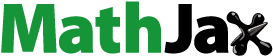
ABSTRACT
Multi-planar forces and moments are known to injure the anterior cruciate ligament (ACL). In ACL injury risk studies, however, the uni-planar frontal plane external knee abduction moment is frequently studied in isolation. This study aimed to determine if the frontal plane knee moment (KM-Y) could classify all individuals crossing a risk threshold compared to those classified by a multi-planar non-sagittal knee moment vector (KM-YZ). Recreationally active females completed three sports tasks—drop vertical jumps, single-leg drop vertical jumps and planned sidesteps. Peak knee abduction moments and peak non-sagittal resultant knee moments were obtained for each task, and a risk threshold of the sample mean plus 1.6 standard deviations was used for classification. A sensitivity analysis of the threshold from 1–2 standard deviations was also conducted. KM-Y did not identify all participants who crossed the risk threshold as the non-sagittal moment identified unique individuals. This result was consistent across tasks and threshold sensitivities. Analysing the peak uni-planar knee abduction moment alone is therefore likely overly reductionist, as this study demonstrates that a KM-YZ threshold identifies ‘at risk’ individuals that a KM-Y threshold does not. Multi-planar moment metrics such as KM-YZ may help facilitate the development of screening protocols across multiple tasks.
Introduction
External forces applied to athletes during dynamic tasks are balanced by internal muscle forces which in combination result in net resultant moments acting across joints. The motion of an athlete is therefore the result of net resultant moments acting about multiple joints through the kinematic and kinetic chain. In the context of anterior cruciate ligament (ACL) injury, the net resultant moments around the knee are known to contribute to ACL strain. In fact, moments resolved into individual anatomical planes stress the ACL differently due to its oblique nature. In vitro testing has shown multi-planar force and moment combinations cause the greatest ACL strain (Bates et al., Citation2019; Kiapour et al., Citation2016; Markolf et al., Citation1995; Shin et al., Citation2011). Specifically, the combination of the internal rotation and the abduction moment cause the greatest ACL strain in the presence of an anterior shear force (Kiapour et al., Citation2016; Markolf et al., Citation1995). This multiplanar injury mechanism has also been supported by in-silico (McLean et al., Citation2004) and in-vivo(Koga et al., Citation2010; Quatman et al., Citation2010) observations. The relevance of mutli-planar loads in examining ACL injury is therefore unequivocal.
In-vivo research into ACL injury risk factors often considers multi-planar challenges to knee through dynamic tasks during which ACL injuries are known to occur. Typical tasks include the bilateral drop vertical jump, single-leg drop vertical jumps and sidestepping. Within these tasks, resultant knee moments are resolved into an orthogonal coordinate system according to an anatomical reference frame to consider moment components in individual planes. The external knee abduction moment specifically (also known as the knee valgus moment or internal knee adduction moment) has been implicated as a predictive biomechanical risk factor for ACL injury (Hewett et al., Citation2005) yet subsequent prospective studies and a recent meta-analysis contend this (Cronström et al., Citation2020; Krosshaug et al., Citation2016; Leppänen et al., Citation2017). This is perhaps not surprising given that this moment is inherently variable (Malfait et al., Citation2014; Sankey et al., Citation2015), and that one variable alone is unlikely to separate injured from uninjured athletes (Bahr, Citation2016). Nevertheless, the knee abduction moment continues to be a primary variable of interest in the context of ACL injury risk. It has also become common practice to report the knee abduction moment without consideration of the other components contributing to the resultant knee moment. A recent systematic review in sidestepping found that all 23 studies considered had examined the knee abduction moment with only 3 of those considering other moment components (Donelon et al., Citation2020).
Contrary to a multi-planar ACL injury mechanism, it is somewhat surprising that so little focus is given to the transverse plane rotation moment. Given that the transverse plane rotation moment tends to be quite small and variable, it may be that consideration of this moment component has been deemed insufficiently relevant and/or reliable. However, the knee abduction moment alone may fail to represent the complexity of the multi-planar loading experienced in dynamic tasks (Robinson et al., Citation2015), justifying future risk factor analyses that incorporate a multi-component approach. In the context of developing ‘at-risk’ classification as part of injury screening, we propose that if the transverse plane moment component was redundant for multi-component classification, then the knee abduction moment alone would identify all ‘at risk’ individuals.
The aim of this study was to evaluate if the uni-planar knee abduction moment can identify all individuals with high multi-planar (non-sagittal) knee joint moments. We address this by comparing the classification of ‘at risk’ individuals using the knee abduction moment alone versus using a non-sagittal resultant moment vector. We hypothesise that the uni-planar knee abduction moment will not identify all individuals with high multi-planar (non-sagittal) knee joint moments.
Methods
Participants
Forty-five recreationally active female participants (mean SD: age, 22.1
3.7 years; mass, 64.0
10.6 kg) volunteered to participate in the study. All were free of lower limb injuries for at least 12 months and regularly participating in highly-dynamic sports such as football, handball, field hockey, basketball and netball at least twice per week. Ethical approval was granted by the university ethics committee (14/SPS/032), and informed consent was obtained prior to testing.
Protocol
After 10 minutes of dynamic warm-up and familiarisation, each participant randomly completed five trials of three tasks. Three tasks were chosen to examine if the results observed were consistent across dynamic tasks commonly used to evaluate ACL injury risk given that between task correlations are not strong (Donohue et al., Citation2015; Jones et al., Citation2014). Adequate rest was given between trials and tasks. A bilateral drop vertical jump (BDVJ) and single-leg drop vertical jump (SLDVJ) were performed maximally from a 30 cm high box. Planned sidestepping (SS) manoeuvres were performed by cutting at 45 after a 10 m straight run. This task was deemed successful when the approach speed was between 4–5 m
s
and the foot landed entirely on the force platform. The SLDVJ and SS tasks were performed for both legs. Whilst unplanned sidestepping provides a greater biomechanical challenge to the knee (Brown et al., Citation2014) this was not possible in this study due to time constraints, as additional measurements were made that are not reported here.
Data analysis
For all tasks, participants landed on 90 × 60 cm force platforms sampling at 1500 Hz (Kistler, Winterthur, Switzerland). Their 3-dimensional movement was captured at 250 Hz (10 Oqus Cameras and QTM v.2.14 Qualisys AB, Gothenburg, Sweden) and 44 spherical markers were used to create and track geometric models of the trunk, pelvis and lower limbs according to the 6 degrees-of-freedom LJMU model (Malfait et al., Citation2014; Vanrenterghem et al., Citation2010). This model has functional knee axes and hip joints. Both marker positions and ground reaction forces were low-pass filtered at 20 Hz (Bisseling & Hof, Citation2006).
Knee joint moments were estimated using inverse dynamics in Visual 3D (v.5.02.30, C-Motion, Germantown, MD, USA) and normalised to body mass. For the BDVJ, and SLDVJ, the peak knee abduction moment (KM-Y) was obtained during the first landing, for the sidestepping trials the peak was obtained during the weight acceptance phase (Dempsey et al., Citation2007). In the few trials where there was no abduction moment, the minimum adduction moment was taken. The peak non-sagittal resultant moment (KM-YZ) was also calculated from the vector sum of the frontal and transverse plane moment components, this therefore reflects undesirable kinetics holistically rather than direction-specific mechanisms. Note that peak values of KM-Y and KM-YZ may therefore not be coincident. All peaks were averaged across the five trials to get a mean response for each leg for each participant.
To evaluate how each moment variable classified individual participants, the KM-Y and KM-YZ for each leg were added to scatter plots for each task separately. Next, a ‘risk threshold’ for this cohort was considered. A threshold of 25.3 Nm was associated with an increased risk of ACL injury in young girls (Myer et al., Citation2015), which corresponded to approximately 0.4 Nmkg
in this cohort, but this was specific to the BDVJ and we deemed it too low for single-leg tasks. Instead, a ‘risk threshold’ of the inter-subject mean +1.6 standard deviations (SD) was primarily considered. In the absence of an externally validated injury risk threshold across multiple tasks, this was thought to be somewhat conservative and would by definition account for 94.5% area under the normal distribution curve. Previous studies have used the range +1 SD to +1.6 SD (Chinnasee et al., Citation2018; Kristianslund & Krosshaug, Citation2013; Robinson et al., Citation2014). As the choice of any arbitrary threshold is subjective we also conducted a sensitivity analysis of the classification by varying the threshold between the mean +1 SD to mean +2 SD to ensure the results were not threshold-specific. A participant’s leg was classed as ‘at risk’ if it exceeded a threshold. It was therefore possible for a single leg for a given task to be classified at ‘at risk’ by exceeding only the KM-Y threshold, only the KM-YZ threshold, or by exceeding both thresholds. To address our hypothesis we were primarily interested to see if any participants exceeded the KM-YZ threshold but not the KM-Y threshold, as this would indicate that they were classed as ‘at risk’ by KM-YZ but not by KM-Y.
Results
The frontal plane moment (KM-Y) did not identify all participants that crossed the risk threshold (). The non-sagittal resultant moment (KM-YZ) identified unique legs and individuals that the KM-Y did not. This result was consistent across all three tasks examined (). Neither KM-Y or KM-YZ identified substantially more than the other. In the BDVJ task, both KM-Y and KM-YZ identified the majority of legs/individuals crossing the threshold (n = 5, 56%); however, this was lower for the SLDVJ (n = 1, 10%) and SS (n = 1, 20%) tasks. The remaining legs were identified by KM-Y only or KM-YZ only. All legs crossing the risk threshold were unique individuals except in the SS task, where one individual exceeded the threshold with both legs. KM-Y identified two individuals ‘at risk’ across multiple tasks, KM-YZ identified three different individuals who were ‘at risk’ across multiple tasks.
Table 1. The number of legs and individuals crossing mean +1.6 SD moment thresholds (KM-Y only, KM-YZ only, or both) in three tasks (BDVJ: bilateral drop vertical jump, SLDVJ: single-leg drop vertical jump, SS: sidestepping).
Figure 1. Scatter plots of the frontal plane knee moment (KM-Y) versus the non-sagittal plane knee moment vector (KM-YZ) for three tasks (BDVJ: bilateral drop vertical jump, SLDVJ: single-leg drop vertical jump, SS: sidestepping). Left legs are blue circles and right legs are red triangles. Thresholds are annotated at the mean + 1.6 standard deviations for each variable. Individuals within the vertical shaded area are classified as ‘at risk’ by KM-Y, those within the horizontal shaded area are classed as ‘at risk’ by KM-YZ. Those in the top right shaded area are classified as ‘at risk’ by both variables. Participants that exceeded any threshold are identified by their participant number. The white numbers in each threshold area are the total legs identified in this area. Note one leg in the SS condition (40) classified by both variables was outside the axis limits so is not shown.
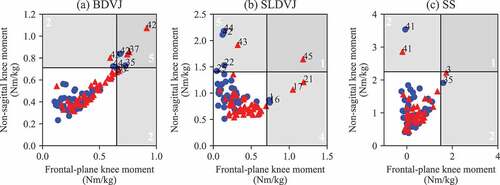
The reported results were generally robust to the risk threshold selected (). Across all tasks when the threshold was made more strict (1.6 SD) the KM-YZ always identified unique participants that the KM-Y did not. Only a threshold mean
1.2 SD in the BDVJ had no unique participants identified by KM-YZ although the majority of participants crossing the threshold were identified by both moment variables.
Figure 2. A sensitivity analysis of the number of legs crossing thresholds of the mean +1 to +2 standard deviations across three tasks (BDVJ: bilateral drop vertical jump, SLDVJ: single-leg drop vertical jump, SS: sidestepping). In the top row the total participants identified are shown for each threshold along with those identified separately by the frontal plane knee moment (KM-Y) and non-sagittal plane knee moment vector (KM-YZ). The second row plots the same total participants identified, but separates out participants that were uniquely identified by the KM-Y and KM-YZ, bars without colour are participants identified by both variables. The presence of cyan bars indicates that some participants are identified only by KM-YZ. Results from the 1.6 SD threshold are presented in table 1.
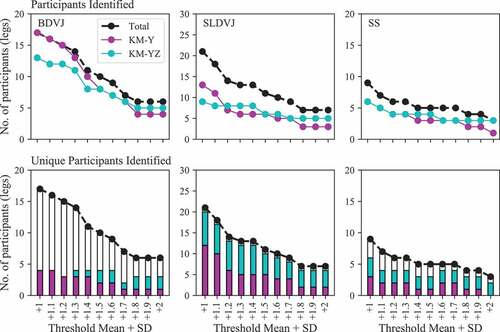
Discussion and implications
The aim of this study was to determine if the knee abduction moment could identify all individuals with high multi-planar knee joint moments. The knee abduction moment did not identify all individuals with high multi-planar knee joint moments. The non-sagittal plane knee moment uniquely identified individuals that the knee abduction moment did not across all tasks. These results were robust to changes in the risk threshold selected.
Identifying athletes ‘at risk’ of ACL injury is a challenge (Bahr, Citation2016). This study has shown that the knee abduction (KM-Y) alone does not identify all individuals that have high multi-planar loading. This is perhaps to be expected given (1) the knee abduction moment in vivo is inherently variable (Malfait et al., Citation2014; Sankey et al., Citation2015), (2) moment components are sensitive to anatomical co-ordinate system definition, and clearly (1) and (2) interact. Furthermore, given that the KM-YZ identified unique individual there would appear to be unique and relevant information about individuals with high multi-planar moments that is not always captured using KM-Y alone. This therefore provides initial evidence to suggest that the KM-YZ may be as valuable as KM-Y to identify at-risk individuals. A similar concept explored during walking suggested combining multiple moment components within a regression model showed more effective prediction of medial compartment loading than the adduction moment alone (Manal et al., Citation2015). The future consideration of KM-YZ, would help to identify individuals with large transverse plane but small frontal plane moments yet still exhibiting high multi-planar knee joint moments. This is important because studies frequently report frontal plane moments but not other moment components (Donelon et al., Citation2020). To be clear, as both KM-Y and KM-YZ identified unique individuals we do not advocate investigating one variable at the expense of the other. Having unique individuals identified by KM-Y is perhaps counter-intuitive but is explained by the magnitude of the transverse plane moment; those individuals identified by KM-Y but not KM-YZ would have had smaller transverse plane (Z) moments. Any individuals with a high multi-planar knee joint moment should be identified by an ACL-specific screening protocol. We therefore sought to verify if these results might also extend to males. The same protocol was repeated for a separate male dataset (n = 46) from the same university. The main result that KM-Y fails to identify all individuals with high multi-planar (non-sagittal) moments was supported (see supplementary material).
The three tasks considered in this study generally identified different legs as ‘at risk’ though there were some exceptions. Task specificity adds to the complexity of screening for undesirable ‘at risk’ behaviours in athletes (Bahr, Citation2016; Donohue et al., Citation2015). All tasks had individuals that were not identified by the frontal plane moment only. Qualitatively, in the BDVJ the frontal plane moment increased linearly with the non-sagittal plane moment and had the greatest number of individuals classified by both variables. The SLDVJ and SS tasks, however, showed no clear relationship between the frontal plane and non-sagittal plane suggesting that it would be more difficult to identify individuals with high multi-planar moments from the frontal plane moment only. The SLDVJ also showed some between limb differences that were also seen in a separate male dataset (see supplementary material), which warrants further investigation as limb dominance effects remain inconclusive (Dos’Santos et al., Citation2019). Task specificity is supported by multiple studies in the literature which show a wide range of correlations/differences for knee moments according to the exact nature of the task comparisons made (Critchley et al., Citation2020; Donohue et al., Citation2015; Heebner et al., Citation2017; Jones et al., Citation2014; Nedergaard et al., Citation2020; Taylor et al., Citation2016). Risk classification differences across tasks are also possible (Chinnasee et al., Citation2018; Kristianslund & Krosshaug, Citation2013; Sharir et al., Citation2017). For screening to become feasible both the variable/s of interest and multi-task behaviours need to be considered.
Our results support the notion that analysing the peak knee abduction moment is convenient but likely overly reductionist. Inclusion of the transverse plane moment into a multi-component non-sagittal plane vector would be a small step towards more complete representation of the ACL injury mechanism. This method however considers all moments within a given plane rather than direction-specific moments (cf. Podraza et al., Citation2018) which could provide more targeted undesirable load combinations. Multi-planar knee joint loading is sometimes referred to as analysing separate peak uni-planar moment components in isolation through scalar (uni-variate) analysis (Donelon et al., Citation2020). Our results consider multi-planar loading by combining moment components to find a multi-planar peak moment. Extracting peak loads only however fails to consider either the rate of loading or the timings of key multi-planar moment characteristics (which may not occur coincidently with the peak knee abduction moment). Techniques such as statistical parametric mapping (Pataky, Citation2012; Pataky et al., Citation2013) and functional data analysis (Ramsay & Silverman, Citation2007) can provide a holistic consideration of the moment vectors. Our earlier work examining time-varying (multivariate) knee moment vectors has identified loading effects that individual moment components alone cannot (Robinson et al., Citation2015). These approaches may therefore provide further insights into multi-planar loading and risk classification research paradigms. Other promising methods include multi-planar and multi-joint support moments which also warrant further investigation (Podraza et al., Citation2018; Winter, Citation2009). Whilst the consideration of all such methods and parameters may have merit, their value as ACL injury risk factors or for ACL injury screening is yet to be determined.
This study has a number of limitations. A first limitation is that these data were not complimented with injury registrations and so these results cannot be verified against actual ACL injury risk. It remains unknown therefore if the non-sagittal moment vector may be a meaningfully improved predictor of ACL injuries than the knee abduction moment alone. These results are also specific to this cohort, tasks and protocol and require further verification in independent cohorts and laboratories—we did however verify these results in a separate male cohort (see supplementary material). Another limitation concerns the need to consider additional complexity to evaluate ACL injury risk (Bittencourt et al., Citation2016), not least multi-joint moment patterns (Podraza et al., Citation2018). Altogether, future large-scale concerted efforts seem necessary to adequately handle injury risk evaluations based on complex biomechanical observations as part of prospective cohort studies.
Conclusion
The knee abduction moment did not identify all individuals with high non-sagittal knee joint moments. This result was consistent across multiple tasks. Non-sagittal moment metrics may therefore enhance the development of feasible multi-task screening protocols. These results support the consideration of transverse plane knee joint moments in addition to frontal plane moments when evaluating ACL injury risk.
Supplemental Material
Download PDF (55.9 KB)Disclosure statement
No potential conflict of interest was reported by the author(s).
Supplementary material
Supplemental data for this article can be accessed here.
References
- Bahr, R. (2016). Why screening tests to predict injury do not work—and probably never will … : A critical review. British Journal of Sports Medicine, 50(13), 776–780. https://doi.org/10.1136/bjsports-2016-096256
- Bates, N. A., Schilaty, N. D., Nagelli, C. V., Krych, A. J., & Hewett, T. E. (2019). Multiplanar loading of the knee and its influence on anterior cruciate ligament and medial collateral ligament strain during simulated landings and noncontact tears. The American Journal of Sports Medicine, 47(8), 1844–1853. https://doi.org/10.1177/0363546519850165
- Bisseling, R. W., & Hof, A. L. (2006). Handling of impact forces in inverse dynamics. Journal of Biomechanics, 39(13), 2438–2444. https://doi.org/10.1016/j.jbiomech.2005.07.021
- Bittencourt, N. F., Meeuwisse, W., Mendonça, L., Nettel-Aguirre, A., Ocarino, J., & Fonseca, S. (2016). Complex systems approach for sports injuries: Moving from risk factor identification to injury pattern recognition—narrative review and new concept. British Journal of Sports Medicine, 50(21), 1309–1314. https://doi.org/10.1136/bjsports-2015-095850
- Brown, S. R., Brughelli, M., & Hume, P. A. (2014). Knee mechanics during planned and unplanned sidestepping: A systematic review and meta-analysis. Sports Medicine, 44(11), 1573–1588. https://doi.org/10.1007/s40279-014-0225-3
- Chinnasee, C., Weir, G., Sasimontonkul, S., Alderson, J., & Donnelly, C. (2018). A biomechanical comparison of single-leg landing and unplanned sidestepping. International Journal of Sports Medicine, 39(8), 636–645. https://doi.org/10.1055/a-0592-7422
- Critchley, M. L., Davis, D. J., Keener, M. M., Layer, J. S., Wilson, M. A., Zhu, Q., & Dai, B. (2020). The effects of mid-flight whole-body and trunk rotation on landing mechanics: Implications for anterior cruciate ligament injuries. Sports Biomechanics, 19(4), 421–437. https://doi.org/10.1080/14763141.2019.1595704
- Cronström, A., Creaby, M. W., & Ageberg, E. (2020). Do knee abduction kinematics and kinetics predict future anterior cruciate ligament injury risk? A systematic review and meta-analysis of prospective studies. BMC Musculoskeletal Disorders, 21(1), 563. https://doi.org/10.1186/s12891-020-03552-3
- Dempsey, A. R., Lloyd, D. G., Elliott, B. C., Steele, J. R., Munro, B. J., & Russo, K. A. (2007). The effect of technique change on knee loads during sidestep cutting. Medicine and Science in Sports and Exercise, 39(10), 1765–1773. https://doi.org/10.1249/mss.0b013e31812f56d1
- Donelon, T. A., Dos’Santos, T., Pitchers, G., Brown, M., & Jones, P. A. (2020). Biomechanical determinants of knee joint loads associated with increased anterior cruciate ligament loading during cutting: A systematic review and technical framework. Sports Medicine-Open, 6(1), 53. https://doi.org/10.1186/s40798-020-00276-5
- Donohue, M. R., Ellis, S. M., Heinbaugh, E. M., Stephenson, M. L., Zhu, Q., & Dai, B. (2015). Differences and correlations in knee and hip mechanics during single-leg landing, single-leg squat, double-leg landing, and double-leg squat tasks. Research in Sports Medicine, 23(4), 394–411. https://doi.org/10.1080/15438627.2015.1076413
- Dos’Santos, T., Bishop, C., Thomas, C., Comfort, P., & Jones, P. A. (2019). The effect of limb dominance on change of direction biomechanics: A systematic review of its importance for injury risk. Physical Therapy in Sport, 37, 179–189. https://doi.org/10.1016/j.ptsp.2019.04.005
- Heebner, N. R., Rafferty, D. M., Wohleber, M. F., Simonson, A. J., Lovalekar, M., Reinert, A., & Sell, T. C. (2017). Landing kinematics and kinetics at the knee during different landing tasks. Journal of Athletic Training, 52(12), 1101–1108. https://doi.org/10.4085/1062-6050-52.11.25
- Hewett, T. E., Myer, G. D., Ford, K. R., Heidt, R. S., Jr, Colosimo, A. J., McLean, S. G., Van Den Bogert, A. J., Paterno, M. V., & Succop, P. (2005). Biomechanical measures of neuromuscular control and valgus loading of the knee predict anterior cruciate ligament injury risk in female athletes: A prospective study. The American Journal of Sports Medicine, 33(4), 492–501. https://doi.org/10.1177/0363546504269591
- Jones, P. A., Herrington, L. C., Munro, A. G., & Graham-Smith, P. (2014). Is there a relationship between landing, cutting, and pivoting tasks in terms of the characteristics of dynamic valgus? The American Journal of Sports Medicine, 42(9), 2095–2102. https://doi.org/10.1177/0363546514539446
- Kiapour, A. M., Demetropoulos, C. K., Kiapour, A., Quatman, C. E., Wordeman, S. C., Goel, V. K., & Hewett, T. E. (2016). Strain response of the anterior cruciate ligament to uniplanar and multiplanar loads during simulated landings: Implications for injury mechanism. The American Journal of Sports Medicine, 44(8), 2087–2096. https://doi.org/10.1177/0363546516640499
- Koga, H., Nakamae, A., Shima, Y., Iwasa, J., Myklebust, G., Engebretsen, L., Bahr, R., & Krosshaug, T. (2010). Mechanisms for noncontact anterior cruciate ligament injuries: Knee joint kinematics in 10 injury situations from female team handball and basketball. The American Journal of Sports Medicine, 38(11), 2218–2225. https://doi.org/10.1177/0363546510373570
- Kristianslund, E., & Krosshaug, T. (2013). Comparison of drop jumps and sport-specific sidestep cutting: Implications for anterior cruciate ligament injury risk screening. The American Journal of Sports Medicine, 41(3), 684–688. https://doi.org/10.1177/0363546512472043
- Krosshaug, T., Steffen, K., Kristianslund, E., Nilstad, A., Mok, K.-M., Myklebust, G., Andersen, T. E., Holme, I., Engebretsen, L., & Bahr, R. (2016). The vertical drop jump is a poor screening test for ACL injuries in female elite soccer and handball players: A prospective cohort study of 710 athletes. The American Journal of Sports Medicine, 44(4), 874–883. https://doi.org/10.1177/0363546515625048
- Leppänen, M., Pasanen, K., Kujala, U. M., Vasankari, T., Kannus, P., Ä Yrämö, S., Krosshaug, T., Bahr, R., Avela, J., Perttunen, J., & Parkkari, J., & others. (2017). Stiff landings are associated with increased ACL injury risk in young female basketball and floorball players. The American Journal of Sports Medicine, 45(2), 386–393. https://doi.org/10.1177/0363546516665810
- Malfait, B., Sankey, S., Firhad Raja Azidin, R. M., Deschamps, K., Vanrenterghem, J., Robinson, M. A., Staes, F., & Verschueren, S. (2014). How reliable are lower limb kinematics and kinetics during a drop vertical jump? Medicine and Science in Sports and Exercise, 46(4), 678–685. https://doi.org/10.1249/MSS.0000000000000170
- Manal, K., Gardinier, E., Buchanan, T. S., & Snyder-Mackler, L. (2015). A more informed evaluation of medial compartment loading: The combined use of the knee adduction and flexor moments. Osteoarthritis and Cartilage, 23(7), 1107–1111. https://doi.org/10.1016/j.joca.2015.02.779
- Markolf, K. L., Burchfield, D. M., Shapiro, M. M., Shepard, M. F., Finerman, G. A., & Slauterbeck, J. L. (1995). Combined knee loading states that generate high anterior cruciate ligament forces. Journal of Orthopaedic Research, 13(6), 930–935. https://doi.org/10.1002/jor.1100130618
- McLean, S. G., Huang, X., Su, A., & Van Den Bogert, A. J. (2004). Sagittal plane biomechanics cannot injure the ACL during sidestep cutting. Clinical Biomechanics, 19(8), 828–838. https://doi.org/10.1016/j.clinbiomech.2004.06.006
- Myer, G. D., Ford, K. R., Di Stasi, S. L., Foss, K. D. B., Micheli, L. J., & Hewett, T. E. (2015). High knee abduction moments are common risk factors for patellofemoral pain (PFP) and anterior cruciate ligament (ACL) injury in girls: Is pfp itself a predictor for subsequent ACL injury? British Journal of Sports Medicine, 49(2), 118–122. https://doi.org/10.1136/bjsports-2013-092536
- Nedergaard, N. J., Dalb, S., Petersen, S. V., Zebis, M. K., & Bencke, J. (2020). Biomechanical and neuromuscular comparison of single-and multi-planar jump tests and a side-cutting maneuver: Implications for ACL injury risk assessment. The Knee, 27(2), 324–333. https://doi.org/10.1016/j.knee.2019.10.022
- Pataky, T. C. (2012). One-dimensional statistical parametric mapping in python. Computer Methods in Biomechanics and Biomedical Engineering, 15(3), 295–301. https://doi.org/10.1080/10255842.2010.527837
- Pataky, T. C., Robinson, M. A., & Vanrenterghem, J. (2013). Vector field statistical analysis of kinematic and force trajectories. Journal of Biomechanics, 46(14), 2394–2401. https://doi.org/10.1016/j.jbiomech.2013.07.031
- Podraza, J. T., White, S. C., & Ramsey, D. K. (2018). Multi-plane, multi-joint lower extremity support moments during a rapid deceleration task: Implications for knee loading. Human Movement Science, 58, 155–164. https://doi.org/10.1016/j.humov.2018.02.001
- Quatman, C. E., Quatman-Yates, C. C., & Hewett, T. E. (2010). A ‘plane’ explanation of anterior cruciate ligament injury mechanisms. Sports Medicine, 40(9), 729–746. https://doi.org/10.2165/11534950-000000000-00000
- Ramsay, J. O., & Silverman, B. W. (2007). Applied functional data analysis: Methods and case studies. Springer.
- Robinson, M. A., Donnelly, C. J., Tsao, J., & Vanrenterghem, J. (2014). Impact of knee modeling approach on indicators and classification of anterior cruciate ligament injury risk. Medicine and Science in Sports and Exercise, 46(7), 1269–1276. https://doi.org/10.1249/MSS.0000000000000236
- Robinson, M. A., Donnely, C., Vanrenterghem, J., & Pataky, T. C. (2015). Sagittal plane kinematics predict non-sagittal joint moments in unplanned sidestepping. In The 25th congress of the international society of biomechanics, glasgow, uk. 12th–16th july.
- Sankey, S. P., Azidin, R. M. F. R., Robinson, M. A., Malfait, B., Deschamps, K., Verschueren, S., Staes, F., & Vanrenterghem, J. (2015). How reliable are knee kinematics and kinetics during side-cutting manoeuvres? Gait & Posture, 41(4), 905–911. https://doi.org/10.1016/j.gaitpost.2015.03.014
- Sharir, R., Vanrenterghem, J., Robinson, M., & George, K. (2017). What separates an individual at risk of ACL injury? A first step towards an ACL-risk movement passport. British Journal of Sports Medicine, 51(4), 388. https://doi.org/10.1136/bjsports-2016-097372.264
- Shin, C. S., Chaudhari, A. M., & Andriacchi, T. P. (2011). Valgus plus internal rotation moments increase anterior cruciate ligament strain more than either alone. Medicine and Science in Sports and Exercise, 43(8), 1484–1491. https://doi.org/10.1249/MSS.0b013e31820f8395
- Taylor, J. B., Ford, K. R., Nguyen, A.-D., & Shultz, S. J. (2016). Biomechanical comparison of single- and double-leg jump landings in the sagittal and frontal plane. Orthopaedic Journal of Sports Medicine, 4(6), 2325967116655158. https://doi.org/10.1177/2325967116655158
- Vanrenterghem, J., Gormley, D., Robinson, M., & Lees, A. (2010). Solutions for representing the whole-body centre of mass in side cutting manoeuvres based on data that is typically available for lower limb kinematics. Gait & Posture, 31(4), 517–521. https://doi.org/10.1016/j.gaitpost.2010.02.014
- Winter, D. A. (2009). Biomechanics and motor control of human movement. John Wiley & Sons.