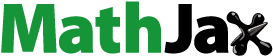
ABSTRACT
Background
This study aims to demonstrate the utility of a threshold logistic approach to identifying thresholds for specific antibiotic use associated with Clostridioides difficile infection (CDI) in an English teaching hospital.
Methods
A combined approach of nonlinear modeling and logistic regression, named threshold logistic, was used to identify thresholds and risk scores in hospital-level antibiotic use associated with hospital-onset, healthcare-associated (HOHA) CDI cases.
Results
Using a threshold logistic regression approach, an incidence greater than 0.2645 cases/1000 occupied bed-days (OBD; 85th percentile) was determined as the cutoff rate to define a critical (high) incidence rate of HOHA CDI. Fluoroquinolones and piperacillin-tazobactam were found to have thresholds at 84.8 and 54 defined daily doses (DDD)/1000 OBD, respectively. Analysis of data allowed calculating risk scores for HOHA CDI incidence rates exceeding the 85th percentile, i.e. entering critical incidence level. The threshold-logistic model also facilitated performing ‘what-if scenarios’ on future values of fluoroquinolones and piperacillin-tazobactam use to understand how HOHA CDI incidence rates may be affected.
Conclusion
Using threshold logistic analysis, critical incidence levels and antibiotic use targets to control HOHA CDI were determined. Threshold logistic models can be used to inform and enhance the effective design and implementation of antimicrobial stewardship programs.
1. Introduction
Clostridioides difficile infection (CDI) is a leading nosocomial infection, causing an increased burden on health-care systems and incurring significant direct and indirect costs [Citation1–3]. Clostridioides difficile (C. difficile) is an anaerobic spore-forming bacterium that colonizes the large intestine and is transmitted among humans through the fecal – oral route [Citation4]. C. difficile can cause conditions ranging from mild diarrhea to toxic megacolon, colonic perforation, and death and is associated with longer hospital stays and readmissions [Citation2–5]. This bacterium can also be carried asymptomatically in the gut, providing a potential reservoir for onward transmission in health-care facilities [Citation5]. Risks of CDI transmission in health-care setting and acquisition by patient are multifactorial. National UK guidelines on infection prevention and control and management of CDI inform hospital policies and high preventative standards. Important factors that increase the risk of CDI acquisition include antibiotic exposure, advanced age, underlying morbidity, and longer health-care admissions [Citation4–9]. Of importance, antibiotic exposure remains an important modifiable risk factor for the CDI [Citation4,Citation8]. Antibiotic use can cause changes in the natural balance of indigenous colonic microbiota, leading to C. difficile proliferation [Citation5]. A recent systematic review and meta-analysis updating the synthesis of evidence in relation to the risk of health-care facility-associated CDI showed that recent exposure to carbapenems, third- and fourth-generation cephalosporins, fluoroquinolones, clindamycin, and beta-lactamase inhibitor combination penicillin antibiotics was observed prior to developing CDI case [Citation8].
Antimicrobial stewardship (AMS) aims to improve antibiotic prescribing in health-care settings. Balancing access to effective antimicrobials with the prevention of the emergence of antibiotic resistance is challenging. Complete restrictions of certain antibiotics in clinical practice require significant efforts to implement and may select for other resistance mechanisms due to the need to compensate with other antibiotics [Citation10]. In a number of evaluations of the relationship between antibiotic use and resistance, analysis of data showed non-linear relationships and allowed determining a threshold of antibiotic use beyond which resistance would be triggered [Citation11–16]. Recently, Aldeyab and Lattyak introduced a threshold-logistic modeling concept to improve our understanding of the relationship between antibiotic use and the incidence of resistant pathogens [Citation17,Citation18]. The threshold-logistic approach is a combination of nonlinear modeling and logistic regression, which we used to identify thresholds and risk scores in hospital-level antibiotic use associated with incidence rates of extended-spectrum β-lactamase (ESBL) producing Escherichia coli (E. coli), and meticillin-resistant Staphylococcus aureus (MRSA) [Citation17,Citation18]. For ESBL-producing E. coli and MRSA, we demonstrated a way to facilitate near real-time feedback on the effectiveness of antimicrobial stewardship models, and we provided an opportunity to perform ‘what-if scenarios’ using different levels of antibiotic use to assess the expected impact on pathogen incidence rates in future months [Citation17,Citation18].
This study aims to demonstrate the utility of the recently developed threshold logistic approach in identifying thresholds for specific antibiotic use in association with CDI in one teaching hospital in England. We used data from the same population for which we previously examined the incidence of ESBL-producing E. coli and MRSA [Citation17,Citation18]. This is to show a case for mapping high-risk antibiotics against important pathogens in one hospital, therefore, providing relevant antibiotic use targets and tailored recommendations to inform AMS.
2. Methods
2.1. Study design and population
The study took place at Pinderfields General Hospital (PGH) (700 beds), Mid Yorkshire Teaching NHS Trust in West Yorkshire, England. The Trust, with three hospital sites including PGH, cares for nearly 500,000 people, providing medical and surgical services, intensive care, hematology/oncology, regional burns and spinal injuries units, and community services. The study included only adult inpatients admitted to PGH. Data were retrospectively collected for the study period from January 2015 to December 2021. The analysis methods for this study required a minimum of 5 years of monthly antibiotic use and microbiology data [Citation17,Citation18]. Considering the availability of the longest period of consistent antibiotic use and CDI data, the study analyzed 7 years of monthly data. We hypothesized that using carbapenems, second- and third-generation cephalosporins, fluoroquinolones, co-amoxiclav, piperacillin-tazobactam, macrolides, and clindamycin could explain variations in CDI incidence rates. These antibiotics were identified a priori based on published evidence of their role as risk factors for driving the incidence of CDI in health-care settings [Citation8,Citation9].
2.2. Microbiology and pharmacy data
A CDI case was defined as any adult inpatient (≥18 years), who was admitted to PGH between 1 January 2015 and 31 December 2021, and who had three or more episodes of loose stools (diarrhea) and fecal samples, conforming to Bristol Stool Chart 5–7 (essentially taking the shape of the container) with positive glutamate dehydrogenase (GDH) and toxin test for C. difficile during their admission. As per the hospital policy, stool samples must only be submitted for testing once an assessment has been undertaken to determine if any other cause (such as laxatives, enemas, or other preparations to stimulate the bowel, enteral feeding, inflammatory colitis, overflow, bowel disorders, bowel surgery, and medication) for diarrhea (Bristol stool chart type 5–7) is excluded. The hospital laboratory standard operating procedure mandates testing only samples that confirm to Bristol Stool Chart 5–7; laboratory reports both non-C. difficile infectious causes of diarrhea and C. difficile PCR and toxin. Only hospital-onset, healthcare-associated (HOHA) CDI cases were included for this study. HOHA CDI was defined as any NHS patient with diarrhea and fecal specimens taken on the third day of admission onwards (i.e. ≥day 3 when the day of admission is day 1). Duplicated positive cases were excluded, and an isolate from the same patient identified within 30 days of a previous isolate with the same identification was considered as the same case. An infection control software (ICNET; Clinical Surveillance Software NG 1.7.1.0, Baxter International Inc., UK) was used to obtain this data.
The hospital pharmacy information systems were used to obtain monthly antibiotic use data. The classification of antimicrobials for systemic use (J01) in the WHO/ATC index was used, and antibiotic data were then converted into Defined Daily Doses (DDD) and were expressed as DDD per 1000 occupied bed-days (OBD) [Citation19].
2.3. Modelling and statistical analysis
Threshold-Logistic analysis was applied in modeling the relationship between antibiotic use and an elevated level of HOHA CDI [Citation17,Citation18]. Antibiotics suspected to increase the incidence rate of the studied pathogen were initially considered. HOHA CDI incidence rate was used as our response variable. Upon completing this initial analysis phase, we identified candidate antibiotic series with lagged positive cross-correlation to HOHA CDI incidence rates that were statistically significant at the 10% level or better (p ≤ 10). The latter significance level was chosen to perform an initial variable selection of candidate explanatory variables (univariate methods). Lags of 1–5 months were considered when examining the linear association of antibiotic usage with increased pathogen incidence rate. The maximum cross-correlation value was used to choose the optimal lag for the antibiotic series to be considered as candidate explanatory variables in this screening stage of drivers. In the next phase, we applied a threshold search algorithm to identify break points in levels of the candidate antibiotic series that resulted in an increased response over the overall linear relationship. By doing so, we expanded our individual candidate antibiotic series with a threshold dimension.
In the final analysis phase, we applied a multi-dimensional threshold-logistic search algorithm that evaluated the combination of all candidate antibiotic series, with respect to their potential lag and threshold adaptations; potential multicollinearity was tested. Since we applied a logistic approach, the HOHA CDI incidence rate was transformed into a binary event. This was accomplished within the multi-dimensional threshold-logistic search algorithm that partitioned the HOHA CDI incidence rate by various percentiles, specifically between the 50th and 85th percentiles, in search of the greatest separation of the binary class (1 = exceeding cutoff; 0 = below cutoff) and best classification accuracy. The 50th–85th percentiles were selected as a plausible target since this target would be defined in the upper 50% of the historical data and would include enough observations so as not to be considered a rare event. Akaike information criterion (AIC) and area under the curve (AUC) from the receiver operator characteristic (ROC) curve were used to select the 85th percentile (0.2645) of HOHA CDI as the critical level, defining the binary event as
Event = Ifelse (CDI incidence rate ≥ 0.2645, 1, 0).
It was at this cutoff for the binary event that dominated classification performance for both accuracy and number of modeled results in the top decile of accuracy measures.
The possible impact of COVID-19 pandemic was also considered in the model by introducing a binary dummy variable consisting of 0’s prior to April 2020 and 1’s thereafter. The estimation of the threshold-logistic model has shown the impact of the COVID-19 pandemic period to be statistically insignificant at the 5% level.
After identifying and estimating the final threshold-logistic model, predicted probabilities (risk scores) of the threshold logistic regression model were generated. A coded alert signal (High, Medium, or Low risk) was created based on the MinMax transformation of the predicted probabilities (risk score) coming from the threshold logistic model.
This MinMax transformation is helpful to produce a heuristic alert signal (High-Med-Low) for policy purposes that categorize risk scores from unbalanced classification data. To maximize the overall distribution accuracy of a low signal, classifying an infection rate as being below the 85th percentile, and a high signal classifying an infection rate as being greater than the 85th percentile, we computed the cutoff ranks using a linear programming (LP) technique. To define Low, Medium, and High coded alert signals, the cutoff ranks optimized through LP were 0.0 to ≤0.30, >0.30 to <0.84, and ≥0.84 to 1.0, respectively. For the purpose of estimating the reduction in studied pathogens resulting from antibiotic use maintained below threshold values, the weighted difference between the mean incidence rates relative to antibiotic use threshold criteria (subgroups) was used. A 95% confidence range on the expected reduction of pathogen incidence rate was reported by computing the standard error of the mean (SEM) for each subgroup along with the standard error between paired subgroup means.
The SCA Statistical System version 8.2 (Scientific Computing Associates Corp., River Forest, Illinois, USA) and R software version 4.3.0 (R Foundation for Statistical Computing, Vienna, Austria) were used to perform analysis.
3. Results
Over the study period, 260 non-duplicated HOHA CDI cases were identified. The average monthly HOHA CDI incidence rate was 0.154 cases/1000 OBD (range: 0.00–0.500). Average fluoroquinolone use was 67.2 DDD/1000 OBD (range: 37.4–123.0), and average piperacillin-tazobactam use was 57 DDD/1000 OBD (range: 14.07–86.16). A plot of the relationship between the use of fluoroquinolones and piperacillin-tazobactam and the incidence of HOHA CDI cases is shown in .
Figure 1. Monthly hospital-onset, healthcare-associated (HOHA) incidence (thick line, no. Of cases/1000 OBD) versus use of fluoroquinolones (thin line, DDD/1000 OBD), January 2015 to December 2021.
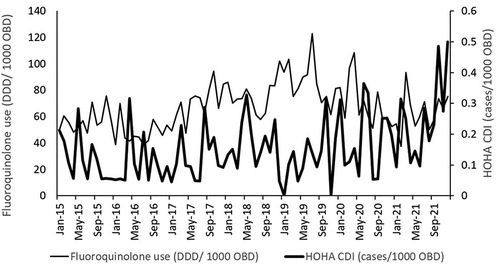
Figure 2. Monthly hospital-onset, healthcare-associated (HOHA) incidence (thick line, no. Of cases/1000 OBD) versus use of piperacillin-tazobactam (thin line, DDD/1000 OBD), January 2015 to December 2021.
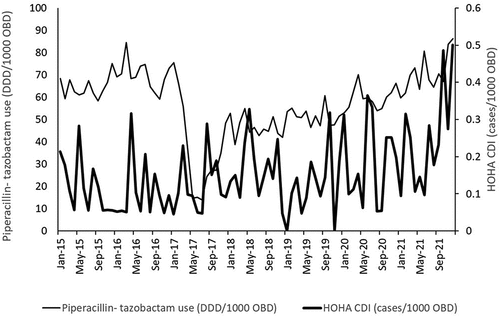
3.1. Defining critical (high) HOHA CDI incidence as the binary event
Applying threshold logistic regression in a search algorithm, the 85th percentile (0.2645 cases/1000 OBD) of HOHA CDI incidence was selected as the cutoff percentile to define the critical (high) incidence rate ().
Figure 3. The empirical cumulative distribution function for hospital-onset, healthcare-associated (HOHA) CDI historical data. The solid vertical line represents the 85th percentile (0.2645 cases/1000 OBD).
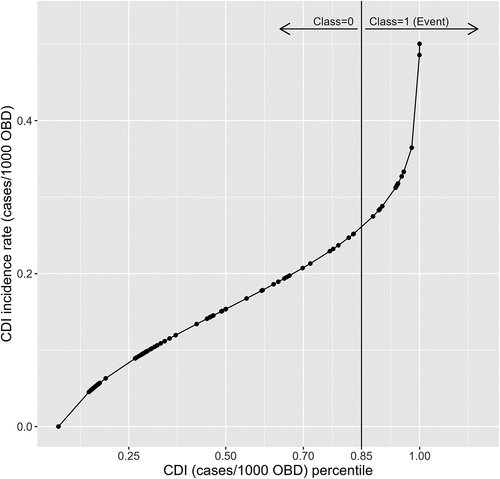
The impact of the COVID-19 pandemic on HOHA CDI was found to be insignificant (coefficient = 1.2209; p = 0.0787), and it was removed from the models to maintain parsimony.
3.2. Identified risk antibiotics and targeted consumption rates
Threshold logistic analysis showed that fluoroquinolones and piperacillin-tazobactam exhibited thresholds at 84.8 and 54 DDD/1000 ODB, respectively (). The model showed that for every one-unit increase in fluoroquinolone and piperacillin-tazobactam use above 84.8 and 54 DDD/1000 OBD, the average odds of HOHA CDI incidence rate exceeding the 85th percentile of historical levels increased by 13.1% and 10.3%, respectively (). The AUC measure for the ROC curve was 71% (). shows the cumulative CDI incidence rates relative to fluoroquinolones and piperacillin-tazobactam being above or below their respective thresholds.
Figure 4. Receiver operator characteristic (ROC) chart showing the true positive classification rate against the false positive classification rate at different probability cutoff thresholds. The area under the curve (AUC) is an aggregate measure of performance across all possible classification thresholds.
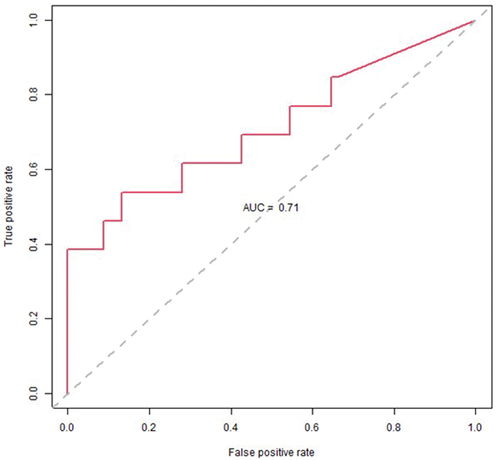
Figure 5. The cumulative CDI incidence rates relative to fluoroquinolone and piperacillin-tazobactam use being above or below their respective thresholds.
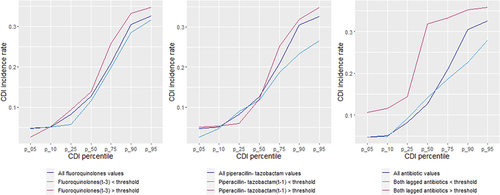
Table 1. Threshold logistic results in modeling hospital-onset, healthcare-associated (HOHA) CDI incidence rate at the 85th percentile, January 2015 to December 2021.
3.3. Near real-time performance monitoring and feedback
The risk scores for 2020 and 2021, which were determined using the threshold logistic analysis for HOHA CDI incidence rates exceeding the 85th percentile (0.2645 cases/1000 OBD), are shown in . Probability risk scores were coded using three alert signal levels (Low, Medium, and High; ).
Table 2. Risk scores for CDI incidence rate exceeding the 85th percentile for 2020 and 2021.
3.4. HOHA CDI incidence projections
The threshold-logistic model included lagged relationships between antibiotic use and CDI incidence rate. This facilitated an opportunity to use the model to perform ‘what-if scenarios’ on future values of fluoroquinolone and piperacillin-tazobactam use to understand how the expected CDI incidence rate may change. In our example, the last historical observation for CDI incidence rate was December 2021. The risk score and coded alert signal were already predicted by the model for January 2022 which was based on fluoroquinolone use in October 2021 (lag 3) and piperacillin-tazobactam use in December 2021 (lag 1). It was a high alert signal, and although we were unable to affect the risk score, there would be a month based on the prediction to prepare mitigation responses to an increased level of infection. The model also had the information on fluoroquinolone use to predict risk scores for February and March 2022, but it does not yet have information on piperacillin-tazobactam use. This allowed the opportunity to permutate values for piperacillin-tazobactam use and evaluate its scenario effect on risk. Since piperacillin-tazobactam use has a one-month lag in the model, antibiotic use for January 2022 (not yet realized) and its effect on CDI incidence rate in February 2022 and subsequent months could be explored.
In , we reviewed the result of a ‘what-if scenarios’ for February 2022, with piperacillin-tazobactam use set to 55 (DDD/1000) for January 2022 (Lag 1), and fluoroquinolone use in November 2021 (Lag 3, 66.4040) already known. This resulted in a Medium alert signal with a 6% probability that HOHA CDI incidence rate may exceed the 85th percentile. Risk scores are shown for March and April 2022, controlling for levels of antibiotic use. If piperacillin-tazobactam use was maintained below the recommended threshold of 53.99 DDD/1000 OBD in February 2022 and given that fluoroquinolone use in December 2021 was below the recommended threshold of 84.82 DDD/1000 OBD, we could reduce the probability of a critical increase in HOHA CDI rate for March 2022. In contrast, a high coded alert signal was produced if we allowed fluoroquinolone use in January 2022 (Lag 3) and piperacillin-tazobactam use in March 2022 (Lag 1) to exceed thresholds.
Table 3. What-if threshold logistic model exploration.
3.5. Case reduction estimates for HOHA CDI
We segmented HOHA CDI incidence rates using the antibiotic thresholds from the model and compared the difference in monthly means. shows the incremental reduction in incidence rate when antibiotic use was maintained below the identified thresholds. The number of months in which historic antibiotic use was above or below threshold conditions is provided in the months column and the associated HOHA CDI average for the threshold condition is provided in the HOHA CDI (mean) column. The difference in HOHA CDI averages being above or below the threshold condition is also displayed. In order to compute the weighted difference in HOHA CDI average and the expected reduction in HOHA CDI cases for 2021, the number of months was converted into a proportion. The proportion of months in which fluoroquinolone use was less than or equal to the threshold was 85.2% compared to fluoroquinolone use greater than the threshold, which was 14.8%. These proportions were then normalized (41.1% + 18.6% + 40.2% = 100%). The weighted reduction in HOHA CDI mean (0.0485) was then computed using the sum product of the difference in means and the normalized proportion of months in which the threshold condition was observed. Finally, the expected reduction in HOHA CDI cases for 2021 was computed by multiplying the 63 reported HOHA CDI cases in 2021 with the 0.0485 weighted reduction in HOHA CDI rate.
Table 4. HOHA CDI incidence rate distribution when antibiotic use is below/above thresholds.
It was estimated that the number of cases could have been reduced by 3.06 with 95% CI (2.04 to 4.07) if antibiotics were maintained below identified thresholds. This represents a potential 4.85% (standard error (σ) = 0.82%) reduction in HOHA CDI cases.
3.6. Case reduction estimates for ESBL-producing E. coli and MRSA [Citation17,Citation18]
For the purpose of estimating the reduction in the incidence of studied pathogens in this hospital, Table S1 and Table S2 show the incremental reduction in the incidence of the previously examined ESBL-producing E. coli and MRSA incidence rates when antibiotic use was maintained below the identified thresholds [Citation17,Citation18]. It was estimated that the number of ESBL-producing E. coli and MRSA cases could have been reduced by 2.93 with 95% CI (2.08–3.77) and 1.70 with 95% CI (0.47–2.94), respectively, if the antibiotics were maintained below identified thresholds. This represents a potential 4.12% (σ = 0.60%) and 3.04% (σ = 1.12%) reduction in ESBL-producing E. coli and MRSA cases, respectively.
3.7. Overall case reduction estimates
The overall reduction in the incidence of pathogens, including HOHA CDI, ESBL-producing E. coli, and MRSA, is shown to be a combined 12.02% (σ = 2.54%) () if the antibiotic use were maintained below identified thresholds.
Table 5. Estimated reduction in the number of cases of studied pathogens.
4. Discussion
In this study, we aimed to identify thresholds for specific antibiotic use in association with HOHA CDI in hospitalized patients and to determine the probability of a hospital reaching a critical level of HOHA CDI in advance of such an event occurring. The findings showed that fluoroquinolones and piperacillin-tazobactam were associated with the incidence of HOHA CDI. The other assessed antibiotics were eliminated in the variable selection process because they could not be shown to have a statistically significant impact on CDI incidence rate. Using a threshold logistic regression approach, an incidence greater than 0.2645 cases/1000 OBD (85th percentile) was determined as the cutoff rate to define the critical (high) incidence rate of HOHA CDI. Threshold logistic analysis showed that fluoroquinolones and piperacillin-tazobactam were found to have thresholds at 84.8, and 54 DDD/1000 OBD, respectively, significantly increasing the probability of high pathogen incidence rate. Analysis of data allowed calculating risk scores for HOHA CDI incidence rates exceeding the 85th percentile, i.e. entering high incidence level. The threshold-logistic model also facilitates performing ‘what-if scenarios’ on future values of fluoroquinolones use and piperacillin-tazobactam use to understand how HOHA CDI incidence rate may be affected.
CDI is commonly associated with the use of certain antibiotics. The antibiotic use disrupts the indigenous microflora of the colon, allowing C. difficile to overgrow and produce toxins [Citation5]. The findings of this study were consistent with other published research that documented the association between fluoroquinolones, piperacillin-tazobactam, and the incidence of CDI [Citation8,Citation9]. Both fluoroquinolones and piperacillin-tazobactam are classified as Watch antibiotics (crucial for human medicine and at higher risk of resistance) according to the WHO Access, Watch, Research (AWaRe) classification [Citation20]. These antibiotics should be subjected to close monitoring by the hospital AMS management team and their use should be reduced where appropriate (https://adoptaware.org/). However, it is important to consider the balance between how far we can reduce the use of these antibiotics to control resistance while we still provide access to them and avoid over-restriction undesirable effect [Citation10].
In this study, a two-stage approach was used to determine relevant quantitative targets to limit identified antibiotic use and control HOHA CDI incidence rate. Firstly, a threshold value is an inflection point that is the start of an accelerated change in the pathogen’s incidence rate that is in response to an increase in antibiotic usage, relative to lower values of antibiotic usage. This inflection point does not indicate an immediate jump in the pathogen incidence rate that is outside normal variation. Therefore, the critical level of a pathogen incidence rate should be determined from the outset. A pre-defined cutoff value that defines a critical level of a pathogen incidence rate for hospitals, can be based on (i) a tolerance level set by government recommendation, that is the number of cases per 1000 OBD determined to be a high level of pathogen incidence outside the normal range of the national hospital system, or (ii) a hospital guideline, that is the hospital’s goal to keep the pathogen incidence rate below a certain number of cases per 1000 OBD, or (iii) an empirical analysis of historical pathogen incidence rate for a specific hospital. In this study, we used empirical analysis of historical HOHA CDI incidence rates to define when a critical level of HOHA CDI incidence rate was reached. Using a threshold logistic regression search algorithm, an incidence greater than 0.2645 cases/1000 OBD was selected as the cutoff value to define the critical incidence rate of HOHA CDI.
Second, using threshold logistic models, thresholds for fluoroquinolones and piperacillin-tazobactam that would accelerate HOHA CDI incidence rates and increase the probability of reaching critical levels were identified. Fluoroquinolones and piperacillin-tazobactam were found to have a threshold at 84.8, and 54 DDD/1000 OBD, respectively. The identification of the cutoff value for critical HOHA CDI incidence and the thresholds of the latter antibiotics provide targets for the AMS team and the hospital policymakers. Adhering to the provided thresholds will allow for a decrease in average HOHA CDI incidence rates over time as future months of HOHA CDI incidence are going to be below the identified critical incidence rate. This will eventually lead to controlling the incidence rate of HOHA CDI, allowing for the fact that reducing high risk antibiotic use is one of several components to reducing overall CDI rates. The threshold logistic models, used in this study, also allowed calculating risk scores or probabilities of HOHA CDI incidence rates exceeding a critical level. This has the utility of providing near real-time feedback to prescribers on their performance of keeping antibiotic use below identified thresholds. We showed this utility via a sample scorecard with risk scores being coded into alert signals of low, medium and high risk. Providing regular feedback to prescribers is essential to maintain the effectiveness of implemented antibiotic policies. Importantly, threshold logistic models facilitate predictive analysis, resulting in ‘what-if scenarios’ based on varied antibiotic usage scenarios to assess the expected impact on the studied pathogen. In the present work, the AMS and infection prevention and control (IPC) management teams will have the predicted probability of approaching the critical HOHA CDI incidence, based on their antibiotic use, months in advance (depending on the identified fluoroquinolone and piperacillin-tazobactam lags). This will help the AMS and IPC teams in their risk assessment and forward plans to determine the best countermeasures to mitigate the risk of an increase in the incidence rate of HOHA CDI.
It is also important to note that the threshold logistic model can be used to strictly identify the association of the explanatory variables to the binary response, in this case, pathogen incidence rate in the upper 85th percentile. There is less utility for the model to identify associations of the explanatory variables at lower percentiles of pathogen incidence rate. Furthermore, the model demonstrates the statistical significance of threshold levels of fluoroquinolone and piperacillin-tazobactam use on increased pathogen incidence rate. The consistency of the effect of antibiotic threshold levels on increased incidence rate (>50th percentile) is also demonstrated in the difference between incidence rates when partitioned with respect to the condition of antibiotic use above or below identified thresholds (). Undoubtedly other important explanatory variables may exist that increase the overall predictive power of the model above the adequate predictive power of the current model (AUC > .70),
Due to the evolutionary nature of resistant pathogens to adapt to changing environmental conditions, it is challenging to model and forecast AMR. In a recent article by Pei et al. (2023) [Citation21], the authors discussed such challenges and highlighted the absence of real-time forecasting models for antimicrobial-resistant organisms and emphasized the need for improved predictive intelligence to better estimate the emergence and spread of population AMR in health-care settings. Addressing the time-varying effects of explanatory variables on the response function and considering changes in the structural form of the relationship between the explanatory variables and the outcome variable is essential to generate results that can inform health-care policies [Citation22]. The use of threshold transfer function modeling, which includes Autoregressive Moving-Average (ARMA) components, aims to help address such issues by identifying locally linear relationships in the data. In this case, the threshold indicates where there is a change in response (e.g. AMR correlates to a consistently higher incidence rate or higher probability of entering a period of critical-level infection rate when the predictor variable exceeds a given threshold). It is within the locally linear conditions of the model that are associated with higher incidence rates and lower predictive error rates. Whereas the above characteristics of nonlinear threshold models can help, model recalibration also comes into play. First, because the models are parametric, the model parameters can be re-estimated after a new observation is recorded. In this sense, only the parameters are updated to reflect the new information, and no change in the structural form of the model would occur. However, the structural form of the model may need to be modified when the statistical significance of a model parameter falls below a defined confidence level. Second, the usefulness of the predictive model can be monitored by evaluating predictive error to a base. For a threshold-logistic model, the AUC can be used to assess the predictive power of the model and its ability to differentiate classes (binary events). In practice, model estimation, assessment, and the forecasting accuracy can be automated. The frequency of model recalibration is dependent upon the stability of the identified relationships in the model and the degree of observed AMR evolutionary changes [Citation22].
In this study, threshold logistic analysis showed that fluoroquinolones and piperacillin-tazobactam were found to have a threshold in association with HOHA CDI at 84.8 and 54 DDD/1000 ODB, respectively. In a previous assessment of two other pathogen incidence rates [Citation17,Citation18], threshold logistic models identified thresholds for (i) fluoroquinolones (61.1 DDD/1000 OBD) and third-generation cephalosporins (9.2 DDD/1000 OBD) to control hospital ESBL-producing E. coli incidence, and (ii) fluoroquinolones (55.96 DDD/1000 OBD) and co-amoxiclav (312.19 DDD/1000 OBD) to control hospital MRSA incidence. Importantly, the assessment of the three pathogens, i.e. HOHA CDI, ESBL-producing E. coli, and MRSA, took place in the same study site hospital using the same study period and population. The findings of this study helped with mapping antibiotics that are known to promote resistance against certain alert pathogens in hospitalized patients. To control HOHA CDI, ESBL-producing E. coli, and MRSA, the AMS management team at Pinderfields General Hospital needs to monitor the following antibiotics and to adhere to the identified thresholds, that is, fluoroquinolones (<56 DDD/1000 OBD), third-generation cephalosporins (<9 DDD/1000 OBD), co-amoxiclav (<312 DDD/1000 OBD), and piperacillin-tazobactam (<54 DDD/1000 ODB). Regarding fluoroquinolones, it was associated with the three pathogens, and the lowest identified threshold was considered to set targets for informing antibiotic policies.
Estimation of number of HOHA CDI, ESBL-producing E. coli, and MRSA cases reduction, if the antibiotic use kept below identified thresholds, showed an annual decrease of 4.85% (3 cases), 4.12% (3 cases), and 3.04% (2 cases), respectively. This provided a total annual reduction of 12.02% (σ = 2.54%) in these gram-positive and gram-negative pathogens if antibiotics kept below identified thresholds. These pathogens are associated with significant costs to health-care systems. In a meta-analysis of 42 studies to estimate the financial impact of CDI in the US, the average cost for CDI case management per case was found to be $42,316 with an attributable cost per case of $21,448 [Citation23]. Hospital-onset CDI-attributable cost per case was $34,157 [Citation23]. A cost modeling of HCAI 2016/17, estimated the total annual cost of £2.7 billion, with 7.1 million bed days needed for NHS England [Citation24]. It was reported that CDI accounts for 5.6% of all infections within NHS England [Citation25]. A retrospective cohort study of 134 E. coli bacteremias in an Italian hospital estimated the mean cost per case for ESBL bacteremia to be €13,709 and non-ESBL to be €8,683 [Citation26]. A larger cohort study in China of 580 patients found that costs were for ESBL bacteremia to be $8,048.68, compared to non-ESBL of $7,476.84 [Citation27]. Costs of MRSA may vary in different places [Citation28]. A study in the US examining the whole hospital excess costs of MRSA indicated adjusted costs of $51,252 to $84,436 for MRSA [Citation29].
The study has the strength of using robust analysis methods. The data that were used for this study were routinely collected for all adult inpatients, therefore selection and information bias are unlikely. The study has some limitations. The hospital has a policy on the prevention and management of CDI which is aligned to national guidance and addresses various aspects of infection prevention and control (IPC) guidance surrounding CDI. The hospital has established AMS activities and reports on national measures such as Commissioning for Quality and Innovation (CQUIN) scheme. Overall, the hospital policy on the IPC of CDI, and the hospital AMS measures were consistently applied throughout the study period. Nevertheless, the analysis can be improved by including other explanatory factors, for example proxy measures for patient case mix, infection prevention and control activities, and factors adjusting for community antibiotic and resistance potential impact, if possible [Citation30–32]. In addition, this work was done in one hospital and warrants being evaluated in a multi-center study.
5. Conclusion
Through the application of the threshold logistic concept, we provided an approach that can be utilized to inform and enhance the effective design and implementation of antimicrobial stewardship programs. A Threshold Logistic modeling approach was used to (i) define target critical pathogen incidence rates, (ii) derive threshold values of consequence that antibiotic usage should not exceed, (iii) provide probability scores to inform the ‘risk’ of entering a month of high pathogen incidence rate, (iv) address ease of monitoring policy using near real-time feedback and heuristic risk score levels (Low-Med-High) as alert signals, and (v) provide an opportunity to perform ‘what-if scenarios’ using different levels of antibiotic use to assess the expected impact on the predicted incidence rate of pathogens for future months; a high-risk alert signal should manifest into increased ‘preparedness’ to control the pathogen incidence rate. The threshold logistic model is a classification process in which classification accuracy can be monitored and components of the model (thresholds, coefficients, etc.) can be assessed over time for changes in pathogen response via the technical management team. Although our proposed models were created using real hospital datasets, the findings of these predictive models have not yet been implemented and tested in hospitals so that their operational utility can be assessed in daily clinical practice, an area that warrants further research.
Article highlights
Balancing access to effective antimicrobials with the prevention of the emergence of antibiotic resistance is challenging.
The non-linear relationship between antibiotic use and resistance creates a threshold of antibiotic use beyond which resistance would be triggered.
Threshold logistic analysis provided the critical pathogen incidence level and antibiotic use targets to control resistance.
Threshold logistic analysis provided near-real time performance feedback and future projections to control resistance.
Threshold logistic models can be used to inform and enhance the effective design and implementation of antimicrobial stewardship programs.
Declaration of interest
The authors have no relevant affiliations or financial involvement with any organization or entity with a financial interest in or financial conflict with the subject matter or materials discussed in the manuscript. This includes employment, consultancies, honoraria, stock ownership or options, expert testimony, grants or patents received or pending, or royalties.
Reviewer disclosures
Peer reviewers on this manuscript have no relevant financial or other relationships to disclose.
Author contributions
Conceptualization and design, M Aldeyab and W Lattyak; methodology, all authors; software, W Lattyak; data acquisition and investigation: all authors; formal analysis, M Aldeyab and W Lattyak; W Lattyak was the principal analyst; writing – original draft preparation, M Aldeyab; writing – review and editing, all authors. All authors have read and agreed to the published version of the manuscript.
Supplemental Material
Download MS Word (22.1 KB)Supplemental data
Supplemental data for this article can be accessed online at https://doi.org/10.1080/14787210.2023.2263642.
Correction Statement
This article has been republished with minor changes. These changes do not impact the academic content of the article.
Additional information
Funding
References
- Balsells E, Shi T, Leese C, et al. Global burden of Clostridium difficile infections: a systematic review and meta-analysis. J Glob Health. 2019;9(1):010407. doi: 10.7189/jogh.09.010407
- Robertson C, Pan J, Kavanagh K, et al. Cost burden of Clostridioides difficile infection to the health service: a retrospective cohort study in Scotland. J Hosp Infect. 2020;106(3):554–561. doi: 10.1016/j.jhin.2020.07.019
- Wiegand PN, Nathwani D, Wilcox MH, et al. Clinical and economic burden of Clostridium difficile infection in Europe: a systematic review of healthcare-facility-acquired infection. J Hosp Infect. 2012;81:1–14. doi: 10.1016/j.jhin.2012.02.004
- Leffler DA, Lamont JT, Longo DL. Clostridium difficile infection. N Engl J Med. 2015;372:1539–1548. doi: 10.1056/NEJMra1403772
- Martin JS, Monaghan TM, Wilcox MH. Clostridium difficile infection: epidemiology, diagnosis and understanding transmission. Nat Rev Gastroenterol Hepatol. 2016;13:206–216. doi: 10.1038/nrgastro.2016.25
- McDonald LC, Gerding DN, Johnson S, et al. Clinical practice guidelines for Clostridium difficile infection in adults and children: 2017 update by the Infectious Diseases Society of America (IDSA) and Society for Healthcare Epidemiology of America (SHEA). Clin Infect Dis. 2018;66(7):e1–e48. doi: 10.1093/cid/cix1085
- Eze P, Balsells E, Kyaw MH, et al. Risk factors for Clostridium difficile infections - an overview of the evidence base and challenges in data synthesis. J Glob Health. 2017;7(1):010417. doi: 10.7189/jogh.07.010417
- Slimings C, Riley TV. Antibiotics and healthcare facility-associated Clostridioides difficile infection: systematic review and meta-analysis 2020 update. J Antimicrob Chemother. 2021;76:1676–1688. doi: 10.1093/jac/dkab091
- Slimings C, Riley TV. Antibiotics and hospital-acquired Clostridium difficile infection: update of systematic review and meta-analysis. J Antimicrob Chemother. 2014;69:881–891. doi: 10.1093/jac/dkt477
- Conlon-Bingham GM, Aldeyab M, Scott M, et al. Effects of antibiotic cycling policy on incidence of healthcare-associated MRSA and Clostridioides difficile infection in secondary healthcare settings. Emerg Infect Dis. 2019;25:52–62. doi: 10.3201/eid2501.180111
- Levy SB. Balancing the drug-resistance equation. Trends Microbiol. 1994;2(10):341–342. doi: 10.1016/0966-842X(94)90607-6
- López-Lozano JM, Lawes T, Nebot C, et al. A nonlinear time-series analysis approach to identify thresholds in associations between population antibiotic use and rates of resistance. Nat Microbiol. 2019;4(7):1160–1172. doi: 10.1038/s41564-019-0410-0
- Lawes T, Lopez-Lozano JM, Nebot CA, et al. Effect of a national 4C antibiotic stewardship intervention on the clinical and molecular epidemiology of Clostridium difficile infections in a region of Scotland: a non-linear time-series analysis. Lancet Infect Dis. 2017;17(2):194–206. doi: 10.1016/S1473-3099(16)30397-8
- Al-Hashimy ZS, Conway BR, Al-Yaqoobi M, et al. Identifying targets for antibiotic use for the management of carbapenem-resistant Acinetobacterbaumannii (CRAb) in hospitals-A multi-centre nonlinear time-Series study. Antibiotics. 2022;11(6):775. doi: 10.3390/antibiotics11060775
- Chen S, Li Z, Shi J, et al. A nonlinear time-series analysis to identify the thresholds in relationships between antimicrobial consumption and resistance in a Chinese tertiary hospital. Infect Dis Ther. 2022;11(3):1019–1032. doi: 10.1007/s40121-022-00608-w
- Hayajneh WA, Al-Azzam S, Yusef D, et al. Identification of thresholds in relationships between specific antibiotic use and carbapenem-resistant Acinetobacterbaumannii (CRAb) incidence rates in hospitalized patients in Jordan. J Antimicrob Chemother. 2021;76(2):524–530. doi: 10.1093/jac/dkaa463
- Aldeyab MA, Bond SE, Conway BR, et al. Identifying antibiotic use targets for the management of antibiotic resistance using an extended-spectrum β-lactamase-producing Escherichia coli case: a threshold logistic modeling approach. Antibiotics. 2022;11(8):1116. doi: 10.3390/antibiotics11081116
- Aldeyab MA, Bond SE, Conway BR, et al. A threshold logistic Modelling approach for identifying thresholds between antibiotic use and methicillin-resistant Staphylococcus aureus incidence rates in hospitals. Antibiotics. 2022;11(9):1250. doi: 10.3390/antibiotics11091250
- WHO Collaborating Centre for Drug Statistics Methodology. Guidelines for ATC classification and DDD assignment 2023. Oslo: Norway; 2022. https://www.whocc.no/atc_ddd_index_and_guidelines/guidelines/
- WHO Access. Watch, reserve (AWaRe) classification of antibiotics for evaluation and monitoring of use, 2021. Geneva: World Health Organization; 2021. (WHO/MHP/HPS/EML/2021.04).
- Pei S, Blumberg S, Vega JC, et al. Challenges in forecasting antimicrobial resistance. Emerg Infect Dis. 2023;29(4):679–685. doi: 10.3201/eid2904.221552
- Aldeyab MA, Lattyak WJ. Challenges in forecasting antimicrobial resistance. Emerg Infect Dis. 2023;29(7):1496. doi: 10.3201/eid2907.230489
- Zhang S, Palazuelos-Munoz S, Balsells EM, et al. Cost of hospital management of Clostridium difficile infection in United States – a meta-analysis and modelling study. BMC Infect Dis. 2016;16:447. doi: 10.1186/s12879-016-1786-6
- Guest JF, Keating T, Gould D, et al. Modelling the annual NHS costs and outcomes attributable to healthcare-associated infections in England. BMJ Open. 2020;10(1):e033367. doi: 10.1136/bmjopen-2019-033367
- Health Protection Agency. English national point prevalence survey on healthcare-associated infections and antimicrobial use, preliminary data, 2011. Available: https://webarchive.nationalarchives.gov.uk/ukgwa/20140714085429/http://www.hpa.org.uk/Publications/InfectiousDiseases/AntimicrobialAndHealthcareAssociatedInfections/1205HCAIEnglishPPSforhcaiandamu2011prelim/
- Tumbarello M, Spanu T, Di Bidino R, et al. Costs of bloodstream infections caused by Escherichia coli and influence of extended-spectrum-beta-lactamase production and inadequate initial antibiotic therapy. Antimicrob Agents Chemother. 2010;54:4085–4091. doi: 10.1128/AAC.00143-10
- Wang Y, Xiao T, Zhu Y, et al. Economic burden of patients with bloodstream infections caused by extended-spectrum β-lactamase-producing Escherichia coli. Infect Drug Resist. 2020;13:3583–3592. doi: 10.2147/IDR.S271230
- Gould IM, Reilly J, Bunyan D, et al. Costs of healthcare-associated methicillin-resistant staphylococcus aureus and its control. Clin Microbiol Infect. 2010;16(12):1721–1728. doi: 10.1111/j.1469-0691.2010.03365.x
- Filice GA, Nyman JA, Lexau C, et al. Excess costs and utilization associated with methicillin resistance for patients with staphylococcus aureus infection. Infect Control Hosp Epidemiol. 2010;31(4):365–373. doi: 10.1086/651094
- Aldeyab MA, McElnay JC, Scott MG, et al. Hospital antibiotic use and its relationship to age-adjusted comorbidity and alcohol-based hand rub consumption. Epidemiol Infect. 2014;142:404–408. doi: 10.1017/S0950268813001052
- Aldeyab MA, McElnay JC, Scott MG, et al. A modified method for measuring antibiotic use in healthcare settings: implications for antibiotic stewardship and benchmarking. J Antimicrob Chemother. 2014;69:1132–1141. doi: 10.1093/jac/dkt458
- Aldeyab MA, Harbarth S, Vernaz N, et al. The impact of antibiotic use on the incidence and resistance pattern of extended-spectrum beta-lactamase-producing bacteria in primary and secondary healthcare settings. Br J Clin Pharmacol. 2012;74:171–179. doi: 10.1111/j.1365-2125.2011.04161.x