ABSTRACT
Introduction: At birth, the gastrointestinal (GI) tract is colonized by a complex community of microorganisms, forming the basis of the gut microbiome. The gut microbiome plays a fundamental role in host health, disorders of which can lead to an array of GI diseases, both short and long term. Pediatric GI diseases are responsible for significant morbidity and mortality, but many remain poorly understood. Recent advancements in high-throughput technologies have enabled deeper profiling of GI morbidities. Technologies, such as metagenomics, transcriptomics, proteomics and metabolomics, have already been used to identify associations with specific pathologies, and highlight an exciting area of research. However, since these diseases are often complex and multifactorial by nature, reliance on a single experimental approach may not capture the true biological complexity. Therefore, multi-omics aims to integrate singular omic data to further enhance our understanding of disease.
Areas covered: This review will discuss and provide an overview of the main omic technologies that are used to study complex GI pathologies in early life.
Expert opinion: Multi-omic technologies can help to unravel the complexities of several diseases during early life, aiding in biomarker discovery and enabling the development of novel therapeutics and augment predictive models.
1. Background
The ‘gut microbiome’ describes the extensive consortia of microorganisms that colonize a host. In doing so, the gut microbiome plays fundamental roles in health, including the digestion of dietary components and education of the immune system [Citation1]. Disorders of this microbiome can also lead to a diverse range of gastrointestinal (GI) and systemic diseases and have been linked to obesity, diabetes, and cardiovascular disease in later life [Citation2–4]. The relationship between the gut microbiome and the occurrence of diseases as diverse as GI diseases, autoimmune disorders, and mental health illnesses, is complex and associated at all stages with a disordered microbial composition [Citation5].
Early life development of the gut microbiome is of critical importance in short- and long-term GI health [Citation6–9]. Changes in the microbiome can lead to disordered, dysregulated host stability, and increased susceptibility to disease [Citation10–13]. Such diseases often present in variable ways and can be challenging to diagnose with current conventional techniques, relying on clinical symptoms and patterns of nonspecific blood biomarkers, such as C-reactive protein [Citation14,Citation15]. The gut is perhaps the most critical in providing opportunities for host–microbiome interactions and modifications of the host immune system, as it is here that there is the largest density of bacteria [Citation16].
The term omics encompasses many technologies, including genomics, metagenomics, transcriptomics, proteomics and metabolomics [Citation17]. Next generation omic technologies have allowed for a much more detailed understanding of host genetics and functional genetics in relation to disease [Citation18]. In addition, meta-omics studies (metagenomics, metatranscriptomics, metaproteomics and metabolomics) have increasing use in studying the gut microbiome community, with their ability to provide an insight into strain level taxonomic resolution, functional and metabolic capabilities of the microbiome [Citation19]. These technologies have demonstrated powerful potential in clinical research and provided insight into omic signatures of pediatric disease [Citation20–23]. For instance, improvement in molecular sequencing coupled with rapidly declining costs has enabled research into the differences in the microbiome between individuals, populations, and species [Citation24]. The ability to model multi-omic data and correlate this with clinical findings allow the possibility to identify underlying biomarkers and other modifiable factors that will allow for early detection of disease [Citation25,Citation26]. The use of real-time techniques to identify disease has gone some way to more rapid diagnosis and treatment of potentially pathogenic illness [Citation27]. Thus, increasingly, omic technologies can be used to target therapies to treat these varied pathologies.
It is noteworthy that experimental design is critical to generating accurate and actionable results. Cohort studies are important to understand human disease, where various samples can be analyzed using omic pipelines, including stool, serum, urine and resected tissue [Citation28] (). Stool samples are important for understanding what the intestine is exposed to urine includes the host metabolites which are excreted from the body; blood gives the picture of the systemic response to the disease and resected tissue can give information on the response mounted at the site of disease [Citation29,Citation30]. Notwithstanding, the complexity and individual variation in clinical and environmental exposures can pose significant challenges in data analysis that is ultimately underpinned by the quality and granularity of accompanying metadata.
Figure 1. Schematic representation of a multi-omic study. Specifically, the multi-omic workflow from sample collection through to the application of findings is shown
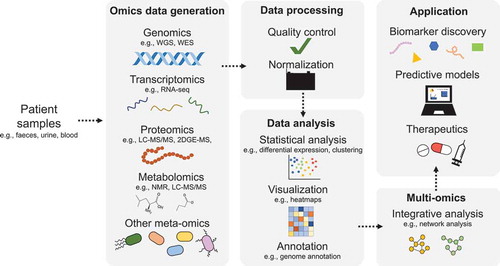
In this review, we focus on describing the different omic technologies that are currently used for pediatric disease diagnostics and characterization. In addition, we appraise recent advances in multi-omics that have increased our understanding of the gut microbiome and its interaction with GI diseases in childhood.
2. Omic techniques used for the diagnosis and characterization of pediatric diseases
As will be discussed in this section, the advancement of high-throughput and increasingly sensitive techniques for profiling clinical samples, offers huge promise for medical research and translation into clinical medicine. Interest in utilizing omic approaches is on the rise, as demonstrated by the exponential increase in omic papers over the past 20 or so years () [Citation31]. It is therefore important to underscore the need for appropriate study design and sample collection to ensure reliability of the results. Each technique requires specific consideration for collection and storage of samples. To this end, commercial products containing optimized buffers exist to allow stabilization of samples. However, there is currently no such product that would allow downstream analysis across all omic platforms. For instance, kits are used to stabilize DNA for genomics and microbiome studies would likely render the sample unusable for metabolomics and proteomics. To overcome this, multiple-collection methods can be used, but this will increase the overall cost of studies and add extra burden to staff, carers, and patients. Following sample collection, and depending on the collection method used, samples should be treated uniformly across a study. Specifically, samples should be processed or frozen within comparable timeframes to prevent introducing potential bias. Failure to collect and store samples under consideration for the intended analysis can render them unsuitable for many/all omic platforms.
Figure 2. The trend of omic-related studies over time. This was defined as the number of papers published, as measured by the yearly number of articles in PubMed containing the respective omic keyword
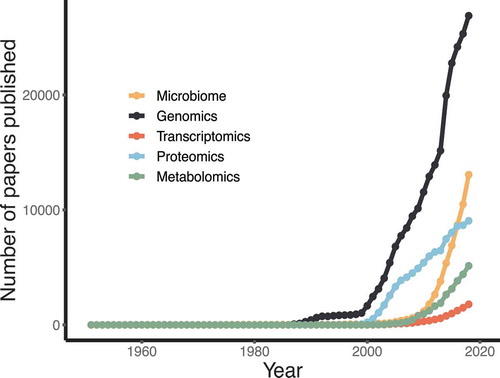
Regardless of the planned analysis, the generation of accurate and detailed metadata is of crucial importance [Citation32]. This is vital in uncovering meaningful and biologically robust associations, while correcting for potentially confounding factors that could influence outcomes [Citation33].
2.1. Genomics and metagenomics
Genetic predisposition to pediatric disease makes genomic studies of significant importance. Genomics is the study of the whole genome of an organism, and in a clinical context it can be used to map and identify genetic variants and potential risk factors that can contribute to certain diseases [Citation34]. There are a number of genomic associated studies, namely genome-wide association studies (GWAS), whole genome sequencing (WGS) and whole exome sequencing (WES). GWAS looks to identify genetic variation associated with specific diseases by genotyping thousands of individuals for genetic markers, which then allows specific genetic variants to be associated with disease [Citation35]. WGS aims to sequence the entire genome rather than just focusing on genetic markers, which ultimately captures the more rare genetic variants associated with both common and rare diseases [Citation35,Citation36]. Finally, WES involves sequencing the protein-coding regions of the genome, otherwise known as the exome. This approach is less expensive than WGS and well justified, since the vast majority of allelic variants known to underlie Mendelian disorders disrupt protein-coding sequences [Citation37].
Another important contributing factor to many pediatric GI diseases, as discussed, is the human gut microbiome. To study the microbiome, at a taxonomic level, metagenomics techniques are commonly employed. Unlike genomics techniques which study the genetic material of a single organism (the patient), metagenomics focuses on the study of all the genetic material present in a given environment, such as the GI tract. In a clinical context, metagenomics is very powerful for microbiome studies and enables researchers to identify specific bacteria that may be associated with disease states. Traditionally, microbiome studies have relied on culture-based techniques and more recently amplicon gene sequencing, which allows genus-level identification based on a phylogenetic marker gene, typically the 16S rRNA gene [Citation38]. However, metagenomics offers advantages over these methods, allowing strain-level classification of both culturable and unculturable species and analysis of the functional content of samples [Citation38]. This more granular level of information is of great benefit when studying the intestinal microbiome due to the complexity of such a community and its genetic content, as it is estimated that there are approximately 5 million unique genes, many of which contribute to host health and metabolism [Citation39].
Typically, a metagenomics study would comprise the following workflow: (a) sample collection; (b) DNA extraction; (c) sequencing; (d) bioinformatic processing of sequencing reads; (e) taxonomic and functional profiling and statistical analysis, and (f) validation [Citation32].
2.2. Transcriptomics and metatranscriptomics
Given the importance of identifying underlying mechanisms and biomarkers for many GI pediatric diseases, gene expression studies represent yet another crucial field. Transcriptomics is the comprehensive study of all RNA transcripts transcribed by the genome, usually in a specific cell, at a given developmental stage, or under a specific set of conditions [Citation40]. This includes both coding and non-coding RNA transcripts. Transcriptome profiling studies, therefore, are a very powerful way of dissecting specific transcriptional signatures of disease by allowing the identification of differentially expressed genes between healthy and diseased patients. The transcriptome of a patient can be captured using similar methods to those used for screening genetic content, for example, by RNA-microarrays or RNA-sequencing [Citation41]. RNA-sequencing offers a more comprehensive analysis of the transcriptome than microarray experiments and does not require a priori knowledge [Citation41].
To give insight into the active functional profile of the gut microbiome, metatranscriptomics studies are used [Citation42]. Currently, metatranscriptomics analyses rely on the same methods as used in transcriptomic experiments, i.e. RNA-seq, but aim to study all the transcripts from microbial communities within a sample. Since metagenomics focuses only on the presence of organisms or genes within a community, it fails to capture whether these organisms are active members [Citation43]. Metatranscriptomics offers a solution, allowing a deeper insight into gene expression within the microbiome, looking, in particular, at longitudinal changes in response to the environment. However, in clinical samples, such as stool, the transcription profiles will be derived from a range of cell types, including cells that have sloughed off at varying hours previously. The impacts on gene expression levels as cells transit through the gut GI tract are not well understood, which limits the ability to make accurate biological associations.
The workflow for a typical (meta)transcriptome study is as follows: (a) purifying RNA of interest; (b) converting RNA to complementary DNA (cDNA); (c) labeling and hybridizing cDNA to probes on chip (RNA-microarray) or fragmenting cDNA and building library to sequence (RNA-seq); (d) running microarray or sequencing; (e) quality control; (f) statistical analysis [Citation41].
Over the past decade, single-cell RNA sequencing (scRNA-seq) has been increasingly implemented in clinical research. This technology offers notable advances over bulk-RNAseq, which in effect averages across a huge pool of individual cells. This averaging can fail to detect biologically meaningful differences within a given population of cells. scRNA-seq overcomes this by providing gene expression data for each individual cell, ultimately allowing biologically meaningful cell-specific information to be captured without ‘noise’ from unrelated cells in a mixed population [Citation44]. Building on from the importance of understanding complex cellular populations, attention has more recently turned to the spatial orientation of cells within a given tissue sample. While the technologies described above involve the destruction of a sample in the initial stage of the protocol, spatial transcriptomics maintains the integrity of tissue to show site-specific gene-expression. Such is the advancement and enormous potential for single cell and spatial transcriptomics, these two methods have been named ‘Nature Method of the Year’ in 2019 and 2020, respectively [Citation45,Citation46].
2.3. Proteomics and metaproteomics
Proteins can be considered as the functional entities of cells, involved in cellular structure and activity, providing the mechanisms for signaling between cells and tissues and catalyzing metabolic reactions [Citation47,Citation48]. The central dogma refers to the unidirectional passage of information within cells, from DNA to RNA to protein [Citation48]. Proteomics is the study of all the protein contents within a given cell, tissue or organism in a given state. Alike transcriptomics, proteomics can be used to identify differential expression between healthy and disease states. This large-scale detection and characterization of proteins have proven crucial for biomarker discovery, early disease diagnosis, and prognosis [Citation49,Citation50]. The flow of information from DNA to RNA and from RNA to protein is expected to correlate; however, owing to protein degradation, post-transcriptional and translational regulatory processes, transcriptomics may not provide a robust estimate of protein abundance. Proteomics is also inherently more complex than transcriptomics, due in part to the 20-character alphabet of amino acids and complications associated with post-translational modifications including phosphorylation and glycosylation.
In this field, proteomics employs a variety of methodologies which over time, have built upon the conventional workflow. A typical proteomic workflow consists of sample collection and preparation, separation of proteins within samples and analysis by mass spectrometry (MS). Traditionally, separation of proteins within a sample is achieved by two-dimensional gel electrophoresis, whereby proteins are separated based on isoelectric point and molecular weight [Citation51]. More recently, however, separation is often conducted using chromatography-based methods such as high-performance liquid chromatography (HPLC). Following separation, MS is used to measure the mass-to-charge ratio of proteins, and is therefore useful in determining the molecular weight of proteins [Citation50]. Mass spectrometers generally consist of an ion source, a mass analyzer, and an ion current detector [Citation42]. Combining the two analyzers allows for tandem MS, which is currently the preferred technique for proteomic analyses [Citation42]. The most commonly used ionization methods for proteomic MS are electrospray ionization (ESI) and matrix assisted laser desorption ionization (MALDI), although there are several variations on MS-based techniques [Citation42,Citation50]. Owning to extensively annotated databases, it is possible to identify the vast majority of peptides/proteins detected.
The analysis of the protein content from microbial communities, such as the gut microbiome, is known as metaproteomics [Citation52]. Much like metatranscriptomics, metaproteomic-based studies provide insight into the functional activity of microbial communities and can therefore provide a deeper understanding of the relationship between the microbiome and host [Citation52]. Metaproteomics again follows the same workflow as proteomic experiments and exploits tools, such as MS, but differs slightly in sample type and subsequent analysis.
2.4. Metabolomics
The complex, dense, community of microorganisms in the GI tract is also responsible for the production of a number of metabolic compounds [Citation53]. Metabolites are functional small molecules that represent the intermediate or end product of metabolism. They modulate a variety of signaling pathways which facilitate intestinal mucosa homeostasis [Citation53]. The host metabolome is therefore heavily intertwined with and connected to gut microbial communities. Metabolomics is the study of all the metabolites from a given ecosystem, providing insight into the function of the microbiome and the host metabolic profile. Metabolite profiles can differ between healthy and disease subjects in a range of conditions and pathologies, some of which can be detected at unrelated body sites. For instance, the detection of metabolites in urine in relation to conditions that primarily impact the gut or brain. Such detection of metabolic alterations provides targets for noninvasive biomarkers and enables the mechanisms of disease development to be elucidated [Citation21].
Metabolomics studies typically utilize MS or nuclear magnetic resonance (NMR) based techniques. For MS-based techniques, metabolites are first separated using one of a variety of methods, including but not limited to gas chromatography (GC) and ultra-performance liquid chromatography (UPLC) [Citation53]. The choice of chromatography will impact which metabolites are retained and subsequently detected by MS. The choice of column used is therefore important and should reflect the study hypotheses or multiple different columns could be used, such as combining reverse-phase liquid chromatography (RPLC) and hydrophilic interaction liquid chromatography (HILIC) [Citation54]. Recent efforts have also focused on combining different chromatography to maximize the number of metabolites detected. NMR spectroscopy, on the other hand, exploits the local magnetic field that exists around atomic nuclei, allowing the molecular structure of metabolites to be elucidated. MS-based techniques are more sensitive than NMR, but require known standards to be run alongside samples in order to identify metabolites of interest [Citation55]. Without doing so, any identification can only be putative and therefore most features in an LC-MS experiment will remain unidentified.
2.5. Multi-omics
Single omics approaches have provided important progress in the understanding of a range of complex diseases. Individually, however, these tools are often unable to capture the true biological complexity of most diseases [Citation18]. For instance, changes in specific bacterial species using microbiome sequencing may have little or very profound impacts on the overall function of the microbiome and microbe–host interaction. Advances in systems biology have enabled the integration of multiple types of omics data, termed multi-omics, which allows for a more comprehensive analysis and may provide important advances in pediatric disease [Citation18,Citation34,Citation56]. This is especially true in the case of diseases, such as inflammatory bowel disease (IBD) and necrotizing enterocolitis (NEC), which arise as a result of the interplay between a range of host and environmental factors [Citation57]. Thus, integrative approaches are advantageous, particularly when studying these kinds of diseases, as they provide a more holistic view. The advent of this more recent integrative approach paves an exciting possibility for the future of pediatric disease characterization and diagnostics.
3. Application of omic techniques in pediatric gastrointestinal disease
In the following section, we will discuss the current application of omic techniques in pediatric gastrointestinal disease, and consider the recent advances in this field. A summary of the information discussed can be found in .
Table 1. Summary of the pediatric gastrointestinal diseases, omic techniques, samples and discoveries described in this review
3.1. Preterm neonates – necrotizing enterocolitis and sepsis
NEC and late onset sepsis (LOS) are two severe morbidities primarily affecting preterm infants born <32 weeks of gestation and are the leading cause of death in this vulnerable population [Citation58]. NEC is a potentially catastrophic GI inflammatory disease leading to bowel perforation, cardiovascular compromise, and death [Citation59]. It has a mortality of 30% and in infants who go on to require surgical intervention this can be as high as 50% [Citation60]. Due to the multifactorial etiology of NEC, there are challenges in differentiating presenting clinical features from LOS. Despite decades of research, there are currently no biomarkers for predicting or diagnosing these diseases, and NEC pathophysiology is still poorly understood. Early clinical presentation of NEC is nonspecific and diverse, with typical features (e.g. the radiological finding of pneumatosis intestinalis) found in later stages of disease, and not universally. NEC and LOS in preterm infants are pathologies that would lend themselves to metabolomic or proteomic profiling to identify biomarkers that could predict or differentiate these pathologies [Citation61].
Stool samples collected from preterm infants have been characterized over the years, with a particular focus on the microbiome composition [Citation62]. A gut microbiome rich in Proteobacteria, low in diversity, and with a delayed colonization by strict anaerobic bacteria including Bifidobacterium spp. has been associated with NEC [Citation13,Citation63–65] and LOS [Citation66–69] in multiple studies. A prevalence of Proteobacteria in the intestine of NEC infants has also been reported by the characterization of formalin-fixed paraffin-embedded tissues, suggesting the direct exposure of this phyla at the mucosal level [Citation70,Citation71]. Despite the association between NEC and Proteobacteria dominance, no causative bacterial genera or species has been consistently associated with the disease [Citation13,Citation65,Citation72]. Enteric bacteria such as Klebsiella spp., Pseudomonas spp., Enterococcus spp., and Escherichia coli are dominant members of the preterm gut microbial community and are also common bacteria causing LOS [Citation67,Citation68]. Previous work has reported these causative microorganisms in blood culture positive LOS as being among the most abundant in the infant gut at time of diagnosis [Citation66]. Indeed, owing to the immaturity of and leakiness of the preterm intestinal epithelium, the gut could represent one site of translocation for the organism causing sepsis.
Beyond the detection of bacterial taxa, different bacterial species can interact with the host through shared pathways. For instance, Gram-negative bacteria which belong to the Proteobacteria phylum, are rich in lipopolysaccharide which bind toll-like receptor 4 (TLR4) to induce a pro-inflammatory response in the host. Different microbiome compositions can also be similar in their functional potential, thus deeper information on the gene content and metabolites produced by the gut community could give new insights on NEC and LOS onset. In the largest existing preterm metagenomic dataset of 1163 stool samples, Olm et al. (2019) found increased bacterial replication and fimbriae-encoding bacteria to be associated with NEC onset [Citation73].
Metabolomics is still relatively under-explored in preterm infants, and few studies have coupled microbiome and metabolome analyses. Stewart et al. (2016) reported that stool metabolites involved in linoleate metabolism inversely correlated with a bifidobacteria-dominated gut microbiome, which together was associated with onset of NEC [Citation13]. In another study by the same group, raffinose, sucrose, and acetic acid correlated to a microbiome rich in bifidobacteria, which was associated with reduced risk of LOS [Citation66]. Conversely, Wandro et al. (2018) reported no association of the gut microbiome component or stool metabolites with NEC nor LOS [Citation74]; however, this study was a small cohort and utilized GC-MS, while the studies from the Stewart group applied UPLC-MS.
In addition to stool, urine metabolomic studies have also been performed, which should provide a more readily available biofluid, especially in diseased preterm infants who may have reduced or nil feeds and thus no stool for periods of time. In a novel approach, Morrow et al. (2013) coupled stool 16S rRNA gene sequencing with urine metabolite characterization. Two distinct types of NEC microbial dysbiosis were observed, which were subsequently associated with a range of metabolites in the urine [Citation65]. Notably, no metabolite could significantly distinguish between all NEC cases together versus controls, underscoring the importance of separation between potentially different NEC pathologies. Finally, a small NMR and LC-MS focused study identified many possible urine metabolites for NEC and LOS in preterm infants, particularly reduced amino acids [Citation75]. Building on this previous work, several studies are now underway which combine stool microbiome, stool metabolome, and urine metabolome, including the mechanisms affecting the gut of preterm infants in enteral feeding trials (MAGPIE) study [Citation28].
While urine and stool have been used to noninvasively investigate preterm infant metabolism and microbiome in health and disease, blood samples can be utilized to provide insight into the systemic immune system and potentially specific biomarkers. Since salvageable blood volume in preterm infants will be relatively low, some studies have utilized proteomics and metabolomics to study this biofluid. In one of the first studies of its kind, metabolome and proteome analyses of blood serum from NEC, LOS, and control babies revealed a highly conserved blood profile between healthy and diseased patients [Citation14]. In another study on serum metabolomics, Wilcock et al. (2014) reported an alteration in 16 metabolites between NEC and control infants, of which 7 were involved in interleukin (IL) 1 beta (IL1B) upregulation and increased in NEC infants [Citation76]. In both studies, alterations observed in diseased babies appeared personalized and no consistent biomarker detected, potentially reflecting different mechanisms underlying disease onset. Alteration in blood apolipoproteins has also been associated with NEC and LOS in two different studies applying proteomic analysis on blood samples [Citation77,Citation78]. While some of the altered proteins could be potential biomarkers, both studies analyzed samples starting from the day of diagnosis and thus further analysis is needed to verify how early these alterations can be observed.
The importance of the immune system in NEC development has been reported multiple times, with alterations observed in transforming growth factor beta (TGFB) production and regulatory T cells (Tregs) representation. A study measuring immune proteins in blood reported TGFB, IL2 and IL8 associated with NEC [Citation79]. Importantly, TGFB was lower in NEC infants from birth and could be used for early identification of babies at risk of developing the disease. Pang et al. (2018) also reported lower production of TGFB and IL10 in Tregs from NEC babies [Citation80]. A lower representation of Tregs in NEC compared to non-NEC infants has also been found in resected tissue, coupled with an increased expression of pro-inflammatory cytokines, such as IL1B and IL8 [Citation81], highlighting the inflammatory nature of this disease.
Transcriptome analysis of tissue from the site of disease can also give insights into what is the underlying mechanism bringing to the damage in NEC and spontaneous intestinal perforation (SIP), which is a less severe and less fatal complication when compared to NEC. Chan et al. (2012) reported differences in tissue resected from NEC and SIP babies, whereby NEC tissue showed more pronounced inflammatory gene expression which was associated with the interaction of Gram-positive and Gram-negative bacteria [Citation82]. Also, a resemblance between NEC and Crohn’s disease (CD) transcriptome was reported, which could help guide the development of a universal biomarker for gut inflammation. In line with these findings, Tremblay et al. reported similarities between NEC and CD tissue in two different studies. In 2016, they observed that more than 60% of genes altered in NEC tissue were also associated with CD, with immunity and infection pathways being involved [Citation83]. Moreover, an up-regulation of TLR4 was reported in NEC tissue. In a more recent study (2021), a specific role of IL17-mediated inflammation was reported, with increased expression of anti-microbial genes and inflammatory mediators [Citation84].
Mothers’ own breast milk significantly influences the development of the microbiome and is known to be the single most important factor for reducing disease in preterm infants, but babies receiving breast milk can still develop the disease, suggesting potentially important alterations in breast milk composition [Citation85]. For instance, alterations in concentrations of complex sugars present in breast milk, termed human milk oligosaccharides (HMOs), have been reported in mothers of infants who develop NEC compared to control infants. In particular, a single sugar called disialyllacto-N-tetraose (DSLNT) has been repeatedly found in higher levels in mothers breast milk fed to healthy control infants compared to NEC infants [Citation86,Citation87]. Moreover, a recent study found this HMO could separate healthy vs. NEC infants with specificity and sensitivity of 0.9 [Citation87]. This provides tangible possibilities for the utilization of DSLNT as a biomarker to identify preterm infants at higher risk of NEC, as well as a target for therapeutic supplementation and to prioritize donor milk high in DSLNT for use in preterm infants.
3.2. Pediatric inflammatory bowel disease
IBD is an umbrella term encompassing a range of complex, chronic GI conditions with a relapsing course, primarily ulcerative colitis (UC) and CD, and the incidence is increasing in the pediatric population. Alike NEC, IBD is multifactorial, with the environment, host genetics, and gut microbiome being involved and leading to a dysregulation in the host-microbiome cross-talk [Citation88]. Indeed, numerous genes associated with IBD susceptibility are involved in the immune response and epithelial function, which has been linked with an alteration in the gut microbiome [Citation89]. While UC affects the mucosa of rectum and colon and is associated with an increase in neutrophils and depletion of goblet cells, CD can impact any part of the GI tract and is accompanied by an aggregation of macrophages [Citation89]. Multi-omics techniques prove a novel way to differentiate between CD and UC. However, these are mainly extrapolated from adults to children, and should be interpreted with caution, as metabolic status in children is also intrinsically linked to growth and development in relation to changing physiology [Citation21].
As in the adult cohort, pediatric IBD (pIBD) has been associated with altered gut microbiome composition, which also links to the metabolism of the patients. Higher abundance of facultative anaerobic bacteria and pathobionts is observed in children affected by IBD [Citation90–95]. However, the gut microbiome alteration could be a consequence of the host genetic profile which has been shown to influence the intestinal microbial community, rather than a causative factor leading to the disease. Lower bacterial diversity in the gut has been repeatedly reported in pIBD when compared to healthy controls [Citation91,Citation94–96]. Contradictory results have been found in tissue biopsies and in mucosal-luminal interface (MLI) aspirates, with some studies reporting no difference in bacterial diversity [Citation93,Citation97]. Analysis of other microorganisms in the gut has shown higher prevalence in fungal colonization [Citation98], but no difference has been observed in archaea composition [Citation94,Citation96]. A study applying microbiome characterization in both biopsies and stool samples reported the bacteria that were significantly associated with disease varied depending on sample, suggesting that the stool microbiome might not reflect the microbial community at the site of disease [Citation92]. Importantly, biopsy samples could predict disease severity 6 months earlier than stool samples. Schirmer et al. (2018) instead analyzed rectal biopsies (i.e. more accessible but not reflective of the site of disease) and stool samples, finding comparable relative abundance between the two sample types [Citation99]. This could have important implications for appropriate samples for biomarker development, where stool offers reliable profiling of the microbiome for the distal colon in the absence of invasive biopsy samples.
Many bacterial genera and families have been repeatedly reported as altered in pIBD patients within a range of sample types including stool, biopsies, or MLI aspirates. The most frequent alterations in the pIBD microbiome include higher abundances of Veillonellaceae, Haemophilus, and Enterobacteriaceae, which have also been correlated with disease severity, and lower abundances of Akkermansia, Clostridiales and Bacteroidales when compared to healthy controls [Citation90,Citation92–95,Citation97,Citation98,Citation100,Citation101]. Supporting the importance of the microbiome in pIBD, early antibiotic administration has been identified as a risk factor for disease development [Citation89]. In this regard, antibiotic usage has been associated with depletion of Clostridiales, Lachnospiraceae, and Ruminococcaceae, all of which are associated with disease severity [Citation99]. Moreover, these types of bacteria are known for producing short-chain fatty acids (SCFA) which have beneficial effects on human health, particularly at immune system regulation level. However, like NEC and LOS in preterm infants, not all pIBD patients have the same pattern of microbial development and a single or combination of pathobionts has not been consistently associated with disease onset. For instance, two distinct pIBD microbiome clusters were reported in a study involving pIBD and healthy and diseased relatives, with one cluster harboring a high degree of dysbiosis and the other a composition intermediate between the highly dysbiotic and the healthy cluster [Citation95]. An emerging and exciting area in microbiome research involves the study of how the microbial community can impact response to a given therapy. A recent metagenomic study has found children responding to therapies, such as anti-tumor necrosis factor (TNF) alpha and exclusive enteral feeding, showed a shift in the microbial community toward that of healthy controls [Citation94].
Multiple studies have investigated the metabolome in IBD, applying different techniques and utilizing different sample types, including tissue, MLI, stool, urine, and serum. Noninvasive biofluids and blood samples offer a quick and convenient means of determining biological activity. Kolho et al. (2017) analyzed the metabolome of serum and stool from pIBD patients, including UC, CD, unclassified IBD, and healthy controls [Citation102]. Amino acid metabolism, folate biosynthesis, and signaling pathways were enriched in serum obtained from CD patients, while UC patients additionally showed enrichment in sphingolipid metabolic pathways and bile acid metabolism but no alteration in signaling pathways. While differences were reported in serum, a higher separation between IBD and controls and between subtypes was observed in fecal metabolome, with CD patients showing intermediate metabolite levels which were higher in UC. Hippurate has also been repeatedly found altered in pIBD, and it is associated to microbiome metabolism, further supporting the presence of an altered microbiome in IBD, which, with its metabolites, could provide potential biomarkers measured either in urine or stool [Citation102–104]. In another study involving CD and UC patients and healthy relatives, CD stool metabolome showed the greatest alterations with enrichment in amino acid derivatives and bile acids [Citation95]. Finally, urine metabolomics has also reported differences in amino acid and fatty acid metabolism and bile acid bacterial co-metabolism between pIBD and matched controls [Citation103]. Notably, the concentration of urea in urine is inversely correlated with the most severe cases.
While noninvasive urine/stool and blood are convenient samples and ideal targets for biomarkers, they cannot provide specific information relating to the site of disease, which is more likely to provide mechanistic insight. Mottawea et al. (2016) characterized the microbiome and proteome of MLI aspirates, showing that CD patients had increased bacteria producing hydrogen sulfide (H2S) and downregulation in mitochondrial proteins necessary for H2S detoxification [Citation93]. However, the presence of butyrate-producing bacteria was associated with an increase in mitochondrial proteins, suggesting host-microbial cross-talk is altered in CD patients. In a separate study, the same group reported an increase in proteins associated with defense mechanisms from the microbiome and higher levels of L-cysteine desulfidase involved in the production of H2S, further suggesting an altered host-microbiome cross-talk [Citation98]. A proteome profile enriched in anti-bacterial and general inflammatory immune response has also been reported in a separate study utilizing MLI aspirates [Citation105]. This latter study identified a panel of four proteins able to correctly classify >95% pIBD and healthy children. Alteration of two of the panel proteins was also confirmed in stool through enzyme-linked immunosorbent assay (ELISA), demonstrating clear potential for diagnosis using this noninvasive sample. Moreover, a different panel of four proteins (one in common with the other panel) could classify UC severity with 100% accuracy. These panels included leukotriene A4 hydrolase, which added to a panel of 11 other proteins separated CD and UC in patient biopsies [Citation106]. However, in both studies, the proteome levels were analyzed starting from invasive samples (MLI aspirates and biopsies), and analysis using more accessible noninvasive samples would improve prospective diagnostic. Nonetheless, when patients undergo biopsy for pIBD diagnosis, protein panels are valuable to guide disease stratification and identification of the best treatment.
Alongside the gut microbiome, transcriptomic analysis of intentional biopsies has also been a major area of research in pIBD. In the RISK cohort [Citation107], Haberman et al. (2014) compared the transcriptome of CD and UC patients and healthy controls, also subtyping CD depending on the involvement of the ileum area. Ileal CD biopsies showed an alteration in the immune system signaling and lipid metabolic function, with higher DUOX2 (an innate anti-microbial gene), and lower APOA1 (involved in lipid metabolism). While DUOX2 was increased in the ileum of all forms of pIBD, ileal APOA1 suppression was specific to CD patients independent of ileum inflammation. Thus, analyzing the expression of APOA1 and co-expressed genes, could help distinguish between UC and CD, even for pIBD impacting non-ileal intestinal sites. Moreover, dysbiosis was present in the ileum of colonic CD patients, with increased taxa belonging to the Proteobacteria phylum. Such taxa were increased in patients and also correlated with DUOX2 levels, suggesting that alterations in the microbial community might be a cause of, rather than effect of, the inflammatory status. In the end, baseline expression of APOA1 correlated with later response to therapeutics, again indicating the importance of having a clear diagnosis to decide the best therapy. A subsequent study on pediatric CD patients from the RISK cohort, found that baseline luminal narrowing was associated with decreased abundance of butyrate-producing commensals, coupled with increased proinflammatory and profibrotic gene expression [Citation108]. In a different cohort, Haberman et al. (2019) investigated the transcriptome of UC patients. In concordance with alterations reported in proteomic and metabolomic studies, they confirmed a reduction in mitochondrial genes and pathways, and genes associated with lipid metabolism [Citation90]. The study also highlighted correlations between specific bacteria and gene expression, including Clostridiales negatively correlated with genes related to disease severity and poor treatment response. Combining microbiome analysis (from stool or samples from the site of disease) with host transcriptomics of intestinal biopsies is a powerful approach for elucidating potential disease mechanisms and identifying targets to distinguish between the different pIBD subtypes and help guide the most appropriate therapy.
4. Conclusion
This review has covered several aspects of omics in modern clinical research. With continued improvement to technologies, the potential of omics continues to offer huge promise for both research and clinical use. However, technological advances do not overcome shortcomings in experimental design, sample collection, appropriate controls, and robust statistical analyses. It therefore remains critical that studies are designed with considerations for the most appropriate methods to test the hypotheses. The completeness and extensiveness of the supporting metadata, which at a minimum, needs to account for all potential confounders, will also be imperative in deriving robust and meaningful conclusions. Appropriate validation in external cohorts and relevant model systems will also be necessary to narrow potential biomarker targets and allow mechanistic understanding. While there is still much work to be done, omics technologies are undoubtedly advancing our understanding of many diseases impacting early life and beyond.
5. Expert opinion
Technological and scientific advancement continue to enable deeper understanding of a range of pediatric diseases. However, translating “clinical research” into ‘clinical practice’ is not trivial. Such is the interest and excitement in omic fields, researchers are often faced with the question of “so what?”. It’s a fair question and one that should be considered for omic studies where the results are largely based on associations, meaning causality cannot be implicated, and where mechanistic understanding is lacking. Yet, despite an explosion in related papers over the past decade, the omic field is relatively new and to develop new biomarkers/therapeutics and run large randomized controlled trials requires significant time and funding. In time, it is likely we will increasingly see omic technologies directly applied in a clinical setting, as well as targeted biomarkers established from the findings of omic studies. There are already examples of success stories, for instance, “The 100,000 Genomes Project”, a UK wide effort to sequence the 100,000 genomes from patients with rare diseases and cancer, which potentially allows targeted treatment [Citation109].
As with all scientific endeavors, the reported conclusions are only as robust as the underpinning study design, sample collection, data acquisition, and statistical analysis. In many respects, the importance of these elements is amplified in early life studies, where both the host and the microbiome are rapidly developing. Failure to accurately record comprehensive metadata and account for this in matching controls will invariably lead to reported observations between health and disease being confounded. The power of the study should also be considered and it is notable that large multi-site studies rarely recapitulate the findings of small single center cohorts [Citation110]. Indeed, large multi-site, multi-geographical studies are urgently needed to increase the generalizability of research findings.
Many molecular-based omics rely on accurate identification of sequences from databases of known genes/genomes, so read length and quality of database annotation are critical to deriving robust results. Great strides have been made in recent years to generate longer sequence reads and such a breakthrough will greatly enhance the ability of de novo assembly, potentially putting the technology one step closer to routine clinical use. In metabolomics, it is often the case that data analysis will find features of unknown identity to be significant, limiting the utility of such results. Nonetheless, with continual advancement in chromatography and instrument sensitivity, the ability to accurately identify features of interest is likely to improve. Another important consideration that applies to most omic studies is that the resulting data is often sparse and compositional. This poses additional challenges for commonly used statistical analyses that expect count data. Multiple comparisons (i.e. analysis of 100–1000 s of features) and repeated measures (i.e. multiple samples from the same individual) must also be accounted for in any analysis. One expects that in the coming years robust statistical packages will be developed to specifically analyze multi-omic datasets, helping with standardization and ultimately increasing comparability between studies and generalizing the results.
With little advancement in the understanding or clinical care for many multifactorial complex pediatric diseases, a new wave of multi-omic discovery research may offer huge progress. Such integrative technologies can offer a comprehensive starting point for narrowing down targets, including biomarker candidates or specific stimuli for subsequent mechanistic study. They can themselves also be used directly in the clinic to diagnose disease, tailor treatments, and determine a patient’s response to therapeutics. Omic technologies will continue to develop, with higher resolution, increased sensitivity, and better identification packaged within smaller and more user-friendly instruments.
The past five years give a modest insight into the speed at which omic techniques are currently evolving. We therefore anticipate that 5 years from now, clinical trials will be underway to provide guided therapeutic intervention based on an individual’s gut microbiome, by way of stratified or personalized medicine. In parallel, the success of a given intervention will be monitored based on host metabolites detected in noninvasive samples. Ultimately, whatever the future brings, this is only the start for the utility of omic techniques in health and disease across the life course.
Article Highlights
The gut microbiome in early life plays a pivotal role in shaping human health, and alterations in its composition are linked to various diseases, including necrotizing enterocolitis and late onset sepsis in preterm infants, and pediatric intestinal bowel disease.
Technological improvements coupled with reduced costs has led to a huge uptake in applying omic techniques in clinical research
Omic technologies, such as metagenomics, transcriptomics, proteomics, and metabolomics offer great potential to untangle the complexity of gastrointestinal diseases and to discover new biomarkers and potential therapies.
Standardized methods, extensive supporting metadata, and robust statistical approaches are needed to derive meaningful conclusions from omic studies.
Abbreviations
GI: gastrointestinal; GWAS: genome-wide association studies; WGS: whole genome sequencing; WES: whole exome sequencing; cDNA: complementary DNA; scRNA-seq: single-cell RNA sequencing; HPLC: high-performance liquid chromatography; MS: mass spectrometry; ESI: electrospray ionization; MALDI: matrix assisted laser desorption ionization; NMR: nuclear magnetic resonance; GS: gas chromatography; UPLC: ultra-performance liquid chromatography; RPLC: reverse-phase liquid chromatography; HILIC: hydrophilic interaction liquid chromatography; NEC: necrotizing enterocolitis; LOS: late onset sepsis; IBD: inflammatory bowel disease; TLR: toll-like receptor; IL – interleukin; TGFB: transforming growth factor beta; Tregs: regulatory T cells; SIP: spontaneous intestinal perforation; CD: Crohn’s disease; HMOs: human milk oligosaccharides; DSLNT: disialyllacto-N-tetraose; UC: ulcerative colitis; MLI: mucosal–luminal interface; SCFA: short-chain fatty acids; TNF: tumor necrosis factor; ELISA: enzyme-linked immunosorbent assay.
Reviewer disclosures
Peer reviewers on this manuscript have no relevant financial or other relationships to disclose.
Disclosure statement
C. Stewart has acted as a consultant for Astarte Medical and is receiving lecture honoraria from Danone Early Life Nutrition. The authors have no other relevant affiliations or financial involvement with any organization or entity with a financial interest in or financial conflict with the subject matter or materials discussed in the manuscript apart from those disclosed.
Additional information
Funding
References
- Valdes AM, Walter J, Segal E, et al. Role of the gut microbiota in nutrition and health. BMJ. 2018;361:k2179.
- Zhernakova DV, Le TH, Kurilshikov A, et al. Individual variations in cardiovascular-disease-related protein levels are driven by genetics and gut microbiome. Nat Genet. 2018;50(11):1524–1532.
- Durack J, Lynch SV. The gut microbiome: relationships with disease and opportunities for therapy. J Exp Med. 2019;216(1):20–40.
- Kazemian N, Mahmoudi M, Halperin F, et al. Gut microbiota and cardiovascular disease: opportunities and challenges. Microbiome. 2020;8(1):36.
- Marchesi JR, Adams DH, Fava F, et al. The gut microbiota and host health: a new clinical frontier. Gut. 2016;65(2):330–339.
- Berrington JE, Stewart CJ, Embleton ND, et al. Gut microbiota in preterm infants: assessment and relevance to health and disease. Arch Dis Child Fetal Neonatal Ed. 2013;98(4):F286–F290.
- Houghteling PD, Walker WA. Why is initial bacterial colonization of the intestine important to infants’ and children’s health? J Pediatr Gastroenterol Nutr. 2015;60(3):294–307.
- Stewart CJ, Ajami NJ, O’Brien JL, et al. Temporal development of the gut microbiome in early childhood from the TEDDY study. Nature. 2018;562(7728):583–588.
- Bäckhed F, Roswall J, Peng Y, et al. Dynamics and stabilization of the human gut microbiome during the first year of life. Cell Host Microbe. 2015;17(6):852.
- Gómez-Sanchiz M, Cañete R, Rodero I, et al. Influence of breast-feeding and parental intelligence on cognitive development in the 24-month-old child. Clin Pediatr (Phila). 2004;43(8):753–761.
- Martinez I, Maldonado-Gomez MX, Gomes-Neto JC, et al. Experimental evaluation of the importance of colonization history in early-life gut microbiota assembly. Elife. 2018;7:e36521.
- Rautava S. Early microbial contact, the breast milk microbiome and child health. J Dev Orig Health Dis. 2016;7(1):5–14.
- Stewart CJ, Embleton ND, Marrs EC, et al. Temporal bacterial and metabolic development of the preterm gut reveals specific signatures in health and disease. Microbiome. 2016;4(1):67.
- Stewart CJ, Nelson A, Treumann A, et al. Metabolomic and proteomic analysis of serum from preterm infants with necrotising entercolitis and late-onset sepsis. Pediatr Res. 2016;79(3):425–431.
- Ma F, Li S, Gao X, et al. Interleukin-6-mediated CCR9+ interleukin-17-producing regulatory T cells polarization increases the severity of necrotizing enterocolitis. EBioMedicine. 2019;44:71–85.
- Levy M, Thaiss CA, Elinav E. Metabolites: messengers between the microbiota and the immune system. Genes Dev. 2016;30(14):1589–1597.
- Pinu FR, Beale DJ, Paten AM, et al. Systems biology and multi-omics integration: viewpoints from the metabolomics research community. Metabolites. 2019;9(4):76.
- Karczewski KJ, Snyder MP. Integrative omics for health and disease. Nat Rev Genet. 2018;19(5):299–310.
- Knight R, Vrbanac A, Taylor BC, et al., Best practices for analysing microbiomes. Nat Rev Microbiol. 16(7): 410–422. 2018.
- Douglas GM, Hansen R, Jones CMA, et al. Multi-omics differentially classify disease state and treatment outcome in pediatric Crohn’s disease. Microbiome. 2018;6(1):13.
- Filimoniuk A, Daniluk U, Samczuk P, et al. Metabolomic profiling in children with inflammatory bowel disease. Adv Med Sci. 2020;65(1):65–70.
- Kumar M, Garand M, Al Khodor S. Integrating omics for a better understanding of inflammatory bowel disease: a step towards personalized medicine. J Transl Med. 2019;17(1):419.
- Sylvester KG, Ling XB, Liu GY-G, et al. Urine protein biomarkers for the diagnosis and prognosis of necrotizing enterocolitis in infants. J Pediatr. 2014;164(3):607–12.e127.
- Malla MA, Dubey A, Kumar A, et al. Exploring the human microbiome: the potential future role of next-generation sequencing in disease diagnosis and treatment. Front Immunol. 2019;9:2868.
- Bardanzellu F, Fanos V. How could metabolomics change pediatric health? Ital J Pediatr. 2020;46(1):37.
- Tang -Z-Z, Chen G, Hong Q, et al. Multi-omic analysis of the microbiome and metabolome in healthy subjects reveals microbiome-dependent relationships between diet and metabolites. Front Genet. 2019;10:454.
- Kozel TR, Burnham-Marusich AR. Point-of-care testing for infectious diseases: past, present, and future. J Clin Microbiol. 2017;55(8):2313–2320.
- Embleton ND, Berrington JE, Dorling J, et al. Mechanisms affecting the gut of preterm infants in enteral feeding trials. Front Nutr. 2017;4:14.
- Haas R, Zelezniak A, Iacovacci J, et al. Designing and interpreting ‘multi-omic’ experiments that may change our understanding of biology. Curr Opin Syst Biol. 2017;6:37–45.
- D’Adamo GL, Widdop JT, Giles EM. The future is now? Clinical and translational aspects of “omics” technologies. Immunol Cell Biol. 2021;99(2):168–176.
- Corlan AD. Medline trend: Automated yearly statistics of PubMed results for any query, 2004. Accessed 2021-04-27 http://dan.corlan.net/medline-trend.html
- Quince C, Walker AW, Simpson JT, et al., Shotgun metagenomics, from sampling to analysis. Nat Biotechnol. 35(9): 833–844. 2017.
- Vujkovic-Cvijin I, Sklar J, Jiang L, et al. Host variables confound gut microbiota studies of human disease. Nature. 2020;587(7834):448–454.
- Hasin Y, Seldin M, Lusis A. Multi-omics approaches to disease. Genome Biol. 2017;18(1):83.
- Cirulli ET, Goldstein DB. Uncovering the roles of rare variants in common disease through whole-genome sequencing. Nat Rev Genet. 2010;11(6):415–425.
- Liu H-Y, Zhou L, Zheng M-Y, et al. Diagnostic and clinical utility of whole genome sequencing in a cohort of undiagnosed Chinese families with rare diseases. Sci Rep. 2019;9(1):19365.
- Ng SB, Buckingham KJ, Lee C, et al. Exome sequencing identifies the cause of a Mendelian disorder. Nat Genet. 2010;42(1):30–35.
- Gupta S, Mortensen MS, Schjørring S, et al. Amplicon sequencing provides more accurate microbiome information in healthy children compared to culturing. Commun Biol. 2019;2(1):291.
- Walker AW, Duncan SH, Louis P, et al. Phylogeny, culturing, and metagenomics of the human gut microbiota. Trends Microbiol. 2014;22(5):267–274.
- Casamassimi A, Federico A, Rienzo M, et al. Transcriptome profiling in human diseases: new advances and perspectives. Int J Mol Sci. 2017;18(8):1652.
- Manzoni C, Kia DA, Vandrovcova J, et al. Genome, transcriptome and proteome: the rise of omics data and their integration in biomedical sciences. Brief Bioinform. 2018;19(2):286–302.
- Aguiar-Pulido V, Huang W, Suarez-Ulloa V, et al. Metagenomics, metatranscriptomics, and metabolomics approaches for microbiome analysis. Evol Bioinform Online. 2016;12(Suppl 1):5–16.
- Shakya M, Lo CC, Chain PSG. Advances and challenges in metatranscriptomic analysis. Front Genet. 2019;10:904.
- Hwang B, Lee JH, Bang D. Single-cell RNA sequencing technologies and bioinformatics pipelines. Exp Mol Med. 2018;50(8):96.
- Method of the Year 2019: single-cell multimodal omics. Nat Methods. 2020;17(1):1. https://www.nature.com/articles/s41592-019-0703-5
- Marx V. Method of the year: spatially resolved transcriptomics. Nat Methods. 2021;18(1):9–14.
- Altelaar AFM, Munoz J, Heck AJR. Next-generation proteomics: towards an integrative view of proteome dynamics. Nat Rev Genet. 2013;14(1):35–48.
- Timp W, Timp G. Beyond mass spectrometry, the next step in proteomics. Sci Adv. 2020;6(2):eaax8978.
- Gisbert JP, Chaparro M. Clinical usefulness of proteomics in inflammatory bowel disease: a comprehensive review. J Crohns Colitis. 2018;13(3):374–384.
- Aslam B, Basit M, Nisar MA, et al. Proteomics: technologies and their applications. J Chromatogr Sci. 2017;55(2):182–196.
- Magdeldin S, Enany S, Yoshida Y, et al. Basics and recent advances of two dimensional- polyacrylamide gel electrophoresis. Clin Proteom. 2014;11(1):16.
- Issa Isaac N, Philippe D, Nicholas A, et al. Metaproteomics of the human gut microbiota: challenges and contributions to other OMICS. Clin Mass Spectrom. 2019;14:18–30.
- Vernocchi P, Del Chierico F, Putignani L. Gut microbiota profiling: metabolomics based approach to unravel compounds affecting human health. Front Microbiol. 2016;7:1144.
- Contrepois K, Jiang L, Snyder M. Optimized analytical procedures for the untargeted metabolomic profiling of human urine and plasma by combining hydrophilic interaction (HILIC) and reverse-phase liquid chromatography (RPLC)-mass spectrometry. Mol Cell Proteomics. 2015;14(6):1684–1695.
- Emwas AH, Roy R, McKay RT, et al. NMR spectroscopy for metabolomics research. Metabolites. 2019;9(7):123.
- Del Chierico F, Nobili V, Vernocchi P, et al. Gut microbiota profiling of pediatric nonalcoholic fatty liver disease and obese patients unveiled by an integrated meta-omics-based approach. Hepatology. 2017;65(2):451–464.
- Mirza AH, Berthelsen CH, Seemann SE, et al. Transcriptomic landscape of lncRNAs in inflammatory bowel disease. Genome Med. 2015;7(1):39.
- Berrington JE, Hearn RI, Bythell M, et al. Deaths in preterm infants: changing pathology over 2 decades. J Pediatr. 2012;160(1):49–53.e1.
- Neu J, Walker WA. Necrotizing enterocolitis. N Engl J Med. 2011;364(3):255–264.
- Allin BSR, Long A-M, Gupta A, et al. One-year outcomes following surgery for necrotising enterocolitis: a UK-wide cohort study. Arch Dis Child Fetal Neonatal Ed. 2018;103(5):F461–F466.
- Hackam DJ, Sodhi CP, Good M. New insights into necrotizing enterocolitis: from laboratory observation to personalized prevention and treatment. J Pediatr Surg. 2019;54(3):398–404.
- Masi AC, Stewart CJ. The role of the preterm intestinal microbiome in sepsis and necrotising enterocolitis. Early Hum Dev. 2019;138:104854.
- Wang Y, Hoenig JD, Malin KJ, et al. 16S rRNA gene-based analysis of fecal microbiota from preterm infants with and without necrotizing enterocolitis. Isme J. 2009;3(8):944–954.
- Warner BB, Deych E, Zhou Y, et al. Gut bacteria dysbiosis and necrotising enterocolitis in very low birthweight infants: a prospective case-control study. Lancet. 2016;387(10031):1928–1936.
- Morrow AL, Lagomarcino AJ, Schibler KR, et al. Early microbial and metabolomic signatures predict later onset of necrotizing enterocolitis in preterm infants. Microbiome. 2013;1(1):13.
- Stewart CJ, Embleton ND, Marrs ECL, et al. Longitudinal development of the gut microbiome and metabolome in preterm neonates with late onset sepsis and healthy controls. Microbiome. 2017;5(1):75.
- Shaw AG, Sim K, Randell P, et al. Late-onset bloodstream infection and perturbed maturation of the gastrointestinal microbiota in premature infants. PLoS One. 2015;10(7):e0132923.
- Mai V, Torrazza RM, Ukhanova M, et al. Distortions in development of intestinal microbiota associated with late onset sepsis in preterm infants. PLoS One. 2013;8(1):e52876.
- Taft DH, Ambalavanan N, Schibler KR, et al. Center variation in intestinal microbiota prior to late-onset sepsis in preterm infants. PLoS One. 2015;10(6):e0130604.
- Smith B, Bode S, Petersen BL, et al. Community analysis of bacteria colonizing intestinal tissue of neonates with necrotizing enterocolitis. BMC Microbiol. 2011;11(1):73.
- Stewart CJ, Fatemizadeh R, Parsons P, et al. Using formalin fixed paraffin embedded tissue to characterize the preterm gut microbiota in necrotising enterocolitis and spontaneous isolated perforation using marginal and diseased tissue. BMC Microbiol. 2019;19(1):52.
- Sim K, Shaw AG, Randell P, et al. Dysbiosis anticipating necrotizing enterocolitis in very premature infants. Clin Infect Dis. 2015;60(3):389–397.
- Olm MR, Bhattacharya N, Crits-Christoph A, et al., Necrotizing enterocolitis is preceded by increased gut bacterial replication, Klebsiella, and fimbriae-encoding bacteria. Sci Adv. 5(12): eaax5727. 2019.
- Wandro S, Osborne S, Enriquez C, et al. The microbiome and metabolome of preterm infant stool are personalized and not driven by health outcomes, including necrotizing enterocolitis and late-onset sepsis. mSphere. 2018;3(3). DOI:10.1128/mSphere.00104-18.
- Thomaidou A, Chatziioannou AC, Deda O, et al. A pilot case-control study of urine metabolomics in preterm neonates with necrotizing enterocolitis. J Chromatogr B Analyt Technol Biomed Life Sci. 2019;1117:10–21.
- Wilcock A, Begley P, Stevens A, et al. The metabolomics of necrotising enterocolitis in preterm babies: an exploratory study. J Matern Fetal Neonatal Med. 2016;29(5):758–762.
- Ng PC, Ang IL, Chiu RW, et al. Host-response biomarkers for diagnosis of late-onset septicemia and necrotizing enterocolitis in preterm infants. J Clin Invest. 2010;120(8):2989–3000.
- Chatziioannou AC, Wolters JC, Sarafidis K, et al. Targeted LC-MS/MS for the evaluation of proteomics biomarkers in the blood of neonates with necrotizing enterocolitis and late-onset sepsis. Anal Bioanal Chem. 2018;410(27):7163–7175.
- Maheshwari A, Schelonka RL, Dimmitt RA, et al. Cytokines associated with necrotizing enterocolitis in extremely-low-birth-weight infants. Pediatr Res. 2014;76(1):100–108.
- Pang Y, Du X, Xu X, et al. Impairment of regulatory T cells in patients with neonatal necrotizing enterocolitis. Int Immunopharmacol. 2018;63:19–25.
- Weitkamp J-H, Koyama T, Rock MT, et al. Necrotising enterocolitis is characterised by disrupted immune regulation and diminished mucosal regulatory (FOXP3)/effector (CD4, CD8) T cell ratios. Gut. 2013;62(1):73–82.
- Chan KY, Leung KT, Tam YH, et al. Genome-wide expression profiles of necrotizing enterocolitis versus spontaneous intestinal perforation in human intestinal tissues: dysregulation of functional pathways. Ann Surg. 2014;260(6):1128–1137.
- Tremblay É, Thibault M-P, Ferretti E, et al. Gene expression profiling in necrotizing enterocolitis reveals pathways common to those reported in Crohn’s disease. BMC Med Genomics. 2016;9(1):6.
- Tremblay É, Ferretti E, Babakissa C, et al. IL-17-related signature genes linked to human necrotizing enterocolitis. BMC Res Notes. 2021;14(1):82.
- Granger CL, Embleton ND, Palmer JM, et al. Maternal breastmilk, infant gut microbiome and the impact on preterm infant health. Acta Paediatr. 2021;110(2):450–457.
- Autran CA, Kellman BP, Kim JH, et al. Human milk oligosaccharide composition predicts risk of necrotising enterocolitis in preterm infants. Gut. 2018;67(6):1064–1070.
- Masi AC, Embleton ND, Lamb CA, et al. Human milk oligosaccharide DSLNT and gut microbiome in preterm infants predicts necrotising enterocolitis. Gut. 2020:gutjnl-2020-322771. DOI:10.1136/gutjnl-2020-322771.
- Glassner KL, Abraham BP, Quigley EMM. The microbiome and inflammatory bowel disease. J Allergy Clin Immunol. 2020;145(1):16–27.
- Cococcioni L, Panelli S, Varotto-Boccazzi I, et al. IBDs and the pediatric age: their peculiarities and the involvement of the microbiota. Dig Liver Dis. 2021;53(1):17–25.
- Haberman Y, Karns R, Dexheimer PJ, et al., Ulcerative colitis mucosal transcriptomes reveal mitochondriopathy and personalized mechanisms underlying disease severity and treatment response. Nat Commun. 10(1): 38. 2019.
- Hansen R, Russell RK, Reiff C, et al. Microbiota of de-novo pediatric IBD: increased Faecalibacterium prausnitzii and reduced bacterial diversity in Crohn’s but not in ulcerative colitis. Am J Gastroenterol. 2012;107(12):1913–1922.
- Gevers D, Kugathasan S, Denson Lee A, et al. The treatment-naive microbiome in new-onset Crohn’s disease. Cell Host Microbe. 2014;15(3):382–392.
- Mottawea W, Chiang C-K, Mühlbauer M, et al. Altered intestinal microbiota–host mitochondria crosstalk in new onset Crohn’s disease. Nat Commun. 2016;7(1):13419.
- Lewis JD, Chen EZ, Baldassano RN, et al. Inflammation, antibiotics, and diet as environmental stressors of the gut microbiome in pediatric Crohn’s disease. Cell Host Microbe. 2015;18(4):489–500.
- Jacobs JP, Goudarzi M, Singh N, et al. A disease-associated microbial and metabolomics state in relatives of pediatric inflammatory bowel disease patients. Cell Mol Gastroenterol Hepatol. 2016;2(6):750–766.
- Chehoud C, Albenberg LG, Judge C, et al. Fungal signature in the gut microbiota of pediatric patients with inflammatory bowel disease. Inflamm Bowel Dis. 2015;21(8):1948–1956.
- Assa A, Butcher J, Li J, et al. Mucosa-associated ileal microbiota in new-onset pediatric Crohn’s disease. Inflamm Bowel Dis. 2016;22(7):1533–1539.
- Zhang X, Deeke SA, Ning Z, et al. Metaproteomics reveals associations between microbiome and intestinal extracellular vesicle proteins in pediatric inflammatory bowel disease. Nat Commun. 2018;9(1):2873.
- Schirmer M, Denson L, Vlamakis H, et al. Compositional and temporal changes in the gut microbiome of pediatric ulcerative colitis patients are linked to disease course. Cell Host Microbe. 2018;24(4):600–610.e4.
- Wang Y, Gao X, Zhang X, et al. Microbial and metabolic features associated with outcome of infliximab therapy in pediatric Crohn’s disease. Gut Microbes. 2021;13(1):1–18.
- Cortez RV, Moreira LN, Padilha M, et al. Gut microbiome of children and adolescents with primary sclerosing cholangitis in association with ulcerative colitis. Front Immunol. 2021;11:598152.
- Kolho K-L, Pessia A, Jaakkola T, et al. Faecal and serum metabolomics in paediatric inflammatory bowel disease. J Crohns Colitis. 2016;11(3):321–334.
- Martin F-P, Ezri J, Cominetti O, et al. Urinary metabolic phenotyping reveals differences in the metabolic status of healthy and inflammatory bowel disease (IBD) children in relation to growth and disease activity. Int J Mol Sci. 2016;17(8):1310.
- Martin F-P, Su -M-M, Xie G-X, et al. Urinary metabolic insights into host-gut microbial interactions in healthy and IBD children. World J Gastroenterol. 2017;23(20):3643–3654.
- Deeke SA, Starr AE, Ning Z, et al. Mucosal-luminal interface proteomics reveals biomarkers of pediatric inflammatory bowel disease-associated colitis. Am J Gastroenterol. 2018;113(5):713–724.
- Starr AE, Deeke SA, Ning Z, et al. Proteomic analysis of ascending colon biopsies from a paediatric inflammatory bowel disease inception cohort identifies protein biomarkers that differentiate Crohn’s disease from UC. Gut. 2017;66(9):1573–1583.
- Haberman Y, Tickle TL, Dexheimer PJ, et al., Pediatric Crohn disease patients exhibit specific ileal transcriptome and microbiome signature. J Clin Invest. 124(8): 3617–3633. 2014.
- Ta AD, Ollberding NJ, Karns R, et al. Association of baseline luminal narrowing with ileal microbial shifts and gene expression programs and subsequent transmural healing in pediatric Crohn disease. Inflamm Bowel Dis. 2021;16:izaa339.
- Griffin BH, Chitty LS, Bitner-Glindzicz M. The 100 000 genomes project: what it means for paediatrics. Arch Dis Child Educ Pract Ed. 2017;102(2):105–107.
- Casals-Pascual C, González A, Vázquez-Baeza Y, et al. Microbial diversity in clinical microbiome studies: sample size and statistical power considerations. Gastroenterology. 2020;158(6):1524–1528.