ABSTRACT
Introduction
The field of cancer therapy has undergone a major transformation in less than a decade due to the introduction of checkpoint inhibitors, the advent of next generation sequencing and the discovery of neoantigens. The key observation that the breadth of each patient’s immune response to the unique mutations or neoantigens present in their tumor is directly related to their survival has led oncologists to focus on driving immune responses to neoantigens through vaccination. Oncology has entered the era of precision immunotherapy, and cancer vaccine development is undergoing a paradigm shift.
Areas covered
Neoantigens are short peptide sequences found in tumors, but not noncancerous tissues, the vast majority of which are unique to each patient. In addition to providing a description of the distinguishing features of neoantigen discovery platforms, this review will address cross-cutting personalized cancer vaccine design themes and developmental stumbling blocks.
Expert opinion
Immunoinformatic pipelines that can rapidly scan cancer genomes and identify ‘the best’ neoantigens are in high demand. Despite the need for such tools, immunoinformatic methods for identifying neoepitopes in cancer genomes are diverse and have not been well-validated. Validation of ‘personalized vaccine design pipelines’ will bring about a revolution in neoantigen-based vaccine design and delivery.
1. Introduction
Three specific events have contributed to a fundamental shift in the treatment of cancer over the past 10 years: 1) the approval of checkpoint blocking monoclonal antibodies for the treatment of cancer, 2) the development of ‘next generation sequencing’ (NGS) methods for identifying tumor mutations, and 3) the realization that neoepitopes are a primary target of the effective immune responses unleashed after checkpoint inhibitor therapy. These three advances have led to the revolutionary proposal that nearly every cancer patient could benefit from the immune boost provided by a neoantigen-driven, personalized cancer vaccine (NEO-PCV). It follows that robust, validated, and rapid NEO-PCV design tools are needed for this new era of precision cancer immunotherapy.
1.1. Immune checkpoint blocking monoclonal antibodies
Following FDA approval of the CTLA-4 blocking antibody ipilimumab in 2011 [Citation1], four PD-1 blocking antibodies (nivolumab, pembrolizumab, cemiplimab, and dostarlimab) from 2014 to 2021 [Citation2–5], and three PD-L1 blocking antibodies (atezolizumab, avelumab, and durvalumab) in 2016 and 2017 [Citation6–8], the field of cancer immunotherapy has been entirely transformed. Checkpoint inhibitors (CPI) boost immune responses against cancer by disrupting inhibitory interactions between T cells and antigen presenting tumor cells, leading to significantly improved survival in some patients [Citation9]. It is believed that the CPI ‘take the brakes off’ the immune response, enabling effector T cells to attack tumors due to their recognition of neoepitopes [Citation10,Citation11].
While it is clear that patient survival rates are improving with CPI therapies, improved survival often comes with a significant cost in terms of ‘off-target effects.’ Because CPI mechanism of action is to ‘release the brakes’ on immune responses, treatment with CPI is frequently associated with the development of immune responses to ‘self’ proteins, leading to CPI-associated autoimmune syndromes such as gastroenteritis, diabetes, thyroid disease, and inflammation of the heart, eye and brain [Citation12,Citation13]. In fact, some studies have noted that combination trials of selected CPI have exacerbated these autoimmune side effects [Citation14]. Methods for targeting the immune response activated by CPI therapy to mutations contained in the tumor, while reducing activation of immune responses to normal tissue, are needed. Neoantigen-based vaccines may offer one solution.
1.2. New sequencing methods
Nearly simultaneously with the development of CPI, ‘next generation sequencing’ (NGS) methods have led to significant reductions in the time and cost required to sequence tumor genomes. According to the National Human Genome Research Institute (NHGRI) Genome Sequencing Program (GSP), the cost of sequencing a genome (such as a cancer genome) dropped from more than $10 M to roughly $1,000 between 2008 and 2015 when most sequencing centers transitioned from Sanger-based sequencing to ‘next-generation’ DNA sequencing technologies () [Citation15]. This fundamental shift has enabled consumers to obtain information about their ancestors and risks for certain diseases, while also placing revolutionary new cancer treatments within the reach of individuals seeking care in their local cancer centers, where NGS is becoming routine.
Figure 1. Genome sequencing costs as compiled by the National Human Genome Research Institute since 2001. In this figure, adapted from data provided by the National Human Genome Research Institute [Citation15], the cost of sequencing a single human genome is shown to drop precipitously by 100,000-fold over less than 20 years. This precipitous drop in the cost of sequencing has made it feasible to compare the entire genome of a single cancer patient to their own normal (noncancerous) genome, on a routine basis.
![Figure 1. Genome sequencing costs as compiled by the National Human Genome Research Institute since 2001. In this figure, adapted from data provided by the National Human Genome Research Institute [Citation15], the cost of sequencing a single human genome is shown to drop precipitously by 100,000-fold over less than 20 years. This precipitous drop in the cost of sequencing has made it feasible to compare the entire genome of a single cancer patient to their own normal (noncancerous) genome, on a routine basis.](/cms/asset/85ddec73-22f4-40ea-af0a-3543d5a79281/ierv_a_2012456_f0001_b.gif)
1.3. Neoepitopes and neoantigens
The convergence of CPI and NGS in the field of oncology has revealed the fundamental importance of ‘neoepitopes’ as targets for effective anti-tumor immune responses. Neoepitopes are distinguished from neoantigens because the former defines a specific sequence that is distinct from self as defined by the nine to ten amino acid frame of the sequence that fits into a Major Histocompatibility Complex (MHC) molecule (or Human Leukocyte Antigen (HLA) in human), while the latter defines a protein that contains a cancer-induced mutation, which differentiates the entire sequence of a nonmutated existing version of the same protein. Neoepitopes that are restricted by any of a patient’s HLA molecules may engage specific T cells activated by the immune system against their own tumor.
The first studies to demonstrate the potential importance of neoepitopes in patient outcome were published by Rizvi et al. and Van Allen et al., in which the prevalence (total number) of nonsynonymous mutations in the tumor genome was associated with clinical benefit in retrospective studies of non-small cell lung cancer (NSCLC) patients treated with pembrolizumab [Citation16] and of metastatic melanoma patients treated with ipilimumab [Citation17]. A subsequent study by Morris et al. demonstrated the additional importance of intra-tumoral heterogeneity in a retrospective analysis of data from The Cancer Genome Atlas (TCGA) database, suggesting that increased heterogeneity might be associated with poor survival [Citation18]. McGranahan et al. confirmed that strong responses to clonal neoantigens (that would be present in most tumor cells) as well as the total number of mutations present (total mutational burden) were associated with improved survival in a separate retrospective study [Citation19]. Many studies have highlighted the link between Tumor Mutational Burden (TMB) and response to checkpoint inhibitor therapy, with increased TMB associated with improved response to immunotherapy presumably resulting from the higher number of neoepitopes [Citation20,Citation21]. Extrapolation of CPI objective response rates from tumor mutational burden is well established. Yarchoan et al. developed a predictive algorithm that is based on total mutational burden, and proposed that this algorithm could be used to design future CPI trials [Citation22]. Although TMB is a promising biomarker for response to immunotherapy, many patients with high TMB remain unresponsive to such therapies due to numerous resistance mechanisms [Citation23,Citation24]. The team of Martin and De Groot has developed an alternative approach to defining neoepitopes using the Ancer tool, and determined that the number of Ancer-defined neoepitopes was significantly associated with patient survival in a retrospective study of TCGA bladder cancer patients that were not treated with a CPI. This evaluation with Ancer proved to be superior to comparative analyses employing patient TMB or neoepitope counts as determined by public prediction tools [Citation25].
The correlation between number of mutations present in a subset of patients and their ability to clear the tumor following CPI therapies has provided clues for understanding the role of CPI on immune responses. Although not observed across the entire patient population, the link between TMB and clinical benefit suggests that while CPI enable T cells to act, T cells that recognize neoepitopes are directly responsible for the observed immune response. What is not known is how many distinct T cells are required to recognize the neoepitopes nor which of the identified neoepitopes are ultimately responsible for an effective immune response. Thus, the precise number of mutations that is sufficient to ‘win’ the battle remains a puzzle and, consequently, it is not known whether expanding the number of T cells that recognize the neoepitopes, for example by using a vaccine targeting these neoepitopes, will improve outcome.
1.4. Personalized cancer vaccines
In essence, NGS and CPI have uncovered the importance of neoepitopes in the immune system’s fight against cancer. Since response to treatment with immune-activating CPI appears to be correlated with the immune response to neoepitopes (implying that more T cells responding to more neoepitopes is better), many immunotherapy researchers now believe that improving and expanding the immune response to the maximum number of neoepitopes possible in each individual patient’s tumor is likely to improve the outcome of immunotherapy. Therefore, the next logical step for many immuno-oncologists is to design personalized cancer vaccines for each patient targeting their individual neoepitopes (NEO-PCV) and to examine the impact of immunizing with the NEO-PCV in combination with, or without, CPI therapy. Immunization with NEO-PCV enables the expansion of new and memory T cell responses directed toward neoepitopes. While CPI therapies ‘jump start’ a patient’s immune system in a nonspecific manner, NEO-PCV have the potential to ‘steer’ these new immune responses toward the tumor. Newly expanded T cells can directly kill tumor cells, potentially leading to tumor debulking and elimination of micrometastases.
Recent publications by Ott and Sahin have confirmed the hypothesis that NEO-PCV can drive effective immune responses in advanced cancer patients [Citation26,Citation27]. In both of these seminal studies, and subsequent studies in glioblastoma [Citation28], personalized vaccines composed of as few as 5 to as many as 20 neoepitope sequences (defined for each individual) have been shown to induce effective immune responses, leading to tumor regression and clinical response in some patients. Long-term follow-up studies confirmed T cell responses generated by NEO-PCV can persist for years following immunization, demonstrating the durability of these therapies [Citation29]. This exciting news has precipitated a push to develop and validate computer algorithms and vaccine design pipelines that can rapidly and precisely define neoepitopes from tumor genomes. Since all vaccine platforms have limitations on the number of antigens that can be included in vaccines, tools that filter and rank the most immunogenic neoantigens are also in high demand.
Successful deployment of the new pipelines for designing PCV also depends on identifying the most effective vaccine delivery vehicle and adjuvant, and FDA approval of these N = 1 (singular, or unique) biologics. Thus, selection of neoepitopes, formulation, and FDA regulation are just a few of the hurdles that face NEO-PCV development. Others include: selection of ‘clonal’ epitopes that are present in all of the subsequent generations of cells in the evolving tumor; avoiding mutated epitopes that may induce tolerance (known as Treg epitopes) or off-target (anti-self) effects; delivering the vaccine (as short peptides, long peptides, or strings of epitopes); vaccine synthesis (methods range from production of the neoepitopes as individual synthetic long peptides or encoding the neoepitopes in synthetic platforms such as mRNA, RNA replicon, DNA or using vector-based platforms such as viral or bacterial vectors); selection of the appropriate adjuvant; selection of the dosing schedule; working with the FDA to revisit the traditional IND approval process (which, if unmodified, would require one IND package per individual vaccine); and design of the clinical trials. While the barriers to NEO-PCV development are many, the benefits of NEO-PCV are becoming more apparent to cancer vaccine developers, patients, and their oncologists.
2. Personalized cancer vaccines: from design to delivery
Administering NEO-PCV to patients involves a series of steps starting from tumor sequencing, bioinformatics analyses, drug product manufacturing, to vaccine formulation. While there is not a unique way to conceive and produce NEO-PCV, we detail in the next sections the main steps involved in their design.
2.1. Tumor mutanome sequencing
The process of identifying tumor specific mutations starts with the collection of tumor DNA, typically from a biopsy stored in a formalin-fixed paraffin-embedded (FFPE) block, and the patient’s reference DNA, also referred to as germline or ‘normal’ DNA, from nonmalignant tissue such as peripheral blood. Sequencing a patient’s normal DNA enables the removal of germline variants found in tumor tissues, which would be inadequately maintained when only comparing a tumor’s genome with a reference, or generic, human genome assembly.
Sequencing of DNA material can be performed using Whole Exome Sequencing (WES) or Whole Genome Sequencing (WGS) techniques, both high-throughput sequencing technologies. WES remains the method of choice in most PCV pipelines as it limits sequencing to protein coding regions, the very same regions that contain tumor mutations accessible to the immune system once expressed as proteins following translation. A deeper characterization of the tumor genome can be achieved with WGS, at a greater cost compared to WES, as chromosomes will be sequenced in their entirety, giving the opportunity to identify more complex and large-scale structural variations such as chromosomal inversions or translocations. The additional information provided by WGS over WES has had, so far, limited utility for the design of PCVs since the bulk of the WGS will cover intergenic regions whose mutations are not translated under normal circumstances and, therefore, are not available for recognition by the immune system. New reports however suggest that the immune system can recognize neoantigens arising from the translation of noncoding or dark matter DNA, giving rise to a new class of intergenic, noncoding, or cryptic neoantigens [Citation30–32]. Identifying these novel neoantigens usually requires steps involving liquid chromatography-tandem mass spectrometry (LC-MS/MS), which limits their utility for quick and inexpensive NEO-PCV designs.
Identification of tumor specific somatic mutations can be done after pre-processing sequencing data, which aligns raw sequencing reads to a reference human genome and flags sequencing artifacts or low-quality base callings, which could be interpreted as tumor variants in subsequent bioinformatics analyses. The process of identifying tumor mutations, or variant calling, can be done with one or more algorithms that in essence compare tumor, normal, and reference genomes to provide lists of tumor-specific genomic alterations, such as single nucleotide variants (SNVs), insertions, or deletions [Citation33–39]. Of note, somatic tumor variant identification remains a more challenging task compared to germline variant identification. The added difficulty is largely due to the bulk sequencing of heterogenous tumor cell populations, each with a potentially unique genome and distinct set of mutations, while admixed with surrounding healthy cells collected during biopsy. In comparison, germline variant identification relies on sequencing data obtained from a homogenous cell population presenting an identical genome. Increasing the depth of coverage during the sequencing step can provide some help to identify rare tumor variants that may otherwise be missed. In addition, a common approach is to combine the results from multiple somatic variant callers, although no standard exists for merging such results (e.g. intersection versus union).
To comprehensively characterize patients’ tumors, transcriptome analyses, or RNA-Seq analyses, are performed on the tumor samples to confirm the presence of the tumor mutations in expressed proteins and to quantify their abundance. While sequencing the tumor RNA is not required to identify most of the tumor DNA alterations compared to WES techniques, the advantage of including RNA-Seq in mutanome analyses lies in the improvement of the quality of the selected neoepitopes for vaccine inclusion, as highly expressed mutations can be prioritized over nonexpressed variants.
However, the correlation between mRNA expression and presentation of peptide-MHC complexes (pMHC) on the surface of tumor cells at levels sufficient for recognition by effector T cells has not been established. Very low levels of surface pMHC are required to trigger effector T cell responses [Citation40–42], with no more than three pMHC class I complexes required for CD8+ T cell mediated killing [Citation43] and one pMHC class II complex required for cytokine production by CD4+ T cells [Citation44]. Neoepitope specific effector T cells, such as those primed by a vaccine containing tumor-derived neoepitopes, would require minimal levels of pMHC expression to effectively target a tumor cell for destruction. Furthermore, peptide production for presentation on MHC class I molecules can occur from extremely small amounts of cellular protein [Citation45,Citation46]. These peptides are often sourced from the pioneer round of translation, including translation from short-lived mRNA degraded through nonsense-mediated decay [Citation47]. In fact, studies examining the correlation between pMHC class I and the mRNA encoding the presented peptides have demonstrated that the MHC class I presented peptides are often generated from proteins produced from mRNA with very low or undetectable levels [Citation48,Citation49]. Therefore, using tumor mRNA levels to select neoepitope sequences for inclusion in tumor vaccines may exclude a significant cohort of effective T cell targets.
2.2. Identifying mutations presented by HLA molecules
While large-scale cancer genome modifications exist, including gene fusions, gene inversions, and gene duplications, which all may create novel epitopes at their breakpoints, most efforts in the neoantigen field have focused on three types of nucleic acid modifications: in-frame insertions and deletions (indels), out-of-frame insertions and deletions (frameshifts), and single base substitutions (SNVs), the latter representing over 90% of all mutations in the TCGA and the International Cancer Genome Consortium (ICGC) repositories [Citation50]. Any nucleic acid modifications that affect coding sequences may be relevant to immunotherapy, as mutated or otherwise abnormal proteins mark cancer cells as ‘non-self’ to the immune system. Mutated peptides may be presented on the surface of the cancer cell or may be presented to the immune system by other cells (antigen presenting cells) that are constantly sampling the tumor environment. Once mutated sequences are identified for a given tumor, in silico tools are used to identify the best T cell epitopes.
When the mutated protein enters the degradation pathway, it is chopped up into small peptide fragments, which may bind to other molecules called HLA that carry the fragments to the surface of the antigen presenting cell. If these mutated fragments carried by HLA look like self, the immune system will try to reduce the possibility of anti-self or autoimmune responses by engaging T cells that induce tolerance known as regulatory T cells (Tregs). If the fragments contain a mutation that makes them look foreign, T cells able to recognize foreign or ‘neoepitopes’ may become activated and attack nearby cells that carry the same mutation.
T cell response is the primary immune defense against cancer cells, and once unleashed, each patient’s own T cells are able to attack the mutated cells. The immuno-oncology field has for the most part focused on CD8+ cytotoxic T cells, and consequently on the identification of HLA class I neoepitopes, as they directly kill tumor cells presenting non-self antigens. CD4+ helper T cells play a significant role in mounting a durable (memory) and robust immune response, and while there have been significantly fewer studies in the oncology field compared to CD8+ T cells, their relevance in tumor control has been highlighted in several pivotal murine and human studies [Citation26–28,Citation51,Citation52]. Other T cells, known as regulatory T cells (Tregs), can suppress immune responses and act as natural off switches to the immune response. These cells may be triggered by mutated T cell epitopes and can be either CD4+ or CD8+. Their ability to recognize self-proteins has been known for quite some time [Citation53]. They also have a role in cancer as many tumors are actively infiltrated by regulatory T cells, which may reduce the local immune response to ‘foreign’ mutations present in the tumor.
It comes as no surprise that cancer cells, which evolve rapidly, are able to develop mechanisms for avoiding immune responses. Two of these mechanisms involve cell surface proteins that attenuate the cellular immune response: PD-1/PD-L1 and CTLA-4. Checkpoint modulators, approved and in development, target these proteins, their ligands, and other immune checkpoint proteins to abrogate this defense by tumor cells. Tumor cells have other means of evading immune responses, which are summarized in other excellent reviews [Citation23,Citation24]. In addition to these escape mechanisms, the team of De Groot has proposed that some tumor mutations may harbor inhibitory, or simply tolerated, neoepitopes that play a role in camouflaging the tumor from immune control [Citation54]. Other groups have confirmed that the inclusion of inhibitory neoantigens in personalized cancer vaccines have detrimental consequences, leading to poorly effective vaccines [Citation55]. Recently, investigators have been exploring the potential for ‘immune camouflaging’ mutations to drive effective T cell responses, and in contrast, the ability of cancer cells to use the presentation of ‘self-like’ epitopes as a means of evading or down regulating immune defenses.
2.2.1. HLA binding motifs
Since binding motifs can be deduced by observing patterns in large databases of peptides known to bind to individual HLA molecules, such as the Immune Epitope Database (IEDB) [Citation56], or more recently from large sets of HLA ligands identified by LC-MS/MS [Citation57–60], it is feasible to develop tools for predicting which peptides will bind to which HLA.
Generating binding prediction models for HLA class I molecules is facilitated due to their close-ended binding groove feature. HLA ligands identified using in vitro methods, typically 9 or 10 amino acids in length, fit entirely in the HLA binding groove, rendering an unambiguous identification of the binding register for machine learning techniques. Modeling efforts have mostly focused on the HLA-A and HLA-B loci due to their higher expression compared to the HLA-C locus [Citation61].
HLA class II prediction tools may have been underappreciated in the immuno-oncology field, despite their standard usage in immunogenicity risk assessments of biologics for the past decade [Citation62,Citation63]. Modeling interactions between peptides and HLA class II molecules faces specific challenges not shared with HLA class I molecules. The identification of the binding register for HLA class II molecules is ambiguous due to longer peptides, typically 15 to 25 amino acids in length, interacting with the open-ended HLA class II binding groove. This added difficulty at pinpointing the core 9-mer frame binding to HLA class II molecules is a reason why class II binding predictions have lower accuracies than class I binding predictions. Furthermore, HLA-DP and HLA-DQ are particularly challenging to model due to polymorphisms found in both of their alpha and beta chains, unlike HLA-DR, where polymorphism is only observed in the beta chain. Most modeling attention has, therefore, been given to the HLA-DR locus, which has also been shown to be more expressed than the HLA-DP and HLA-DQ loci [Citation64,Citation65].
2.2.2. Neoepitopes
Neoepitopes that are introduced as a result of single amino acid mutations are extremely common (as discussed above), and these most often effectively distinguish cancer proteins from their normal counterparts from the perspective of the immune system. These mutations make the tumor appear foreign, allowing the immune system to attack it as it would any other pathogen within the human body. The more foreign the sequence appears, the more likely it is that a high-affinity T cell receptor that is not tolerized to the antigen is present in the peripheral circulation. It is important to note that, unlike tumor-associated antigens (TAAs) that may be present in more than one tumor type and across normal tissue of patients, neoepitopes are mostly specific to each patient’s tumor (‘private’ neoepitopes). While the vast majority of neoepitopes are ‘private’ or unique to each patient’s tumors [Citation66], certain neoepitopes are shared across patients (‘shared’ neoepitopes) [Citation67,Citation68]. The frequency of these shared neoepitopes is dependent on the tumor type [Citation69]. Neoepitopes (private and shared) have been found across a very wide range of tumor types.
Many epitope prediction tools can swiftly pinpoint neoepitopes that bind a patient’s specific HLA, however, HLA binding does not guarantee immunogenicity. In the next section, we discuss recent improvements in the methods used for the rapid identification of immunogenic neoepitopes using in silico tools.
2.3. Defining immunogenic T cell epitopes among HLA binders
It has been postulated that the reason many therapeutic cancer vaccines have failed is that the antigens used in these vaccines are based on TAAs, which may be recognized as ‘self’ by the immune system. In addition, the presence of Treg inducing epitopes in TAAs may be responsible for an immune-suppressive or modulating effect. Furthermore, the presence of cross-reactive epitopes in TAAs may be responsible for immune related adverse events [Citation70,Citation71]. In contrast with prior cancer vaccine programs, NEO-PCV are based on ‘unique’ neoepitopes (unique to the patient and to the tumor) which are broadly recognized as ‘non-self’ and therefore should be capable of generating a potent immunogenic and clinical response, with less risk of inducing autoimmunity.
Much less is known about the T cell receptor (TCR)-facing side of the T cell epitope because the interaction between the outward-facing amino acids, the borders of the HLA molecule into which the peptide is bound, and the TCR forms a three-dimensional interaction. Each TCR is educated (in the thymus) based on recognition of the specific combination of peptide and the presenting HLA molecule. TCRs appear to recognize a complex surface that includes the outer face of the T cell epitope and the HLA to which it is bound. Thus, many millions of TCRs can be postulated and it is nearly impossible to identify those that are the most important for responding to a single peptide. Structural modeling of the TCR-face has confirmed the importance of TCR facing residues in the definition of immunogenic epitopes in the immune response to cancer [Citation72]. Murine studies have also highlighted the importance of considering the position of mutations within epitope sequences, affecting TCR versus MHC-facing residues, when predicting immunogenicity [Citation73]. Moise et al. described in 2013 the JanusMatrix tool which addresses this issue, where epitopes that have extensive cross-conservation with self epitopes are removed from consideration when designing novel vaccines [Citation74]. This approach has the advantage of removing from consideration neoepitopes that may be recognized by cross-reactive T cells, such as nTregs, which suppress immune responses to self-epitopes. Balachandran and Łuksza more recently described an alternative approach prioritizing neoepitopes that exhibit similarities with other known immunogenic sequences, such as epitopes derived from bacterial and viral pathogens [Citation75,Citation76].
2.4. Additional filters for refining epitope selection for vaccine design
Filtering and ranking candidate neoantigens remains a key differentiating factor between the approaches of different immuno-oncology groups. Here we summarize the additional factors that are considered when prioritizing candidate neoantigens.
2.4.1. Clonality of the mutations
Ideal immunotherapies are ones that target all tumor clones at once to avoid immune escape and tumor resurgence. As such, clonal mutations, also known as truncal mutations which arose early on during tumor evolution and are theoretically present in most tumor clones, have become increasingly prioritized as targets. While substantial effort has been put toward understanding tumor evolution and identifying early mutational events that should be shared across all tumor clones [Citation77,Citation78], many groups employ variant allele frequencies (VAF) as proxies for clonality, as this information is more readily available from sequencing pipelines. Other approaches focus on mutations that are shared across several tumor biopsies from an individual patient, collected from different sites and/or points in time, information that is not always available for all patients.
2.4.2. In vitro/ex vivo screening
Some neoantigen pipelines include both in silico and in vitro components for 1) identifying candidate neoantigens, and 2) validating them in various in vitro or ex vivo assays, such as ELISpot assays employing autologous tumor infiltrating lymphocytes (TILs) or PBMCs [Citation79–81]. This approach has the advantage of including a validation step in order to enrich immunotherapies for neoantigens that show immune activating properties. However, a major hurdle lies in the significant time (and cost) needed to perform such validations, which some cancer patients critically lack. In addition, current in vitro high-throughput screenings only retrieve neoepitopes for which a preexisting T cell response exists and miss neoepitopes capable of generating de novo T cell responses.
2.5. Comprehensive, cloud-based vaccine design toolkits
In addition to stand-alone epitope prediction tools such as those available on IEDB (the Immune Epitope Database) [Citation82], several of these epitope-selection algorithms have also been incorporated into computational pipelines that can automatically generate mutanome-to-vaccine personalized vaccines. The key to success for these neoepitope mapping and NEO-PCV pipeline efforts will be accuracy, rapidity, and validated software, within a process that is entirely in silico to avoid unnecessary delays in bringing therapies to patients. While the majority of neoantigen companies are developing their own proprietary end-to-end NEO-PCV pipelines, several academic groups have made their workflows available to the community, including the OpenVax, pVACTools, and MuPeXi suites [Citation83–85].
Over the past 20 years, De Groot and Martin have developed a suite of advanced vaccine design tools (iVAX) that have been applied broadly to human pathogens [Citation86]. More recently, Richard and Martin began to construct a pipeline for production of NEO-PCV that capitalizes on iVAX and their vaccine development and T cell immunology expertise, called Ancer () [Citation25,Citation87].
Figure 2. The Ancer pipeline. Tumor/normal Next Generation Sequencing (NGS) data is inputted in Ancer which first identify somatic variants and HLA types of the patient. Mutations are then processed with EpiMatrix to identify ones that generate HLA class I or HLA class II neoepitopes. Neoepitopes are then refined with JanusMatrix to remove sequences capable of eliciting regulatory T cells or other deleterious cross-reactive T cells. Neoantigens containing multiple neoepitopes are then automatically designed by the platform and subsequently ranked using proprietary parameters. The Ancer pipeline is a locked process aimed at minimizing human and manual interventions.
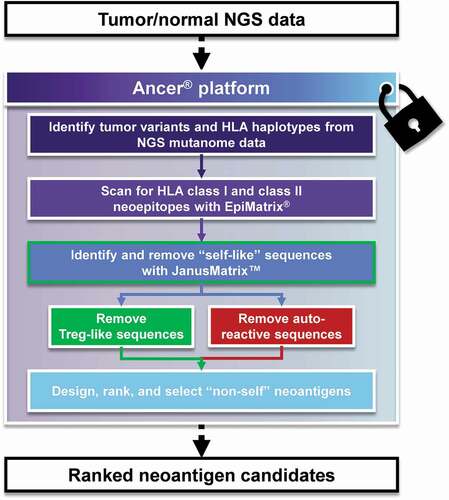
2.6. The Ancer pipeline
The key concepts that informed the development of the Ancer toolkit by the team of De Groot were that improving NEO-PCV would require highly accurate epitope prediction and removal of epitopes that were tolerated or inhibitory. Two algorithms lie at the core of Ancer. They are: EpiMatrix, an algorithm that models the interaction between a linear peptide and an MHC or HLA molecule [Citation88]; and JanusMatrix, a tool that compares HLA-binding epitopes to epitopes in the human genome, enabling the identification of potentially tolerated or inhibitory epitopes [Citation74].
EpiMatrix is a proprietary, highly accurate epitope-mapping tool that has been validated in prospective studies [Citation86,Citation89–93]. Recent analyses of elution datasets show a 96% agreement between predictions from EpiMatrix and peptides eluted from common HLA class I molecules [Citation86]. Advances in the development of the tool and careful curation of the training data (sets of T cell epitopes known to bind to the target HLA) have improved the accuracy of EpiMatrix over time [Citation62,Citation86].
Moise et al. discovered, in 2013, a means of predicting epitopes from viral pathogens that activate regulatory T cells (Tregs) using the JanusMatrix algorithm [Citation74,Citation89,Citation90,Citation94]. This approach involves identifying self-epitopes that are similar to the target epitope at the TCR facing residues. More recently, JanusMatrix has been validated in studies of pathogens [Citation91,Citation95–99] including SARS-CoV-2 [Citation100], self antigens [Citation101–103], and cancer vaccines [Citation87,Citation93].
Ancer analyzes each peptide for all four types of possible responses (Th, CTL, Treg, and tolerated or Null), thereby accelerating the process of identifying tumor neoepitopes to design a vaccine that is unique to each patient’s genetic background and cancer. Ancer identifies epitopes with a higher degree of accuracy due to the incorporation of ‘filters’ integrated in the EpiMatrix and JanusMatrix algorithms. Top-ranked neoepitopes selected by Ancer have been shown to be highly immunogenic in prospective studies [Citation87,Citation104]. The JanusMatrix tool enriches the process of neoantigen selection in Ancer for epitopes that stimulate effector T cells by removing epitopes that are highly conserved with self-epitopes, such as epitopes inducing Treg or other detrimental T cells (including T cells with potential host cross-reactivity and/or anergic T cells), leading to more accurate selection of MHC class I- and class II-restricted neoepitopes. Use of these tools in the oncology field may allow for the prioritization of neoepitopes exhibiting reduced potential for inducing Tregs, whose activation can diminish the efficacy of cancer vaccines.
While most of the focus in immuno-oncology revolves around the identification of MHC class I neoepitopes recognized by CD8+ T cells, another class of neoepitopes, recognized by CD4+ T cells, have been largely overlooked due to the higher degree of difficulty in accurately predicting MHC class II ligands. The prediction of CD4+ T cell epitopes is a key step within the development pipeline of new biologics and has been a focus of the EpiMatrix algorithm for over a decade [Citation63,Citation105]. Recent in vitro validation work of over 200 prospectively selected HLA class II candidate epitopes highlights an average concordance of 74% between EpiMatrix predictions and observed HLA binding compared to 54–66% for other publicly available HLA class II prediction tools [Citation86]. In addition, proof-of-concept experiments showcased that cancer vaccines designed with Ancer are both immunogenic and effective in a murine setting [Citation87].
2.7. Formulation and delivery of PCV
Classically, any given drug product is developed and used for the treatment of a broad cohort of eligible patients. In the case of NEO-PCV, a unique and personalized therapeutic cancer vaccine, a cocktail of up to 20 specific peptides will be manufactured for every single patient to be treated and will be used exclusively for that patient. There will, therefore, be as many different ‘products’ as patients to be treated.
Considering that NEO-PCV is intended to treat cancer patients, it is critical that the time-window from enrollment to first administration of the vaccine is as short as possible. In particular, a rapid vaccination timeframe will allow NEO-PCV to be used in the neoadjuvant clinical setting, before tumor resection, where T cells are healthier and more likely to trigger a potent anti-tumor response. The timeframe from tissue biopsy to first vaccine administration typically ranges from 8 to 16 weeks across different NEO-PCV platforms and hence limits its use in the neoadjuvant clinical setting(). Platforms not using in silico approaches for selecting neoantigens or not using fully synthetic antigen delivery modalities will increase the overall process timeline and tend to be non-scalable. The De Groot team successfully developed a novel synthetic peptide based NEO-PCV process, using the Ancer neoantigen prediction algorithm, with a biopsy-to-needle timeframe of under 4 weeks. One of the challenges resides in the ability to produce different sets of heterogeneous GMP grade peptides for individual patients, in a time-effective manner. Additional hurdles include a lack of complete control over peptide quality, peptide solubility, and interactions between peptides (if pooled), which all affect the composition of the vaccine product. Accurate targeting and trafficking of the vaccine should also not be undervalued to ensure proper processing and presentation of the delivered neoantigens. The development of unique vaccine products that need to be produced ‘on demand’ for every patient to be treated differs in many aspects from conventional drug development and requires innovative approaches. This requires paradigm shifts along the entire therapeutic cancer vaccine development process, including logistics, manufacturing, nonclinical and regulatory aspects, as well as patient management.
Figure 3. Neoantigen vaccine administration timeline. Patients have for the most part received NEO-PCVs in adjuvant settings due to slow design and production. Optimized vaccine design systems that employ diagnostic biopsies to identify neoantigens along with accelerated manufacturing and formulation processes allow vaccines to be administered in the neoadjuvant setting, where patients and their immune system are overall healthier. CPI: checkpoint inhibitor.
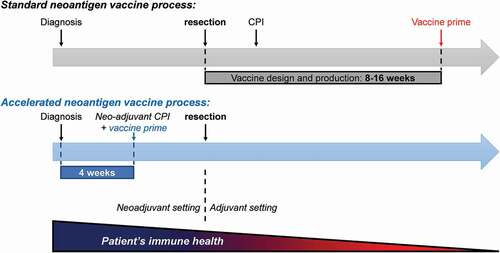
Peptide vaccines have a long history in oncology and infectious diseases. Novel and creative technologies have emerged as alternatives for delivering NEO-PCV. RNA and DNA platforms, the former having been popularized by the first FDA approved mRNA SARS-CoV-2 vaccines, present some advantages over peptide vaccines, such as offering a platform that can encode a more flexible number of neoantigens (hypothetically up to hundreds), and enabling neoantigens to undergo a more ‘natural’ processing through the HLA presentation pathway. Improvements in formulation will allow to alleviate current stability issues experienced with these vaccines, which currently demand strict and rigid shipment requirements. Viral vector vaccines, which use a modified virus backbone to deliver a neoantigen payload, are also being investigated for NEO-PCV delivery. Similarly to RNA and DNA vaccines, viral vectors allows the delivery of a quasi-unlimited neoantigen payload, although acquired immunity to the viral vector itself after initial priming, preventing neoantigens from being properly delivered, can be a hindrance in settings where repeated immunizations are required.
2.8. Potential regulatory hurdles
The approval process of NEO-PCV therapies by regulatory agencies such as the FDA or the EMA is not standardized. The complexity lies in the fact that obtaining traditional regulatory approval for a drug becomes problematic when each patient receives a different product of unique composition, albeit similarly formulated and administered. Requiring a traditional approval for each newly designed vaccine, i.e. one IND application for each patient, would be logistically cumbersome, costly, lengthy, and would significantly impact our ability to bring these novel therapies to patients. These additional hurdles have galvanized interest in vaccination approaches centered around shared neoantigens for which a more traditional regulatory approval process can be employed.
Regulatory agencies are aware that new approval processes are needed for NEO-PCV products and are exploring the option of providing a blanket ‘process’ approval. Under this scenario, NEO-PCV would need to be designed using a precisely defined, locked, ‘verified’ (in the context of software systems), and repeatable process with minimal human intervention. Fully automated vaccine design platforms, such as Ancer, offer vaccinologists a means to develop new therapies following many of the above requirements.
3. Conclusion – every cancer patient deserves their own personal cancer vaccine
The widespread accessibility of next generation sequencing has opened the door to precision cancer therapies targeting mutations expressed by tumor cells. New, faster, tumor genome sequencing has revealed this truth. That is, despite the identification of ‘driver mutations’ in specific genes that enable cancer cells to proliferate far faster than normal cells, and despite the occurrence of selected TAAs with specific tumors, there actually are a very limited number of mutations within the proteins of individual cancer cells that are shared between individuals.
Reduction in the cost of genome sequencing has had a dramatic impact on the availability of information for the development of personalized cancer vaccines. Only recently has it been possible to identify mutations in a cancer and compare to the normal genome within a few days. Processes are being developed to further accelerate and decrease the cost.
As with every new therapy, there are risks and benefits that must be considered. Since each vaccine will be unique to the individual, risks are somewhat mitigated, and the approval process may be limited to demonstrating no excess of harm over what many consider to be therapies that are already associated with a broad range of side effects. One major advantage for NEO-PCV may be that they make it possible to lower the dose of CPI (when used in combination with a CPI), limiting off-target effects, and/or that CPI-activated immune responses are restricted to tumor epitopes and not normal tissue. Another major advantage of NEO-PCV may be that that they can turn ‘cold,’ non-inflamed and poorly immunogenic tumors (such as pancreatic, prostate, glioblastoma, breast, and liquid tumors such as lymphoma and leukemia) into ‘hot,’ inflamed and highly immunogenic tumors that are responsive to immunotherapy. Exactly how PCV will be integrated into the new landscape of potential therapies for cancer vaccines remains to be determined. What is more certain is that every cancer patient stands to benefit from a personalized cancer vaccine, and therefore, we are entering a new era of personalized vaccine design.
4. Expert commentary and opinion
Over the past 20 years, researchers and commercial companies have developed immunoinformatic tools to discover the epitopes that activate T cells in infectious disease, autoimmunity, allergy, and biologic therapies. The process of validating the predictions is well defined and, as a result, databases such as IEDB now contain millions of T cell epitopes that have been identified using epitope-prediction tools such as SYFPEITHI, Tepitope, NetMHC, and others. The group of De Groot and Martin uses EpiMatrix for epitope prediction and has developed companion tools that enable the identification of conserved epitopes across proteins (Conservatrix), the design of ‘consensus’ epitopes (EpiAssembler) and developed the first tool for the detection of T cell epitopes that are potentially regulatory in nature (JanusMatrix). These tools were integrated in the first commercially available cloud-based vaccine design software platform, iVAX, enabling vaccine developers to start from protein sequences and develop epitope-based vaccines [Citation86,Citation106]. iVAX and the related immunoinformatics product for biologic immunogenicity screening and immune engineering, ISPRI, have been validated experimentally in retrospective and prospective studies, and have demonstrated efficacy in product development. The research and development teams led by De Groot are active collaborators and have collectively published more than 160 articles describing the application of the iVAX and ISPRI toolkits to vaccines and biologics design. More recently, they have created a seamless pipeline for the design of personalized cancer vaccines that capitalizes on iVAX and their vaccine development and T cell immunology expertise called Ancer.
Tumor mutation calling from NGS data and accurate T cell epitope predictions are the two stumbling blocks to identifying the best epitopes for cancer vaccine design. Concerns regarding both of these aspects of NEO-PCV development recently contributed to the formation of the Tumor Neoantigen Selection Alliance (TESLA) by the Parker Institute for Cancer Immunotherapy (PICI) [Citation107]. Recognizing the potential impact of inaccurate epitope selection on the efficacy of neoepitope-based vaccines, PICI worked with a few selected participating groups to test their various MHC class I epitope prediction algorithms starting from the same tumor DNA and RNA sequences [Citation108]. While limited to MHC class I neoepitopes, this effort has already highlighted the wide variability across neoepitope prediction and selection pipelines and may provide other valuable insights for the field.
Within five years, NEO-PCV should be approved by regulators and should be facing rapid adoption both in combination with checkpoint inhibitors and possibly as single agents, across a wide range of cancers. NEO-PCV will emerge as the first application of precision medicine, potentially benefiting millions of cancer patients. Further improvement in sequencing techniques and cost reduction will allow the switch from standard sequencing of tumor/normal exomes to sequencing full genomes, allowing the identification of novel intergenic and more complex genomic alterations, further increasing the pool of actionable targets. In silico predictive algorithms will become the preferred method for selecting the neoepitopes used in NEO-PCV, over cumbersome methods relying on mass spectrometry, peptide elution or high-throughput screening. Predictive algorithms that are accurate, robust and validated will be preferred for selecting the most immunogenic and clinically relevant epitopes. The overall ‘biopsy-to-needle’ NEO-PCV manufacturing process will have to be rapid and ‘scalable’, i.e. designed to be able to serve, in a timely and cost-efficient way, hundreds of thousands of cancer patients per year. We believe the future lies in fully decentralized production systems, where local hospitals treating cancer patients will sequence tumor samples in their sequencing core (already performed routinely), download a vaccine recipe via cloud-based analysis toolkits, and produce the vaccine components on site via portable and scalable synthesizers, further alleviating logistical hurdles of shipping biopsies and vaccines across countries and continents. Only then will every patient worldwide have the chance of receiving their own personal cancer vaccine.
Article highlights
• Advances in Next Generation Sequencing and immune checkpoint inhibitor therapies have highlighted the role of neoantigens in eliciting anti-tumor responses.
• Neoantigens are short, mutated protein fragments which help T cells distinguish tumor from normal cells.
• Custom neoantigen-based vaccines can now be designed and delivered to individual patients in order to stimulate T cell directed anti-tumor responses.
• This review details the general steps employed to identify neoantigens and design neoantigen-based personalized vaccines.
Declaration of interest
AS De Groot and WD Martin are senior officers and majority shareholders of EpiVax, Inc, a privately owned immunoinformatics and vaccine design company. MF Princiotta and GD Steinberg are senior officers, D Bridon was a senior officer, and G Richard is currently an employee of EpiVax Therapeutics, Inc., a precision immunotherapy company and subsidiary of EpiVax, Inc. MF Princiotta, GD Steinberg, D Bridon, and G Richard have equity in EpiVax Therapeutics. GD Steinberg is a member of Clinical Trial Protocol Committees for the following companies: Merck, BMS, Janssen, Cold Genesys, Pfizer, PhotoCure, Fidia, is or has been a scientific advisor/consultant within the past 5 years for the following companies: Heat Biologics, Cold Genesys, PhotoCure, Merck, Roche/Genentech, Ciclomed, Taris Biomedical, MDxHealth, Fidia Farmaceuticals, Urogen, Ferring, Aduro, Boston Scientific, Bristol Myers Squibb, Astra Zeneca, Pfizer, Janssen, EpiVax Therapeutics, Natera, FKD, Ferring, EnGene Bio, SesenBio, BioCanCell, Nucleix, Ipsen, Combat Medical, Astellas, Fergene, Dendreon, Abbvie, Seattle Genetics, and has equity stock/options in EpiVax Therapeutics and Urogen. The authors have no other relevant affiliations or financial involvement with any organization or entity with a financial interest in or financial conflict with the subject matter or materials discussed in the manuscript apart from those disclosed.
Reviewer disclosures
Peer reviewers on this manuscript have no relevant financial or other relationships to disclose.
Author contributions
G Richard and AS De Groot drafted the manuscript. All authors made substantial edits, reviewed, and approved the manuscript for submission.
Funding
This paper was not funded.
References
- Cameron F, Whiteside G, Perry C. Ipilimumab: first global approval. Drugs. 2011;71(8):1093–1104.
- Poole RM. Pembrolizumab: first global approval. Drugs. 2014;74(16):1973–1981.
- Deeks ED, Deeks ED. Nivolumab: a review of its use in patients with malignant melanoma. Drugs. 2014;74(11):1233–1239.
- Markham A, Duggan S. Cemiplimab: first global approval. Drugs. 2018;78(17):1841–1846.
- Markham A. Dostarlimab: first approval. Drugs. 2021;81(10):1213–1219.
- Markham A. Atezolizumab: first global approval. Drugs. 2016;76(12):1227–1232.
- Kim ES. Avelumab: first global approval. Drugs. 2017;77(8):929–937.
- Syed YY. Durvalumab: first global approval. Drugs. 2017;77(12):1369–1376.
- Alexander W. The checkpoint immunotherapy revolution: What started as a trickle has become a flood, despite some daunting adverse effects; new drugs, indications, and combinations continue to emerge. Pharm Ther. 2016;41:185–191.
- Gubin MM, Zhang X, Schuster H, et al. Checkpoint blockade cancer immunotherapy targets tumour-specific mutant antigens. Nature. 2014;515(7528):577–581.
- Snyder A, Makarov V, Merghoub T, et al. Genetic basis for clinical response to CTLA-4 blockade in melanoma. N Engl J Med. 2014;371(23):2189–2199.
- Friedman CF, Proverbs-Singh TA, Postow MA. Treatment of the immune-related adverse effects of immune checkpoint inhibitors: a review. JAMA Oncol. 2016;2(10):1346–1353.
- Läubli H, Balmelli C, Kaufmann L, et al. Influenza vaccination of cancer patients during PD-1 blockade induces serological protection but may raise the risk for immune-related adverse events. J Immunother Cancer. 2018;6(1):1–10.
- Gunawan F, George E, Roberts A, et al. Combination immune checkpoint inhibitor therapy nivolumab and ipilimumab associated with multiple endocrinopathies. 2018;Endocrinol. Diabetes Metab. Case Reports. 2018:17–146.
- Wetterstrand KA. DNA sequencing costs: data from the NHGRI large-scale Genome Sequencing Program (GSP) [cited 2020 Dec 10]. Available at: www.genome.gov/sequencingcostsdata
- Rizvi NA, Hellmann MD, Snyder A, et al. Mutational landscape determines sensitivity to PD-1 blockade in non-small cell lung cancer. Science. 2015;348(6230):124–128.
- Van Allen EM, Miao D, Schilling B, et al. Genomic correlates of response to CTLA-4 blockade in metastatic melanoma. Science. 2015;350(6257):207–211.
- Morris LGT, Riaz N, Desrichard A, et al. Pan-cancer analysis of intratumor heterogeneity as a prognostic determinant of survival. Oncotarget. 2016;7(9):10051–10063.
- McGranahan N, Furness AJS, Rosenthal R, et al. Clonal neoantigens elicit T cell immunoreactivity and sensitivity to immune checkpoint blockade. Science. 2016;351(6280):1463–1469.
- Rizvi H, Sanchez-Vega F, La K, et al. Molecular determinants of response to anti-programmed cell death (PD)-1 and anti-programmed death-ligand 1 (PD-L1) blockade in patients with non-small-cell lung cancer profiled with targeted next-generation sequencing. J Clin Oncol. 2018;36(7):633–641.
- Samstein RM, Lee CH, Shoushtari AN, et al. Tumor mutational load predicts survival after immunotherapy across multiple cancer types. Nat Genet. 2019;51(2):202–206.
- Yarchoan M, Hopkins A, Jaffee EM. Tumor mutational burden and response rate to PD-1 inhibition. N Engl J Med. 2017;377(25):2500–2501.
- Pitt JM, Vétizou M, Daillère R, et al. Resistance mechanisms to immune-checkpoint blockade in cancer: tumor-intrinsic and -extrinsic factors. Immunity. 2016;44(6):1255–1269.
- O’Donnell JS, Teng MWL, Smyth MJ. Cancer immunoediting and resistance to T cell-based immunotherapy. Nat Rev Clin Oncol. 2019;16(3):151–167.
- Richard G, De Groot AS, Steinberg GD, et al. Multi-step screening of neoantigens’ HLA- and TCR-interfaces improves prediction of survival. Sci Rep. 2021;11(1):9983.
- Ott PA, Hu Z, Keskin DB, et al., An immunogenic personal neoantigen vaccine for patients with melanoma. Nature. 2017;547(7662): 217–221.
- Sahin U, Derhovanessian E, Miller M, et al., Personalized RNA mutanome vaccines mobilize poly-specific therapeutic immunity against cancer. Nature. 2017;547(7662): 222–226.
- Keskin DB, Anandappa AJ, Sun J, et al., Neoantigen vaccine generates intratumoral T cell responses in phase Ib glioblastoma trial. Nature. 2019;565(7738): 234–239.
- Hu Z, Leet DE, Allesøe RL, et al., Personal neoantigen vaccines induce persistent memory T cell responses and epitope spreading in patients with melanoma. Nat Med. 2021;27(3): 515–525.
- Laumont CM, Vincent K, Hesnard L, et al. Noncoding regions are the main source of targetable tumor-specific antigens. Sci Transl Med. 2018;10(470):1–12.
- Kanaseki T, Torigoe T. Proteogenomics: advances in cancer antigen research. Immunol. Med. 2019;42(2):65–70
- Erhard F, Dölken L, Schilling B, et al. Identification of the cryptic HLA-I immunopeptidome. Cancer Immunol Res. 2020;8(8):1018–1026.
- Ye K, Schulz MH, Long Q, et al. Pindel: a pattern growth approach to detect break points of large deletions and medium sized insertions from paired-end short reads. Bioinformatics. 2009;25(21):2865–2871.
- Larson DE, Harris CC, Chen K, et al. Somaticsniper: identification of somatic point mutations in whole genome sequencing data. Bioinformatics. 2012;28(3):311–317.
- Koboldt DC, Zhang Q, Larson DE, et al. VarScan 2: somatic mutation and copy number alteration discovery in cancer by exome sequencing. Genome Res. 2012;22(3):568–576.
- Chen X, Schulz-Trieglaff O, Shaw R, et al. Manta: rapid detection of structural variants and indels for germline and cancer sequencing applications. Bioinformatics. 2016;32(8):1220–1222.
- Fan Y, Xi L, Hughes DST, et al. MuSE: accounting for tumor heterogeneity using a sample-specific error model improves sensitivity and specificity in mutation calling from sequencing data. Genome Biol. 2016;17(1):178.
- Kim S, Scheffler K, Halpern AL, et al. Strelka2: fast and accurate calling of germline and somatic variants. Nat Methods. 2018;15(8):591–594.
- Benjamin D, Sato T, Cibulskis K, et al. Calling somatic SNVs and indels with mutect2. bioRxiv. 2019 861054 ;1–8 doi:https://doi.org/10.1101/861054.
- Eisen HN, Sykulev Y, Tsomides TJ. Antigen-specific T-cell receptors and their reactions with complexes formed by peptides with major histocompatibility complex proteins. Adv Protein Chem. 1996;49:1–56.
- Sykulev Y, Joo M, Vturina I, et al. Evidence that a single peptide-MHC complex on a target cell can elicit a cytolytic T cell response. Immunity. 1996;4(6):565–571.
- Valitutti S, Lanzavecchia A. Serial triggering of TCRs: a basis for the sensitivity and specificity of antigen recognition. Immunol Today. 1997;18(6):299–304.
- Purbhoo MA, Irvine DJ, Huppa JB, et al. T cell killing does not require the formation of a stable mature immunological synapse. Nat Immunol. 2004;5(5):524–530.
- Huang J, Brameshuber M, Zeng X, et al. A single peptide-major histocompatibility complex ligand triggers digital cytokine secretion in CD4+ T cells. Immunity. 2013;39(5):846–857.
- Wolf BJ, Princiotta MF. Processing of recombinant listeria monocytogenes proteins for MHC Class I presentation follows a dedicated, high-efficiency pathway. J Immunol. 2013;190(6):2501–2509.
- Cosma GL, Lobby JL, Fay EJ, et al. Kinetically distinct processing pathways diversify the CD8 + T cell response to a single viral epitope. Proc Natl Acad Sci. 2020;117(32) :19399–19407.
- Apcher S, Daskalogianni C, Lejeune F, et al. Major source of antigenic peptides for the MHC class I pathway is produced during the pioneer round of mRNA translation. Proc Natl Acad Sci U S A. 2011;108(28):11572–11577.
- Weinzierl AO, Lemmel C, Schoor O, et al. Distorted relation between mRNA copy number and corresponding major histocompatibility complex ligand density on the cell surface. Mol Cell Proteomics. 2007;6(1):102–113.
- Pearson H, Daouda T, Granados DP, et al. MHC class I-associated peptides derive from selective regions of the human genome. J Clin Invest. 2016;126(12):4690–4701.
- Campbell PJ, Getz G, Korbel JO, et al. Pan-cancer analysis of whole genomes. Nature. 2020;578:82–93.
- Kreiter S, Vormehr M, Van De Roemer N, et al. Mutant MHC class II epitopes drive therapeutic immune responses to cancer. Nature. 2015;520(7549):692–696.
- Ott PA, Hu-Lieskovan S, Chmielowski B, et al. A Phase Ib trial of personalized neoantigen therapy plus anti-PD-1 in patients with advanced melanoma, non-small cell lung cancer, or bladder cancer. Cell. 2020;183(2):347–362.e24.
- Shevach EM. Mechanisms of Foxp3+ T regulatory cell-mediated suppression. Immunity. 2009;30(5):636–645.
- De Groot AS, Moise L, Liu R, et al. Immune camouflage: relevance to vaccines and human immunology. Hum Vaccines Immunother. 2014;10(12):3570–3575.
- Lam H, McNeil LK, Starobinets H, et al. An empirical antigen selection method identifies neoantigens that either elicit broad anti-tumor T cell responses or drive tumor growth. Cancer Discov. 2021;11(3):696–713. candisc.0377.2020.
- Fleri W, Paul S, Dhanda SK, et al. The immune epitope database and analysis resource in epitope discovery and synthetic vaccine design. Front Immunol. 2017;8:1–16.
- Abelin JG, Keskin DB, Sarkizova S, et al. Mass spectrometry profiling of HLA-associated peptidomes in mono-allelic cells enables more accurate epitope prediction. Immunity. 2017;46(2):315–326.
- Bulik-Sullivan B, Busby J, Palmer CD, et al. Deep learning using tumor HLA peptide mass spectrometry datasets improves neoantigen identification. Nat Biotechnol. 2019;37(1):55–71.
- Abelin JG, Harjanto D, Malloy M, et al. Defining HLA-II ligand processing and binding rules with mass spectrometry enhances cancer epitope prediction. Immunity. 2019;51(4):766–779.e17.
- Sarkizova S, Klaeger S, Le PM, et al. A large peptidome dataset improves HLA class I epitope prediction across most of the human population. Nat Biotechnol. 2020;38(2):199–209.
- Apps R, Meng Z, Del Prete GQ, et al. Relative expression levels of the HLA Class-I proteins in normal and HIV-Infected cells. J Immunol. 2015;194(8):3594–3600.
- De Groot AS, Martin W. Reducing risk, improving outcomes: bioengineering less immunogenic protein therapeutics. Clin Immunol. 2009;131(2):189–201.
- Jawa V, Terry F, Gokemeijer J, et al. T-cell dependent immunogenicity of protein therapeutics pre-clinical assessment and mitigation–updated consensus and review 2020. Front Immunol. 2020;11:1301.
- Amatruda TT, Bohman R, Ranyard J, et al. Pattern of expression of HLA-DR and HLA-DQ antigens and mRNA in myeloid differentiation. Blood. 1987;69(4):1225–1236.
- Lecchi M, Lovisone E, Genetta C, et al. γ-IFN induces a differential expression of HLA-DR, DQ and DP antigens on peripheral blood myeloid leukemic blasts at various stages of differentiation. Leuk Res. 1989;13(3):221–226.
- Hartmaier RJ, Charo J, Fabrizio D, et al. Genomic analysis of 63,220 tumors reveals insights into tumor uniqueness and targeted cancer immunotherapy strategies. Genome Med. 2017;9(1):16.
- Wang QJ, Yu Z, Griffith K, et al. Identification of T-cell receptors targeting KRAS-mutated human tumors. Cancer Immunol Res. 2016;4(3):204–214.
- Chheda ZS, Kohanbash G, Okada K, et al. Novel and shared neoantigen derived from histone 3 variant H3.3K27M mutation for glioma T cell therapy. J Exp Med. 2018;215(1):141–157.
- Castle JC, Uduman M, Pabla S, et al. Mutation-derived neoantigens for cancer immunotherapy Front Immunol. 2019;10:1–7.
- Linette GP, Stadtmauer EA, Maus MV, et al., Cardiovascular toxicity and titin cross-reactivity of affinity-enhanced T cells in myeloma and melanoma. Blood. 2013;122(6): 863–871.
- Brian J. Cameron BKJ. identification of a Titin-Derived HLA-A1–presented peptide for MAGE A3 T cells. Sci Transl Med. 2013;10:1–13.
- Devlin JR, Alonso JA, Ayres CM, et al., Structural dissimilarity from self drives neoepitope escape from immune tolerance. Nat Chem Biol. 2020;16(11): 1269–1276.
- Capietto A-H, Jhunjhunwala S, Pollock SB, et al. Mutation position is an important determinant for predicting cancer neoantigens. J Exp Med. 2020;217(4). https://doi.org/10.1084/jem.20190179.
- Moise L, Gutierrez AH, Bailey-Kellogg C, et al. The two-faced T cell epitope: examining the host-microbe interface with JanusMatrix. Hum Vaccin Immunother. 2013;9(7):1577–1586.
- Łuksza M, Riaz N, Makarov V, et al. A neoantigen fitness model predicts tumour response to checkpoint blockade immunotherapy. Nature. 2017;551(7681):517–520.
- Balachandran VP, Łuksza M, Zhao JN, et al. Identification of unique neoantigen qualities in long-term survivors of pancreatic cancer. Nature. 2017;551(7681):S12–S16.
- El-Kebir M, Satas G, Oesper L, et al. Inferring the mutational history of a tumor using multi-state perfect phylogeny mixtures. Cell Syst. 2016;3(1):43–53.
- Gerstung M, Jolly C, Leshchiner I, et al. The evolutionary history of 2,658 cancers. Nature. 2020;578(7793):122–128.
- Danilova L, Anagnostou V, Caushi JX, et al. The mutation-associated neoantigen functional expansion of specific T cells (MANAFEST) assay: a sensitive platform for monitoring antitumor immunity. Cancer Immunol Res. 2018;6(8):888–899.
- Nogueira C, Kaufmann JK, Lam H, et al. Improving cancer immunotherapies through empirical neoantigen selection. Trends Cancer. 2018;4(2):97–100.
- Cafri G, Yossef R, Pasetto A, et al. Memory T cells targeting oncogenic mutations detected in peripheral blood of epithelial cancer patients. Nat Commun. 2019;10(1):1–9.
- Vita R, Mahajan S, Overton JA, et al. The Immune Epitope Database (IEDB): 2018 update. Nucleic Acids Res. 2019;47(D1):D339–D343.
- Rubinsteyn A, Kodysh J, Hodes I, et al. Computational pipeline for the PGV-001 neoantigen vaccine trial. Front Immunol. 2018;8:1807.
- Hundal J, Kiwala S, McMichael J, et al. PVACtools: a computational toolkit to identify and visualize cancer neoantigens. Cancer Immunol Res. 2020;8(3):409–420.
- Bjerregaard AM, Nielsen M, Hadrup SR, et al. MuPeXI: prediction of neo-epitopes from tumor sequencing data. Cancer Immunol Immunother. 2017;66(9):1123–1130.
- De Groot AS, Moise L, Terry F, et al. Better epitope discovery, precision immune engineering, and accelerated vaccine design using immunoinformatics tools. Front Immunol. 2020;11:1–13.
- Maine CJ, Richard G, Spasova DS, et al. Self-replicating RNAs drive protective anti-tumor T cell responses to neoantigen vaccine targets in a combinatorial approach. Mol Ther. 2021;29(3):1186–1198.
- De Groot AS, Sbai H, Saint AC, et al. Immuno-informatics: mining genomes for vaccine components. Immunol Cell Biol. 2002;80(3):255–269.
- Losikoff PT, Mishra S, Terry F, et al. HCV epitope, homologous to multiple human protein sequences, induces a regulatory T cell response in infected patients. J Hepatol. 2015;62(1):48–55.
- Liu R, Moise L, Tassone R, et al. H7N9 T-cell epitopes that mimic human sequences are less immunogenic and may induce Treg-mediated tolerance. Hum Vaccines Immunother. 2015;11(9):2241–2252.
- Wada Y, Nithichanon A, Nobusawa E, et al. A humanized mouse model identifies key amino acids for low immunogenicity of H7N9 vaccines. Sci Rep. 2017;7(1):1283.
- Scholzen A, Richard G, Moise L, et al. Promiscuous Coxiella burnetii CD4 epitope clusters associated with human recall responses are candidates for a novel T-cell targeted multi-epitope Q Fever vaccine. Front Immunol. 2019;10:1–22.
- Hoffmann PR, Hoffmann FW, Premeaux TA, et al. Multi-antigen vaccination with simultaneous engagement of the OX40 receptor delays malignant mesothelioma growth and increases survival in animal models. Front Oncol. 2019;9:1–11.
- He L, De Groot AS, Gutierrez AH, et al. Integrated assessment of predicted MHC binding and cross-conservation with self reveals patterns of viral camouflage. BMC Bioinformatics. 2014;15(S4):S1–S1.
- Kruiswijk C, Richard G, Salverda MLM, et al. In silico identification and modification of T cell epitopes in pertussis antigens associated with tolerance. Hum Vaccines Immunother. 2020;16(2):277–285.
- Salerno-Gonçalves R, Tettelin H, Luo D, et al. Differential functional patterns of memory CD4(+) and CD8(+) T-cells from volunteers immunized with Ty21a typhoid vaccine observed using a recombinant Escherichia coli system expressing S. Typhi proteins. Vaccine. 2020;38(2):258–270.
- Scholzen A, Richard G, Moise L, et al. Coxiella burnetii epitope-specific T-cell responses in patients with chronic Q fever. Infect Immun. 2019;87(10):1–12.
- Jang H, Meyers LM, Boyle C, et al. Immune-engineered H7N9 influenza hemagglutinin improves protection against viral influenza virus challenge. Hum Vaccines Immunother. 2020;16(9):2042–2050.
- Khan S, Parrillo M, Gutierrez AH, et al. Immune escape and immune camouflage may reduce the efficacy of RTS,S vaccine in Malawi. Hum Vaccines Immunother. 2020;16(2):214–227.
- Meyers LM, Gutiérrez AH, Boyle CM, et al. Highly conserved, non-human-like, and cross-reactive SARS-CoV-2 T cell epitopes for COVID-19 vaccine design and validation. Npj Vaccines. 2021;6(1):1–14.
- De Groot AS, Kazi ZB, Martin RF, et al. HLA- and genotype-based risk assessment model to identify infantile onset pompe disease patients at high-risk of developing significant anti-drug antibodies (ADA). Clin Immunol. 2019;200:66–70.
- De Groot AS, Rosenberg AS, Miah SMS, et al. Identification of a potent regulatory T cell epitope in factor V that modulates CD4+ and CD8+ memory T cell responses. Clin Immunol. 2021;224:108661.
- De Groot AS, Desai AK, Lelias S, et al. Immune tolerance-adjusted personalized immunogenicity prediction for pompe disease. Front Immunol. 2021;12:1–14.
- Richard G, Berdugo G, Princiotta M, et al. P684 highly efficient selection of tumor neoantigens improves therapeutic cancer vaccine efficacy, 34th annual meeting & pre-conference programs of the society for immunotherapy of cancer (SITC 2019): part 2. J Immunother Cancer. 2019;7:283.
- Jawa V, Cousens LP, Awwad M, et al. T-cell dependent immunogenicity of protein therapeutics: preclinical assessment and mitigation. Clin Immunol. 2013;149(3):534–555.
- Moise L, Gutierrez A, Kibria F, et al. iVAX: an integrated toolkit for the selection and optimization of antigens and the design of epitope-driven vaccines. Hum Vaccines Immunother. 2015;11(9):2312–2321.
- Ledford H. Programs face off in cancer contest: predictive algorithms may help to whittle down the possible candidates for personalized cancer vaccines. Nature. 2016;540(7633):328–329.
- Wells DK, van Buuren MM, Dang KK, et al., Key parameters of tumor epitope immunogenicity revealed through a consortium approach improve neoantigen prediction. Cell. 2020;183(3): 818–834.e13.