and cancer cell survivalCitation18,Citation29,Citation30. The study highlights that the majority of compounds have potential to interact with the examined targets, representing an outstanding starting point to drive biological evaluation in a rapid and cost-effective fashion.
2. Results and discussion
2.1. Heterocyclic small-molecule dataset
The dataset of compounds is composed by 32 terms () which have been easily obtained through standard synthetic methodologies (see Section 1, Supporting Information), in order to introduce (alkoxy)phenyl- and (halo)phenyl-based residues (typically recurrent in bioactive agents)Citation31–34 in six heterocyclic scaffolds (i.e. indazole for 1a–f, indole for 2a–h, quinoline for 3a–d, naphtyridone for 4a–j, phthalazinone for 5 and phthalhydrazide 6a–d; ). The experimental procedures and characterisation data of all new intermediates and final compounds are reported in Supporting Information (Section 2).
Table 1. Structures of the heterocyclic small-molecules analysed by iVS screening.
2.2. Molecular modelling
The compound library was screened in iVS modality against a panel of 32 cellular targets (Table 1S, Supporting Information), which have been selected for their association to cancer progression and survival. This in silico approach allows the prediction of activity and selectivity through the evaluation of binding energies. Therefore, a large dataset of compounds can be narrowed to a defined group of promising candidates for following biological evaluation. For our purpose, calculations were performed with Autodock Vina, a validated software for iVS applicationsCitation29,Citation30. Docking analysis of crystallised ligands, with an established binding mode, were carried out in order to obtain a minimum energy level which has been used as the cut-off for the assessment of binding energies of the new ligands. In particular, the binding efficiency was evaluated through the ratio between the binding energies of analysed ligands and reference ligands co-crystallised in the protein, by applying EquationEquation (1)(1)
(1) :
(1)
(1)
The values of binding energies have been organised in a matrix of 32 structures versus 32 selected cellular targets (as shown in Table 2S, Supporting Information). Each significant result was manually checked, to avoid odd or impossible interactions. From the library, compounds showing a δ ≥ 1 in a particular protein were selected and further analysed. A mathematical filter was also applied to the resulting energies as suggested by Bifulco et al.Citation29,Citation30, in order to overcome the lack of selectivity and occurrence of false positives, as well as to avoid systematic errors associated with the interaction of ligands and biological targets. EquationEquation (2)(2)
(2) was used to normalise the binding energy values in the matrix:
(2)
(2)
Table 2. Results of calculated V values for the analysed biological targets in the study.
In this formula, V is the new value associated with each compound, V0 is the value of binding energy obtained from the docking calculation, ML is the average binding energy of each ligand (in the different targets) and MR is the average binding energy associated with each target (for the different ligands). Each single value in the matrix () represents the interaction between a single ligand versus a specific cellular protein (Table 3S and Figure1S–32S, Supporting Information). This was normalised by simultaneously taking into account the influence of the two specific averages from EquationEquation (2)(2)
(2) . The values obtained led to the selection of various compounds against the different proteins, highlighting nine targets from the entire collection (i.e. PDB code: 3l3l, 3oyw, 4qmz, 2fb8, 3lbz, 4ks8, 4u5j, 4ual and 5h2u; for correspondence between PDB codes and proteins, see Table 1S, Supporting information). Specifically, these cellular proteins showed a higher trend of V values for the compound dataset, in comparison to the V values of the specific co-crystallised inhibitor. V values against the selected targets are summarised in .
Table 3. Selected hit compounds for each target.
Once identified the suitable targets for the library, we focussed on defining potency and overall binding affinity of the compounds. We used a cut-off of 30% potency to define the most active compounds for each protein. Interestingly, 27 out of 32 analogues demonstrated to possess high binding energies for one or more of the nine identified targets (i.e. 3l3l, 3oyw, 4qmz, 2fb8, 3lbz, 4ks8, 4u5j, 4ual and 5h2u). Indeed, some active compounds show high predicted affinity for more than one target, particularly compound 6d. The lack of selectivity is not always desirable in drug discovery, although this behaviour could also represent an advantage (e.g. in the case of improved pharmacological effects of multi-target drugs)Citation35,Citation36. Therefore, additional mathematical filters (i.e. ligand efficiency or binding efficiency index) can be adopted for a more accurate analysis of the calculated selectivity for each compound. In contrast, five compounds were completely devoid of activity (i.e. 1a,c, 2d,e and 4j), with regards to the calculated binding energies. resumes the most potent ligands for each cellular protein.
As previously mentioned, the proteins analysed in this study play critical roles in tumour events and new binders are regarded as the potential agents for anti-cancer therapiesCitation37–49. Therefore, we examined literature records in order to cross-validate our method. Noteworthy, analogues based on the same core scaffolds have already demonstrated a good profile as inhibitors of the cellular targets analysed in this study. For instance, it has been reported that indole-based compounds are inhibitors of serine/threonine-protein kinase B-raf (BRAF)Citation50,Citation51, B-cell lymphoma 6 (BCL-6)Citation52, proto-oncogene tyrosine-protein kinase Src (c-Src)Citation53,Citation54 and poly (ADP-ribose) polymerase (PARP)Citation55, in clear agreement with our model. Similarly, indazole-based derivatives have been reported as inhibitors of BRAFCitation56, phthalazinone core is present in inhibitors of PARPCitation57, as well as quinoline scaffold is common in molecules acting as c-SrcCitation58 and mammalian sterile20-like protein kinase 3 (MST3) inhibitorsCitation59. These evidences validate our iVS method in order to enable the identification of suitable targets for a particular molecular library, foreseeing successful biological investigation.
2.3. Drug score and ADME assessment
The in silico assessment has been expanded through the evaluation of pharmacokinetic profiles and possible adverse side effects for the 32 new compounds reported in this study. In the first instance, we have determined the toxicity risk, the fragment-based druglikeness and the drug score (see Table 4S, Supporting Information; data calculated with DataWarrior version 4.7.2)Citation60. The assessment of toxicity risk aims to locate substructures within the chemical structure which are indicative of risk within the four major toxicity classes – i.e. mutagenicity, tumorigenicity, irritating effects, and reproductive effects. The fragment-based druglikeness is based on a list of distinct substructure fragments with associated scores. The druglikeness is calculated summing up score values of those fragments that are present in the particular molecule under investigation. The drug score combines druglikeness, cLogP, LogS, molecular weight, and toxicity risks in one value that may be used to judge the overall potential of the compound to qualify as a drug. The results of these calculations for the entire library propose several compounds with a positive druglikeness and drug score > 0.3. In particular, 2 g, 2 h, 4 h, 5 and 6d demonstrated valuable profiles as drug candidate. For the five hits, the ADME properties were also calculated and the results are reported in . The results show that compounds 2 g, 2 h, 4 h, 5 and 6d exhibit also a good oral bioavailability (i.e. human intestinal absorption > 95%) and Caco-2 cell permeability >22 nm s−1 (). Although their high plasma protein binding (PPB >85%), 2 h, 4 h, 5 and 6d are also supposed to satisfyingly permeate the blood-brain barrier (BBB penetration <1).
Table 4. In silico ADME profile of selected hits.
3. Conclusions
We have described here the computational evaluation of a newly synthesised series of 32 heterocyclic small-molecules to explore molecular diversity and scaffold hopping through iVS approaches. Standard synthetic procedures allow the ease production of these compounds which are based on different heterocyclic scaffolds (i.e. indole, indazole, quinoline, naphtyridone, phthalazinone, and phthalhydrazide). The increase of scaffold diversity in small-molecules is recognised as an efficient way to implement the structural variation of molecular libraries, in order to reach specific interaction with a particular biological macromolecule.
iVS represents a validated computational tool for the assessment of binding towards targets of pharmacological interest. We have used this approach to define preliminary evaluation of the compound dataset versus a panel of cellular proteins involved in cancer progression and cancer cell survival. In the calculations, the normalisation of predicted binding energies allows to identify effective interactions for the compounds with nine biological targets – i.e. PARP, MST3, BCL6, c-Src, B-Raf kinase, galectin-1, serine/threonine-protein kinase PAK 6, serine/threonine-protein kinase MRCK beta, and protein-tyrosine kinase 6. Moreover, this study highlights a defined set of biological targets relevant for each active compound, which will drive subsequent biological screening.
SI_v5.docx
Download MS Word (413 KB)Disclosure statement
The authors declare no conflicts of interest.
References
- Franzen RG. Recent advances in the preparation of heterocycles on solid support: a review of the literature. J Comb Chem 2000;2:195–214.
- Dolle RE, Le Bourdonnec B, Morales GA, et al. Comprehensive survey of combinatorial library synthesis: 2005. J Comb Chem 2006;8:597–635.
- Kaushik NK, Kaushik N, Attri P, et al. Biomedical importance of indoles. Molecules 2013;18:6620–62.
- Macarron R, Banks MN, Bojanic D, et al. Impact of high-throughput screening in biomedical research. Nat Rev Drug Discov 2011;10:188–95.
- Shi F, Zeng XN, Cao XD, et al. Design and diversity-oriented synthesis of novel 1,4-thiazepan-3-ones fused with bioactive heterocyclic skeletons and evaluation of their antioxidant and cytotoxic activities. Bioorg Med Chem Lett 2012;22:743–6.
- Galloway WR, Spring DR. Is synthesis the main hurdle for the generation of diversity in compound libraries for screening? Expert Opin Drug Discov 2009;4:467–72.
- Galloway WRJD, Isidro-Llobet A, Spring DR. Diversity-oriented synthesis as a tool for the discovery of novel biologically active small molecules. Nat Commun 2010;1:1.
- Isidro-Llobet A, Murillo T, Bello P, et al. Diversity-oriented synthesis of macrocyclic peptidomimetics. Proc Natl Acad Sci USA 2011;108:6793–8.
- Galloway WRJD, Bender A, Welch M, Spring DR. The discovery of antibacterial agents using diversity-oriented synthesis. Chem Commun 2009;18:2446–62.
- Spandl RJ, Bender A, Spring DR. Diversity-oriented synthesis; a spectrum of approaches and results. Org Biomol Chem 2008;6:1149–58.
- Lipinski C, Hopkins A. Navigating chemical space for biology and medicine. Nature 2004;432:855–61.
- Spring DR. Diversity-oriented synthesis; a challenge for synthetic chemists. Org Biomol Chem 2003;1:3867–70.
- Burke MD, Berger EM, Schreiber SL. Generating diverse skeletons of small molecules combinatorially. Science 2003;302:613–8.
- Kennedy JP, Williams L, Bridges TM, et al. Application of combinatorial chemistry science on modern drug discovery. J Comb Chem 2008;10:345–54.
- Shelat AA, Guy RK. Scaffold composition and biological relevance of screening libraries. Nat Chem Biol 2007;3:442–6.
- Galloway WRJD, Diáz-Gavilán M, Isidro-Llobet A, Spring DR. Synthesis of unprecedented scaffold diversity. Angew Chem Int Ed Engl 2009;48:1194–6.
- Sauer WH, Schwarz MK. Size doesn't matter: Scaffold diversity, shape diversity and biological activity of combinatorial libraries. CHIMIA Int J Chem 2003;57:276–83.
- Sauer WH, Schwarz MK. Molecular shape diversity of combinatorial libraries: a prerequisite for broad bioactivity. J Chem Inf Comput Sci 2003;43:987–1003.
- Austin ND, Sahinidis NV, Trahan DW. Computer-aided molecular design: An introduction and review of tools, applications, and solution techniques. Chem Eng Res Design 2016;116:2–26.
- Floresta G, Amata E, Dichiara M, et al. Identification of potentially potent heme oxygenase 1 inhibitors through 3D-QSAR coupled to scaffold-hopping analysis. ChemMedChem 2018;13:1336–42.
- Greish KF, Salerno L, Al Zahrani R, et al. Novel structural insight into inhibitors of heme oxygenase-1 (ho-1) by new imidazole-based compounds: Biochemical and in vitro anticancer activity evaluation. Molecules 2018;23:1209.
- Salerno L, Amata E, Romeo G, et al. Potholing of the hydrophobic heme oxygenase-1 western region for the search of potent and selective imidazole-based inhibitors. Eur J Med Chem 2018;148:54–62.
- Ruiz-Torres V, Encinar JA, Herranz-Lopez M, et al. An updated review on marine anticancer compounds: The use of virtual screening for the discovery of small-molecule cancer drugs. Molecules 2017;22:1037.
- Lavecchia A, Di Giovanni C. Virtual screening strategies in drug discovery: a critical review. Curr Med Chem 2013;20:2839–60.
- Zhao H, Lin C, Hu K, et al. Discovery of novel estrogen-related receptor alpha inverse agonists by virtual screening and biological evaluation. J Biomol Struct Dyn 2018;1–8. doi:10.1080/07391102.2018.1462736.
- Xu X, Huang M, Zou X. Docking-based inverse virtual screening: Methods, applications, and challenges. Biophys Rep 2018;4:1–16.
- Song Y, Xue X, Wu X, et al. Identification of n-phenyl-2-(n-phenylphenylsulfonamido)acetamides as new RORγ inverse agonists: virtual screening, structure-based optimization, and biological evaluation. Eur J Med Chem 2016;116:13–26.
- Perez GM, Salomon LA, Montero-Cabrera LA, et al. Integrating sampling techniques and inverse virtual screening: Toward the discovery of artificial peptide-based receptors for ligands. Mol Divers 2016;20:421–38.
- Lauro G, Masullo M, Piacente S, et al. Inverse virtual screening allows the discovery of the biological activity of natural compounds. Bioorg Med Chem 2012;20:3596–602.
- Lauro G, Romano A, Riccio R, Bifulco G. Inverse virtual screening of antitumor targets: Pilot study on a small database of natural bioactive compounds. J Nat Prod 2011;74:1401–7.
- Tong YF, Zhang P, Chen F, et al. Synthesis and biological evaluation of novel n-(alkoxyphenyl)-aminocarbonylbenzoic acid derivatives as ptp1b inhibitors. Chinese Chemical Letters 2010;21:1415–8.
- Abdel-Aziz HA, Eldehna WM, Fares M, et al. Synthesis, biological evaluation and 2d-qsar study of halophenyl bis-hydrazones as antimicrobial and antitubercular agents. International Journal of Molecular Sciences 2015;16:8719–43.
- Moldoveanu CC, Mangalagiu II. 4‐methyl‐and 4‐(halophenyl) pyrimidinium (4‐halobenzoyl) methylides. Correlation of structure, stability, reactivity, and biological activity. Helvetica Chimica Acta 2005;88:2747–56.
- Gonec T, Zadrazilova I, Nevin E, et al. Synthesis and biological evaluation of n-alkoxyphenyl-3-hydroxynaphthalene-2-carboxanilides. Molecules 2015;20:9767–87.
- Abad-Zapatero C, Metz JT. Ligand efficiency indices as guideposts for drug discovery. Drug Discov Today 2005;10:464–9.
- Floresta G, Pistara V, Amata E, et al. Molecular modeling studies of pseudouridine isoxazolidinyl nucleoside analogues as potential inhibitors of the pseudouridine 5'-monophosphate glycosidase. Chem Biol Drug Des 2018;91:519–25.
- Minoo P, Zlobec I, Baker K, et al. Prognostic significance of mammalian sterile20-like kinase 1 in colorectal cancer. Mod Pathol 2007;20:331–8.
- de Souza PM, Lindsay MA. Mammalian sterile20-like kinase 1 and the regulation of apoptosis. Biochem Soc Trans 2004;32:485–8.
- Han S, Ren Y, He W, et al. Publisher correction: Erk-mediated phosphorylation regulates sox10 sumoylation and targets expression in mutant braf melanoma. Nat Commun 2018;9:1404.
- Han S, Ren Y, He W, et al. Erk-mediated phosphorylation regulates sox10 sumoylation and targets expression in mutant braf melanoma. Nat Commun 2018;9:28.
- Boshuizen J, Koopman LA, Krijgsman O, et al. Cooperative targeting of melanoma heterogeneity with an axl antibody-drug conjugate and braf/mek inhibitors. Nat Med 2018;24:203–12.
- Lavoie H, Sahmi M, Maisonneuve P, et al. Mek drives braf activation through allosteric control of ksr proteins. Nature 2018;554:549–53.
- Wang Q, Ding W, Ding Y, et al. Homoharringtonine suppresses imatinib resistance via the bcl-6/p53 pathway in chronic myeloid leukemia cell lines. Oncotarget 2017;8:37594–604.
- Jandl C, Liu SM, Canete PF, et al. Il-21 restricts t follicular regulatory t cell proliferation through bcl-6 mediated inhibition of responsiveness to il-2. Nat Commun 2017;8:14647.
- Qian XL, Zhang J, Li PZ, et al. Dasatinib inhibits c-src phosphorylation and prevents the proliferation of triple-negative breast cancer (tnbc) cells which overexpress syndecan-binding protein (sdcbp). PLoS One 2017;12:e0171169.
- Zhang J, Wang S, Jiang B, et al. C-src phosphorylation and activation of hexokinase promotes tumorigenesis and metastasis. Nat Commun 2017;8:13732.
- Pishvaian MJ, Slack RS, Jiang W, et al. A phase 2 study of the parp inhibitor veliparib plus temozolomide in patients with heavily pretreated metastatic colorectal cancer. Cancer 2018;124:2337–46.
- Chen X, Huan X, Liu Q, et al. Design and synthesis of 2-(4,5,6,7-tetrahydrothienopyridin-2-yl)-benzoimidazole carboxamides as novel orally efficacious poly(adp-ribose)polymerase (parp) inhibitors. Eur J Med Chem 2018;145:389–403.
- Langelier MF, Zandarashvili L, Aguiar PM, et al. NAD + analog reveals PARP-1 substrate-blocking mechanism and allosteric communication from catalytic center to DNA-binding domains. Nat Commun 2018;9:844.
- Lee JT, Li L, Brafford PA, et al. Plx4032, a potent inhibitor of the b-raf v600e oncogene, selectively inhibits v600e-positive melanomas. Pigment Cell Melanoma Res 2010;23:820–7.
- Wu Z, Yan M, Hu SH, et al. Design, synthesis and biological evaluation of indole derivatives as novel inhibitors targeting b-raf kinase. Chin Chem Lett 2014;25:351–4.
- Melnick A, Cerchietti LCA, Cardenas MG, et al. Bcl6 inhibitors as anticancer agents in Bcl6 inhibitors as anticancer agents, Google Patents; 2018.
- Olgen S, Akaho E, Nebioglu D. Evaluation of indole esters as inhibitors of p60(c-src) receptor tyrosine kinase and investigation of the inhibition using receptor docking studies. Journal of Enzyme Inhibition and Medicinal Chemistry 2003;18:485–90.
- Kiliç Z, Işgör YG, Olgen S. Evaluation of new indole and bromoindole derivatives as pp60(c-src) tyrosine kinase inhibitors. Chem Biol Drug Des 2009;74:397–404.
- Cosi C. New inhibitors of poly(adpribose) polymerase and their potential therapeutic targets. Expert Opinion on Therapeutic Patents 2002;12:1047–71.
- Ren L, Wenglowsky S, Miknis G, et al. Non-oxime inhibitors of b-raf(v600e) kinase. Bioorg Med Chem Lett 2011;21:1243–7.
- Johannes JW, Almeida L, Daly K, et al. Discovery of az0108, an orally bioavailable phthalazinone parp inhibitor that blocks centrosome clustering. Bioorg Med Chem Lett 2015;25:5743–7.
- Thaimattam R, Daga PR, Banerjee R, Iqbal J. 3d-qsar studies on c-src kinase inhibitors and docking analyses of a potent dual kinase inhibitor of c-src and c-abl kinases. Bioorg Med Chem 2005;13:4704–12.
- Olesen SH, Zhu J-Y, Martin MP, Schönbrunn E. Discovery of diverse small-molecule inhibitors of mammalian sterile20-like Kinase 3 (MST3)). ChemMedChem 2016;11:1137–44.
- Sander T, Freyss J, von Korff M, Rufener C. Datawarrior: An open-source program for chemistry aware data visualization and analysis. J Chem Inf Model 2015;55:460–73.