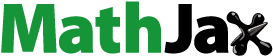
Abstract
Parkinson’s disease is one of the most common neurodegenerative disorders in elderly age. One of the mechanisms involved in the neurodegeneration appears related to the aggregation of the presynaptic protein alpha synuclein (α-syn) into toxic oligomers and fibrils. To date, no highly effective treatment is currently available; therefore, there is an increasing interest in the search of new therapeutic tools. The modulation of α-syn aggregation represents an emergent and promising disease-modifying strategy for reducing or blocking the neurodegenerative process. Herein, by combining in silico and in vitro screenings we initially identified 3-(cinnamylsulfanyl)-5-(4-pyridinyl)-1,2,4-triazol-4-amine (3) as α-syn aggregation inhibitor that was then considered a promising hit for the further design of a new series of small molecules. Therefore, we rationally designed new hit-derivatives that were synthesised and evaluated by biological assays. Lastly, the binding mode of the newer inhibitors was predicted by docking studies.
Graphical Abstract
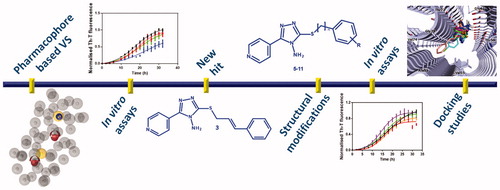
1. Introduction
Parkinson’s disease (PD) is a neurodegenerative disorder characterised by the loss of dopaminergic neurons in the substantia nigra of the brain. These cells are involved in the production of the neurotransmitter dopamine which regulates the muscular movementsCitation1. Typical manifestations of PD include motor symptoms due to the dopaminergic loss, like bradykinesia, rigidity, postural instability and rest tremorCitation2. Additionally, non-motor features such as olfactory dysfunction, constipation, cognitive impairments, depression and sleep disorders can occur; these further symptoms are due to the implication of the neurodegenerative process in other areas of the peripheral and central nervous systemCitation3. The hallmark of PD is represented by the presence of neuronal inclusions, termed Lewis Bodies, mainly composed of aggregates of misfolded alpha synuclein (α-syn)Citation4. α-Syn is a 140 aa presynaptic protein which regulates the release of neurotransmitters from the synaptic vesiclesCitation5. From a structural point of view, α-syn is organised in three different regions: the N-terminal domain (aa 1-60), the central NAC domain (aa 61-95) and the C-terminal domain (aa 96-140)Citation6. In its monomeric soluble form, α-syn assumes an alpha helical conformation upon interaction with phospholipids,Citation7 while in the pathological misfolded state, it aggregates into amyloid fibrils composed by parallel hydrogen bonded β-sheetsCitation8,Citation9. The formation of these aggregates causes cytotoxicity through lipid membrane permeabilisation, mitochondrial damage and oxidative stressCitation10. Another relevant mechanism that contributes to the propagation of neurodegeneration is the prion-like spread of α-syn aggregates. Indeed, experimental studies revealed that the injection of α-syn inclusions in animal’s brain promotes the formation of cellular inclusions at the injection site from where they can spread in other brain regionsCitation11. To date, the therapies available for the treatment of PD are addressed to reduce the motor symptoms and include the administration of drugs able to restore the level of dopamine. Among them the most used is L-Dopa, which acts as a prodrug being converted in dopamine in the brainCitation1,Citation12. Other dopaminergic drugs used for the treatment of PD are dopamine agonists like ropinirole or rotigotine, monoamine oxidase B (MAO-B) inhibitors such as rasagiline and selegiline and catechol-O-methyltransferase (COMT) inhibitors such as tolcapone and entacapone which inhibit the enzymes responsible of dopamine metabolismCitation2,Citation13. Unfortunately, the use of these drugs induces unwanted side effects such as dyskinesia, dizziness, headaches, nausea and somnolenceCitation13. More serious problems like hallucinations, confusion and impulse control disorders are often associated with the assumption of dopamine agonistsCitation14. Furthermore, these therapies lose their efficacy as the disease progresses and are unable to block or reduce the neurodegenerative processCitation15,Citation16. In the last decade, several efforts have been made to find a disease modifying strategy to halt or slow the neurodegenerationCitation17. In this context, the inhibition of α-syn aggregation by small molecules proved to be a valid approach for the development of new therapeutics for the treatment of PD and several inhibitors have been discovered through high-throughput screening campaigns and drug repositioningCitation18,Citation19.
In this work, we applied a pharmacophore-based virtual screening approach as effective tool to discover novel α-syn aggregation inhibitors. Firstly, we developed a computational model that was subsequently combined with in vitro experiments to test their ability to reduce α-syn aggregation; as result we discovered a small molecule as promising inhibitor, which was used as lead compound for the development of a further series of compounds. Then, the designed molecules were synthesised, tested in vitro and studied to decipher the putative binding mode by molecular docking simulation.
2. Materials and Methods
2.1. Pharmacophore modelling and virtual screening
LigandScout V4.4Citation20 was used for the pharmacophore generation and the virtual screening experiments. Three small molecules able to bind to the N-terminal region of α-syn have been selected from literatureCitation21 and used as training set. A shared-feature pharmacophore model was created applying the default settings.
All virtual screening runs were performed by setting the option “Get best matching conformation” as retrieval mode.
2.2. Chemistry
All reagents were used without further purification and bought from common commercial suppliers. Melting points were determined on a Buchi B-545 apparatus (BUCHILabortechnik AG Flawil, Switzerland). By combustion analysis (C, H, N) carried out on a Carlo Erba Model1106-Elemental Analyser we determined the purity of synthesised compounds; the results confirmed a ± 95% purity. Merck Silica Gel 60 F254 plates were used for analytical TLC (Merck KGaA, Darmstadt, Germany). For detection, iodine vapour and UV light (254 nm) were used. 1H and 13C NMR spectra were measured in dimethylsulfoxide-d6 (DMSO-d6) with a Varian Gemini 500 spectrometer (Varian Inc. Palo Alto, California USA); chemical shifts are expressed in δ (ppm).
2.2.3. General procedure for the synthesis of pyridinyl-triazole derivatives 5-11
The 4-amino-5–(4-pyridinyl)-4H-1,2,4-triazole-3-thiol 12 (200 mg, 1,03 mmol) was dissolved in a mixture of NaOH (41 mg, 1,03 mmol) and MeOH (5 ml); after complete dissolution, the suitable benzyl bromide derivative (194,7 mg, 1,03 mmol) was added. The reaction mixture was stirred for 15 min at room temperature for desired compounds 5 and 7–11, whereas for desired compound 6 the reaction time was 120 min. The resulting solid residue was washed with water, dried and recrystallized from ethanol to provide pure desired final pyridyl-triazole derivatives 5–11. For all synthesised compounds the registered CAS numbers have been already assigned; However, the synthetic procedure, chemical and structural characterisation are not available in literature with exception of compound 5, for which the structural characterisation is an agreement with literatureCitation22.
2.2.3.1. 3-(Phenethylthio)-5-(pyridin-4-yl)-4H-1,2,4-triazol-4-amine (6)
CAS number: 691366-30-0. Yield 12%. Yellow solid. M.p. 168–169 °C. 1H NMR (DMSO-d6): δ 3.04 (t, 2H, J = 7.74 Hz, CH2); 3.44 (t, 2H, J = 7.74 Hz, CH2); 6.19 (s, 2H, NH2); 7.21–8.72 (m, 9H, ArH) Anal. for C15H15N5S: C, 60.58%; H, 5.08%; N, 23.55%. Found C, 60.48%; H, 5.33%; N, 23.43%.
2.2.3.2. 3-((4-Fluorobenzyl)thio)-5-(pyridin-4-yl)-4H-1,2,4-triazol-4-amine (7)
CAS number: 575460-68-3. Yield 39%. Yellow solid. M.p. 186–187 °C. 1H NMR (DMSO-d6): δ 4.44 (s, 2H, CH2); 6.21 (s, 2H, NH2); 7.13–8.71 (m, 8H, ArH). 13C NMR (DMSO-d6): δ 34.0; 115.1 (d, 2JC-F = 19.1 Hz), 115.3 (d, 2JC-F = 19.1 Hz); 121.2; 121.3; 131.0 (d, 3JC-F = 8.6 Hz); 131.1 (d, 3JC-F = 8.6 Hz); 133.8 (d, 4JC-F = 2.9 Hz) 134.0; 150.1; 152.0; 154.5; 161.4 (d, 1JC-F = 243.2 Hz). Anal. for C14H12FN5S: C, 55.80%; H, 4.01%; N, 23.24%. Found C, 55.68%; H, 4.31%; N, 23.44%.
2.2.3.3. 3-((3-Fluorobenzyl)thio)-5-(pyridin-4-yl)-4H-1,2,4-triazol–4-amine (8)
CAS number: 674812-86-3. Yield 52%. Yellow solid. M.p. 164–165 °C. 1H NMR (DMSO-d6): δ 4.45 (s, 2H, CH2); 6.25 (s, 2H, NH2); 7.06–8.72 (m, 8H, ArH); 13C NMR (DMSO-d6): δ 34.6; 114.6 (d, 2JC-F = 20.9 Hz), 116.3 (d, 2JC-F = 21.0 Hz); 121.7; 121.8; 125.6 (d, 4JC-F = 2.7 Hz); 130.8 (d, 3JC-F = 8.2 Hz); 134.4, 141.0 (d, 3JC-F = 8.2 Hz); 150.5; 152.5; 154.8; 162.4 (d, 1JC-F = 243.4 Hz). Anal. for C14H12FN5S: C, 55.80%; H, 4.01%; N, 23.24%. Found C, 55.89%; H, 4.00%; N, 23.15%.
2.2.3.4. 3-((4-Methylbenzyl)thio)-5-(pyridin-4-yl)-4H-1,2,4-triazol-4-amine (9)
CAS number: 676147-32-3. Yield 13%. Yellow solid. M.p. 180–181 °C 1H NMR (DMSO-d6): δ 2.25 (s, 3H, CH3); 4.40 (s, 2H, CH2); 6.19 (s, 2H, NH2); 7.10–8.71 (m, 8H, ArH). 13C NMR (DMSO-d6): δ 21.0; 35.2; 110.0; 121.7; 121.8; 129.3; 129.6 134.4; 134.7; 137.1; 141.0; 150.4; 150.7; 152.4; 155.1. Anal. for C15H15N5S: C, 60.58%; H, 5.08%; N, 23.55%. Found C, 60.23%; H, 5.26%; N, 23.40%.
2.2.3.5. 3-((3-Methylbenzyl)thio)-5-(pyridin-4-yl)-4H-1,2,4-triazol-4-amine (10)
CAS number: 676548-28-0. Yield 16%. Whitish solid. M.p 175–176 °C; 1H NMR (DMSO-d6): δ 2.26 (s, 3H, CH3); 4.40 (s, 2H, CH2); 6.19 (s, 2H, NH2); 7.07–8.71 (m, 8H, ArH). 13C NMR (DMSO-d6): δ 21.3; 35.4; 121.7; 126.7; 128.5; 128.8; 130.0; 134.4; 137.6; 138.0; 150.6; 152.4; 155.1. Anal. for C15H15N5S: C, 60.58%; H, 5.08%; N, 23.55%. Found C, 60.70%; H, 5.01%; N, 23.32%.
2.2.3.6. 3-((4-Chlorobenzyl)thio)-5-(pyridin-4-yl)-4H-1,2,4-triazol–4-amine (11)
CAS number: 901093-20-7. Yield 39%. Yellow solid. M.p 198–199 °C; 1H NMR (DMSO-d6): δ 4.44 (s, 2H, CH2); 6.22 (s, 2H, NH2); 7.37–8.70 (m, 8H, ArH). 13C NMR (DMSO-d6): δ 34.4; 121.7; 121.8; 128.61; 129.0; 131.2; 131.5; 132.4; 134.4; 137.2; 150.4; 150.7; 152.5; 154.8. Anal. for C14H12ClN5S: C, 52.91%; H, 3.81%; N, 23.04%. Found C, 52.66%; H, 3.78%; N, 23.36%.
2.2. 2.3. Alpha synuclein aggregation and inhibition in vitro assays
WT α-Syn was expressed and purified as previously describedCitation23. The resultant protein was kept lyophilised at −80 °C. Lyophilised protein was delicately resuspended in sterile PBS 1X and filtered through 0.22-µm membrane to eliminate small aggregates. Aggregation assays were performed as previously described for ZPD-2 and SynuClean-D, the latter used as reference compound for kinetic studiesCitation16,Citation24. Briefly, 70 µM of α-Syn, in a final volume of 150 µL, was placed in a sealed 96-well plate, which also contains 40 μM Th-T in PBS 1X, a 1/8” diameter Teflon polyball (Polysciences Europe GmbH, Eppelheim, Germany) and 100 μM of the different compounds or DMSO (in control samples). The plates were fixed in an orbital shaker Max-Q 4000 (ThermoScientific, Waltham, Massachusetts, USA) and incubated at 37 °C and 100 rpm. Th-T fluorescence emission was measured every 2 h in a Victor3.0 Multilabel Reader (PerkinElmer, Waltham, Massachusetts, USA) by exciting through a 430–450 nm filter and collecting with a 480-510 filter. Triplicates were performed and the kinetics were fitted with the following equation:
(1)
(1)
In which kb and ka represent the homogeneous nucleation rate constant and the secondary rate constant (it means, fibril elongation and secondary nucleation), respectivelyCitation25.
Light-scattering measurements were performed in a Cary Eclipse Fluorescence Spectrophotometer (Agilent, Santa Clara, California, USA). 80 µL of end-point aggregates was placed into a quartz cuvette and excited at 300 nm, the 90° emission was thus collected between 280 and 360 nm.
For transmission electron microscopy (TEM) assays, endpoint treated and untreated aggregates diluted 1:10 in PBS 1X. Samples were then softly sonicated for 5 min and 5 µL of the resultant mixture were placed on a carbon-coated copper grid for 5 min. The grids were dried with filter paper to remove the excess and washed twice in miliQ water, whose excess was also removed. Finally, 5 μL of 2% (w/v) uranyl acetate solution was added and left incubate for 2 min. As previously, the excess of uranyl acetate was removed, and grids were left to air-dry for 10 min. Images were obtained using a Transmission Electron Microscopy Jeol 1400 (Peabody, Massachusetts, USA) operating at an accelerating voltage of 120 kV. A minimum of 30 fields were screened per sample, in order to collect representative images.
2.4. Docking studies
Docking studies were performed by Autodock4.2 software by using the solid-state NMR of alpha-synuclein fibrils retrieved from RCSB Protein Data Bank (PDB code 2N0A). The structure is characterised by a central part with a greek-key topology and terminal flexible loops. A grid box with dimension of 126 × 126 × 126 Å3 and centre x = 97.487, y = 148.695 and z = −34,111, was applied in order to include the aminoacid residues of the N-terminal region discarding the ones present in the unstructured flexible loops. Ligand structures were constructed by Vega ZZ software and energy minimised by following a conjugate gradient minimisation by AMMP calculation as implemented in VEGAZZ programCitation26. The Lamarckian Genetic Algorithm was used to calculate 10 protein − ligand binding poses for each compound by using the default settings. The highest scored docking pose was chosen for analysis and representation. PyMOL software was used to visualise docking results while the analysis of the putative ligand–protein interactions was performed by Discovery Studio VisualiserCitation27.
3. Results and discussion
3.1. Pharmacophore model generation, virtual screening and preliminary biological assay
With the aim to find new molecules able to inhibit α-syn aggregation, we used a computational approach to generate a ligand-based pharmacophore model by means of the software LigandScoutCitation20.
To build the model, we used a training set (TS) including α-syn aggregation inhibitors that target the N-terminal portion of the protein. Specifically, we selected three small molecules from literatureCitation21 belonging to different chemotypes as polyphenolic and non-polyphenolic inhibitors () for which the binding interaction to N-terminal portion of α-syn is corroborated by experimental data.
Table 1. Chemical structures of the α-syn aggregation inhibitors used as training set (TS).
In particular, we considered only the common features for all the selected molecules of the TS for the model construction generating shared feature pharmacophore models.
As result, we obtained ten pharmacophore hypotheses and selected the hypothesis possessing the highest score to perform virtual screening studies. As displayed in , the above-mentioned pharmacophore model consisted of five features: (i) two hydrogen bond acceptors, (ii) two hydrophobic features, (iii) one aromatic feature and 45 excluded volumes as forbidden areas.
Figure 1. (A) Best scored ligand-based pharmacophore model constituted by two hydrogen bond acceptors (red spheres), two hydrophobic features (yellow spheres), one aromatic feature (blue circle). Forty-five excluded volumes are represented by grey spheres. (B) TS molecules aligned with the highest scored pharmacophore model.
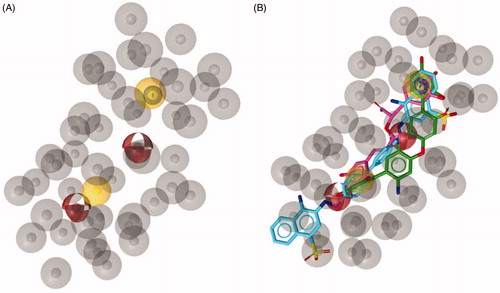
The obtained pharmacophore model was used as a query to screen two three-dimensional databases containing small molecules belonging to different structural chemotypes: (i) the in-house 3 D database CHIME collecting 1329 molecules designed and synthesised by our group over the years, (ii) the MyriaScreen Diversity Library II, consisting of 10,000 compounds with druglike properties (https://www.sigmaaldrich.com/chemistry/chemistry-services/high-throughput-screening/screening-compounds.html). From CHIME, the virtual screening led to the identification of three hits 1a–c (). Considering that these molecules shared a very similar structure, we decided to select only 2-[4-[(4-fluorophenyl)methyl]-1-piperidyl]-1-(6-methoxy-1H-indol-3-yl)ethenone (1a) displaying the highest pharmacophore fit-score as representative inhibitor filtered through virtual screening on CHIME database ().
Figure 2. (A) Chemical structure of compounds 1a-c. (B) Compound 1a aligned to the pharmacophore model. Compound 1a is represented by grey sticks.
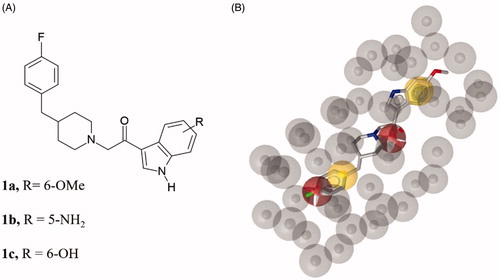
The virtual screening on MyriaScreen Diversity Library II resulted in 113 hits (see supporting material). Among them, we selected three compounds () such as 3-[5-[(4-methoxyphenyl)methylsulfanyl]-4-methyl-1,2,4-triazol-3-yl]pyridine (2), 3-(cinnamylsulfanyl)-5–(4-pyridinyl)-1,2,4-triazol-4-amine (3) and 3-(3-chloro-4-fluoro-anilino)-1-(2-naphthyl)propan-1-one (4); the selection of the compounds 2–4 was made basing on the pharmacophore-fit score value (>57) and the commercial availability from the supplier.
Figure 3. (A) Chemical structures of compounds 2, 3 and 4. (B) Alignment of each of the reported hits (represented by pink (2), orange (3) and yellow (4) sticks) with the pharmacophore model.
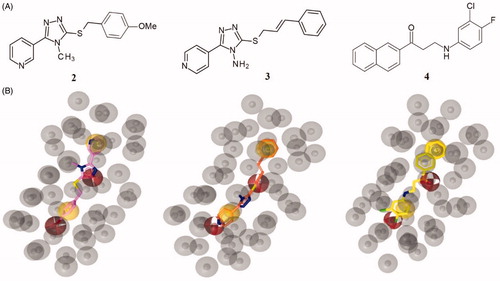
All selected compounds 1–4 proved to respect the Lipinski’s rule and no PAINS were in silico predicted by the online tool SwissAdme platform [http://www.swissadme.ch/]. To evaluate the ability to inhibit α-syn aggregation, we have got compounds 1–4 as follows. Compound 1 was readily synthesised in our laboratory following a previously optimised synthetic approach;Citation28 while the three commercially available compounds 2–4 were purchased from Sigma Aldrich as supplier of bioactive molecules [https://www.sigmaaldrich.com/italy.html].
The anti-aggregation potential of selected compounds (1–4) was evaluated by a robust protocol previously applied in the identification of strong inhibitors of α-syn aggregationCitation16,Citation24 like SynuClean-D, a well-known inhibitor of α-synuclein (α-Syn) aggregationCitation24 that was used as reference compound in the same test. The presence of 100 µM of the compounds during the incubation of 70 µM of α-Syn, reduced Thioflavin-T (Th-T) fluorescence emission up to a 10, 26, 31 and 10% for compound 1, compound 2, compound 3 and compound 4, respectively. As result, all tested compounds impacted the kinetic constants (see ). Particularly, the compound 2 reduced the homogeneous nucleation rate constant (kb = 0.01591) when compared to untreated sample (kb = 0.03234). In contrast, compound 3 reduced the autocatalytic rate constant (ka = 0.1892 h−1), compared to the control (ka = 0.2037 h−1). The compounds 1 and 4 did not impact significantly the aggregation rate constants. To confirm these results light-scattering measurements at 300 nm were performed at the end-point of the reaction, thus revealing a reduction of 13 and 43% of aggregates in the presence of compounds 2 and 3, respectively.
Figure 4. Inhibitory in vitro characterisation of compounds 1 to 4. (A) Aggregation kinetic of α-syn in absence (black) and presence of 100 µM of Compound 1 (red), compound 2 (green), compound 3 (blue), compound 4 (orange) and SynuClean-D (grey), followed by Th-T fluorescence emission. (B) Light-scattering measurements at 300 nm in the absence (black) and presence of 100 µM of Compound 1 (red), compound 2 (green), compound 3 (blue) and compound 4 (orange). (C and D) Representative TEM images of untreated (C) and compound 3 treated samples (D). Th-T fluorescence is plotted as normalised means. Final points were obtained at 48 h. Error bars are represented as SE of mean values; ** p < 0.01 and *** p < 0.001.
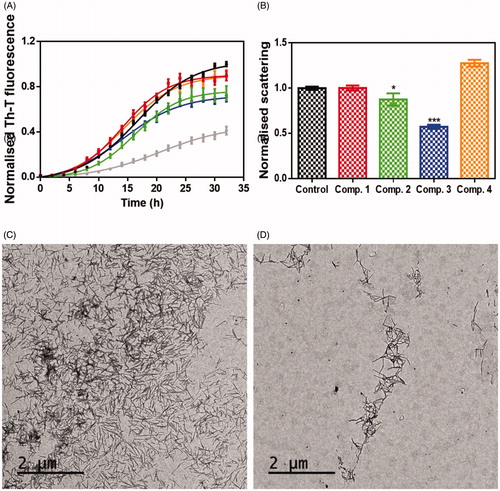
To further assess the impact of the best candidate 3 on α-syn aggregation, end-point samples were analysed by transmission electron microscopy (TEM). Notably, TEM images certified a significant reduction in the amount of fibrillar material in the presence of compound 3 (), when compared to untreated samples ().
Therefore, this first screening highlighted that the best in vitro results were obtained for compound 3. This compound is characterised by the presence of a pyridinyl-triazole moiety similarly to compound 2, in which the nitrogen of the triazole ring presents a methyl substituent while an amino group is present in compound 3 in the same position. Considering the obtained results, we can speculate that the amino group could establish fundamental interaction with the protein important for the inhibition of α-syn aggregation.
3.2. Design and synthesis of new small molecules as derivatives of compound 3
Based on the above reported results of biological screening, we chose compound 3 as promising lead compound for the rational design of a further series of pyridinyl-triazole derivatives 5–11 (Scheme 1) for which a very simple synthetic approach might be carried out.
In particular, we chose to maintain the pyridinyl-triazole moiety of inhibitor 3 and reduce the length of the linker between the sulphur atom and the phenyl ring. Moreover, we planned to extend the series by introducing selected substituents in meta and in para positions of phenyl ring. In detail, we introduced F, Cl or CH3 substituents in order to preliminary probe the influence of electron withdrawing group (EWG) or electron donating group (EDG) on the aggregation of α-syn. For each designed derivative we evaluated the adherence to the Lipinski’s rule and the absence of PAINS in silico by using SwissADME. Scheme 2 shows the synthetic procedure to obtain compounds 5-11 starting from the 4-amino-5–(4-pyridinyl)-4H-1,2,4-triazole-3-thiol 12, that was coupled with the appropriate benzyl bromide in alkaline medium at room temperature.
3.3. Screening of the activity of the new derivatives 5–11
The synthesised derivatives were tested in order to study their ability to reduce α-syn aggregation in vitro by using the method described above. Incubation of 70 µM α-Syn in the presence of 100 µM of the different compounds, followed by Th-T fluorescence measurements at the end of the reaction indicated that 5, 8, 9 and 11 could reduce the aggregation up to a 33, 15, 19 and 29%, respectively (). Light-scattering measurements at 300 nm indicated that 5, 6, 7, 8, 9 and 10 reduced the amount of aggregates up to a 23, 16, 26, 14, 24, 17%, respectively (). However, TEM analysis indicated that only 5 (), 8 (), 9 () and 11 () reduced the number of amyloid fibrils when compared to control samples (), in good agreement with the kinetic analysis.
Figure 5. Inhibitory in vitro characterisation of tested compounds 5–11. (A and B) Aggregation kinetics of α-syn in the absence (black) and presence of 100 µM of compounds 5 (red), 6 (green), 7 (violet), 8 (orange), 9 (blue), 10 (brown), 11 (yellow) and SynuClean-D (grey), followed by Th-T fluorescence emission. (C) Light-scattering measurements at 300 nm in the absence (black) and presence of 100 µM of compounds 5 (red), 6 (green), 7 (violet), 8 (orange), 9 (blue), 10 (brown) and 11 (yellow). (D to G) Representative TEM images of untreated (D) and 5 (E), 8 (F), 9 (G) and 11 (H) treated samples. Th-T fluorescence is plotted as normalised means. Final points were obtained at 48 h. Error bars are represented as SE of mean values; ** p < 0.01 and *** p < 0.001.
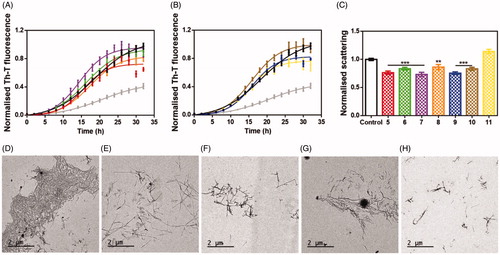
3.4. Docking studies
In order to ascertain the binding mode of pyridinyl-triazole derivatives 3, 5, 8, 9 and 11 that demonstrated the ability to reduce α-syn aggregation in vitro, we carried out molecular docking simulation by means of Autodock 4.2 suite;Citation29 the computational studies were based on NMR structure of α-syn (PDB code 2N0A) as structural coordinatesCitation30.
Considering that in our above reported molecular modelling studies we selected polyphenolic and non-polyphenolic inhibitors (see ) that bind the N-terminal region of α-syn, we hypothesised that also derivatives 3, 5, 8, 9 and 11 could interact with same portion of the protein. For this reason, we defined this region as the search space for the docking simulation (see Material and Methods section). The docking results confirmed that all the tested compounds bind in the same site of α-syn located between the N-terminal and the NAC domains of the protein and lined by Ala53, Val55, Thr59, Glu61, Thr72, Gly73 and Val74 ().
Figure 6. Binding site of α-syn identified through docking studies for this class of inhibitors. The image is created by PyMOL software (https://pymol.org).
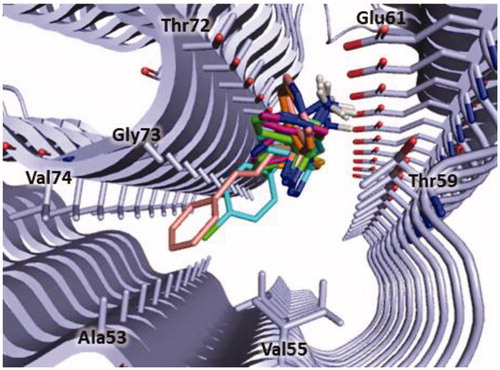
Notably, in a recent work, Pujols and co-workers identified the same portion of the protein as binding site of their inhibitor 2-hydroxy-5-nitro-6-(3-nitrophenyl)-4-(trifluoromethyl)nicotinonitrile through a induced-fit docking simulationCitation24; therefore, these evidences further supported the hypothesis that this region could represent a binding site for small molecules on α-syn fibrils.
In detail, compound 3 could bind to α-syn by establishing a salt bridge between the amino group and Glu61 and π-anionic interaction between the pyridine ring and Glu61B. The cinnamyl moiety is oriented towards Ala53, Gly73 and Val74 and might engage hydrophobic interaction with Ala53. Furthermore, van der Waals interactions with Val55, Thr59, Thr72, Gly73 and Val74 could be observed ().
Figure 7. Plausible binding mode of compound 3 (pink stick). The interacting residues of the binding site are represented as light blues stick. The image is created by PyMOL software (https://pymol.org).
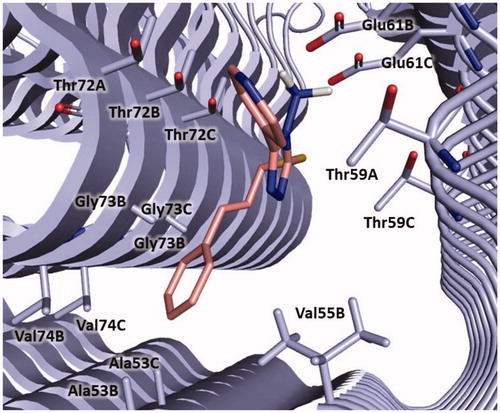
In , the binding mode of compounds 5, 8, 9 and 11 is displayed. The new derivatives showed a slight different binding orientation in comparison to the parent compound 3, probably due to the shorter and more flexible linker between the sulphur atom and the aromatic ring.
Figure 8. Plausible binding modes for compound 5 (magenta stick, panel A), 8 (orange stick, panel B), 9 (green stick, panel C) and 11 (cyan stick, panel D). The interacting residues of the binding site are represented as light blues stick. The images are created by PyMOL software (https://pymol.org).
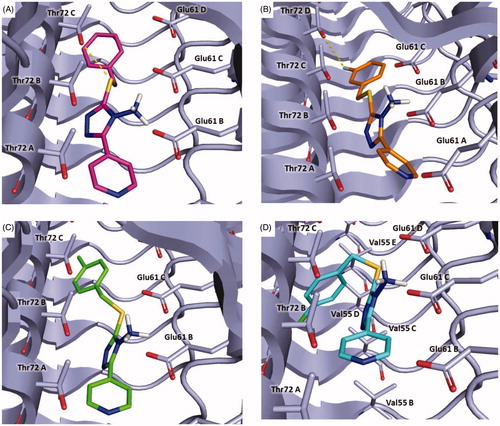
In particular, all the inhibitors could interact with α-syn by a crucial salt bridge between the amino group and the side chain of Glu61. Moreover, for inhibitors 5, 8, 9 a pi-anion interaction was observed between the pyridine ring and Glu61B.
Additionally, compound 5 might form a hydrogen bond with Thr72C through the sulphur atom and pi-sigma interaction with Thr72C (, Panel A). Concerning compound 8, it might establish a halogen bond between the fluorine atom and Thr72D (, Panel B). Instead, 9 could engage pi-sigma interaction with Thr72C (, Panel C). Finally, compound 11 might establish van der Waals interactions with Val55C (, Panel D).
4. Conclusion
The search for a cure of PD represents an important challenge in the pharmaceutical research field. The inhibition of α-syn aggregation has emerged as promising new therapeutic strategy for the treatment of PD. In this work, we described the generation of a ligand-based pharmacophore model, which was used as query to screen two chemical libraries. The hits selected from the virtual screening were tested in vitro to probe their ability to inhibit α-syn aggregation, resulting in the identification of the new hit 3. Structural modifications were carried out on compound 3 obtaining four interesting derivatives. Finally, the binding mode of this new class of inhibitors on α-syn was investigated by molecular docking simulation. All the information gained from these studies could be useful for the design of novel inhibitors α-syn aggregation inhibitors bearing a pyridinyl-triazole scaffold.
Disclosure statement
The authors declare that there is no conflict of interest.
Additional information
Funding
References
- Chakraborty A, Brauer S, Diwan A. Possible therapies of Parkinson’s disease: a review. J Clin Neurosci 2020;75:1–4.
- Stoker TB, Torsney KM, Barker RA. Emerging treatment approaches for Parkinson’s disease. Front Neurosci 2018;12:693.
- Kalia LV, Lang AE. Parkinson’s disease. Lancet 2015;386:896–912.
- Dickson DW. Parkinson’s disease and parkinsonism: neuropathology. Cold Spring Harb Perspect Med 2012;2:a009258.
- Save SS, Rachineni K, Hosur RV, Choudhary S. Natural compound safranal driven inhibition and dis-aggregation of α-synuclein fibrils. Int J Biol Macromol 2019;141:585–95.
- Savitt D, Jankovic J. Targeting α-synuclein in Parkinson’s disease: progress towards the development of disease-modifying therapeutics. Drugs 2019;79:797–810.
- Afitska K, Priss A, Yushchenko DA, Shvadchak VV. Structural optimization of inhibitors of α-synuclein fibril growth: affinity to the fibril end as a crucial factor. J Mol Biol 2020;432:967–77.
- Kyriukha YA, Afitska K, Kurochka AS, et al. α-Synuclein dimers as potent inhibitors of fibrillization. J Med Chem 2019;62:10342–51.
- Serpell LC, Berriman J, Jakes R, et al. Fiber diffraction of synthetic alpha-synuclein filaments shows amyloid-like cross-beta conformation. Proc Natl Acad Sci USA 2000;97:4897–902.
- Ma L, Yang C, Zheng J, et al. Non-polyphenolic natural inhibitors of amyloid aggregation. Eur J Med Chem 2020;192:112197
- Sangwan S, Sahay S, Murray KA, et al. Inhibition of synucleinopathic seeding by rationally designed inhibitors. Elife 2020;9:e46775.
- Haddad F, Sawalha M, Khawaja Y, et al. Dopamine and levodopa prodrugs for the treatment of Parkinson's disease. Molecules 2017;23: 40.
- Carrera I, Cacabelos R. Current drugs and potential future neuroprotective compounds for Parkinson's disease. Curr Neuropharmacol 2019;17:295–306.
- Borovac JA. Side effects of a dopamine agonist therapy for Parkinson's disease: a mini-review of clinical pharmacology. Yale J Biol Med 2016;89:37–47.
- O'Hara DM, Kalia SK, Kalia LV. Emerging disease-modifying strategies targeting α-synuclein for the treatment of Parkinson's disease. Br J Pharmacol 2018;175:3080–9.
- Peña-Díaz S, Pujols J, Conde-Giménez M, et al. ZPD-2, a small compound that inhibits α-synuclein amyloid aggregation and its seeded polymerization. Front Mol Neurosci 2019;12: 306.
- Singh SK, Dutta A, Modi G. α-Synuclein aggregation modulation: an emerging approach for the treatment of Parkinson's disease. Future Med Chem 2017;9:1039–53.
- Shihabuddin LS, Brundin P, Greenamyre JT, et al. New frontiers in Parkinson’s disease: from genetics to the clinic. J Neurosci 2018;38:9375–82.
- Teil M, Arotcarena ML, Faggiani E, et al. Targeting α-synuclein for PD therapeutics: a pursuit on all fronts. Biomolecules 2020;10:391.
- Wolber G, Langer T. LigandScout: 3-D pharmacophores derived from protein-bound ligands and their use as virtual screening filters. J Chem Inf Model 2005;45:160–9.
- Oliveri V. Toward the discovery and development of effective modulators of α-synuclein amyloid aggregation. Eur J Med Chem 2019;167:10–36.
- Kolodina AA, Gaponenko NI, Lesin AV. Synthesis and opening of the thiadiazine ring in 6,7-dihydro-5H-[1,2,4]triazolo[3,4-b][1,3,4]thiadiazines. Russ Chem B. 2008;57:1273–6.
- Pujols J, Peña-Díaz S, Conde-Giménez M, et al. High-throughput screening methodology to identify alpha-synuclein aggregation inhibitors. Int J Mol Sci 2017;18: 478.
- Pujols J, Peña-Díaz S, Lázaro DF, et al. Small molecule inhibits α-synuclein aggregation, disrupts amyloid fibrils, and prevents degeneration of dopaminergic neurons. Proc Natl Acad Sci USA 2018;115:10481–6.
- Crespo R, Villar-Alvarez E, Taboada P, et al. What can the kinetics of amyloid fibril formation tell about off-pathway aggregation? J Biol Chem 2016;291:2018–32.
- Pedretti A, Villa L, Vistoli G. VEGA - an open platform to develop chemo-bio-informatics applications, using plug-in architecture and script programming. J Comput Aid Mol Des 2004;18:167–73.
- Dassault Systèmes BIOVIA. BIOVIA Discovery Studio Visualizer, v20. San Diego: Dassault Systèmes; 2020.
- Gitto R, De Luca L, Ferro S, et al. Synthesis, modelling and biological characterization of 3-substituted-1H-indoles as ligands of GluN2B-containing N-methyl-d-aspartate receptors. Bioorg Med Chem 2014;22:1040–8.
- Morris GM, Huey R, Lindstrom W, et al. AutoDock4 and AutoDockTools4: automated docking with selective receptor flexibility. J Comput Chem 2009;30:2785–91.
- Tuttle MD, Comellas G, Nieuwkoop AJ, et al. Solid-state NMR structure of a pathogenic fibril of full-length human α-synuclein. Nat Struct Mol Biol 2016;23:409–15.