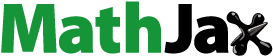
ABSTRACT
The aim of the study was to investigate whether jerk cost (JC) can discriminate between swimming levels. Nine elite and nine non-elite swimmers swam a 50-m front-crawl sprint wearing a 3D accelerometer on their back between the inferior angles of the scapulae. Lap times and JC were calculated from the acceleration signal and compared between groups and between swimmers within a group. The elite swimmers swam significantly faster lap times than the non-elite swimmers (p < 0.001). They did so with significantly lower levels of JC compared to the non-elite swimmers (p = 0.005). Furthermore, a stepwise multiple linear regression showed JC accounted for 32.9% of the variation in lap time of the elite swimmers. These results indicate that it is possible to discriminate elite from non-elite swimmers using JC: elite swimmers swim with lower JCs than non-elite swimmers. Additionally, swimming at higher speed is associated with more accelerations and decelerations in both elite and non-elite swimmers, which is reflected by higher JCs and lower smoothness. In sum, JC provides an index of swimming technique that is easy to use in training practice.
Introduction
Swimming coaches are familiar with their pupils making statements like ‘I don’t feel the water’ or ‘Things feel very smooth today’. Swimming is a highly technical sport (Ingen Schenau & Cavanagh, Citation1990) and improving the ‘feel of the water’, although a subjective expression, is an important aim in the training of elite swimmers (Sweetenham & Atkinson, Citation2003). In biomechanical terms: a swimmer should be able to generate high propulsive forces while keeping the opposing drag forces low, resulting in efficient swimming (Toussaint & Beek, Citation1992). When propulsive and drag forces are in balance, a constant speed is reached. However, the actual speed fluctuates within a stroke cycle due to the propulsive actions of the arms and legs, body-roll and breathing during swimming (Nigg, Citation1983; Tella et al., Citation2008; Toussaint & Beek, Citation1992). These fluctuations are known in the literature as intra-cyclic velocity fluctuations or variation (IVV). IVV is considered an index of skill level and technique, with lower IVVs indicating higher skill levels (Matsuda, Yamada, Ikuta, Nomura, & Oda, Citation2014; Schnitzler et al., Citation2010).
Recent developments have made it possible to quantify IVV almost in real-time by using several, carefully calibrated, cameras (Van Houwelingen, Roerdink, Huibers, Evers, & Beek, Citation2017). Unfortunately, this method is rather expensive and time consuming, and not applicable in daily training settings for generating real-time feedback to coaches and swimmers (Alberty, Sidney, Huot-Marchand, Hespel, & Pelayo, Citation2005). Instead, tri-axial accelerometry might be used in real-time to provide characteristics such as stroke rate (SR), stroke count (SC) and lap times (LT) (Davey, Anderson, & James, Citation2008; Ganzevles, Vullings, Beek, Daanen, & Truijens, Citation2017; Magalhaes, De, Vannozzi, Gatta, & Fantozzi, Citation2015). However, the instantaneous velocity profile is difficult to obtain due to the orientation problem of the sensors (Dadashi, Crettenand, Millet, & Aminian, Citation2012; Stamm & Thiel, Citation2015). Changes in sensor orientation contaminate the acceleration data as a result of the acceleration due to gravity. Therefore, a large error is introduced when integrating acceleration into speed (Stamm, James, Burkett, Hagem, & Thiel, Citation2013). Analysis of the higher derivatives of the position such as acceleration and jerk may be instrumental to overcome these problems of feedback and orientation. Both acceleration and jerk have been linked to smoother and more efficient movement (Hogan & Sternad, Citation2009; Sparrow & Newell, Citation1998; Viviani & Flash, Citation1995).
Hreljac (Citation2000) defined the jerk-cost (JC) as follows to quantify smoothness in running:
where T is total movement time and r is the position vector of a limb segment. Hreljac found that competitive runners tend to have lower jerk-cost at the heel than recreational runners, indicating that competitive runners have smoother strides (Hreljac, Citation2000). Motion smoothness as calculated in formula (1) has been studied in sports such as running and golf (Choi, Bin, Oh, & Mun, Citation2014; Hreljac & Martin, Citation1993), during arm motions (Viviani & Flash, Citation1995), and in patients with diseases that affect movement (Smith, Brandt, & Shadmehr, Citation2000; Teulings, Contreras-Vidal, Stelmach, & Adler, Citation1997), however to our knowledge not in swimming. In these studies, lower JC indicates greater smoothness of movement.
The present study was designed to investigate whether JC could be used to discriminate between swimming levels when swimming a 50-m front-crawl sprint. We studied two groups of participants, elite and non-elite swimmers, to answer the following research questions:
Is it possible to discriminate between elite and non-elite swimmers based on JC?
Is it possible to discriminate between faster and slower elite and non-elite swimmers based on JC?
Based on previously observed differences in IVV (Barbosa, Goh, Morais, & Costa, Citation2016; Matsuda et al., Citation2014; Schnitzler et al., Citation2010), we hypothesised that the elite swimmers would swim more smoothly than the non-elite swimmers, and thus have a lower jerk cost. We further hypothesised that within a group of (elite or non-elite) swimmers the faster swimmers would show more jerk than the slower swimmers because swimming faster is associated with greater accelerations and decelerations (Leblanc, Seifert, Tourny-Chollet, & Chollet, Citation2007) and thus greater jerk.
Methods
Participants
Eighteen males participated in this study. We divided the participants into two groups: elite and non-elite. Elite participants were recruited from the top 20 rankings of the Netherlands in 50-m long course freestyle (obtained from www.swimrankings.net on January 1st, 2016). For the non-elite group, we invited three water polo teams from the Amsterdam region to participate in the study. All teams competed on the second or third national level, implying that they belonged to the best 30 teams of the Netherlands. shows the group-averaged participant characteristics.
Table 1. Characteristics of the elite and non-elite participants, mean ± SD.
The study was approved by the Ethical Committee of the Department of Human Movement Sciences, Vrije Universiteit Amsterdam, The Netherlands. Prior to the measurements, all participants received verbal instructions about the procedure of the study and signed an informed consent form. Written parental consent was obtained for participants younger than 18 years of age (n = 1).
Experimental design
The participants were welcomed in the swimming pool and informed verbally about the measurement procedure. They performed their own regular warm-up protocol both on land and in water for approximately 30 min. This procedure was followed to ensure optimal performance during the experiment proper, assuming that the participants knew well how to prepare for an imminent competition or important test (Balilionis et al., Citation2012). After the warm-up, the participants left the pool, the back of the participant was dried and disinfected with denatured alcohol (70%), and the sensor was placed on the participant’s back. The participants went back into the water holding a starting position with both feet on the wall and the arms extended in front of the body. One of the researchers provided the start signal, after which the participant performed a 50-m all-out front-crawl swimming sprint without imposing any constraints on kicking and breathing pattern or the number of underwater kicks. After the 50-m sprint, the researcher removed the sensor and the participant continued with his own cooling-down before leaving the pool.
Methodology
All measurements were performed in a 50=m indoor swimming pool with a constant water temperature of 27°C (Sloterparkbad, Amsterdam, The Netherlands). The same sensor and sensor position as described previously was used: a Zephyr Bioharness 3, placed on the upper back midway between the inferior angle of the left and right scapula using Tegaderm semi-permeable film dressing (10 cm×12 cm) (Ganzevles et al., Citation2017). This sensor consists of a tri-axial accelerometer with a range of −16 g to +16 g and a 12-bit resolution, which gathered and saved data in the binary format at a sample frequency of 100 Hz. The sensor had the following characteristics: dimensions 28 mm × 7 mm, weight 18 g, operating temperature between −30 and +60°C and waterproof up to 1 m (International Protection Marking 67). The gathered data were stored in the system and transferred via a USB unit to a computer after the measurement was completed.
All data processing was performed in Matlab (The Mathworks™, Natick, MA, USA, R2017a, 9.2.0.556344, 64 bit). First, the raw data were converted from binary format to acceleration in m/s2 following the specifications provided by the manufacturer. The data from the first arm stroke until the final stroke before the finish were used for further processing. LT, SR and SC were calculated from the accelerometer data using the algorithms described in Ganzevles et al. (Citation2017) Next, the data around the z-axis were filtered using a second-order Butterworth band-pass filter between 0.1 and 1 Hz to detect zero-crossings. The zero-crossings were defined as the change from negative to positive around the z-axis and used as an operational definition of the begin and end of a stroke. The z-axis was defined in the mediolateral direction with respect to the swimmer, and thus mainly reflected the roll movements during front-crawl. Mainly, because an exact outline of the sensor with the roll movement of the swimmer was not possible in practice, the z-axis also reflected some pitch and lateral movement. However, the z-axis showed the largest amplitude and was therefore chosen to determine the start and end of a stroke cycle ().
Figure 1. Data processing from raw data to normalised strokes. (a) The raw data in the x- (dark grey), y- (light grey) and z-direction (grey). (b) The filtered data in y-direction and the zero-crossings that were detected (black dots). (c) The normalised strokes obtained from the Euclidean norm using the zero-crossings depicted in (b), each line represents a different stroke. (d) The average, normalised stroke (black line) and corresponding standard deviation (dotted lines).
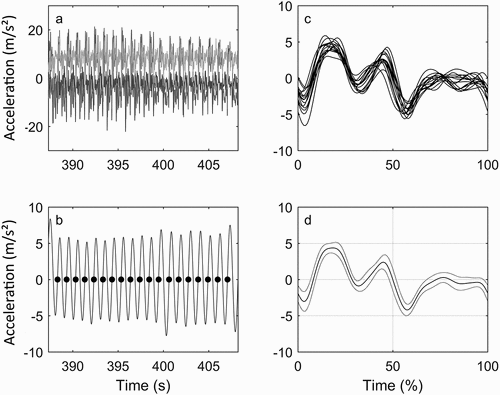
From the raw acceleration data, the Euclidean norm, EN, was obtained to calculate intra-cyclic parameters. EN was filtered with a second-order Butterworth band-pass filter (0.1–5 Hz). From the EN, the JC was calculated, using an adapted version of formula (1):
in which a is the acceleration of the swimming body measured on the back of the participant (Ganzevles et al., Citation2017). The JC was subsequently averaged per lap for each swimmer. In addition, each stroke of each swimmer was normalised over time creating strokes on a 0–100%-time base. From these strokes, the average stroke and corresponding standard deviation (SDACC) were calculated ().
Statistical analysis
All statistical analyses were conducted in SPSS (IBM, version 23). The data were tested for normality using the Shapiro–Wilk test. The significance level was set at 0.05. All data are expressed as means with SD in between brackets or following the ± sign.
An independent-samples t-test was conducted to compare elite and non-elite participants, in case the data were distributed normally. A non-parametric Mann–Whitney test was conducted when the data were not normally distributed. The relationship between JC and LT was determined using simple linear regression analysis. In addition, a stepwise multiple linear regression was performed to assess the influence of SR, SC and JC on LT and to select the best model for the whole group of participants and for both groups separately. Finally, the group-averaged strokes of elite and non-elite participants were compared visually ()) in order to gain an impression on how differences in JC were related to differences in the stroke mechanics.
Results
There was a significant difference between groups for all parameters except SR: non-elite participants swam the 50-m front-crawl sprint slower, with more strokes, higher jerk cost and more variation between strokes, but similar stroke rates compared to the elite swimmers. shows the means and standard deviations for both groups of participant together with the outcomes of the statistical tests.
Table 2. Summary of the results for the elite and non-elite swimmers, including the results of the statistical tests, mean ± SD.
We performed a simple linear regression to predict LT based on JC for the complete group of participants and for the elite and non-elite separately. No significant regression equations were found for the whole group (F(1,16) = 3.53, p = 0.079, R2 = 0.18 SEE = 1.81, equation: LT = 25.01 + 0.01·JC), for the non-elite (F(1,7) = 0.60, p = 0.465, R2 = 0.08, SEE = 1.37, equation: LT = 29.23–5.22 · 10−3JC), or for the elite swimmers (F(1,7) = 2.40, p = 0.165, R2 = 0.26, SEE = 0.51, equation: LT = 26.28–2.10 · 10−3JC, ).
Figure 2. The relation between JC for each swimmer and performance level. NE = non-elite swimmers represented by the black dots and E = elite swimmers represented by the grey triangles. The formulas for the linear relations with the corresponding R2 are given within the rectangles.
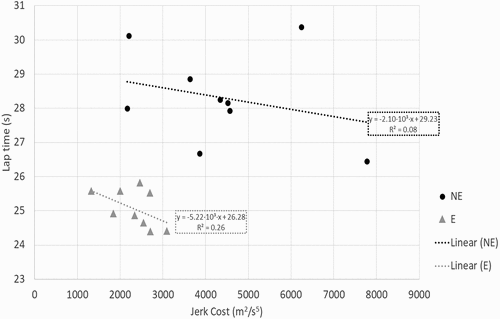
In addition, a stepwise multiple linear regression was performed to predict LT based on SR, SC and JC. For the entire group of participants a significant regression was found (F(3,14) = 40.01, p < 0.001), with an R2 of 0.90 and standard error of the estimate (SEE) of 0.69s. The regression equation was LT = 27.85–0.25(SR) + 0.61(SC)—6.67 · 10–5(JC), where SR is measured in strokes/min, SC in strokes and JC in m2/s5. Both SR and SC were significant predictors of LT, whereas JC was not, with p-values of p < 0.001, p < 0.001 and p = 0.581, respectively.
For the non-elite swimmers a significant regression equation was found between LT and SR, SC and JC (F(3,5) = 12.82, p = 0.009), with an R2 of 0.89 and standard error of the estimate (SEE) of 0.57 s. The regression equation was LT = 34.15–0.38(SR) + 0.67(SC)—7.37 · 10–5(JC). Both SR and SC were significant predictors of LT, whereas JC was not with p-values of p = 0.002, p = 0.008 and p = 0.572, respectively.
Again, a significant regression was found between LT and SR, SC and JC for the elite swimmers: F(3,5) = 10.466, p = 0.014 with an R2 of 0.863 and standard error of the estimate (SEE) of 0.26 s. The regression equation was LT = 29.841–0.110(SR) + 0.263(SC)—6.28 · 10–4(JC). In contrast to the regression for the whole group and the non-elite swimmers, SR and JC were significant predictors of LT, whereas SC was not (p = 0.008, p = 0.0018 and p = 0.263, respectively).
Interestingly, the build-up of R2 differed considerably for the three models (). Note that the influence of SC is reduced from left to right; the influence of SR is only visible within the groups of non-elite and elite swimmers and JC only for the elite swimmers.
Figure 3. R2 (in %) of the three different regression models and the parts that were explained by stroke rate (SR, in grey), count of stroke cycles per lap (SC, in dark grey) and jerk cost (JC, in light grey), depicted from bottom to top. The partial R2 (in %) for the variables is indicated by the numbers in white. The three models were for the entire group of swimmers (All), the non-elite (NE) and the elite swimmers (E).
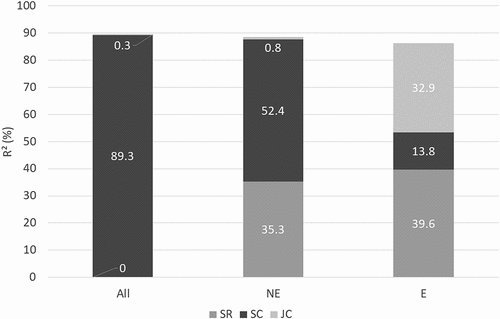
depicts two typical examples of average strokes. shows the average acceleration and average jerk pattern of a non-elite swimmer, and show these for an elite swimmer. The first noteworthy difference is that the amplitude of the acceleration signal seems larger in the non-elite compared to the elite swimmer. Secondly, the elite swimmer shows more peaks in both the acceleration and the jerk pattern. Finally, the non-elite swimmer has a larger SDACC than the elite swimmer.
Figure 4. Average stoke patterns of a non-elite (a and b) and an elite swimmer (c and d). (a) and (c) show the acceleration, (b) and (d) the jerk. The average stroke patterns are indicated by the black lines, the corresponding standard deviations by the dotted lines. The strokes have been time-normalised from 0% to 100%.
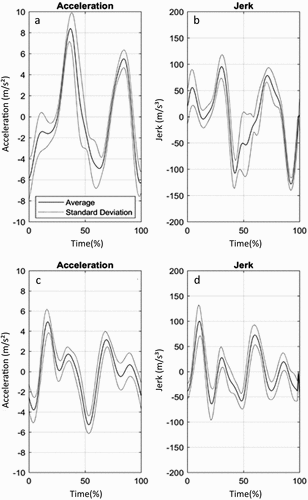
The observed differences were tested statistically on a group level. The difference in amplitude between the non-elite (M = 14.01, SD = 2.45) and elite swimmers (M = 9.91, SD = 1.37) indeed proved to be significant (t(16) = 4.371, p< 0.001, 95% CI of the difference 2.11–6.08 and effect size 2.06). In addition, we counted the number of peaks in the acceleration signal with a minimal prominence of 2 m/s2 and in the jerk signal with a prominence of at least 50 m/s3 for each participant. We found a median count of peaks of 2 and 3 for the non-elite and 3 and 4 for the elite swimmers in the acceleration and jerk signal, respectively. Finally, the difference in SDACC was confirmed on a group level, see : non-elite M = 1.34, SD = 0.25, elite M = 1.07, SD = 0.19; t(16) = 2.54, p = 0.022, 95% CI of the difference 0.04–0.49 and effect size 1.20.
Discussion and implications
In the present study, two hypotheses were tested: 1) elite swimmers swim more smoothly than non-elite swimmers (the water polo players), as reflected in a significantly lower jerk cost, 2) the fastest swimmers swim smoother, with less jerk than the slower swimmers in each group when swimming a 50-m front-crawl sprint. The first hypothesis was confirmed: the elite swimmers had significantly lower levels of JC compared to the non-elite swimmers. These findings are in accordance with the literature. Research in golf (Choi et al., Citation2014) and running (Hreljac, Citation2000) demonstrated that elite athletes show smoother movement patterns than non-elite swimmers. The differences observed between the elite and non-elite swimmers in the present study are relatively large compared to the findings observed in other studies: the JC of the non-elite swimmers was almost twice as high as the JC of the elite swimmers, while elite runners had a JC of about 80% of the non-runners (Hreljac, Citation2000). In golf, similar differences were found (Choi et al., Citation2014). It is unlikely that the differences found in this study are due to training for different sports, since Hreljac (Citation2000) did not find similar differences between non-runners who participated in different sports (e.g., tennis, hockey, soccer and weight training). An explanation for the differences between activities on land and swimming may be found in the fact that swimmers have to propel themselves forward through water. This is very different from moving on land through air. The efficiency of movement is much lower due to the kinetic energy lost to setting water in motion (Ingen Schenau & Cavanagh, Citation1990). Swimmers with a higher skill level usually have a higher propelling efficiency (Craig & Pendergast, Citation1979; Schleihauf, Citation1986). This is consistent with the finding of Toussaint (Citation1990) that competitive swimmers are characterised by a higher propelling efficiency than triathlon swimmers as measured with the measure active drag (MAD) system. Specifically, Toussaint found a propelling efficiency of 62.5 (SD = 7.3)% for the competitive swimmers and 45.6 (SD 5.6)% for the triathlon swimmers. We might assume that the differences between elite swimmers and non-elite swimmers are similar and thus the differences in JC are due to the aquatic environment and the technique and skill level of the participants.
A remark should be made about the lack of restrictions on kicking, breathing pattern and the number of underwater kicks in the current study. We did not impose any restrictions, since we were interested primarily in the different technical solutions that would be chosen by elite and non-elite swimmers while swimming at the water surface. Therefore, the underwater kicks were removed from the data by the algorithms. A swimmer’s technique encompasses all bodily movements that are taking place, including head, torso, arm, leg and breathing movements (Costill, Lee, & D’Acquisto, Citation1987). Thus, the lack of restrictions will lead to different technical solutions performed by the participants. We assumed the elite swimmers would swim more smoothly than the non-elite swimmers, which is confirmed by the lower JC.
Similarly, fatigue during the 50-m sprint has to be discussed. The elite swimmers were trained to perform precisely the task of the current study. The non-elite swimmers, in contrast, were waterpolo players of lower level who trained for 5 h per week and participated in waterpolo competitions on a regular basis. Moreover, they were trained to perform multiple sprints during those competitions. Therefore, we assumed that both groups of swimmers were able to complete a 50-m all-out sprint and would have similar levels of fatigue with a similar effect on the intra-cycle variability. The extant literature is unclear on the effects of fatigue on the intra-cycle variability. Tella et al. (Citation2008) found a clear difference in IVV between a rested 25-m sprint and a fatigued 25-m sprint. On the other hand, Alberty et al. (Citation2005) found no differences in IVV when comparing each 50 m of a 200-m freestyle race. The methods used in the current study (the stroke by stroke analysis and the JC) might be interesting for future research on intra-cyclic variability, provided a specific protocol will be designed to answer questions about the effects of fatigue.
The second hypothesis was confirmed for the elite group of swimmers only. The results of a multiple linear regression analysis indicated that the lap time of elite swimmers can be explained by JC for 32.9%, in such a way that for each m2/s5 increase of JC, LT decreases with 6.28 · 10–4 s. This indicates that higher JC and thus lower smoothness occur when swimming at higher speed within the elite swimming group. For the non-elite swimmers, an increase of JC did not have a significant effect on lap time with an R2 of only 0.8%. Based on the results of the multiple linear regression, the non-elite swimmers should focus on lower stroke counts and higher stroke rates (with a significant effect on lap time of, respectively, 52.4% and 35.3%). In general, as was clear from the analysis of the entire group, one should focus on decreasing the stroke count.
It is not surprising that higher jerk cost is related to faster swimming from a mechanical point of view. If a person is swimming fast, he or she has to perform at the top range of his or her ability. High accelerations will be required to reach a certain speed, while a higher speed will lead to more drag and more drag to greater decelerations (Toussaint & Beek, Citation1992). To maintain this cycle, a higher jerk cost is inevitable, resulting in a decrease in swimming smoothness.
The elite swimmers performed in a different and smaller range of lap times. This might be seen as a limitation of the current study, because, as explained, jerk cost depends on swimming speed. However, even at different speeds, the difference between elite and non-elite swimmers is striking. Elite swimmers clearly swam smoother than non-elite swimmers (). For the mechanical reasons given above, one might expect that these differences will increase when swimmers would swim at a similar speed. Elite swimmers would deliver the same performance in a much more efficient way (Toussaint, Citation1990). In addition, there is a different relationship between jerk cost and lap time between both groups. In the stepwise, multiple linear regression analysis it became apparent that higher jerk cost contributes significantly to faster lap times for the elite swimmers, but not for the non-elite swimmers. It seems that the elite swimmers swam more explosively. This is in accordance with the study of Leblanc et al. (Citation2007) in which it appeared that one of the discriminating factors of skill level is the ability to ‘feel the water’ to ultimately increase the intra-cyclic acceleration time.
Another limitation is the number of participants that participated in the present study. We are aware that at least 16 participants should be allocated in both groups in order to perform an independent t-test. Unfortunately, the number and availability of elite sprinters in the Netherlands are limited (see ). However, the results show a striking difference between both groups. The variation within the group of elite swimmers was low, while the variation in the group of non-elite swimmers was markedly higher. We expect that with a higher number of participants, the variation in the group of elite swimmers will be preserved and the variation in the group of non-elite swimmers will decrease, which will further confirm the first hypothesis and might lead to a confirmation of the second hypothesis for both groups.
The in-depth analysis of the average acceleration provides more information about the differences between elite and non-elite swimmers (). The elite swimmers showed smaller peak accelerations than the non-elite swimmers, but with more peaks within a stroke cycle. Furthermore, the variation around the average stroke was less. This smaller variation around a certain pattern constitutes by itself an indication of a higher skill level (Wagner, Pfusterschmied, Klous, von Duvillard, & Müller, Citation2012). Because of the lack of video recordings in the present study, it is unclear what exactly occurred during these peaks in the pattern. We can therefore only speculate about what happened. If it was a kicking issue, six peaks or at least three peaks would be expected, since kicking is usually performed with a six-beat pattern (Sanders & Psycharakis, Citation2009). Therefore, it is more likely an effect of different pulling strategies. In the literature on swimming the arm cycle is usually divided into four phases: entry, pull, push, recovery (McCabe & Sanders, Citation2011). As there is no or very little propulsion in the entry and recovery phase, the four observed peaks (see ) were likely generated in the pull and push phase. Apparently, something happens that causes the acceleration to drop to almost 0 m/s2, possibly the recovery of the other arm. This would mean that the main difference between elite and non-elite swimmers occurs in the first part of the stroke because a peak in the acceleration was observed for both elite and non-elite swimmers at around 40% and 80% of the complete stroke. Similar differences were found between sprint and distance swimmers in the pull phase as a percentage of the duration of the whole stroke during sprints. Sprinters spend relatively less time in that phase of the stroke (McCabe & Sanders, Citation2011).
For the daily training situation, we concluded from the multiple linear regression analysis that:
In general, one should focus on the stroke count per lap, since variation in lap time was explained for 89.3% by variation in stroke count on a group level;
Non-elite swimmers should focus on the ability to move with a higher stroke rate and lower stroke count per lap (with R2 of, respectively, 35.3% and 52.4%);
Elite swimmers should focus on the combination of the abilities to reach high stroke rates, low stroke count and to achieve high jerk cost levels (with R2 of, respectively, 39.6%, 13.8% and 32.9%).
An important aspect of our study is that the jerk cost was measured with one accelerometer on the back of the swimmer in contrast to studies in other sports where the jerk cost was measured with several markers and video recordings (Choi et al., Citation2014; Hreljac, Citation2000). This method has the benefit of the practicality of use in the daily training situation for longer periods, i.e., a complete training session (Ganzevles et al., Citation2017). This opens up a wide range of possibilities for coaches and researchers. For example, it should be possible to determine if there is a daily variation in the smoothness and if and how this relates to the feel of the swimmers. This might provide coaches with an additional and readily measurable index of technique that can be used in the training of elite swimmers. If a swimmer is able to swim at the same speed with lower jerk cost, this might be beneficial energetically, in that lower jerk will lead to smaller energy expenditure. On the other hand, the ability to produce a higher jerk cost might lead to faster swimming.
Conclusion
This study investigated the use of jerk cost as a measure to discriminate groups of swimmers of different levels and to discriminate between swimmers of a certain level. The results show that it is possible to discriminate elite from non-elite swimmers: elite swimmers swim smoother than non-elite swimmers. Additionally, more accelerations and decelerations are necessary to swim at higher speed, which is reflected in a higher jerk cost.
Acknowledgments
We would like to thank Jaap Harlaar and Robert Ton for their assistance in the normalisation of the strokes, Levy Hoeven for his assistance in performing the measurements and the participating swimmers for their time and effort. All three were affiliated to the Department of Human Movement Sciences, Faculty of Behavioural and Movement Sciences, Free University Amsterdam, The Netherlands, during the study’s conductance.
Disclosure statement
No potential conflict of interest was reported by the author(s).
Correction Statement
This article has been republished with minor changes. These changes do not impact the academic content of the article.
Additional information
Funding
References
- Alberty, M., Sidney, M., Huot-Marchand, F., Hespel, J. M., & Pelayo, P. (2005). Intracyclic velocity variations and arm coordination during exhaustive exercise in front crawl stroke. International Journal of Sports Medicine, 26, 471–475. doi:10.1055/s-2004-821110
- Balilionis, G., Nepocatych, S., Ellis, C. M., Richardson, M. T., Neggers, Y. H., & Bishop, P. A. (2012). Effects of different types of warm-up on swimming performance, reaction time, and dive distance. Journal of Strength and Conditioning Research, 26, 3297–3303. doi:10.1519/JSC.0b013e318248ad40
- Barbosa, T. M., Goh, W. X., Morais, J. E., & Costa, M. J. (2016). Variation of linear and nonlinear parameters in the swim strokes according to the level of expertise. Motor Control, 21, 1–27. doi:10.1123/mc.2015-0097
- Choi, A., Bin, J. S., Oh, E., & Mun, J. H. (2014). Kinematic evaluation of movement smoothness in golf: Relationship between the normalized jerk cost of body joints and the clubhead. Biomedical Engineering Online, 13, 20. doi:10.1186/1475-925X-13-20
- Costill, D. L., Lee, G., & D’Acquisto, L. J. (1987). Video-computer assisted analysis of swimming technique. The Journal of Swimming Research, 3, 5–9.
- Craig, A. B., & Pendergast, D. R. (1979). Relationships of stroke rate, distance per stroke, and velocity in competitive swimming. Medicine and Science in Sports, 11, 278–283.
- Dadashi, F., Crettenand, F., Millet, G. P., & Aminian, K. (2012). Front-crawl instantaneous velocity estimation using a wearable inertial measurement unit. Sensors, 12, 12927–12939. doi:10.3390/s121012927
- Davey, N. P., Anderson, M., & James, D. A. (2008). Validation trial of an accelerometer-based sensor platform for swimming. Sports Technology, 1, 202–207. doi:10.1002/jst.59
- Ganzevles, S., Vullings, R., Beek, P. J. P. J., Daanen, H., & Truijens, M. (2017). Using tri-axial accelerometry in daily elite swim training practice. Sensors, 17, 990. doi:10.3390/s17050990
- Hogan, N., & Sternad, D. (2009). Sensitivity of smoothness measures to movement duration, amplitude, and arrests. Journal of Motor Behavior, 41, 529–534. doi:10.3200/35-09-004-RC
- Hreljac, A. (2000). Stride smoothness evaluation of runners and other athletes. Gait and Posture, 11, 199–206. doi:10.1016/S0966-6362(00)00045-X
- Hreljac, A., & Martin, P. E. (1993). The relationship between smoothness and economy during walking. Biological Cybernetics, 69, 213–218. doi:10.1007/BF00198961
- Ingen Schenau, G. J. V., & Cavanagh, P. R. (1990). Power equations in endurance sports. Journal of Biomechanics, 23, 865–881. doi:10.1016/0021-9290(90)90352-4
- Leblanc, H., Seifert, L., Tourny-Chollet, C., & Chollet, D. (2007). Intra-cyclic distance per stroke phase, velocity fluctuations and acceleration time ratio of a breaststroker’s hip: A comparison between elite and nonelite swimmers at different race paces. International Journal of Sports Medicine, 28, 140–147. doi:10.1055/s-2006-924205
- Magalhaes, F. A., De Vannozzi, G., Gatta, G., & Fantozzi, S. (2015). Wearable inertial sensors in swimming motion analysis: A systematic review. Journal of Sports Sciences, 33, 732–745. doi:10.1080/02640414.2014.962574
- Matsuda, Y., Yamada, Y., Ikuta, Y., Nomura, T., & Oda, S. (2014). Intracyclic velocity variation and arm coordination for different skilled swimmers in the front crawl. Journal of Human Kinetics, 44, 67–74. doi:10.2478/hukin-2014-0111
- McCabe, C. B., & Sanders, R. H. (2011). Kinematic differences between front crawl sprint and distance swimmers at a distance pace. Journal of Sports Sciences, 29, 115–123. doi:10.1080/02640414.2012.660186
- Nigg, B. M. (1983). Selected methodology in biomechanics with respect to swimming. Biomechanics and Medicine in Swimming V, 72–80.
- Sanders, R. H., & Psycharakis, S. G. (2009). Rolling rhythms in front crawl swimming with six-beat kick. Journal of Biomechanics, 42, 273–279. doi:10.1016/j.jbiomech.2008.10.037
- Schleihauf, R. E. (1986). Swimming skill: A review of basic theory. Journal of Swimming Research, 2, 11–20.
- Schnitzler, C., Seifert, L., Alberty, M., Chollet, D., Saint, A. M., Saint, A. M., & Aignan, M. S. (2010). Hip velocity and arm coordination in front crawl swimming. International Journal of Sports Medicine, 31, 875–881. doi:10.1055/s-0030-1265149
- Smith, M. A., Brandt, J., & Shadmehr, R. (2000). Motor disorder in Huntington’s disease begins as a dysfunction in error feedback control. Nature, 403, 544–549. doi:10.1038/35000576
- Sparrow, W. A., & Newell, K. M. (1998). Metabolic energy expenditure and the regulation of movement economy. Psychonomic Bulletin & Review, 5, 173–196. doi:10.3758/BF03212943
- Stamm, A., James, D. A., Burkett, B. B., Hagem, R. M., & Thiel, D. V. (2013). Determining maximum push-off velocity in swimming using accelerometers. Procedia Engineering, 60, 201–207. doi:10.1016/j.proeng.2013.07.067
- Stamm, A., & Thiel, D. V. (2015). Investigating forward velocity and symmetry in freestyle swimming using inertial sensors. Procedia Engineering, 112, 522–527. doi:10.1016/j.proeng.2015.07.236
- Sweetenham, B., & Atkinson, J. (2003). Championship swim training. Champaign, IL: Human Kinetics.
- Tella, V., Toca-Herrera, J. L., Gallach, J. E., Benavent, J., González, L. M., & Arellano, R. (2008). Effect of fatigue on the intra-cycle acceleration in front crawl swimming: A time-frequency analysis. Journal of Biomechanics, 41, 86–92. doi:10.1016/j.jbiomech.2007.07.012
- Teulings, H. L., Contreras-Vidal, J. L., Stelmach, G. E., & Adler, C. H. (1997). Parkinsonism reduces coordination of fingers, wrist, and arm in fine motor control. Experimental Neurology, 146, 159–170. doi:10.1006/exnr.1997.6507
- Toussaint, H. M. (1990). Differences in propelling efficiency between competitive and triathlon swimmers. Medicine and Science in Sports and Exercise, 22, 409–415. doi:10.1249/00005768-199006000-00020
- Toussaint, H. M., & Beek, P. J. (1992). Biomechanics of competitive front crawl swimming. Sports Medicine, 13, 8–24. doi:10.2165/00007256-199213010-00002
- Van Houwelingen, J., Roerdink, M., Huibers, A. V., Evers, L. L. W., & Beek, P. J. (2017). Pacing the phasing of leg and arm movements in breaststroke swimming to minimize intra-cyclic velocity fluctuations. PloS One, 12, e0186160. doi:10.1371/journal.pone.0186160
- Viviani, P., & Flash, T. (1995). Minimum-jerk, two-thirds power law, and isochrony: Converging approaches to movement planning. Journal of Experimental Psychology. Human Perception and Performance, 21, 32–53. doi:10.1037/0096-1523.21.1.32
- Wagner, H., Pfusterschmied, J., Klous, M., von Duvillard, S. P., & Müller, E. (2012). Movement variability and skill level of various throwing techniques. Human Movement Science, 31, 78–90. doi:10.1016/j.humov.2011.05.005