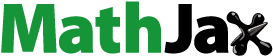
Abstract
Antimicrobial resistance (AMR) is a pressing global issue exacerbated by the abuse of antibiotics and the formation of bacterial biofilms, which cause up to 80% of human bacterial infections. This study presents a computational strategy to address AMR by developing three novel quantitative structure–activity relationship (QSAR) models based on molecular topology to identify potential anti-biofilm and antibacterial agents. The models aim to determine the chemo-topological pattern of Gram (+) antibacterial, Gram (−) antibacterial, and biofilm formation inhibition activity. The models were applied to the virtual screening of a commercial chemical database, resulting in the selection of 58 compounds. Subsequent in vitro assays showed that three of these compounds exhibited the most promising antibacterial activity, with potential applications in enhancing food and medical device safety.
Introduction
The emergence of bacterial resistance to antibiotics has become a significant concern in public health, resulting in diminished efficacy of numerous antibiotics and posing challenges in the treatment of bacterial infections. A prominent contributing factor to this resistance phenomenon is the development of biofilms. Biofilms denote communities of bacteria that adhere to surfaces and generate a protective extracellular matrix composed of polymeric substances. This matrix acts as a physical barrier that impedes the penetration of antibiotics, rendering the bacteria residing within the biofilm less susceptible to treatment. Furthermore, the bacteria residing within biofilms often enter a dormant state, further reducing their vulnerability to antibiotic action. The capacity of bacteria to form biofilms is particularly problematic in clinical environments, such as hospitals, where surfaces can become contaminated with bacteria, giving rise to biofilm formation on medical devices such as catheters and implants. These biofilms serve as reservoirs for antibiotic-resistant bacteria, posing challenges in eradicating infections and elevating the risk of transmission to other patients.Citation1
Bacterial pneumonia, the most common infectious cause of mortality worldwide, arises from various kinds of microorganismCitation2. One of these agents is the Gram (−) and opportunistic pathogen, Klebsiella pneumoniae. This infectious agent has been observed as a cause of hospital-acquired pathogens and asymptomatically colonises mucosal surfaces of both the gastrointestinal and the upper respiratory tract becoming one the main sources of bloodstream infections worldwide.Citation3,Citation4 This bacterium is resistant to common antibiotics such as amoxicillin or carbenicillin and has developed multidrug resistance determinants like aminoglycosides, cephalosporins, or carbapenems.Citation5 One of the virulence factors of K. pneumoniae is its ability to form biofilms.Citation6 Biofilms provide a protective environment for bacteria, shielding them from the immune system and making it more difficult for antibiotics to reach and kill the bacteria.
Another pathogen that crowned this list is the Gram (+) human commensal Staphylococcus aureus on the human skin and mucous surfaces. They are recognised as one of the main causes of infection in any medical device that penetrates those surfaces due to their capacity to be associated with resistant biofilms. Thus, S. aureus has non-specific mechanisms of antibiotic resistance where the biofilm formation is the most important along with genetic factors Citation7. The so-called Methicillin-resistant S. aureus (MRSA) has now emerged as a widespread example of community infection that can spread rapidly among healthy individuals; consequently, it has become an epidemic in the United States.Citation8 Inside the Staphylococcal genre, more species are leading skin or mucous membrane infections. Staphylococcus epidermidis causes infection in immune-compromised patients after penetration of the skin due to trauma, inoculation, or implantation of surface-colonised medical devices with a biofilm of this bacteria. In fact, it has been demonstrated the higher antibiotic tolerance of cells within biofilm hence it is considered the most important factor involved in the pathogenesis of S. epidermidis.Citation9
In addition to the clinical contamination, more pathogens make up a major problem in food safety for contaminated fresh and stored products. Food poisoning outbreaks commonly occur through the consumption of raw vegetables and fruits or processed food where Bacillus cereus, a natural inhabitant of soils, is present.Citation10 Depending on the toxins produced by the strain, food poisoning caused by B. cereus is classified into two main categories, emetic and diarrhoeic.Citation11 The persistence of B. cereus in both processed and ready-to-eat food respond to several factors including the highly adhesive and resistant to stress spores but also it is attributed to the ability to form biofilms. In fact, more than 80% of microbial infections worldwide involve biofilms according to a study of the US National Institutes of Health.Citation12 Bacterial biofilm formation is a significant issue also for the food business.
In bacterial surface colonisation, bacterial diffusion across the surface, and biofilm development, is crucial. To perform a biofilm, bacteria tend to aggregate on surfaces forming ordered communities embedded in a secreted extracellular matrix made up of a variety of molecules including exopolysaccharides and amyloid proteins among others.Citation13 A paradigm for biofilm studies is the soil-dwelling bacterium Bacillus subtilis. The extracellular matrix of this microorganism has been well studied and it is mainly composed of an exopolysaccharide and the amyloid-like protein TasA along with the auxiliary protein TapA.Citation14 Similar to other amyloid proteins, TasA promotes a self-aggregation into insoluble and resistant to degradation fibres based on the enriched β-sheet structure. These features play a key role in the physical and biological function of the Bacillus biofilm which is a contrast to the traditionally pathological point of view associated with the amyloid proteins.Citation15
Food preservation, which is crucial for our survival, is another issue connected to bacterial biofilm. Any alteration in a food product that makes it unpalatable to the consumer from a sensory perspective is referred to as spoilage. The development of off-flavours and off-odours from microbial growth and metabolism in the product is a consequence of spoiling. The most significant bacteria degrading ready-to-eat vegetable products include several Gram (−) species such as Pseudomonas or Erwinia, which are known as specific spoilage organism (SSO). Spoiling potential is measured by its capacity to create the metabolites associated with the degradation of a particular food in a pure culture. In general, numerous isolated organisms from a food product will be able to reproduce endlessly if given the chance.Citation16
The use and abuse of antibacterial compounds both in pharmaceutical and food industry have resulted in the development of antimicrobial resistance (AMR) as a global concern and it has turned into a priority target for the scientific community in the last decades. They can quickly develop multidrug-resistant (MDR) strains and owing to the reduced effectiveness of the therapy alternatives, the mortality rate in patients is increased.Citation2 Under this scenario, the success of the discovery and development of new antimicrobial compounds plays a leading role as the key to battle against the AMR.
Despite being a complex and interdisciplinary process, remarkable advances have been made in drug design and discovery. Among the most relevant strategies are high-throughput screening (HTS), fragment-based drug design, structure-based drug design, and ligand-based drug design.Citation17–20 The latter two methods represent the backbone of computer-aided drug design (CADD) and have completely revolutionised and sped up the entire process of drug discovery and development over the last few decades.Citation21–23 In this study, quantitative structure–activity relationship (QSAR) based on molecular topology has been used to develop a ligand-based algorithm for the identification of new antimicrobial agents with anti-biofilm activity. The reliability of this methodology in the antimicrobial field is established, with several noteworthy results reported in the literature.Citation24–27 Thanks to molecular topology, it is possible to develop QSAR predictive models that rely on a pure chemo-mathematical interpretation of the molecules and their relationship with a specific physicochemical or biological activity.Citation28 Graph theory is used to express chemical structures by means of numbers, making it possible to calculate ad hoc topological descriptors based on molecular connectivity features. Instead of relying solely on physicochemical descriptors, topological and topo-chemical indices allow for a more abstract and neutral interpretation of the relationship between a chemical structure and a biological property, making this method unique among other QSAR techniques.Citation29 These graph-based descriptors can be used to develop predictive algorithms by selecting the most significant ones to establish the predictive relationship under study. In this work, the linear discriminant analysis (LDA) technique has been used to develop three mathematical predictive models based on topological descriptors. The three algorithms aim to determine the chemo-topological pattern of Gram (+) antibacterial, Gram (−) antibacterial, and biofilm formation inhibition activity for the identification, through virtual screening methodologies, of new potential antimicrobial agents as biofilm formation inhibitors.
Material and methods
QSAR strategy and computational section
reports the step-by-step computational strategy established for the rational design of new molecular entities with antimicrobial and anti-biofilm activity against different Gram (+) and Gram (−) bacteria.
Figure 1. Graphic representation of the QSAR strategy workflow for the identification of new compounds with potential antibacterial and anti-biofilm activity.
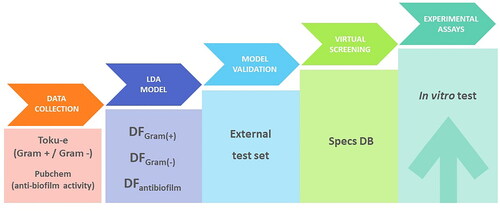
The first step in this study was the creation of a database. Next, predictive models based on linear discriminant algorithms were developed. Once the models were validated, a virtual screening of a commercial database was carried out to search for potential candidates. Finally, the most promising compounds were tested through specific experimental assays.
Database creation
To generate the first two discriminant models, we created a database of compounds with strong antimicrobial activity against different Gram (+) bacteria able to assemble biofilms, such as B. subtilis, B. cereus, S. epidermidis, S. aureus, Enterobacter cloacae, as well as against Gram (−) bacteria such as Erwinia carotovora and K. pneumoniae. To do so, the Toku-e database was employed (http://antibiotics.toku-e.com/m/), and compounds with a range of antimicrobial activity up to 100 μg/mL were labelled as active antimicrobial agents, and compounds with reported antimicrobial activity above 100 μg/mL were labelled as inactive antimicrobial agents. A comprehensive database of 190 compounds with antimicrobial activity against the different strains of bacteria under study was collected. The created database was contrasted in PubchemCitation30 for previously reported assays on anti-biofilm activity. The molecules with reported anti-biofilm activity, regardless of the bacterial species, were selected as the active set for training the third linear discriminant model aimed at determining the chemo-mathematical pattern associated with anti-biofilm activity. The rest of the molecules, with no previously reported anti-biofilm activity, were used as the inactive set.
Molecular descriptors calculation
To develop the current QSAR strategy based on molecular topology, we used graph theory to establish a mathematical relationship between chemical structure and biological activity. We employed topological and topo-chemical descriptors (graph-based descriptors) to develop QSAR predictive equations. To begin, we drew the 2D structures of the molecules using ChemDraw Ultra (version 19.1)Citation31 and calculated the indexes using Alvadesc software.Citation32
Statistical methodology
LDA uses pattern recognition to determine the best linear combination of variables in a specific context, such as topological and topo-chemical descriptors, to qualitatively discriminate between two categories – active and inactive molecules – and allow for their classification.Citation33 In our study, we separated compounds into active or inactive groups based on their antimicrobial and anti-biofilm formation inhibitory activity. The Fischer–Snedecor parameter (F) was used to select variables following a stepwise strategy. The Wilks’ parameter λ was the main classification indicator that determined the discriminant capability of the model. All of these parameters were calculated using the software STATISTICA version 9.Citation34
Biological distribution diagram (BDD)
A biological distribution diagram (BDD) provides a simple and clear interpretation of the distribution of cases during linear discriminant classification. Moreover, they allow for an intuitive way to establish the range of activity and applicability of the models’ domains. In brief, a BDD is a plot of the expectancy of activity versus the numerical outputs of the discriminant function (DF) for a particular biological activity, specifically antimicrobial and anti-biofilm formation inhibitory activity in this context.Citation35 Expectancy of activity is defined as Ea = a/(i + 1), where “a” and “i” are, respectively, the number of active and inactive compounds in a particular interval of DF values. Similarly, we can define Ei or expectancy of inactivity as Ei = i/(a + 1). The use of such diagrams eases the visualisation of DF intervals where there is a maximum probability of activity or inactivity.
Experimental section
Reagents
Compounds used for antimicrobial test were purchased from Molport (Riga, Latvia) (compounds 1–30), Sigma-Aldrich, St. Louis, MO (compounds 31–37), Specs (Zoetermeer, The Netherlands) (compounds 38–55), Mcule (Budapest, Hungary) (compound 56), and Asinex (Moscow, Russia) (compounds 57–58). A stock solution of the molecules was prepared in DMSO at a concentration of 0.2 M and diluted in distilled water to reach a final concentration of 100 and 1000 μM when added to the cultures.
Growth conditions
Luria broth (LB) comprised 1% tryptone (Difco), 0,5% yeast extract (Difco), and 1% NaCl. MSgg broth comprised 100 mM morpholinepropane sulphonic acid (pH 7), 0.5% glycerol, 0.5% sodium glutamate, 5 mM potassium phosphate (pH 7), 50 μg/mL tryptophan, 50 μg/mL phenylalanine, 2 mM MgCl2, 700 μM CaCl2, 50 μM FeCl3, 50 μM MnCl2, 2 μM thiamine, and 1 μM ZnCl2. Ty broth comprised 1% tryptone, 0.5% yeast extract, 0.5% NCl, 10 mM MgSO4 and 1 mM MnSO4. Trypticase Soy Broth (TBS) comprised 1.7% tryptone (Difco), 0.3% soytone (Difco), 0.5% NaCl, 0.25% KH2PO4, 0.25% glucose.
Strains
The following Gram (+) bacteria were used in this study: B. subitlis NCBI3610, B. cereus ATCC14579, B. cereus AHS187, B. cereus UMAF8564, B. cereus DSM4384, B. cereus 6A45, B. cereus AHS8438, S. aureus 1371, S. epidermidis KZ6372, and E. cloacae 1374. Additionally, two Gram (−) strains were included in the study: E. carotovora CECT225, K. pneumoniae KZ1373. Bacteria were cultured at 37 °C on solid LB medium (1% tryptone, 0.5% yeast extract, 1% NaCl, and 1.5% agar).
Antibacterial activity
For the screen, library compounds were added to 48-well plates into 500 µL of culture medium, followed by addition of 5 µL of a suspension of bacteria in PBS to a final OD600 of 0.1–0.2. The final concentration of each compound was 100 or 1000 µM. Plates were incubated without agitation at 30 °C for 72 h in order to test bacterial growth.
Different species were cultured as follows: B. subtilis was grown in MSgg medium, B. cereus species were grown in Ty, and the rest of species were grown in TSB medium. An additional well with DMSO was used as control.
Biofilm quantification with crystal violet
For those strains forming strips over the wall of the well simulating a ring-shape, a protocol of staining with crystal violet (CV) was developed. To do that, the wells were washed three times with 500 µL of distilled water after the medium was withdrawn. Then, the strips were air dried for 1 h and the adherent cells were stained with 500 µL of a CV solution (0.1% w:v). The excess of CV was removed after 45 min and the wells were washed three times with distilled water (500 µL). To quantify biofilm on any sample, 500 µl of a 33% glacial acetic acid solution was added for 30 min to elute the CV dye attached to the biofilm. Finally, the absorbance of the resulting solution was measured at 540 nm using a microplate reader (BMG FLUOstar Omega) transferring 200 µL to a 96-well plate.
Minimum inhibitory concentration (MIC)
To determine the minimal inhibitory concentration (MIC) of library compounds microtiter plate assay was made. The bacterial suspension was adjusted to a concentration of 1.0 × 105 cfu/mL. Compounds to be investigated were dissolved in broth LB medium (100 µL) with bacterial inoculum (1.0 × 104 cfu per well) to achieve the starting concentration (1500 µM) an serially diluted (1:2 dilutions) then inoculated with B. subtilis. Plates were incubated for 24 h at 30 °C without agitation.
Growth curve
A growth curve of each strain was carried out on 48-well plates with the following broth media: B. subtilis was grown in MSgg medium, B. cereus species were grown in Ty, and the rest of species were grown in TSB medium. An additional growth curve with DMSO was used as control. An aliquot (5 µL) of an overnight culture was added to each well and the final concentration of each compound was adjusted to 100 and 1000 µM. The absorbance was measure in a spectrophotometer (FLUORstar ® Omega, BMG Labtech) absorbance plate reader set up at 600, at 30 °C and shaking for a total of 24 h.
Results
QSAR models and predictive classification results
We developed three QSAR algorithms based on LDA to create a computational strategy for easy and accessible identification of potential biofilm formation inhibitors through virtual screening. reports the three DFs along with their respective statistical parameters.
Table 1. Models predicting antibacterial and antibiofilm activity and statistical parameters.
The first model focuses on determining the chemo-mathematical pattern of compounds with potent activity against S. aureus, S. epidermis, B. cereus, B. subtillis, and E. cloacae (all Gram (+) bacteria). The second predictive model, focuses on the identification of the chemo-mathematical pattern related to anti-Gram (−) activity against E. carotovora and K. pneumoniae and the last DF, aims to identify the chemo-mathematical pattern related to the inhibitory activity on biofilm formation for the bacteria species under study (Gram (+): S. aureus, S. epidermis, B. cereus, B. subtillis, and E. cloacae; and Gram (−): E. carotovora and K. pneumoniae. Overall, the discriminant models gave good predictive classification, as reported in .
Table 2. Classification matrix for the training and test set of the three discriminant functions.
For the first discriminant model (DFGram (+)), comparable sensitivity and specificity values were obtained, as shown in , for both active (79%) and inactive compounds (78%). In terms of external validation, when using a set of compounds with reported anti-Gram (+) activity not used for training the model, 75% of the inactive group was correctly classified by the algorithm whereas 62% of the active compounds. This indicates a higher level of specificity than sensitivity, indicating a greater ability to identify false positives compared to false negatives.
The second model (DFGram (−)), reports almost the same sensitivity and specificity for the discriminant equation, reaching a correct classification percentage of at least 90% for both groups (). However, during external validation of the model, a higher sensitivity was observed in classifying anti-Gram (−) compounds.
The third discriminant model (DFantibiofilm), is more specific (80% of correct classification for inactive group) than sensitive (72% of correct classification for active group) and this trend is repeated when the model is subjected to external validation. Therefore, the model has a greater capacity to detect false positives.
Biological distribution diagrams and activity range
To make the interpretation of the classification results easier and establish the activity range for the three QSAR models, a graphic representation of the distribution of the active and inactive population among the analysed data is depicted, thanks to the BDD. In , the active population is represented by blue histograms, while the inactive one is represented by orange histograms.
Figure 2. Biological distribution diagram for a) antibacterial Gram (+) activity, b) antibacterial Gram () activity, c) biofilm inhibitory activity. Blue histograms represent active compounds, while orange histograms represent the inactive compounds.
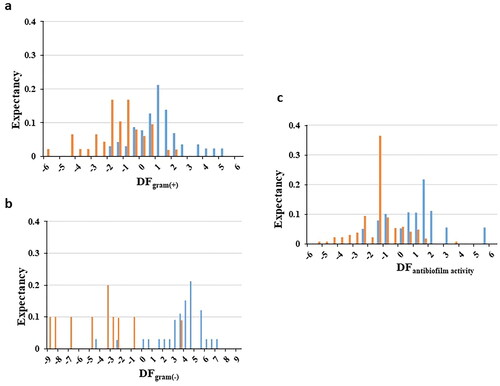
For the first DF (DFGram (+)), the distribution of potential strong antibacterial activity compounds against Gram (+) is illustrated against low activity molecules (see ). A zone of overlapping between DF values of −0.5 and 1 is found, whereas a higher frequency in the distribution of compounds with anti-Gram (+) activity is detected for DF values ≥ 1. Therefore, taking this value as a reference, the presence of false positives should be negligible.
Regarding the second model (DFGram(-)), the analysis of the distribution diagram () reports a clear differentiation in terms of distribution for compounds with high and low Gram negative antibacterial activity; establishing an interval of high probability to find active candidates above 0.
Finally, for the last function (DFantibiofilm), the analysis of the distribution reveals a pattern where active compounds tend to have positive values of the DF, although there is an overlapping zone between −1 and 0.5. Therefore, based on the BDD analysis, molecules with DF values greater than 0.5 are considered to have significant potential for inhibiting biofilm formation.
Key chemo-mathematical features regarding antibacterial and anti-biofilm activity
In this section, we discuss the key chemo-mathematical features highlighted by the topological and topo-chemical descriptors used in the three algorithms developed to predict Gram (±) and biofilm formation inhibitory activity.
In the first model, the following descriptors were present: SpDiam_X (spectral diameter from chi matrix), GATS2e (Geary autocorrelation of lag 2 weighted by Sanderson electronegativity), Chi1_EA(dm) (connectivity-like index of order 1 from edge adjacency matrix, weighted by dipole moment), Eig14_EA(dm) (eigenvalue n0.14 from edge adjacency matrix weighted by dipole moment), nCp (number of terminal primary C(sp3)) – an index related to functional group counts, and SsCH3 (Sum of sCH3 E-states) – an Atom-type E-state index. Among these indices, Chi1_EA(dm) and nCp have a direct relationship with antibiotic activity against Gram (+) bacteria. Chi1_EA(dm) is related to the degree of branching of a molecule. The descriptor’s value increases with the number of bonds at topological distance 1 and the presence of atoms with a greater molecular dipole (as shown in .
Figure 3. Example of Chi1_EA(dm) and nCp descriptor values for different anti-Gram (+) compounds (training set).
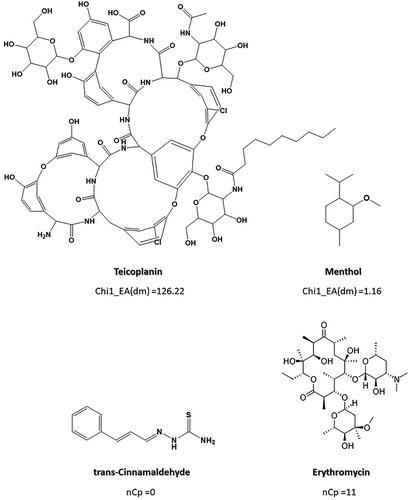
The other descriptor directly related to anti-Gram (+) activity is the nCp descriptor, which takes into account the number of terminal primary sp3 carbons (C) present in the molecule. As can be seen in , the higher the number of terminal sp3 carbons, the higher the value of this index. Trans-Cinnamaldehyde, without C(sp3), adopts a null value for the descriptor, while Erythromycin, with 11 terminal carbons of sp3 hybridisation in its structure, adopts a value of 11. Therefore, according to our model molecules with a higher number of terminal carbons (sp3) and a higher level of branches may have a higher probability of exhibit some antimicrobial effect against Gram (+) bacteria. However, other factors, such as the presence of less electronegative atoms, which therefore contribute to a lower dipole moment, also seem to influence the antimicrobial activity, according to the other descriptors present in the model.
Regarding the second model, 2D autocorrelation-type descriptors (MATS7e, Moran autocorrelation of lag 7 weighted by Sanderson electronegativity), functional group counts indexes (nCt, number of total tertiary C(sp3)), and atom-type E-state indices (SaaaC, Sum of aaaC E-states) are present. All the indices are indirectly related to anti-Gram (−) activity, so compounds giving low values for these descriptors will have a higher probability of developing potential antibacterial activity. MATS7e descriptor, considers the presence of atoms with a lower electronegativity at topological distance 7 in the molecules. MATS7e descriptor is a measure of the similarity in electronegativity between neighbouring atoms at topological distance 7. As can be seen in , both strong and weak anti-Gram (−) activity compounds adopt negative values for this index. Therefore, although MATS7e contributes to the overall chemo-mathematical pattern of anti-Gram (−) activity, it is not significant enough per se to explain it, which is also in accordance with its contribution to the model’s discriminant capability, with the smallest F value among the variables (F = 8.9).
Figure 4. Example of MATS7e, SaaaC and nCt values for different active and inactive compounds from the training set (Gram () activity).
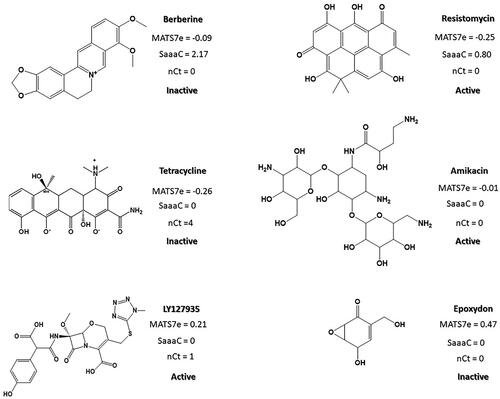
The second most significant descriptor, SaaaC, with an F value of 18.8, is related to the total number of carbon atoms connected to a carbon atom which makes three aromatic bonds, and it represents the molecular reactivity of such atom types (the higher their reactivity and number, the higher the SaaaC value). It can be noticed how compounds with fused aromatic rings surrounded by non-highly electronegative atoms, such as Berberine, adopt higher values for this descriptor (. However, molecules lacking fused aromatic rings, such as Tetracycline and Amikacin, present null values for the SaaaC descriptor.
Finally, nCt, the index that contributes most significantly to the model with an F value of 31.5, contemplates the presence of tertiary carbons with sp3 hybridisation in the molecular structure. A lower presence of tertiary carbons with sp3 hybridisation can be observed in the active group, which is related to noticeable antimicrobial activity against Gram (−) bacteria (Table S3), although some exceptions are found in the inactive group, as exemplified by Berberine (. Taking into consideration all this information, a chemo-mathematical pattern for molecules with activity against Gram (−) bacteria can be deduced: absence of fused aromatic rings and absence of tertiary carbons with sp3 hybridisation in their chemical structure.
The third model is made up of constitutional-type descriptors (N%, percentage of N atoms), walk and path count indexes (SRW05, self-returning walk count of order 5), and P_VSA-like descriptors (P_VSA_MR_8, P_VSA-like on Molar Refractivity, bin 8). The only index that directly relates to the ability to inhibit biofilm formation in the bacteria under study (Gram (+) and/or (−)) is N%. In fact, it can be seen that 83% of the molecules with anti-biofilm capacity show the presence of N in their chemical structure, compared to the 49% of the molecules without anti-biofilm capacity. However, it is not the only factor that determines the inhibitory activity of biofilm formation, given that it can be found in half of the inactive ones (.
Figure 5. Example of N%, SRW05 and P_VSA_MR_08 descriptors values for molecules with (active) and without (inactive) biofilm inhibition activity.
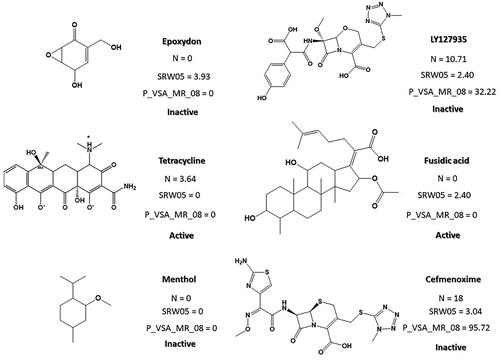
SRW05 is a type of molecular walk count descriptor that counts the number of walks of length 5 that start and end at the same vertex. This means that it takes into account the number of five-membered rings in the chemical structure. Compounds that lack 5-membered rings, such as menthol, have a null value for this descriptor. Given that this descriptor has a negative impact on inhibitory biofilm activity, it is not surprising that there is a greater presence of molecules without 5-membered cycles in the group of active molecules. P_VSA_MR_8, on the other hand, is a VSA-type descriptor that corresponds to a subdivision of the molecular surface area. The surface area of an atom in a molecule is understood as the amount of surface area of that atom not contained in any other atom of the molecule. Each VSA-type descriptor can be characterised as the amount of surface area with Molecular Refractivity (MR) greater than 0.56. Therefore, molecules with lower MR (considered as the sum of either atom or bond refractivity) than 0.56 will have a null value (see Menthol and Tetracycline in ), while compounds with MR > 0.56 surface area of each atom will have a non-zero value for this descriptor (e.g. Cefmenoxime in .
Virtual screening of a commercial database: selection of antimicrobial and anti-biofilm agents
The Specs database was screened for molecules capable of exerting an antibacterial and/or inhibiting biofilm formation effect for the bacteria studied (Gram (+): S. aureus, S. epidermis, B. cereus, B. subtilis, and E. cloacae and Gram (−): E. carotovora and K. pneumoniae). The basic requirement for a molecule to be classified as active by the models was to possess a DF value greater than 0. Following these criteria, 58 compounds were selected for in vitro testing to validate their antimicrobial and/or anti-biofilm activity. The final selection was also conditioned by other factors such as the price and commercial availability of the compounds. Some compounds showed a chemo-mathematical pattern compatible with antibacterial activity against Gram (+), Gram (−), and/or anti-biofilm formation activity (see Tables S7 and S8).
Screening for new antimicrobial molecules
A method for a rapid screening of antimicrobial compounds into the resulting list of molecules with potential activity can be achieved by a simple test under biofilm formation growth conditions in standing liquid cultures. Compared to the planktonic cells, bacteria forming biofilm display a higher resistance to antibiotic treatment thus it is the predominant behaviour of most bacterial species.Citation36 In vitro biofilms from several bacteria are characterised either by wrinkly pellicle such as B. subtilis or a sticked ring in the wall like B. cereus. A list of both Gram (+) and Gram (−) biofilm-forming bacteria was chosen for this aim. The complete list of microorganisms and biofilm-forming conditions used in this study is summarised in .
Table 3. List of biofilm-forming bacteria.
The potential bactericidal effect of selected drugs on the biofilm formation capacity of these strains was tested within the 100–1000 µM concentration range in a total volume of 500 µL employing 48-well plates.
Initially, molecules stocks were prepared by dissolution at dimethyl sulfoxide (DMSO), thus this solvent was used as control to discharge any activity related to the solvent. The corresponding culture media for each strain with molecule at selected concentration (100 and 1000 µM) was inoculated with an aliquot of a bacterial pre-culture and consequently incubated under conditions specified in . The experiments were performed in triplicates for both the strain and drug concentration and the lack of turbidity was used as a key distinguishable feature to confirm a positive activity against the strain. A classification of two main groups of biofilm can be established according to the shape and the arrangement of the biofilm cells. First, a typical pellicle covering the whole well over the standing liquid culture exemplified by the widely studied bacterial species B. subtilis. For those samples, a lack of turbidity can be considered a reason for suspecting that the compound has some kind of activity against bacteria. On the other hand, some species displayed a wall-attached biofilm formed by a ring of cells and extracellular matrix. Several strains of B. cereus are good example of this behaviour and a staining assay with CV is required to confirm the presence or absence of biofilm. summarised the positive test within any condition (strain and molecule concentration).
Table 4. Biofilm assay with selected strains against molecules with inhibition of bacterial growth at different concentrations.
A total of 12 compounds from the resulting list of potential molecules with inhibitory activity were confirmed to arrest bacterial growth on biofilm conditions according to . More significant inhibitory properties were detected for compounds 23, 36, and 42. They also showed the widest range of antimicrobial activity, including both Gram (−) and Gram (+) bacteria. Finally, compound 15 demonstrated the highest specificity among all the tested molecules, making it a promising candidate for a new antimicrobial agent against the B. cereus group.
Table 5. Potential biofilm formation inhibitors according to specific experimental in vitro assays against different strains of Gram (+) and (−).
Additionally, a quantification assay with CV was performed for those strains with adherent biofilm forming ring of cells to the wall. Three strains (B. cereus 14579, E. carotovora CECT225 and K. pneumoniae 1373) showed a substantial decrease in the measurement of the absorbance at 540 nm after treating the stained biofilm with a solution of 33% acetic acid glacial upon treatment with some of the selected molecules (.
Figure 6. Biofilm formation by B. cereus 14579, E. carotovora CECT225 and K. pneumoniae 1373 under the effect of active molecules. A control containing DMSO was used to test the effect of the solvent. Each sample was performed in triplicates.
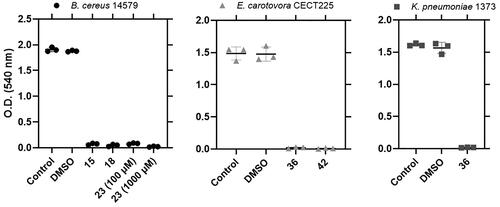
All these compounds (15, 18, 23, 36, and 42) were classified by the QSAR topological models as potential antibacterial molecules under biofilm inducing conditions, presenting DF value greater than 0.5 (Tables S7 and S8), thus confirming as predicted by the biofilm’s BDD, that the highest expectancy to find anti-biofilm activity occurs for compounds with DF values > 0.5 ().
Most of these active compounds shared one or more aromatic moiety such as the fluoro-phenyl group which has been attributed to a good antagonistic functionalityCitation37 (. In fact, analogous compounds have been published in bibliography with acceptable antimicrobial features. For example, good results against E. coli and S. aureus has been reported for 2-benzylsulfanyl-3–(4-fluoro-phenyl)-6-methyl-3H-quinazolin-4-oneCitation38 or N1-aral-N4-(3-chloro-4-fluoro-phenyl)thiosemi-carbazones.Citation39
Figure 7. Compounds identified as bacterial inhibitors in the biofilm assay and their minimal inhibitory concentration (MIC) values on B. subtilis. Structural similarity is remarked with the different aromatic moieties. Molecules 36 and 42 also showed activity against the Gram () strains E. carotovora and K. pneumoniae.
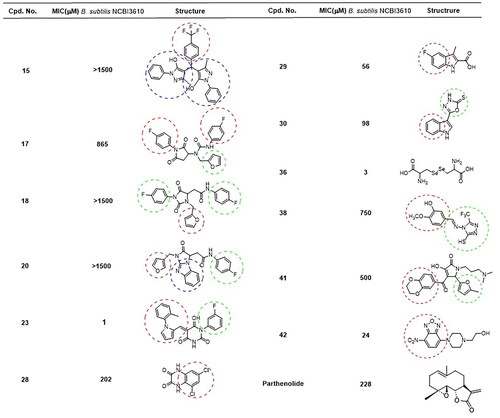
Two of the molecules (36 and 42) selected from the screening showed significant inhibitory activity against Gram (−) bacteria. They are also two of the most potent compounds in the whole study, but their molecular structure considerably differs.
On the other hand, two compounds (15 and 23) were specifically actives against B. cereus species. In such cases, both structures exhibited a comparable arrangement composed of a heterocycle core with several lateral aromatic groups. Most of the selected compounds were confirmed to inhibit biofilm formation of B. subtilis, even at the less concentration used (100 µM). MIC values suggested some molecules to have remarkable anti-biofilm activity against B. subtilis (23, 36, and 42). Parthenolide, a sesquiterpene lactone, was previously confirmed as a good inhibitor of B. subtilis biofilm.Citation40 The MIC value for Parthenolide, compared to the selected anti-biofilm molecules, confirmed the larger strength of some molecules used in this study ( and ).
The exact mechanism by which these chemical groups inhibit bacterial biofilm formation may vary depending on the specific compound and bacterial species involved. However, there are several possible ways in which these molecules could interfere with biofilm formation. i) Disruption of quorum sensing, the signalling machinery used by many bacteria species to coordinate their behaviour, including biofilm formation. Quorum sensing involves the production and detection of small signalling molecules called autoinducers. Pyrrol and pyrazol groups have been shown to interfere with quorum sensing in some bacterial species, which could disrupt biofilm formation.Citation41 ii) Inhibition of enzymes involved in biofilm formation. Bacteria use various enzymes to synthesise and modify the extracellular matrix that makes up the biofilm. Some drugs containing pyrrol or pyrazol groups have been shown to inhibit these enzymes, preventing biofilm formation.Citation42 iii) Alteration of bacterial cell membrane properties. Fluorobenzene-containing compounds can interact with bacterial cell membranes, causing changes in membrane fluidity and permeability. This can make it more difficult for bacteria to form biofilms.Citation43
Overall, the specific mechanism by which these chemical groups inhibit bacterial biofilm formation is likely to be complex and multifaceted. However, these compounds are known to have a broad range of antibacterial activities and are therefore promising candidates for the development of new antimicrobial agents.
Finally, we evaluated the antimicrobial activity of the molecules by measuring the cell density at 600 nm (. Compounds were added to the medium where biofilm assays were conducted, and the cell density adjusted to an initial DO600 of 0.1. As expected, no significant growth was observed under conditions described in the biofilm assay after 24 h of incubation in the presence of the selected compounds (OD600 less than 0.5). As the molecule stocks were prepared in a solution of DMSO, this solvent was also tested to verify a lack of influence in the growth of each strain (.
Figure 8. Dynamic of bacterial growth in the presence of inhibitory molecules. Bacteria were growth at specific conditions (agitation or static), and cell density estimated by optical density at 600 nm. DMSO was also tested to confirm the lack of antimicrobial activity.
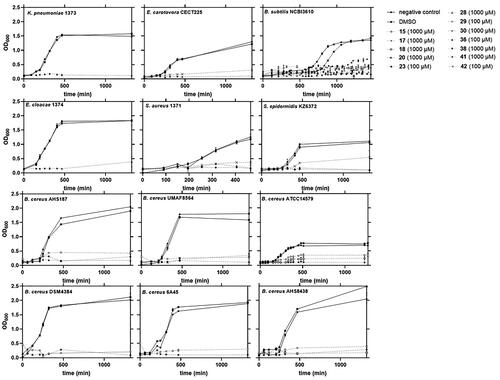
Bacterial inhibition was then confirmed by twelve of the selected molecules by molecular topology against several Gram (+) (B. subtilis, B. cereus, S. aureus, and S. epidermidis) and Gram (−) species (E. carotovora, K. pneumoniae, and E. cloacae). The most noteworthy of these were 36 (diselenide) and 42 (nitrobenzo piperazine), exposing activity versus both Gram (+) and Gram (−) bacteria. Furthermore, these molecules showed the lowest minimum inhibitory concentration (MIC) against B. subtilis. Compound 15 (trifluoro methyl bis pyrazol), however, was found to be an excellent inhibitor specifically against B. cereus strains. No antimicrobial activity was observed against B. subtilis or the rest of bacterial species included in our study. Meanwhile, the widest activity of the selected molecules corresponded to compound 23 (pyrimidine derivative). This compound was able to inhibit every Gram (+) strain and it also showed the lowest MIC value when against B. subtilis. However, Gram (−) strains were not altered by these compounds (15 and 23) or the remaining. In fact, only some positive results have been found for molecules 17, 18, 20, 28, 29, 30, 38, and 41 against B. subtilis and some strains of B. cereus. Most of these drugs showed values of MIC in B. subtilis close or even higher than 1 mM.
Potential antimicrobial agents with anti-biofilm activity
We have conducted experiments using molecules that potentially exhibit activity in inhibiting the biofilm formation of certain bacterial strains, aiming to prevent antibiotic resistance caused by biofilm formation. Remarkably, three of these molecules have demonstrated a potent effect against both Gram (+) and Gram (−) bacteria, with significantly low MIC values on B. subtilis NCBI3610. This discovery holds great value as it not only targets a wide range of bacterial types but also exerts a protective effect against biofilm formation, potentially averting future microbial resistance.
The simultaneous disruption of biofilms and direct targeting of bacteria represents a compelling strategy for addressing infections associated with biofilms. Various authors have reported that combining anti-biofilm compounds with antibiotics leads to a significant reduction in both biofilm formation and bacterial viability. For example, the combination of epigallocatechin gallate with vancomycin has been demonstrated to effectively combat S. epidermidis Citation44–45.
The implications of such findings in the field of medicine are immense, as it could potentially revolutionise the way we combat antibiotic resistance and significantly impact patient care.
Regarding the molecular structures of these compounds, our data suggest that the molecules with highly positive antimicrobial activity possess nitrogen-containing heterocyclic compounds such as pyrrol (23) or benzo-oxadiazol (42) that are known to exhibit antibacterial activity itself together with fluorobenzene (23) (). Fluorobenzene is a fluorinated aromatic compound that is often used as a substituent in drug molecules to enhance their potency and selectivity. While those that have not shown antimicrobial activity against large positive may present the presence of these groups separately.
On the other hand, compounds containing selenium in their structure (36) have demonstrated antibiofilm activity against various bacterial species. Selenium, an essential trace element, can exert antimicrobial effects by interfering with bacterial biofilm formation and stability. These compounds can inhibit bacterial adhesion to surfaces, the production of extracellular matrix, and the cell-to-cell communication necessary for biofilm formation. Furthermore, selenium has shown antioxidant and anti-inflammatory properties, which may contribute to its antibiofilm activity by modulating host response and reducing bacterial capacity to form biofilms. Selenium-based compounds represent a promising strategy in combating bacterial biofilms and could have a significant impact on the development of effective antimicrobial therapies and prevention of bacterial resistance.Citation46
Finally, the potential toxicity of the reported antibacterial and antibiofilm candidates has been assessed using the open-source web tool ADMELAB2.0 Citation47 to calculate the probability of exerting acute oral toxicity in rats (ROA). Evaluating oral acute rat toxicity is a crucial parameter in drug safety assessments. Compounds 23, 36, and 42 exhibit probabilities of 11.8%, 5.9%, and 12.8%, respectively, for oral toxicity in rats. When compared to the result obtained for the reference biofilm inhibition compound Parthenolide (considered a relatively low toxicity compound) at 45.6%, our potential compounds are anticipated to have a low probability of causing acute toxicity.
Conclusions
A computer-aided molecular design strategy based on a molecular topology QSAR approach was developed and is successfully used for the identification of 58 compounds with potential antimicrobial and/or biofilm inhibitory activity. From the experimental biological assays, three compounds (23, 36, and 42) stand as antibiofilm agents with a remarkable wide activity against Gram (+) and Gram (−) species.
Bacterial biofilms can pose a significant threat to food and clinical safety, as they can allow bacteria to persist and thrive in food and clinical environments, increasing the risk of contamination and the spread of foodborne illness. Therefore, inhibition of bacterial biofilms can be an effective strategy for avoiding clinical and food contamination.
This work represents a step forward in the development of effective strategy to design new antimicrobial agents for by-passing AMRs. The discovered compounds exhibit both anti-biofilm and antimicrobial activity against B. subtilis, B. cereus, E. carotovora, and K. pneumoniae therefore could be of interest of Food and Pharmaceutical industry.
Authors’ contributions
Maria Galvez-Llompart: Conceptualisation, Investigation, Writing – original draft, Writing – review and editing. Jesus Hierrezuelo: Investigation, Writing – original draft, Writing – review and editing. Mariluz Blasco-Santamaría: Investigation. Riccardo Zanni: Writing – original draft, Writing – review and editing. Jorge Gálvez: Writing – review and editing. Antonio de Vicente: Writing – review and editing. Alejandro Pérez: Writing – review and editing. Diego Romero: Conceptualisation, Writing – review and editing. All authors read and approved the final manuscript.
Supplemental Material
Download PDF (526.5 KB)Acknowledgement
J. Galvez acknowledges support from the MINECO (Spanish Ministry of Economy, Industry, and Competitivity) under Grant number (PID2019-107464RB-C22).
Disclosure statement
The authors report there are no competing interests to declare.
Additional information
Funding
References
- Mah TF, O’Toole GA. Mechanisms of biofilm resistance to antimicrobial agents. Trends Microbiol. 2001;9(1):1–19.
- Ferri M, Ranucci E, Romagnoli P, Giaccone V. Antimicrobial resistance: a global emerging threat to public health systems. Crit Rev Food Sci Nutr. 2017;57(13):2857–2876.
- Podschun R, Ullmann U. Klebsiella spp. as nosocomial pathogens: epidemiology, taxonomy, typing methods, and pathogenicity factors. Clin Microbiol Rev. 1998;11(4):589–603.
- Diekema DJ, Hsueh PR, Mendes RE, Pfaller MA, RolstonKV, Sader HS, Jones RN. The microbiology of bloodstream infection: 20-year trends from the SENTRY antimicrobial surveillance pro. Antimicrob Agents Chemother. 2019;63(7):e00355-19.
- Wyres KL, Hold KG. Klebsiella pneumoniae as a key trafficker of drug resistance genes from environmental to clinically important bacteria. Curr Opin Microbiol. 2018;45:131–139.
- Souza-Guerra ME, Destro G, Vieira B, Lima AS, Caldas-Ferraz LF, Hakansson AP, Darrieux M, Rojas-Converso T. Klebsiella pneumoniae biofilms and their role in disease pathogenesis. Front Cell Infect Microbiol. 2022;12:877995.
- Otto M. Staphylococcal biofilms. Curr Top Microbiol Immunol. 2016;322:207–228.
- Chipolombwe J, Török ME, Mbelle N, Nyasulu P. Methicillin-resistant Staphylococcus aureus multiple sites surveillance: a systemic review of the literature. Infect Drug Resist. 2016;9:35–42.
- Vadyvaloo V, Otto M. Molecular genetics of Staphylococcus epidermidis biofilms on indwelling medical devices. Int J Artif Organs. 2005;28(11):1069–1078.
- Bottone EJ. Bacillus cereus, a volatile human pathogen. Clin Microbiol Rev. 2010;23(2):382–398.
- Antequera-Gómez ML, Díaz-Martínez L, Guadix JA, Sánchez-Tévar AM, Sopeña-Torres S, Hierrezuelo J, Doan HK, Leveau JHJ, De Vicente A, Romero D. Sporulation is dispensable for the vegetable-associated life cycle of the human pathogen Bacillus cereus. Microb Biotechnol. 2021;14(4):1550–1565.
- Mirzaei R, Mohammadzadeh R, Alikhani MY, Moghadam MS, Karampoor S, Kazemi S, Barfipoursalar A, Yousefimashouf R. The Biofilm-associated bacterial infections unrelated to indwelling devices. Iubmb Life . 2020;72(7):1271–1285.
- Romero D, Aguilar C, Losick R, Kolter R. Amyloid fibers provide structural integrity to Bacillus subtilis biofilms. Proc Natl Acad Sci USA. 2010;107(5):2230–2234.
- Romero D, Vlamakis H, Losick R, Kolter R. Funtional analysis of the accesory protein TapA in Bacillus subtilis amyloid fiber assembly. J Bacteriol. 2014;196(8):1505–1513.
- Romero D, Kolter R. Functional amyloids in bacteria. Res Rev. 2014;17:65–73.
- Gram L, Ravn L, Rasch M, Bruhn JB, Christensen AB, Givskov M. Food spoilage-interactions between food spoilage bacteria. Int J Food Microbiol. 2002;78(1–2):79–97.
- Vamathevan J, Clark D, Czodrowski P, Dunham I, Ferran E, Lee G, Li B, Madabhushi A, Shah P, Spitzer M, et al. Applications of machine learning in drug discovery and development. Nat Rev Drug Discov. 2019;18(6):463–477.
- Macalino SJ, Gosu V, Hong S, Choi S. Role of computer-aided drug design in modern drug discovery. Arch Pharm Res. 2015;38(9):1686–1701.
- Lin X, Li X, Lin X. A review on applications of computational methods in drug screening and design. Molecules. 2020;25(6):1375.
- Shaker B, Ahmad S, Lee J, Jung C, Na D. In silico methods and tools for drug discovery. Comput Biol Med. 2021;137:104851.
- Bruno A, Costantino G, Sartori L, Radi M. The in silico drug discovery toolbox: applications in lead discovery and optimization. Curr Med Chem. 2019;26(21):3838–3873.
- Zagotto G, Bortoli M. Drug design: where we are and future prospects. Molecules. 2021;26(22):7061.
- Dean PM, Lloyd DG, Todorov NP. De novo drug design: integration of structure-based and ligand-based methods. Curr Opin Drug Discov Devel. 2004;7(3):347–353.
- Bueso-Bordils JI, Alemán-López PA, Suay-García B, Martín-Algarra R, Duart MJ, Falcó A, Antón-Fos GM. Molecular topology for the discovery of new broad-spectrum antibacterial drugs. Biomolecules. 2020;10(9):1343.
- Bueso-Bordils JI, Alemán-López PA, Martín-Algarra R, Duart MJ, Falcó A, Antón-Fos GM. Molecular topology for the search of new anti-MRSA compounds. Int J Mol Sci. 2021;22(11):5823.
- Zanni R, Galvez-Llompart M, Machuca J, Garcia-Domenech R, Recacha E, Pascual A, Rodriguez-Martinez JM, Galvez J. Molecular topology: a new strategy for antimicrobial resistance control. Eur J Med Chem. 2017;137:233–246.
- Zanni R, Martínez-Cruz J, Gálvez-Llompart M, Fernández-Ortuño D, Romero D, García-Domènech R, Pérez-García A, Gálvez J. Rational design of chitin deacetylase inhibitors for sustainable agricultural use based on molecular topology. J Agric Food Chem. 2022;70(41):13118–13131.
- Zanni R, Galvez-Llompart M, Garcia-Domenech R, Galvez J. What place does molecular topology have in today’s drug discovery? Expert Opin Drug Discov. 2020;15(10):1133–1144.
- Blay V, García-Domenech R, Gálvez J. Problem solving with Molecular Topology: a walkthrough. ChemTexts. 2017;3(2):1–5.
- Kim S, Chen J, Cheng T, Gindulyte A, He J, He S, Li Q, Shoemaker BA, Thiessen PA, Yu B, et al. PubChem in 2021: new data content and improved web interfaces. Nucleic Acids Res. 2021;49:1388–D1395.
- (a) Mills N. ChemDraw Ultra 10.0. CambridgeSoft, 100 Cambridge Park Drive, Cambridge, MA 02140. www.cambridgesoft.com. J Am Chem Soc. 2006;128:13649–13650.(b) Cousins K. ChemDraw Ultra 9.0. CambridgeSoft, 100 Cambridge Park Drive, Cambridge, MA 02140. www.cambridgesoft.com. J Am Chem Soc. 2005;127:4115–4116.
- Mauri A. alvaDesc: a tool to calculate and analyze molecular descriptors and fingerprints. In: Roy K, editor. Ecotoxicological QSARs. New York (NY): Humana; 2020. Chapter, Methods in pharmacology and toxicology; p. 801–820.
- Solberg HE. Discriminant analysis. CRC Crit Rev Clin Lab Sci. 1978;9(3):209–242.
- StatSoft I. 2001 STATISTICA (data analysis software system), version 6. Vol. 150. Tulsa (OK): StatSoft, 734 Inc.; p. 91–94.
- Gálvez J, García-Domenech R, de Gregorio Alapont C, de Julián-Ortiz JV, Popa L. Pharmacological distribution diagrams: a tool for de novo drug design. J Mol Graph. 1996;14(5):272–276.
- Flemming HC, Wuertz S. Bacteria and archaea on Earth and their abundance in biofilms. Nat Rev Microbiol. 2019;17(4):247–260.
- Qvortrup K, Hultqvist LD, Nilsson M, Jakobsen TH, Jansen CU, Uhd J, Andersen JB, Nielsen TE, Givskov M, Tolker-Nielsen T. Small molecule anti-biofilm agents developed on the basis of mechanistic understanding of biofilm formation. Front Chem. 2019;7:742.
- Geesi MH. Synthesis, antibacterial evaluation, Crystal Structure and Hirshfeld surface analysis of a new 2-Benzylsulfanyl-3-(4-fluoro-phenyl)-6-methyl-3H-quinazolin-4-one. J Mol Struct. 2020;1208:127894.
- Khazi IM, Koti RS, Chadha MV, Mahajanshetti CS, Gadad AK. Synthesis and evaluation of the antibacterial and antitubercular activity of some N1-aral-N4-(3-chloro-4-fluorophenyl) thiosemicarbazones and their copper(I) complexes. Arzneimittelforschung. 2005;55(2):107–113.
- Romero D, Sanabria-Valentín E, Vlamakis H, Kolter R. Biofilm inhibitors that target amyloid proteins. Chem Biol. 2020;20(1):102–110.
- Majik MS, Gawas UB, Mandrekar VK. Next generation quorum sensing inhibitors: accounts on structure activity relationship studies and biological activities. Bioorg Med Chem. 2020;28(21):115728.
- Petri GL, Spanò V, Spatola R, Holl R, Raimondi MV, Barraja P, Montalbano A. Bioactive pyrrole-based compounds with target selectivity. Eur J Med Chem. 2020;208:112783.
- Sardessai Y, Bhosle S. Tolerance of bacteria to organic solvents. Res Microbiol. 2002;153(5):263–268.
- Shinde S, Lee LH, Chu T. Inhibition of biofilm formation by the synergistic action of EGCG-S and antibiotics. Antibiotics. 2021;10(2):102.
- Stenvang M, Dueholm MS, Vad BS, Seviour T, Zeng G, Geifman-Shochat S, Søndergaard MT, Christiansen G, Meyer RL, Kjelleberg S, et al. Epigallocatechin gallate remodels overexpressed functional amyloids in Pseudomonas aeruginosa and increases biofilm susceptibility to antibiotic treatment. J Biol Chem. 2016;291(51):26540–26553.
- Spengler G, Kincses A, Mosolygó T, Marć MA, Nové M, Gajdács M, Sanmartín C, McNeil HE, Blair JMA, Domínguez-Álvarez E. Antiviral, antimicrobial and antibiofilm activity of selenoesters and selenoanhydrides. Molecules. 2019;24(23):4264–4280.
- Xiong G, Wu Z, Yi J, Fu L, Yang Z, Hsieh C, Yin M, Zeng X, Wu C, Lu A, et al. ADMETlab 2.0: an integrated online platform for accurate and comprehensive predictions of ADMET properties. Nucleic Acids Res. 2021;49(W1):W5–W14.