ABSTRACT
Introduction
Knee osteoarthritis (OA) is a joint disease, affecting multiple tissues in the joint. Early detection and intervention may delay OA development and avoid total knee arthroplasty. Specific biomarker profiles for early detection and guiding clinical decision-making of OA have not yet been identified. One technique that can contribute to the finding of this ‘OA biomarker’ is mass spectrometry (MS), which offers the possibility to analyze different molecules in tissues or fluids. Several proteomic, lipidomic, metabolomic and other – omic approaches aim to identify these molecular profiles; however, variation in methods and techniques complicate the finding of promising candidate biomarkers.
Areas covered
In this systematic review, we aim to provide an overview of molecules in knee OA patients. Possible biomarkers in several tissue types of OA and non-OA patients, as well as current limitations and possible future suggestions will be discussed.
Expert opinion
According to this review, we do not believe one specific biomarker will function as predictive molecule for OA. Likely, a group of molecules will give insight in OA development and possible therapeutic targets. For clinical implementation of MS-analysis in clinical decision-making, standardized procedures, large cohort studies and sharing protocols and data is necessary.
1. Introduction
Knee osteoarthritis (OA) is a total joint disease, characterized by cartilage degeneration, thickening and inflammation of the synovium and osteophyte formation, leading to pain and immobility among patients [Citation1]. The incidence of knee OA in the human population has been increasing over the past decades and is expected to increase even further [Citation2]. Not only the increasing elderly population, but also the younger population is confronted with OA more frequently [Citation3]. Risk factors such as osteochondral trauma/injury and obesity are identified [Citation1–3].
Current treatment options for OA focus on diet and moderate physical activity, as well as the reduction of pain and inflammation with non-steroidal anti-inflammatory drugs (NSAIDs) [Citation4]. OA affects multiple, if not all, tissues inside the joint. Its features make it hard to find targeted therapies, leaving total knee arthroplasty (TKA) as inescapable treatment option. Early detection and intervention have been shown to postpone rigorous treatment [Citation5]. This makes it even more important to find biomarkers or molecular factors that contribute to OA development and disease progression, not only to gain insight in disease pathogenesis, but also to guide clinical decision-making and treatment discovery.
Treatments that prevent OA progression or OA from developing are lacking. The development of disease modifying OA drugs (DMOADs) requires knowledge on the structure and homeostasis of the joint [Citation6]. This information can for example be obtained from molecular imaging analysis and might contribute to the development of personalized treatments.
OA has been described as a total joint disease, implicating that all tissues inside the joint are or can be affected [Citation7] and is often the result of trauma or injury. OA is not limited to the knee. It can appear in all joints of the human body. However, knee OA is the most prevalent [Citation2]. In addition, most research is done on knee OA, as waste tissue from the knee after replacement surgery is usually available.
The human knee consists of different tissue types and fluids. Both femur and tibia, as well as the patella, are covered with a layer of hyaline cartilage, supporting subtle movement of the knee. Between these layers of cartilage, a thin layer of synovial fluid is lubricating the cartilage surface for smooth movement. Several ligaments, such as the anterior cruciate ligament (ACL), limit these movements, giving the knee its stability. To prevent bone-to-bone friction and absorb some of the impact on the knee, the menisci are located between the edges of the cartilage layers. All these tissues are surrounded by a layer of synovial membrane, the knee capsule. Finally, yet importantly, underneath the patellar ligament, Hoffa’s fat pad (HFP) or the infrapatellar fat pad is located (). HFP has been proven an important metabolic organ, involved in OA’s inflammatory response [Citation8,Citation9]. In the OA knee, the cartilage is damaged, synovium thickened and inflamed, and there is more synovial fluid present ().
Figure 1. Frontal (left) and parasagittal (middle) view of the knee’s anatomy, as well as a visualization of the osteoarthritic knee (right) with osteoarthritic features such as synovial thickening, cartilage degradation and development of bone spurs. The bones in the human knee are covered by a layer of hyaline cartilage. This cartilage, in combination with the menisci and a layer of lubricating synovial fluid, facilitate the movement of the knee. Other tissues such as synovium and Hoffa’s fat pad are there to maintain joint homeostasis. Image was modified with permission from Servier Medical Art, licensed under a Creative Common Attribution 3.0 Generic License. http://smart.servier.com/
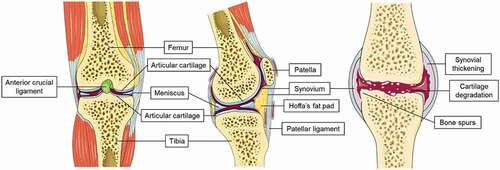
From all techniques employed for biomarker discovery, mass spectrometry (MS) offers the possibility of analyzing thousands of different molecules in a single experiment. Other methods such as microarrays, for DNA, RNA or protein analysis, can also measure thousands of different molecules simultaneously, however this is a more targeted approach compared to most of MS studies. It has been evolving as technique to detect (and locate) possible biomarkers for OA by measurement of the mass to charge ratio of the detected molecules. Proteomic, lipidomic, metabolomic and other – omic approaches aim to identify molecular profiles or signatures of different tissues (cartilage, bone, synovium, meniscus, tendon, HFP) or liquids (synovial fluid) inside the OA knee joint, hoping to find certain predictive molecules or molecular classes responsible for OA development, disease progression or possible therapeutic targets. However, differences in MS approaches, targeted molecular classes and tissue sources make it difficult to appreciate which method and molecules are the most promising candidates as OA biomarkers.
Due to the plethora of available techniques, as well as target compounds, we aimed to look for common molecular signatures that could be used for future research, disease prognosis or treatment. Therefore, in this systematic review, we aim to provide an overview of molecules in the knee that distinguish between (several degrees of) OA and non-OA patients. Possible biomarkers for knee OA, current limitations and possible future suggestions will be discussed.
2. Body
2.1. Literature search and article selection
The literature search and article selection was conducted following the Preferred Reporting Items for Systematic Reviews and Meta-Analysis (PRISMA) [Citation10]. The PubMed database was searched for eligible articles on 28 January 2021. The search included topics on OA, MS, proteomics, lipidomics and metabolomic approaches, including underlying terms (MesH terms) and terms in title and abstract (tiab) (Supplementary Table 1). Two blinded researchers (MH and KE), individually evaluated all records using ‘Rayyan QCRI’ [Citation11]. Conflicts were solved by a third researcher (BCP) after discussion.
Table 1. Data extraction and study characteristics of selected articles: proteins analyzed in synovial fluid, cartilage, synovium or meniscus, sorted on tissue type and mass spectrometry method
Studies were included if the article covered 1) the use of samples directly derived from patients with knee OA, 2) the analysis of local molecular markers in intra-articular fluid or tissue (including HFP) of the knee, 3) a comparison of patients with different stages of OA, 4) a comparison of OA patients with either healthy subjects or patients with other knee injuries and 5) the use of untargeted MS analysis. Exclusion criteria included 1) abstracts only and reviews, 2) the use of non-local fluids like blood/serum or urine, 3) lack of a non-OA control group, 4) tissue and cell culture, 5) full text not in English and 6) lack of reporting of molecular differences between groups.
All data on use of subjects, tissue collection, tissue type, methodological approach, and molecular class of interest, as well as used databases for identification were extracted from the articles of interest (). In this review, we focused on, already hard to find, local markers in the knee, rather than markers in serum, plasma or blood because we believe it is important to unravel the local changes of OA before translating it to systemic changes. In addition, with the exclusion of systemic markers, we prevent possible co-factors of other diseases to interfere with the results.
Table 2. Data extraction and study characteristics of selected articles analyzing metabolites and lipids in synovial fluid and cartilage
2.2. PRISMA results and data extraction
From our search in PubMed, 701 records were identified and screened based on abstract according to the mentioned inclusion and exclusion criteria. From these, 636 records were excluded, whereas 64 records were assessed for eligibility based on full text. In total, 32 and 24 studies were included in the qualitative synthesis and quantitative synthesis (pathway analysis) respectively ().
Figure 2. Prisma flow diagram, screened abstracts and articles. A total of 32 and 24 articles were used for qualitative and quantitative analysis respectively in this systematic review
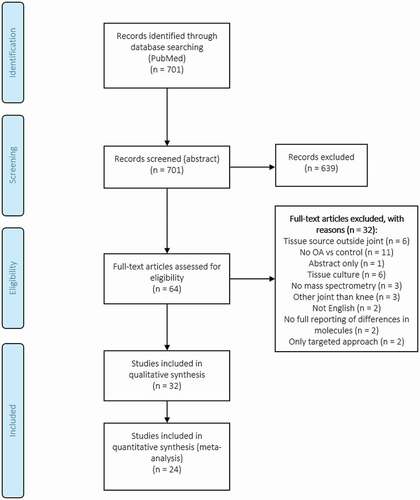
The topics of interest ‘first author name,’ ‘number of subjects,’ ‘sample type,’ ‘molecule of interest,’ ‘MS method’ and ‘identification database’ were extracted from each article and sorted based on molecular class ( for proteins and for lipids and metabolites). Subjects were classified as non-OA (postmortem) healthy, trauma/amputation, knee pain, (sub-) acute ACL, meniscus injury), early OA (eOA, Ahlbäck grades 1 and 2, Kellgren-Lawrence (KL) 1 and 2), late OA (lOA, Ahlbäck grades 3, 4 and 5, KL 3 and 4) and OA without classification.
In addition, we used the data retrieved from the reviewed articles to conduct a joint pathway analysis (Homo Sapiens (human), integrated metabolic and gene pathways) on all upregulated-highlighted proteins (UniProt Protein ID, n = 35), lipids and metabolites (compound name, n = 13) (Supplementary tables 2 and 3) in OA, using the web tool MetaboAnalyst 5.0 [Citation34] to see which pathways, rather than single molecules, are involved in OA pathophysiology. The pathway analysis was conducted using a human (Homo sapiens) database (KEGG), including metabolic and gene pathways. Additionally, for enrichment analysis, topology measure and integration method, a hypergeometric test, degree centrality and combined queries were used respectively.
Table 3. Pathway analysis in MetaboAnalyst 5.0 using all upregulated-highlighted proteins (n = 35), lipids and metabolites (n = 13) in OA patients, found in the article search of this review. Pathways with high impact (impact > 0.1) are highlighted in bold. These pathways include complement and coagulation cascades, extracellular matrix receptor interaction and multiple inflammatory pathways
2.3. Proteins in osteoarthritis
Twenty-three articles included the terms protein and ‘biomarkers.’ Of these, 13 focused on proteins in synovial fluid, 5 in cartilage, 2 in synovium and 2 in meniscus. All authors compared proteins in a non-OA group to either early OA, late OA or OA in general, except for Ahmed et al. (2016) and Corigliano et al. (2017) who only compared early OA vs. late OA ().
Liquid chromatography tandem MS LCMS/MS coupled to electrospray ionization (ESI), was the predominant MS technique used for the detection of peptides and proteins in these samples. To a lesser extent, matrix-assisted laser desorption/ionization MS (imaging) (MALDI-MS(I)) was used to analyze proteins, especially in tissues like cartilage and synovium. MALDI-MSI allows us to, not only detect molecules inside the tissue, but also to determine the spatial distribution of these molecules throughout the tissue. This makes MALDI-MSI a significant tool to analyze tissues that consist of multiple structures, layers or cells, such as cartilage, and understand intra-tissue remodeling [Citation35,Citation36].
Supplementary Table 2 summarizes the proteins that were highlighted in two or more studies. Of these, 35 proteins were upregulated in multiple tissue types and/or fluids of OA patients (Supplementary Table 2 and ). Interestingly, fibronectin was found to be upregulated in all measured tissues/fluids (synovial fluid, synovium, meniscus and cartilage) of OA patients (). Only one protein, transaldolase, was downregulated in the synovial fluid of OA patients (measured by at least two studies, Supplementary Table 2). In addition, histone H2B and serum amyloid A1 (isoform 1) were upregulated in control patients (Supplementary Table 2). Many others suggest important proteins, such as annexin A2, apolipoprotein A-1 (precursor), cartilage oligomeric matrix protein (COMP), versican core protein and collagen α1(II) isoforms but showed contradictive results (variations in up- or down-regulation of proteins) between articles (Supplementary Table 2).
Figure 3. Upregulated proteins in cartilage, synovial fluid, synovium and meniscus of osteoarthritis patients, identified by at least two authors, without contradiction. Recurring proteins measured in at least three tissue types are highlighted in bold. Image was modified with permission from Servier Medical Art, licensed under a Creative Common Attribution 3.0 Generic License. http://smart.servier.com/
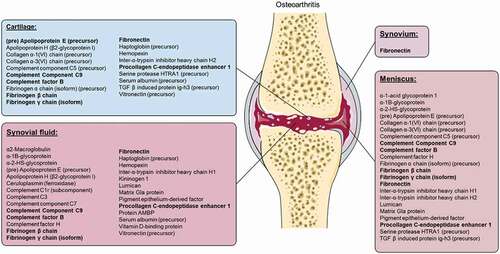
3.4. Small molecules in osteoarthritis: metabolites and lipids
Compared to proteins, the number of studies on small molecules like lipids or metabolites is limited. Five articles that focused on the detection and identification of metabolites in OA vs healthy/non-OA patients and five articles that focused on lipid species were found ().
All metabolite studies analyzed synovial fluid, collected from healthy patients (postmortem or during amputation) and patients with (several degrees of) knee OA (either during treatment or postmortem). The number of samples used varied from 5–21 in the healthy groups and 5–69 in the OA groups (early OA and late OA). All five articles used either untargeted LC-MS or gas chromatography MS (GC-MS) methods for the detection of metabolites in these synovial fluid samples (). The use of standard operating procedures, or SOPs, is clearly important in these type of studies for method standardization and inter or intra-laboratory reproducibility. In addition to these methods, Mickiewicz et al. added a targeted 1 H nuclear magnetic resonance (1 H NMR) spectroscopy approach to their analysis method for an NMR/GC-MS combined dataset to develop a prediction model of OA [Citation37]. All studies were able to distinguish healthy subjects from those with OA using principal component analysis (PCA) and/or orthogonal partial least squares discriminant analysis (OPLS-DA). Interestingly, two phenotypes each for early OA and late OA were identified by Carlson et al. [Citation38].
Although synovial fluid is found to be the preferred source in the search for lipid biomarkers, Cillero-Pastor et al. [Citation39] were the only authors that analyzed cartilage samples, as well as the spatial distribution of molecules. Different number of patient samples with different age ranges were used for lipidomic analysis in each article: 3–16 healthy donors (postmortem), 17–26 early OA patients (during arthroscopy) and/or 3–22 late OA patients (during TKA). Lipid species were either detected with the use of time-of-flight secondary ion MSI (TOF-SIMS), high-performance LC-MS (HPLC-MS) or electrospray ionization tandem MS (ESI-MS/MS) and GC-MS ().
The use of multiple biomarkers, rather than one, increases the power of discriminating OA from non-OA patients. The authors suggested multiple metabolites or lipids in the synovial fluid (or cartilage) of OA patients as possible biomarkers for OA (Supplementary Table 3 and ). Several amino acids, amines, sugar (alcohols), organic acids, (mono-un) saturated and omega-6) fatty acids), phosphates, (lyso)phospholipid(s) (classes), sphingomyelins and ceramides, among others, were shown to be different between early OA and/or late OA patients and controls (Supplementary Table 3). Remarkably, all molecules found to be upregulated in cartilage of OA patients, were also upregulated in the synovial fluid of OA patients, except for the group fatty acids ().
Figure 4. Upregulated metabolites and lipids in cartilage and synovial fluid osteoarthritis patients, identified by at least two authors, without contradiction. Lipid or metabolite species that were found in cartilage and synovium are highlighted in bold. Image was modified with permission from Servier Medical Art, licensed under a Creative Common Attribution 3.0 Generic License. http://smart.servier.com/
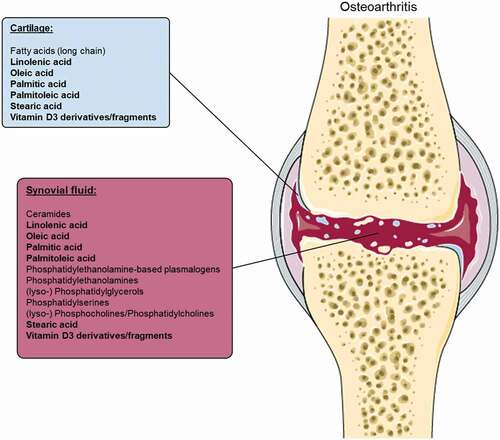
2.5. Osteoarthritis phenotyping
Several OA phenotypes have been described in literature: among others, the senescent phenotype, inflammatory phenotype, metabolic phenotype, genetic phenotype and endocrine phenotype [Citation40,Citation41]. These phenotypes might be important when developing personalized OA treatments. For example, synovitis can be treated with anti-inflammatory drugs [Citation40] or reducing mechanical loading in a mechanical overload phenotype [Citation41]. Also at the molecular level, different OA phenotypes can be identified. For example, Eveque-Mourroux et al. found differences in lipid and protein profiles in patients with or without Type 2 Diabetes Mellitus (T2DM) [Citation36]. Briefly, phospholipase A2 and lysolipids were in general associated to the OA group with T2DM whereas phosphatidylcholine and sphingomyelin species were associated to the group of OA patients without T2DM [Citation36].
Interestingly, arachidonic acid, ethanolamine and malate were decreased in the OA group when compared to non-OA patients [Citation37,Citation42], but increased in the late OA group when compared to early OA [Citation43]. In addition, Kim et al. found differences in metabolites between patients with OA KL grade 1, 2, 3 and 4 specifically [Citation43]. Levels of sugar (alcohols) such as arabitol, galactose, glucose, mannose and tagatose were increased in KL grade 1 compared to other stages. Urate, β-alanine, pyruvate, and terephthalate levels were increased in KL grade 2 patients and fatty acids, proline, phenylalanine, squalene, and trehalose-6-phosphate were increased in KL grade 3 and 4 [Citation43].
Several metabolisms and metabolic pathways were found to be altered in (different stages of) OA: amino acid metabolism, central energy metabolism (gluconeogenesis, glycolysis and citric acid cycle), extracellular matrix component metabolism (including ascorbate metabolism, galactosamine and glucosamine biosynthesis, keratin sulfate metabolism and N-glycan metabolism), fatty acid (biosynthesis) and lipid metabolism (carnitine shuttle, glycerol(phospho)lipid and glycosphingolipid metabolism), inflammation (leukotriene metabolism), oxidative stress (glutathione and vitamin E metabolism) and vitamin C, E, B1, B3, B6, B9 metabolism. In addition, Carlson et al. [Citation38] also found two different phenotypes (inflammatory and structural deterioration) associated to early and late OA groups.
2.6. Joint pathway analysis in MetaboAnalyst 5.0
Twenty-three pathways were significantly upregulated (p < 0.05) in OA patients according to our analysis. These included complement and coagulation cascades (highest significance) and inflammatory pathways among others ( and ). When comparing these pathways to the pathways described in the articles of this review, there were some similarities. OA was associated with altered extracellular matrix (ECM) component metabolism, lipid and fatty acid metabolism, vitamin metabolism, inflammation and complement and coagulation pathways among many others [Citation38,Citation44–46].
Figure 5. The Pathway Enrichment vs. -log10 (p) with p < 0.05 cutoff for proteins, lipids and metabolites in osteoarthritis patients. P-values are subdivided on a white to red color scheme, red indicating higher significance (P < 0.05). Circle size is determined based on Enrichment Ratio (ER), with small circles representing low ER and big circles representing high ER. ECM = extracellular matrix
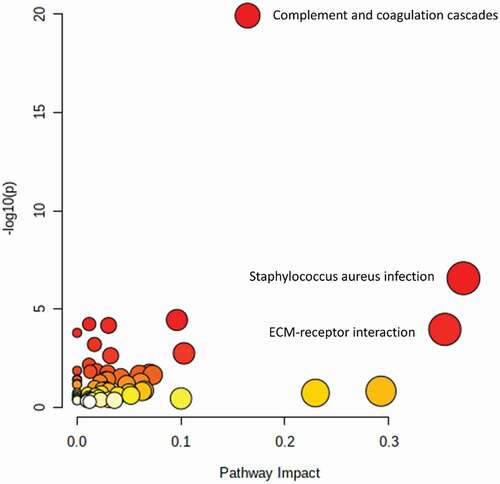
describes all significant (P < 0.05) pathways involved with the highlighted upregulated proteins, lipids and metabolites in OA. Complement and coagulation cascades, ECM-receptor interaction and multiple inflammatory pathways were associated with OA (). In addition, false-discovery rate (FDR) and impact are described ().
In summary, our results describe a variety of possible biomarkers for OA. Multiple patient groups, tissues and analysis methods were described by the authors of included articles. These differences will be elaborated and discussed below.
3. Discussion: Inter-patient variability, tissue types and molecules
3.1. Inter-patient variability
Healthy patient material as control is crucial in the search for potential OA biomarkers. However, healthy ‘living’ material is either not usually available or material is collected postmortem, which might influence analysis results due to the occurrence of degradation products (some authors correct for this). Postmortem samples are suggested as a valid alternative for healthy material, if tissue sampling occurs within foreseeable time and researchers correct for tissue degradation. In the included papers in this review, several non-OA patient groups (trauma, knee pain and ACL or meniscus injury) were used as control group. Some articles did not use a non-OA control, but distinguished between early OA and late OA patients. In addition, the willingness of healthy individuals to undergo synovial fluid, or intra-articular tissue sampling can be an issue as it is rather invasive.
Control groups often consist of a limited sample size (n = 1 or n = 3). Controversially, the OA group is often of bigger magnitude as collection of rest material during surgery occurs more often. The results of this review emphasize the importance of OA grade, as several lipid or metabolite biomarkers showed contradictive results when OA grade is considered a distinguishing factor.
It is problematic that most control groups have a traumatic incident in their joint, as this may induce inflammatory or OA-like features [Citation47]. This might also influence the results, as these joint defects often result in the development of OA when not treated sufficiently. Therefore, the results of this review should be interpreted as markers that are different in a chronic inflammatory and degenerative state (OA) compared to an acute inflammatory state due to a traumatic event. True healthy control tissue is necessary to confirm that these differences are also representative to distinguish OA from non-affected tissue. As ethical consideration may prohibit collection of such tissue from a general population, possible sources may be during healthy knee allograft collection for ankle defects, surgery of bony structures where the knee is unaffected or contra-lateral biopsy during TKA.
3.2. Tissue types and molecules
Synovial fluid is the most frequently used biopsy source for OA biomarkers. It is easily accessible (in most cases), can be harvested from non-OA patients (postmortem or alive) and can be measured by one of the most used and sensitive MS techniques: LC-MS. Synovial fluid, if not contaminated with blood, is easy to process and requires limited actions before analysis. Authors often use 2D gel electrophoresis (2DE) and/or sodium dodecyl sulfate polyacrylamide gel electrophoresis (SDS-PAGE) to separate proteins before LC-MS analysis to measure proteins in synovial fluid.
Synovial fluid can be aspirated from the joint using a syringe prior to or during surgery. This technique is already been used in clinic to reduce swelling or infection, as well as for diagnostic purposes [Citation48]. Aspiration of synovial fluid is an invasive technique, puncturing the knee capsule, which often needs echography guidance. The OA knee often contains more synovial fluid compared to the healthy knee. To detect biomarkers for OA and be able to intervene in its development, synovial fluid should be aspirated early in the disease. However, patients with early stages of OA often do not experience symptoms or seek medical help.
Cartilage is the second most-explored tissue of the human knee and is often harvested postmortem or during TKA. In severe cases of OA, the cartilage is degraded to the bone, making it hard to harvest late-stage OA cartilage. The use of cartilage and other tissue types in combination with LC-MS requires protein extraction from the tissue. Other techniques like MALDI-MS(I) are favored for these ‘solid’ tissue types, as it gives researchers the opportunity to visualize the location of the detected molecule by spatial analysis. Healthy cartilage is even more difficult to obtain. Harvest of healthy human cartilage is only possible postmortem or after trauma. Therefore, most studies use damaged tissues from the knee as control. These tissues are often removed or replaced during surgery and are therefore more likely to acquire.
While we already know that ACL and/or meniscus rupture contributes to the development of OA in the future [Citation49,Citation50], the use of ACL and/or meniscus tissue in the search for biomarkers with MS is limited or absent. Also limited/absent is the search for biomarkers in synovium and HFP, though both tissue types play an important role in the inflammatory and molecular signature of OA [Citation51,Citation52]. In addition, bone, or the bone-cartilage interface, and tendon (injury) are in this search not even suggested as tissue of interest, while these tissues might also be contributing to inflammation or OA development [Citation53–55].
Most articles in our search focus on the analysis of proteins. Proteins fulfill multiple functions in the human body, such as tissue growth, maintenance, and cell/tissue communication. Dysregulation of protein synthesis or expression is often associated with disease pathology [Citation56]. Therefore, it is not surprising that proteins are predominantly used as diagnostic or therapeutic target for OA. Proteins are known to interact with other proteins, but also with lipids in cell membranes [Citation57].
Lipids and metabolites are less studied in disease pathologies such as OA. For example, lipids have been shown to play an important role in the pro-inflammatory phenotype of OA [Citation58]. This suggests that these molecules might also function as diagnostic or therapeutic targets for OA and that more research needs to be done. An important feature of the reviewed articles focusing on lipids is that they often provide sum compositions, whereas possible different lipid isomers might also play different signaling roles in OA.
4. Discussion: Molecular signature dependent on sample preparation and mass spectrometry technique
4.1. Sample preparation
The techniques used for sample preparation varied among authors and are dependent on the MS technique used for analysis. Not only the MS technique has to be taken into account, but also the purpose of the study (homogenate or local analysis), sample volume, sample concentration or composition, and the preparation method/solution are important [Citation59]. In addition, external factors such as inter-person variabilities, (storage) temperature and humidity can play a roll.
Before analyzing tissues or fluids with MS, it is important to consider enhancing the performance of protein digestion and reduce the complexity of the proteome by using several enzyme (protease) combinations for protein digestion. Proteases such as (chymo)trypsin, or alternative proteases such as endoproteinases glutamic-C, lysine-C, lysine-N or aspartic-N, can generate unique peptide sequences and improve protein sequence coverage in different MS applications [Citation60].
Post-translational modifications of proteins could also be of interest while searching for biomarkers. Top-down proteomics allows the detection of specific post-translational modifications such as phosphorylation, glycosylation, ubiquitination, nitrosylation, methylation or acetylation, and typically involves purification steps. Glycoprotein modifications in the cartilage for instance have been described [Citation61]. In many cases these modifications cannot be detected. Chromatographic separation or previous protein purification are often needed. In addition, protocols that disrupt collagen fibers are recommended since the access to proteins with specific roles and PTMs can be better digested then. For example, Hsueh et al. used a strong guanidine extraction buffer for protein extraction in cartilage [Citation62].
4.2. Mass spectrometry and data analysis
Using currently available MS techniques, it is possible to detect molecules including peptides and proteins, lipids, metabolites and other (bio) chemical compounds. The majority of articles in our search focuses on the detection and analysis of peptides and proteins, rather than lipids or metabolites. Hereby, every ionization method (ESI, MALDI, etc.) has a different effect on sample and final analysis result, as sensitivity and detection method are different. In addition, the mass resolution and accuracy of the instrument are important when comparing data between articles.
A mass spectrometer consists of an ion source for sample ionization, a mass analyzer for mass separation and a detector for ion detection. There are several types of mass analyzers available, which separate ionized masses based on charge to mass ratio (m/z) which can be detected by the detector: quadrupole (ion trap), time of flight (TOF), magnetic or electrostatic double sector, and ion cyclotron resonance mass analyzers [Citation63–65]. Which mass analyzer to use is dependent on the application.
In addition, the ionization source also has an influence on the detected molecules. As could be shown from this review, ESI is mainly used in combination with LC-MS instruments. In contrast to ESI, EI and CI are mainly used in GC-MS techniques. With these ionization techniques, electrons or chemicals are used to ionize or charge molecules in the mass spectrometer [Citation66].
Alternatively, some MS techniques make use of ion-mobility to separate ions based on their interaction with collision gas, which leads to an increased number of identifications.
LC-MS, combined with dedicated bioinformatics strategies, is highly specific and well developed over the past years and has become one of the widely used techniques in MS. LC-MS analysis of tissues and fluids give us a detailed overview on the molecular/protein profiles in OA patients. In addition, most LC-MS analyses focus on the detection and identification of proteins in particularly fluids. More specific for synovial fluid; available from different OA patients (TKA surgery) or healthy phenotypes can be purchased. Other tissue types are less available and need multiple processing steps before LC-MS analysis. Different analytical results might be due to differences in sample preparation or type of database used. Protein databases such as UniProt/SwissProt include a high variety of proteins. Still, there can be differences between databases and protein content, resulting in more or less identifications dependent on the database used.
Other techniques such as MALDI-MS(I), GC-MS and SIMS have shown to be a promising addition to the field of biomarker discovery too. Whereas proteins appear to be very important in the development of OA, more and more is known about other tissue types, as well as other molecules, such as lipids and metabolites and their involvement in OA development [Citation67,Citation68]. What techniques such as MALDI-MSI discriminate from techniques such as LC-MS is the ability to study molecular distributions within the tissue and the associated tissue heterogeneity. Analysis is performed on-tissue directly and only needs minor sample processing by matrix application. This is of particular importance when analyzing for instance very heterogeneous tissues, such as HFP [Citation69].
Several imaging software, such as Lipostar [Citation70] and SCILS are then able to visualize molecules and determine their distribution throughout the tissue. In addition, with these programs, tissue typing, segmentation and classification of molecules is possible. It gives detailed information on tissue types, cells and molecules within the tissue. For example, Cillero-Pastor et al. addresses the importance of the location of molecules within the tissue, as well as the importance to measure molecules in multiple polarities (negative and positive), which is not usually applied throughout the reviewed articles [Citation35]. Considering these techniques, analytical programs and databases are less developed and used, the information gathered might be of high importance, but not given consideration due to lack of molecule identification. For example, an unknown molecule is detected, but not present in one of the databases.
5. Discussion: A molecular signature for osteoarthritis?
The need for biomarkers in the development of DMOADs and personalized treatment is high [Citation6]. Ideally, a treatment for OA would reduce symptoms and modify disease development [Citation6]. However, the progression of OA, cause biomarker fluctuations between phases of high and low disease activity [Citation6]. It is therefore unlikely that a single biomarker will be specific and sensitive enough to detect, predict or monitor the disease, rather than a combination of molecular biomarkers [Citation6].
Although several studies show contradictive results (differences in up- or down-regulation of measured molecules), various proteins, lipids and metabolites were found to play an important role in distinguishing OA patients from control patients. Differences in molecular signature between articles might be due to differences in patients, methods, analysis or interpretation. Several isoforms of collagen, as well as fragments from the complement system are well known factors in OA [Citation71,Citation72]. In addition, some less known factors have been shown to play an important role in OA development. For example, several lipid species (e.g. prostanoids) have been shown to be involved in inflammation [Citation73]. These species might be different in acute patients (e.g. patients suffering from cartilage defect or ACL rupture), rather than patient with a chronic inflammatory profile such as in OA. Remarkably, most candidate biomarkers for OA were upregulated molecules, rather than downregulated molecules. We suspect this is related to increased tissue degradation, tissue remodeling and inflammation in the OA knee joint. Relevant proteins, such as cytokines would be produced and transported to the affected joint.
MS has been shown a promising tool for the detection of molecular signatures responsible for OA development, prognosis or treatment. However, its translation to the (outpatient-) clinic is difficult. The lack of concrete molecular phenotypes, as well as manageability of the MS systems available, makes it still challenging for routine usage in a clinical setting. A clinical setting might for example be the direct analysis of taken biopsies. These biopsies could be obtained during surgery, to guide treatment decision making on the spot (for example damaged cartilage, is the patient able to regenerate?), or in outpatient clinic, to decide on next steps for treatment (for example biopsy of HFP).
Biopsy taking of critical tissues such as cartilage and synovium, as well as its storage in an accessible biobank, would facilitate research in large study cohorts and contribute to our knowledge on OA development. However, it would be very invasive, entering the knee capsule and often lacks a healthy control. One other possibility is the use of HFP. HFP has already been shown to contribute to the inflammatory phenotype in OA and is more easily accessible as it is located outside the knee capsule. Fat tissue is already known to contribute to diseases such as obesity and diabetes. More and more evidence is gathered that this is also true for fat tissue inside the knee joint of OA patients [Citation74].
According to our results, we would suggest a combination of proteins, lipids and metabolites as biomarker profile for OA. These include (pre) apolipoprotein E (precursor), complement component C9, complement component factor B, fibrinogen β chain, fibrinogen γ chain (isoform), fibronectin, procollagen C-endopeptidase enhancer 1, linolenic acid, oleic acid, palmitic acid, palmitoleic acid, stearic acid and vitamin D3 derivatives or fragments. Whereas synovial fluid is the most studied and accessible source for biomarker discovery in OA, future research on the discovered biomarker profile according to this review is needed. Nevertheless, other tissue types such as HOFFA´s fat pad, are also interesting.
6. Conclusion
Early detection of OA is important to predict disease progression and outcome. It also gives the opportunity to intervene and treat early in the disease stage, prolonging the development of OA and TKA. Protein, lipid and metabolite profiles have been shown to differ in OA from control patients and have therefore the potential to be used as (diagnostic) biomarkers. Different usage of patient information, patient material and MS techniques herein, increases the variation in the detection of molecules, complicating the discovery of potential biomarkers for OA, as well as the development of targeted treatment options.
OA is a multifactorial disease, affecting the whole joint. This review shows that a combination of proteins, lipids and metabolites might contribute to a biomarker profile for OA rather than one single biomarker. To identify a biomarker profile for OA, which can be implicated in the clinic, experiments should be conducted on large patient cohorts, with standardized methods, combined with shared databases.
7. Expert opinion
The ultimate goal of the use of diagnostic MS is to find a molecular profile that can be used to predict OA development, early diagnosis, the search for new therapeutic targets, and the development of personalized treatments. Early diagnosis of OA and better clinical decision-making can contribute to more efficient treatments. With the use of joint-preserving therapies, such as lifestyle interventions and weight loss, as well as nutritional and pharmacological interventions, intra-or-extra articular biomechanics can be restored; TKA can be postponed, leading to fewer revisions and more mobility among patients.
In this review, we concluded that MS is able to separate disease states based on the difference in molecular signatures. However, due to the limited size of the studies, using different techniques, targets and sources, inconsistency of results is inevitable. To be able to translate these preclinical results toward a clinical application, results should be validated and confirmed by different labs.
In addition, the use of alternative tissues such as HFP (highly available because it is routinely removed with every knee-related surgery and biopsy taking would be minimally invasive), might be more applicable for a biomarker profile for OA. Further analysis on multiple tissue types of patients with multiple disease states, might contribute to the finding of a molecular (biomarker) profile for early OA detection, as well as OA development and progression. This might also contribute to the development of personalized therapeutic strategies and molecular targets.
However, this review highlighted many obstacles on the way to these well-needed clinical goals. Almost all included papers studied the difference between OA and control. However, in clinical practice, the need for predictive or phenotyping molecular profiles is just as large as for diagnostic profiling. To truly aid clinical decision-making, the MS analysis should provide the clinician with information on the prognosis of disease or how well a patient would respond to a certain treatment. Only then, it can contribute to personalized medicine. As it is well known from in vitro studies that the chondrocytes in the cartilage are very responsive to e.g. inflammatory signaling molecules [Citation75], the homeostasis of the joint can be predictive of recovery or degeneration of the cartilage. If analysis of an upregulated pathway is shown, such as the complement and coagulation pathway or several inflammatory pathways, there could be an indication for anti-inflammatory treatment.
7.1. Recommendations for biomarker discovery with mass spectrometry
With the heterogeneity of the different approaches, as highlighted in this review, we are still a long way from validating the clinical value of molecular markers. We would like to propose several recommendations to the field:
First, experiments for biomarker analysis should be conducted on large cohorts, including patients with early and late OA, but also patients with cartilage defects. To gain more insight in OA progression after treatment or intervention, it would be optimal if these patients were followed over time using questionnaires and magnetic resonance imaging (MRI).
Secondly, one of the striking features of this review is that each article uses different protocols and methods. Standardization of protocols for biopsy taking, sample preparation and MS analysis is necessary to combine and compare datasets of biomarkers. In addition, it is not clear for all reviewed articles what exact method was used for molecule ionization or identification. MS/MS analysis is necessary for proper identification of measured molecules. Authors likely assume that the reader already knows what ionization or identification method they have used. The use of standardized procedures for biopsy taking (of multiple tissue types) and biomarker discovery would facilitate the finding of a specific molecular profile for OA or its development. Other methods than LC-MS such as GC-MS, MALDI-MSI and SIMS are promising, but more research needs to be done. Also outstanding is the use of multiple names for the same molecule. If databases would merge their knowledge, molecular identifiers or accession numbers, there will be less confusion on molecule identification. A standardized method to describe the molecules in articles would also contribute to that.
In addition, single cell analysis and spatially resolved – omics techniques will give more insight in the positional context of cells within a tissue. For example, specific inflammatory cells and tissues in cartilage, HFP or synovium of OA patients are responsible for the secretion of pro-inflammatory factors or cartilage degradation factors [Citation76,Citation77].
Thirdly, we should work toward clinical implementation and point-of-care analysis for early detection and intervention of OA. If the molecular signature of multiple tissue types within the knee are known and related to OA progression, blood analysis (not included in the article search of this review) would possibly be a good alternative for the invasive biopsy/surgical methods currently used. The focus now is mainly on synovial fluid, which is close to the source of the problem. However, other tissue types such as meniscus, cartilage and synovium have been shown promising results. In addition, LC-MS has already evolved to a very reliable technique for the detection of biomarkers. Newer advanced, technologies, such as MSI will possibly evolve to highly contributing techniques in the near future, but more research is needed.
Finally, it should be possible to combine data sets using several data analyzing programs and databases. There are several databases available on the internet to analyze proteins (UniProt/SwissProt, NCBInr, DDBJ, etc.) [Citation78], lipids (LIPID MAPS, Lipidomic Net, etc.) or metabolites (METLIN, GOLM, etc.). Each database makes use of a different search technique and includes a different number of proteins or genes. Some make use of the protein name, which can differ even for the same protein; others make use of the gene name or protein accession, which is more reliable and avoids potential mismatches. Protein names can be divided in recommended name (often recommended by database) or alternative name (synonyms, abbreviations, acronyms). The International Protein Nomenclature Guidelines are written to prevent these name differences among databases [Citation79]. In addition, lipid and metabolite databases are of high quality and nomenclature and classification is comparable with proteomic databases [Citation80,Citation81].
7.2. Techniques in development
The possibility of on-tissue analysis of biopsies or possibly during surgery would facilitate clinical decision-making on the spot. This would be feasible with techniques like rapid evaporative ionization MS (REIMS), connected to an intelligent knife: the iKnife. This iKnife generates smoke when in contact with tissue during surgery, which is analyzed in a REIMS system, giving molecular information directly to the surgeon [Citation82]. Direct on- or in-tissue analysis in vivo or on biopsies, would increase our knowledge on OA. However, not much is known about this technique in orthopedic research. In addition, there is currently no clear molecular profile for OA development or progression available. This might be due to the variety in methods, patients, lack of healthy/control samples and lack of molecule identification.
All these steps will eventually lead to better prediction models for OA development, better prediction models for patients after injury and better treatment decisions. Systems such as REIMS/iKnife are promising technologies that might be used at the surgical theater in the near future, contributing to better clinical decision making, less revision surgery, better patient health and decreased health costs. From now on, we expect that all mentioned techniques will develop toward highly recommended and useful techniques to determine patient profiles. For this, standardized methods, bigger patient groups and accessible biobanking of clinical data will make patient profiling more reliant and accessible.
7.3. Not one biomarker for osteoarthritis
In conclusion, we do not believe that one particular biomarker will function as predictive molecule for OA development or early detection. Most likely, a group of molecules (proteins, lipids, metabolites and possibly other molecules combined) will give a better insight in the development OA and possible therapeutic targets. To reach clinical implementation of MS analysis in treatment decision making, standardizing procedures, large relevant cohort studies and sharing of protocols and data are necessary.
Article highlights
Mass spectrometry (imaging) is a promising tool for the detection (and can provide spatial information) of biomarkers for osteoarthritis.
In the literature, synovial fluid is found to be the preferred source in the search for biomarkers for osteoarthritis when analyzing proteins, lipids or metabolites.
A biomarker profile for osteoarthritis is likely to consist of multiple molecules detected in multiple tissues of the effected joint, rather than one specific molecule.
Current studies on biomarkers for osteoarthritis are built on small sample size, a variety of protocols and databases.
Clinical implementation can only be accomplished when studies have been conducted on large patient cohorts, with standardized methods and shared data.
List of abbreviations
1H NMR1H; nuclear magnetic resonance spectroscopy
2DE2D; gel electrophoresis
ACL;anterior cruciate ligament
APC;Iatmospheric pressure chemical ionization
APPI;atmospheric pressure photoionization
CI;chemical ionization
COMP;cartilage oligomeric matrix protein
DESI;desorption electrospray ionization
DMOAD;disease modifying osteoarthritis drug
ECM;extracellular matrix
EI;electron ionization
eOA;early osteoarthritis
ESI;electrospray ionization
FDR;false discovery rate
GC-MS;gas chromatography mass spectrometry
HFPH;offa’s fat pad
HPLC-MS;high performance liquid chromatography mass spectrometry
KLK;ellgren-Lawrence
LC-MS (/MS);liquid chromatography (tandem) mass spectrometry
lOA;late osteoarthritis
MALDI;matrix-assisted laser desorption/ionization
MRI;magnetic resonance imaging
MS;mass spectrometry
MSI;mass spectrometry imaging
NSAID;non-steroidal anti-inflammatory drug
OA;osteoarthritis
OPLS-DA;orthogonal partial least squares discriminant analysis
PCA;principal component analysis
SDS-PAGE;sodium dodecyl sulfate polyacrylamide gel electrophoresis
SIMS;secondary ion mass spectrometry
TKA;total knee arthroplasty
TOF;time of flight
Declaration of interest
The authors have no relevant affiliations or financial involvement with any organization or entity with a financial interest in or financial conflict with the subject matter or materials discussed in the manuscript. This includes employment, consultancies, honoraria, stock ownership or options, expert testimony, grants or patents received or pending, or royalties.
Reviewer disclosures
Peer reviewers on this manuscript have no relevant financial or other relationships to disclose.
Supplemental Material
Download MS Word (117.6 KB)Acknowledgments
were modified from Servier Medical Art, licensed under a Creative Common Attribution 3.0 Generic License. http://smart.servier.com/.
Supplemental Material
Supplemental data for this article can be accessed here
Additional information
Funding
References
- Musumeci G, Aiello FC, Szychlinska MA, et al. Osteoarthritis in the XXIst century: risk factors and behaviours that influence disease onset and progression. Int J Mol Sci. 2015;16(3):6093–6112
- Zhang Y, Jordan JM. Epidemiology of osteoarthritis. Clin Geriatr Med. 2010;26(3):355–369.
- Driban JB, Harkey MS, Liu S-H, et al. Osteoarthritis and Aging: young Adults with Osteoarthritis. Curr Epidemiol Rep. 2020;7(1):9–15.
- Yu SP, Hunter DJ. Managing osteoarthritis. Aust Prescr. 2015;38(4):115–119.
- de Windt TS, Vonk LA, Brittberg M, et al. Treatment and Prevention of (Early) Osteoarthritis Using Articular Cartilage Repair-Fact or Fiction? A Systematic Review. Cartilage. 2013;4(3 Suppl):5S–12S.
- Kraus VB, Karsdal MA. Osteoarthritis: current Molecular Biomarkers and the Way Forward. Calcif Tissue Int. 2020. DOI:https://doi.org/10.1007/s00223-020-00701-7
- Poole AR. Osteoarthritis as a whole joint disease. HSS J. 2012;8(1):4–6.
- Zeng N, Yan ZP, Chen XY, et al. Infrapatellar Fat Pad and Knee Osteoarthritis. Aging Dis. 2020;11(5):1317–1328.
- Ioan-Facsinay A, Kloppenburg M. An emerging player in knee osteoarthritis: the infrapatellar fat pad. Arthritis Res Ther. 2013;15(6):225.
- Moher D, Liberati A, Tetzlaff J, et al. Preferred reporting items for systematic reviews and meta-analyses: the PRISMA statement. Int J Surg. 2010;8(5):336–341.
- Ouzzani M, Hammady H, Fedorowicz Z, et al. Rayyan-a web and mobile app for systematic reviews. Syst Rev. 2016;5(1):210.
- Ahmed U, Anwar A, Savage RS, et al. Protein oxidation, nitration and glycation biomarkers for early-stage diagnosis of osteoarthritis of the knee and typing and progression of arthritic disease. Arthritis Res Ther. 2016;18(1):250.
- Ahrman E, Lorenzo P, Holmgren K, et al. Novel cartilage oligomeric matrix protein (COMP) neoepitopes identified in synovial fluids from patients with joint diseases using affinity chromatography and mass spectrometry. J Biol Chem. 2014;289(30):20908–20916.
- Brophy RH, Cai L, Duan X, et al. Proteomic analysis of synovial fluid identifies periostin as a biomarker for anterior cruciate ligament injury. Osteoarthritis Cartilage. 2019;27(12):1778–1789.
- Gobezie R, Kho A, Krastins B, et al. High abundance synovial fluid proteome: distinct profiles in health and osteoarthritis. Arthritis Res Ther. 2007;9(2):R36.
- Kamphorst JJ, van der Heijden R, DeGroot J, et al. Profiling of endogenous peptides in human synovial fluid by NanoLC-MS: method validation and peptide identification. J Proteome Res. 2007;6(11):4388–4396.
- Liao W, Li Z, Li T, et al. Proteomic analysis of synovial fluid in osteoarthritis using SWATHmass spectrometry. Mol Med Rep. 2018;17(2):2827–2836.
- Roller BL, Monibi F, Stoker AM, et al. Identification of Novel Synovial Fluid Biomarkers Associated with Meniscal Pathology. J Knee Surg. 2016;29(1):47–62.
- Steinbeck MJ, Nesti LJ, Sharkey PF, et al. Myeloperoxidase and chlorinated peptides in osteoarthritis: potential biomarkers of the disease. J Orthop Res. 2007;25(9):1128–1135.
- Timur UT, Jahr H, Anderson J, et al. Identification of tissue-dependent proteins in knee OA synovial fluid. Osteoarthritis Cartilage. 2021;29(1):124–133.
- Corigliano A, Preiano M, Terracciano R. et al. C3f is a potential tool for the staging of osteoarthritis. J Biol Regul Homeost Agents. 2017;31(4 suppl 1):29–35.
- Liao W, Li Z, Wang H, et al. Proteomic analysis of synovial fluid: insight into the pathogenesis of knee osteoarthritis. Int Orthop. 2013;37(6):1045–1053.
- Liao W, Li Z, Zhang H, et al. Proteomic analysis of synovial fluid as an analytical tool to detect candidate biomarkers for knee osteoarthritis. Int J Clin Exp Pathol. 2015;8(9):9975–9989.
- Guo D, Tan W, Wang F, et al. Proteomic analysis of human articular cartilage: identification of differentially expressed proteins in knee osteoarthritis. Joint Bone Spine. 2008;75(4):439–444.
- Rosenthal AK, Gohr CM, Ninomiya J, et al. Proteomic analysis of articular cartilage vesicles from normal and osteoarthritic cartilage. Arthritis Rheum. 2011;63(2):401–411. .
- Wu J, Liu W, Bemis A, et al. Comparative proteomic characterization of articular cartilage tissue from normal donors and patients with osteoarthritis. Arthritis Rheum. 2007;56(11):3675–3684.
- Cillero-Pastor B, Eijkel GB, Blanco FJ, et al. Protein classification and distribution in osteoarthritic human synovial tissue by matrix-assisted laser desorption ionization mass spectrometry imaging. Anal Bioanal Chem. 2015;407(8):2213–2222.
- Tang S, Deng S, Guo J, et al. Deep Coverage Tissue and Cellular Proteomics Revealed IL-1beta Can Independently Induce the Secretion of TNF-Associated Proteins from Human Synoviocytes. J Immunol. 2018;200(2):821–833.
- Roller BL, Monibi F, Stoker AM, et al. Characterization of Meniscal Pathology Using Molecular and Proteomic Analyses. J Knee Surg. 2015;28(6):496–505.
- Zheng K, Shen N, Chen H, et al. Global and targeted metabolomics of synovial fluid discovers special osteoarthritis metabolites. J Orthop Res. 2017;35(9):1973–1981.
- Kosinska MK, Liebisch G, Lochnit G, et al. A lipidomic study of phospholipid classes and species in human synovial fluid. Arthritis Rheum. 2013;65(9):2323–2333. .
- Kosinska MK, Liebisch G, Lochnit G, et al. Sphingolipids in human synovial fluid--a lipidomic study. PLoS One. 2014;9(3):e91769.
- Kosinska MK, Ludwig TE, Liebisch G, et al. Articular Joint Lubricants during Osteoarthritis and Rheumatoid Arthritis Display Altered Levels and Molecular Species. PLoS One. 2015;10(5):e0125192.
- Xia J, Psychogios N, Young N, et al. MetaboAnalyst: a web server for metabolomic data analysis and interpretation. Nucleic Acids Res 2009 W652–60. doi: https://doi.org/10.1093/nar/gkp356.
- Cillero-Pastor B, Eijkel GB, Kiss A, et al. Matrix-assisted laser desorption ionization-imaging mass spectrometry: a new methodology to study human osteoarthritic cartilage. Arthritis Rheum. 2013;65(3):710–720.
- Eveque-Mourroux MR, Emans PJ, Boonen A, et al. Heterogeneity of Lipid and Protein Cartilage Profiles Associated with Human Osteoarthritis with or without Type 2 Diabetes Mellitus. J Proteome Res. 2021. DOI:https://doi.org/10.1021/acs.jproteome.1c00186.
- Mickiewicz B, Kelly JJ, Ludwig TE, et al. Metabolic analysis of knee synovial fluid as a potential diagnostic approach for osteoarthritis. J Orthop Res. 2015;33(11):1631–1638.
- Carlson AK, Rawle RA, Wallace CW, et al. Characterization of synovial fluid metabolomic phenotypes of cartilage morphological changes associated with osteoarthritis. Osteoarthritis Cartilage. 2019;27(8):1174–1184.
- Cillero-Pastor B, Eijkel G, Kiss A, et al. Time-of-flight secondary ion mass spectrometry-based molecular distribution distinguishing healthy and osteoarthritic human cartilage. Anal Chem. 2012;84(21):8909–8916.
- Van Spil WE, Kubassova O, Boesen M, et al. Osteoarthritis phenotypes and novel therapeutic targets. Biochem Pharmacol. 2019;165:41–48.
- Mobasheri A, Saarakkala S, Finnila M, et al. Recent advances in understanding the phenotypes of osteoarthritis. F1000Res 2019 8. doi: https://doi.org/10.12688/f1000research.20575.1
- Van De Vyver A, Clockaerts S, Van De Lest CHA, et al. Synovial Fluid Fatty Acid Profiles Differ between Osteoarthritis and Healthy Patients. Cartilage 2020;11(4):473–478. .
- Kim S, Hwang J, Kim J, et al. Metabolite profiles of synovial fluid change with the radiographic severity of knee osteoarthritis. Joint Bone Spine. 2017;84(5):605–610. .
- Ritter SY, Subbaiah R, Bebek G, et al. Proteomic analysis of synovial fluid from the osteoarthritic knee: comparison with transcriptome analyses of joint tissues. Arthritis Rheum. 2013;65(4):981–992.
- Folkesson E, Turkiewicz A, Ali N, et al. Proteomic comparison of osteoarthritic and reference human menisci using data-independent acquisition mass spectrometry. Osteoarthritis Cartilage. 2020;28(8):1092–1101.
- Carlson AK, Rawle RA, Adams E, et al. Application of global metabolomic profiling of synovial fluid for osteoarthritis biomarkers. Biochem Biophys Res Commun. 2018;499(2):182–188.
- Punzi L, Galozzi P, Luisetto R, et al. Post-traumatic arthritis: overview on pathogenic mechanisms and role of inflammation. RMD Open. 2016;2(2):e000279.
- Courtney P, Doherty M. Joint aspiration and injection and synovial fluid analysis. Best Pract Res Clin Rheumatol. 2013;27(2):137–169.
- Friel NA, Chu CR. The role of ACL injury in the development of posttraumatic knee osteoarthritis. Clin Sports Med. 2013;32(1):1–12.
- Rai MF, Brophy RH, Sandell LJ. Osteoarthritis following meniscus and ligament injury: insights from translational studies and animal models. Curr Opin Rheumatol. 2019;31(1):70–79.
- Mathiessen A, Conaghan PG. Synovitis in osteoarthritis: current understanding with therapeutic implications. Arthritis Res Ther. 2017;19(1):18.
- Belluzzi E, Stocco E, Pozzuoli A, et al. Contribution of Infrapatellar Fat Pad and Synovial Membrane to Knee Osteoarthritis Pain. Biomed Res Int. 2019;2019:6390182.
- Barr AJ, Campbell TM, Hopkinson D, et al. A systematic review of the relationship between subchondral bone features, pain and structural pathology in peripheral joint osteoarthritis. Arthritis Res Ther. 2015;25(17):228.
- Kovacs B, Vajda E, Nagy EE, et al. Interactions of the Wnt and OPG-RANKL-RANK Signaling at the Bone-Cartilage Interface in Osteoarthritis. Int J Mol Sci. 2019;20:18.
- Dakin SG, Martinez FO, Yapp C, et al. Inflammation activation and resolution in human tendon disease. Sci Transl Med. 2015;7(311):311ra173.
- Le Quesne JP, Spriggs KA, Bushell M, et al. Dysregulation of protein synthesis and disease. J Pathol. 2010;220(2):140–151.
- Corradi V, Mendez-Villuendas E, Ingolfsson HI, et al. Lipid-Protein Interactions Are Unique Fingerprints for Membrane Proteins. ACS Cent Sci. 2018;4(6):709–717.
- Ioan-Facsinay A, Kloppenburg M. Bioactive lipids in osteoarthritis: risk or benefit? Curr Opin Rheumatol. 2018;30(1):108–113.
- Klont F, Bras L, Wolters JC, et al. Assessment of Sample Preparation Bias in Mass Spectrometry-Based Proteomics. Anal Chem. 2018;90(8):5405–5413.
- Gundry RL, White MY, Murray CI, et al. Preparation of proteins and peptides for mass spectrometry analysis in a bottom-up proteomics workflow. Curr Protoc Mol Biol. 2009. Chapter 10:Unit10.25
- Hardin JA, Cobelli N, Santambrogio L. Consequences of metabolic and oxidative modifications of cartilage tissue. Nat Rev Rheumatol. 2015;11(9):521–529.
- Hsueh MF, Khabut A, Kjellstrom S, et al. Elucidating the Molecular Composition of Cartilage by Proteomics. J Proteome Res. 2016;15(2):374–388.
- El-Aneed A, Cohen A, Mass Spectrometry BJ. Review of the Basics: electrospray, MALDI, and Commonly Used Mass Analyzers. Appl Spectrosc Rev. 2009;44(3):210–230.
- Domon B, Aebersold R. Mass spectrometry and protein analysis. Science. 2006;312(5771):212–217.
- Kiraly M, Dalmadine Kiss B, Vekey K, et al. [Mass spectrometry: past and present]. Acta Pharm Hung. 2016;86(1):3–11.
- Perkel JM Mass Spectrometry Ionization Sources: labCompare; 2009 [cited 2021 May 28, 2021 May 28]. Available from: https://www.labcompare.com/18-Mass-Spectrometry-Ionization-Sources/
- Gkretsi V, Simopoulou T, Tsezou A. Lipid metabolism and osteoarthritis: lessons from atherosclerosis. Prog Lipid Res. 2011;50(2):133–140.
- Rockel JS, The Metabolome KM. Osteoarthritis: possible Contributions to Symptoms and Pathology. Metabolites 2018;8:4.
- Fontanella CG, Macchi V, Carniel EL, et al. Biomechanical behavior of Hoffa’s fat pad in healthy and osteoarthritic conditions: histological and mechanical investigations. Australas Phys Eng Sci Med. 2018;41(3):657–667.
- Goracci L, Tortorella S, Tiberi P, et al. Lipostar, a Comprehensive Platform-Neutral Cheminformatics Tool for Lipidomics. Anal Chem. 2017;89(11):6257–6264.
- Lahm A, Mrosek E, Spank H, et al. Changes in content and synthesis of collagen types and proteoglycans in osteoarthritis of the knee joint and comparison of quantitative analysis with Photoshop-based image analysis. Archives of Orthopaedic and Trauma Surgery. 2010;130(4):557–564.
- Miosge N, Hartmann M, Maelicke C, et al. Expression of collagen type I and type II in consecutive stages of human osteoarthritis. Histochem Cell Biol. 2004;122(3):229–236.
- Smyth EM, Grosser T, Wang M, et al. Prostanoids in health and disease. J Lipid Res. 2009;50(Suppl):S423–8.
- Paduszynski W, Jeskiewicz M, Uchanski P, et al. Hoffa’s Fat Pad Abnormality in the Development of Knee Osteoarthritis. Adv Exp Med Biol. 2018;1039:95–102.
- Loeser RF. Molecular mechanisms of cartilage destruction: mechanics, inflammatory mediators, and aging collide. Arthritis Rheum. 2006;54(5):1357–1360.
- Ushiyama T, Chano T, Inoue K, et al. Cytokine production in the infrapatellar fat pad: another source of cytokines in knee synovial fluids. Ann Rheum Dis. 2003;62(2):108–112.
- Hoff P, Buttgereit F, Burmester GR, et al. Osteoarthritis synovial fluid activates pro-inflammatory cytokines in primary human chondrocytes. Int Orthop. 2013;37(1):145–151.
- Xu D, Xu Y. Protein databases on the internet. Curr Protoc Mol Biol. 2004. DOI:https://doi.org/10.1002/0471142727.mb1904s68
- Bruford EA, Braschi B, Denny P, et al. Guidelines for human gene nomenclature. Nat Genet. 2020;52(8):754–758.
- Liebisch G, Fahy E, Aoki J, et al. Update on LIPID MAPS classification, nomenclature, and shorthand notation for MS-derived lipid structures. J Lipid Res. 2020;61(12):1539–1555.
- Liebisch G, Vizcaino JA, Kofeler H, et al. Shorthand notation for lipid structures derived from mass spectrometry. J Lipid Res. 2013;54(6):1523–1530.
- Balog J, Sasi-Szabo L, Kinross J, et al. Intraoperative tissue identification using rapid evaporative ionization mass spectrometry. Sci Transl Med. 2013;5(194):194ra93.