ABSTRACT
Introduction
Laser Capture Microdissection (LCM) uses a laser to isolate, or capture, specific cells of interest in a complex heterogeneous tissue section, under direct microscopic visualization. Recently, there has been a surge of publications using LCM for tissue spatial molecular profiling relevant to a wide range of research topics.
Areas Covered
We summarize the many advances in tissue Laser Capture Proteomics (LCP) using mass spectrometry for discovery, and protein arrays for signal pathway network mapping. This review emphasizes: a) transition of LCM phosphoproteomics from the lab to the clinic for individualized cancer therapy, and b) the emerging frontier of LCM single cell molecular analysis combining proteomics with genomic, and transcriptomic analysis. The search strategy was based on the combination of MeSH terms with expert refinement.
Expert Opinion
LCM is complemented by a rich set of instruments, methodology protocols, and analytical A.I. (artificial intelligence) software for basic and translational research. Resolution is advancing to the tissue single cell level. A vision for the future evolution of LCM is presented. Emerging LCM technology is combining digital and AI guided remote imaging with automation, and telepathology, to a achieve multi-omic profiling that was not previously possible.
1. Introduction
1.1. Tissue spatially resolved molecular profiling is an essential component of personalized oncology
Recognition of the limitations of whole-tissue extraction profiling has driven a rapidly expanding field of investigators who are creating technology, software, and analytical tools to explore the tissue cellular spatial interactome. Consequently, a rich set of new research tools now exists to histologically localize proteomic and genomic markers, or to physically procure subpopulations of cells, or individual cells, within a formalin fixed or frozen section [Citation1–3]. Within this cadre of technologies, Laser Capture Microdissection (LCM) [Citation4–6] has proven to be a reliable means of procuring tissue cellular populations, achieving high sensitivity and high precision for multiplex genomic and proteomic profiling [Citation4,Citation7–9]. LCM has been used to generate data for a wide variety of research questions, ranging from entomology [Citation10–12], agriculture research [Citation13,Citation14], embryology [Citation15,Citation16], metabolic disease [Citation17–19], heart disease [Citation20,Citation21], neurobiology [Citation22,Citation23], infectious disease [Citation24–26], and cancer [Citation6] (see below).
Post-translational modifications, such as protein phosphorylation, provide key functional information that cannot be obtained by genomic and transcriptomic profiling. Immunohistochemistry often lacks adequate sensitivity for multiplex quantitation of the full repertoire of phosphoproteins in a histologic field [Citation27–29]. Addressing this critical functional proteomic information, LCM technology has taken a lead position for the quantitative multiplex tissue cell spatial profiling of phosphoproteins, with translation of this approach to full blinded verification in clinical trial testing [Citation30–35]. The first area of emphasis for this review is the transition of LCM from the research lab to clinical trial validation of tissue cellular protein and phosphoprotein quantitation as a means to guide checkpoint inhibitor therapy and kinase inhibitor therapy.
Individualized therapy guided by molecular profiling of biopsy tissue is conventionally conducted by immunohistochemistry and bulk genomic profiling [Citation36–38] [Citation3,Citation30,Citation39]. Nevertheless, while genomic. profiling of whole tissue specimens has shown considerable clinical potential for the classification of patient responses to chemotherapy, extracting a whole-tissue specimen is associated with a very high degree of preanalytical variability due to cellular heterogeneity, the unknown proportion of tumor or host subpopulations that all contribute to the extract, and the destruction of information about the spatial location of cell types within the tissue. For a variety of molecular targeted therapies, an RNA transcript related to the molecular drug target can be expressed in tumor cells as well as non-tumor host stroma and immune cells. Since the proportion of cancer cells in a bulk tumor sample is unknown (and varies in different regions of the tumor, and the relative expression of the RNA transcript in the tumor compared to the host cells is unknown), bulk profiling can generate false positives and false negatives. The need for spatial molecular mapping is particularly important in tumor immunology, and tumor sub-clonal analysis, where the spatial proximity (and class) of tumor cell and immune cell populations provides critical information relevant to immunotherapy and drug resistance phenotypes [Citation7,Citation30,Citation39]. Thus, ideal tissue spatial profiling technology should permit genomic, and proteomic analysis of the same cells or cellular subpopulations while retaining their spatial context. Thus, the second major area of emphasis for this review is the exciting and rapidly emerging frontier of LCM single cell (or 10 cells) molecular analysis that combines proteomics with genomic, and transcriptomic analysis.
The third area of emphasis for this review is the future vision for the field of LCM. Laser Capture Microdissection (LCM) is a reliable tool, with many instrument types, and downstream analysis methods, to choose from [Citation4–6,Citation25,Citation40–43]. It is widely used to study the spatial molecular signatures of individual cell populations within a complex heterogeneous tissue biopsy. Despite this success, LCM suffers from drawbacks related to time-consuming visualization and manual selection and collection of the cells of interest. The choice of LCM cell types to be captured and analyzed is usually done under direct visual microscopic analysis of the instrument. The problem is the poor image quality of the LCM microscopic image since the tissue must remain open-faced (without a cover slip) for cell capture. Nevertheless, digital image capture, liquid coverslip chemistry, A.I./machine learning (ML), and continued automation will play a future role. These technology advances will make LCM more generally acceptable to both basic scientists and anatomic clinical pathologists through remote viewing, annotation, and microdissection guided by the marked-up histologic image [Citation44–49]. Digital and cloud pathology can also offer AI-driven H&E and multiplex immuno-guided analytics for LCM bridging to downstream deep genomic, transcriptomic, and proteomic temporospatial analysis as discussed below for emerging digital histopathological and biodiscovery workflows [Citation50–54].
1.2. A strength of LCM is tissue spatial molecular profiling of PTMs (phosphoproteins): mapping activated, or down-regulated, kinase pathways
RNA transcripts provide limited information about protein–protein interactions and the state of the cellular signaling pathways. A high proportion of molecular targeted therapies are directed at protein targets such as cell surface receptors regulating growth and immune suppression, intracellular protein signaling molecules regulating apoptosis and growth, or protein kinases and/or their substrates. The activation state of these proteins and their networks fluctuate constantly depending on the tumor–host microenvironment cellular cross-talk [Citation28,Citation29,Citation34]. Consequently, profiling the active (e.g. phosphorylated) state of tissue cellular protein signal pathway proteins contains a rich source of information about the active pathways that drive the cancer phenotype and are the drug target candidates. Protein array technologies, such as Reverse Phase Protein Microarrays (RPPAs), which are essentially miniaturized dot-blot immunoassays, using highly specific anti-phospho-epitope monoclonal antibodies, can broadly quantify hundreds of cellular phosphoproteins with sensitivity and precision. RPPA has been successfully combined with LCM to achieve routine multiplex measurement of many hundreds of phosphoproteins in selected cellular subpopulations [Citation55,Citation56]. A variety of additional multiplex immunoassay technologies are also being used to quantify phosphoproteins in tissue. These include mass spectrometry analysis of posttranslational modifications in tissue and cellular extracts, and the application of multiplex sandwich bead immunoassays to extracted tissue samples. Moreover, CyTOF and Nanostring technologies have also offered a set of phosphoprotein analytes on their menu [Citation2,Citation3,Citation52,Citation57–60] .
Each patient’s tumor can have dozens to hundreds of potentially oncogenic genomic derangements. Faced with a plurality of genomic alterations, which of these multiple genomic alterations is driving the cancer and should be the target for therapy? The activity of protein signaling in a cancer cell provides functional information about the dominant drivers of the cellular phenotype that is a consequence of the genomic lesion. Thus, profiling the protein signaling network-associated proteins of a cancer cell, using LCM combined with high specificity phosphoprotein immunoassays, offers therapeutic information within a spatial context: 1) active or quiescent signal pathways in the cancer cell that contain known therapeutic targets for drugs, and 2) cancer-causing molecular changes that can be linked back upstream to a particular genomic lesion in the same microdissected region of the tissue.
1.3. Emerging role of tissue immune cell proteomics for cancer immunotherapy
Tissue cellular proteomics is filling a critical unmet need during the current revolution in cancer immunotherapy. Tumor cell genomic profiling provides limited information about the host immune cell recognition of the tumor [Citation61,Citation62]. The class and subtype of immune cells, and their proteomic phenotype, within the tumor microenvironment is highly relevant for the selection of appropriate immunotherapy [Citation53,Citation57,Citation63]. Expression of cell surface receptors involved in immune cell function and tumor cell recognition, such as PDL-1 and PD-1, and the cellular signaling taking place within the host immune cells in the tumor microenvironment, cannot be ascertained by genomic profiling of the tumor. Consequently, as personalized immunotherapy begins to dominate the next generation of cancer treatment options, more and more emphasis will be placed on host immune cell proteomics. Once again, technologies such as proteomics and phosphor-proteomics, in combination with Laser Capture Microdissection, can offer a direct means to profile the quantitative levels of cell surface receptors targeted by immune checkpoint inhibitors. Dikensen et al., Baldelli et al., and Peirobon et al. have shown that LCM can be used to quantify PDL-1 in subsets of microdissected tumor cells in a variety of tissue and tumor types, including IR treated skin [Citation64–67]. Peirobon reports on an LCM RPPA study of 178 patient tumor samples including: 72 ovarian cancers (OC), 57 lung adenocarcinomas (LC), 30 metastatic breast cancers (MBC), and 19 pancreatic cancers (PC). PDL1 protein expression was measured on a continuous scale using quantitative LCM lysate protein array-based analysis. Each tumor type was processed and arrayed independently. Experimental samples and reference standards used for inter-assay normalization were evaluated in triplicates. PDL1 expression varied greatly across tumor types. LC exhibited the greatest intra-tumor fold dynamic range (>35-fold), followed by OC (<13-fold), MBC (<4-fold), and PC (<2-fold). PDL1 expression of 46/57 (80.7%) LC, 17/30 (56.7%) MBC, 6/19 (31.5%) PC, and 20/72 (27.8%) OC was greater than the population median of all tumors combined. For the LC samples within the top quartile of the population, 10 (71.4%) were KRAS mutant lesions and 4 (28.6%) were WT tumors. PC harboring a KRAS mutation showed an overall lower expression of PDL1 with only 2/19 (10.5%) above the population median and none within the top quartile. The authors conclude that LCM proteomics can achieve continuous quantitative scale, as well as capture its broad dynamic range for checkpoint protein measurement in human biopsy tumor specimens. In the future, it should be feasible to use LCM PTM proteomics [Citation45] to map the signaling pathway activation status of immune cell subtypes within the tumor microenvironment [Citation46,Citation63], with particular emphasis on the spatial proximity of the immune cells, and the class of immune cells, associated with the invading tumor cells.
2. Spatially resolved tissue proteomics technology categories
Spatially resolved tissue proteomics can provide functional molecular information that is distinct from that obtained by spatially resolved transcriptomics [Citation2,Citation3]. Three major classes of technologies exist for spatially (in situ) resolved proteomics ().
Figure 1. Spatially Resolved Tissue Proteomics Technology Categories. Example major classes of technologies that exist for spatially (in situ) resolved proteomics: A) Analyte probe labeled or label-free microscopic imaging of tissue: Example technologies that fall under this category include standard clinical immunohistochemistry using one antibody probe at a time, multiplex sequential or parallel antibody probes, and label free imaging. B) Surface scanning or grid-based surface elution of labeled or unlabeled tissue molecules. Example technologies that fall under this category include scanning MALDI, Tissue laser scanning after the tissue or cells are labeled with heavy metal tagged antibodies (CyTOF), or antibody-labeled nucleic acid barcoded tags (NanoString nCounter), or D) Isolation or capture of full thickness tissue regions of interest followed by external extraction and analysis. Example technologies that fall under this category include Laser Capture Microdissection LCM using ultraviolet laser cutting around the perimeter of the region, or cell of interest, or direct full thickness removal by infrared laser capture embedding onto a capture surface or both UV and IR laser methods combined together
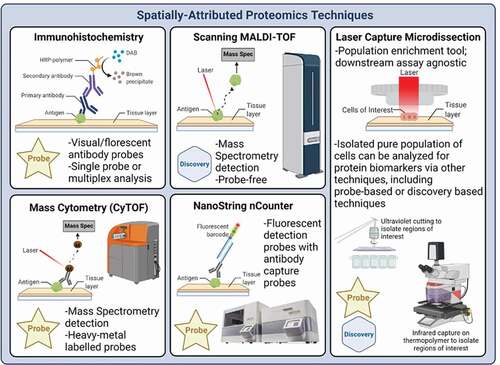
Analyte probe labeled or label-free microscopic imaging of tissue.
Examples of technologies that fall under this category include standard clinical immunohistochemistry using one antibody probe at a time, multiplex sequential or parallel antibody probes, and label-free imaging using non-traditional optical approaches [Citation50,Citation51,Citation68].
(B) Surface scanning or grid-based surface elution of labeled or unlabeled tissue molecules
Examples of technologies that fall under this category include scanning MALDI, Tissue laser scanning after the tissue or cells are labeled with heavy metal tagged antibodies, or antibody-labeled nucleic acid barcoded tags [Citation53,Citation57] .
(C) Isolation or capture of full thickness tissue regions of interest followed by external extraction and analysis.
Examples of technologies that fall under this category include Laser Capture Microdissection LCM using ultraviolet laser cutting [Citation6,Citation42,Citation68,Citation69] around the perimeter of the region, or cell of interest, or direct full thickness removal by infrared laser capture embedding onto a capture surface (), or both UV and IR laser methods combined together. In addition, macro-dissection of tissue regions using tools, needles, or scraping also falls under this category.
Figure 2. LCM technology uses laser cutting or laser induced surface capture (upper insert) to procure select tissue full thickness sample. The procured material is then extracted and subjected to a plurality of downstream analytical tools yielding discovery of spatially localized tissue molecules or multiplex quantitation of known analytes
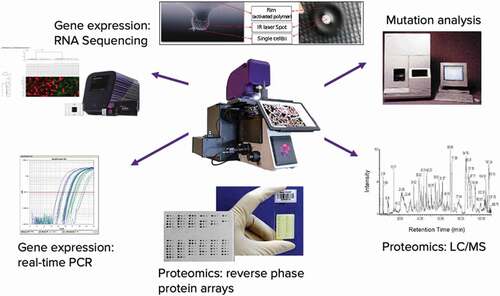
2.1. Advantages and disadvantages (for spatial proteomics) of each technology class
The advantage of microscopic spatial imaging with anti-protein analyte antibodies is low cost, the wide availability of validated antibodies, and the direct high resolution microscopic localization without the requirement for extensive digital image processing or image reconstruction. The disadvantage is the lack of sensitivity for protein PTMs, and the difficulty quantifying or normalizing the data considering the wide variability in diagnostic biopsy fixed or frozen tissue sections. Finally, this is not a method for discovery-based proteomics since the analyte is already known. Surface scanning or grid-based technologies () using antibody-labeled proteins are limited by the surface penetration of the probes, the limited resolution and sensitivity of low abundance proteins or PTMs, and the complicated mechanical nature of the scanning, registry, and quantitative retrieval of the probe. Surface scanning MALDI is useful for discovery-based proteomic applications but also has limitations in resolution and sensitivity.
The advantages of LCM tissue proteomics include the following:
High resolution limited to the wavelength of the IR or UV laser procurement method.
High yield full thickness of the tissue section in the region or cell of interest.
High multiplex capability for parallel downstream analysis of known proteins and PTMs.
Useful for both MS discovery and known analyte measurement
Validated methods exist for combining LCM procured material with combined with genomics and transcriptomics in the same region, f) used widely outside the originator’s labs by researchers and for clinical trials and LDT CAP CLIA (Clinical Laboratory Improvement Amendments of 1988)
medical diagnostic labs. LCM is qualified as a diagnostic CPT billing code.
e. Emerging automated remote selection of regions of interest (see Conclusions).
The weakness of LCM is the laborious time-consuming nature of conducting the cellular or tissue procurement under direct microscopic visualization, the low quality of the non-coverslipped histologic image when microdissection is directly conducted by the operator, and the requirement for downstream analytical tools that are sensitive enough to work with low-volume tissue samples, fixed or unfixed.
2.2. Laser Capture Microdissection PTM spatial resolved cellular signal pathway profiling for personalized neoadjuvant therapy
Over the past decade, breast cancer neoadjuvant trials have focused on pathologic complete remission (pCR) as a primary endpoint to select intensification of therapy or dual blockade for HER2+ breast cancer. Based on the outcome of the St. Gallen Consensus 2017, neoadjuvant therapy is recommended as a standard of care in stage II–III HER2-positive (HER2+) early breast cancer (EBC) [Citation70,Citation71]. The I-SPY TRIAL series established pCR as an attractive means to rapidly identify and graduate drugs to the Phase III setting [Citation37] with FDA guidance on the importance of pCR as a powerful means of identifying new classes of targeted inhibitors [Citation72]. Recent results from the KATHARINE trial indicate that pathological complete response (pCR) status can predict patient outcome, and can be used as a basis to escalate anti-HER2 therapy after surgery [Citation73]. Dual-antibody blockade together with chemotherapy has become the neoadjuvant standard, following the NEOSPHERE [Citation74] results. The chemotherapy backbone consists either of an anthracycline-taxane sequence or of an anthracycline-free regimen, such as docetaxel and carboplatin. Adjuvant anti-HER2 therapy is then selected based on pCR status and initial tumor burden. Thus, pCR status has become an integral component of a multidisciplinary approach striving for optimal treatment results for patients with HER2+ EBC [Citation70,Citation75–77].
While neoadjuvant therapy of HER2 positive breast cancer has been a success story [Citation78,Citation79], further reductions in cardiac toxicity, and increases in the durability of response, can potentially be actualized by the use of analytical tools that predict pCR to personalize de-escalation and escalation regimens [Citation71]. Goutsouliak et al. (2019) [Citation80] recently concluded ‘The development of a molecular approach to differentiate patients who are likely to benefit from HER2-targeted therapy with less or no chemotherapy (treatment de-escalation) from those who require chemotherapy or need additional treatment (escalation) remains an overarching challenge.’ Clinical studies have supported the recent approval of two escalation strategies [Citation81, Citation82, Citation83–86] a) pertuzumab and trastuzumab combined with chemotherapy for up to 1 year for high-risk patients, and, b) extension of adjuvant anti-HER2 treatment with 1 year of neratinib, especially for hormone receptor-negative breast cancer patients who are less than 1 year from trastuzumab completion. Unfortunately, these escalations are associated with considerable increased costs and additional risks of toxicity. Cardiac toxicity is a significant concern, in particular for older patients and those with cardiac comorbidities. Therefore, careful and accurate patient risk stratification up-front, is essential to minimize risk and maximize benefit. For this reason, many investigators have called for a molecular marker to accurately predict the likelihood of pCR [Citation80]. The value and utility of a predictor of pCR to influence the important treatment decision to escalate is strongly supported by the AVATAXHER trial and the prospective, phase II, neoadjuvant WSG-ADAPT trial. The WSG-ADAPT [Citation86] trial and the PER-ELISA [Citation82] also supported the value of a predictor of future pCR. All these aforementioned examples document that a) a predictor that scores a patient at low risk for recurrence may contribute to a justification for reducing the length of HER2 blockade or reducing chemotherapy regimens, and b) a predictor that scores a patient at high risk for recurrence can contribute to a decision for treatment escalation, following clinically validated regimens. As shown in LCM combined with PTM phosphoprotein epitope measurement conducted in microdissected HER2 positive cancer cells is highly predictive of pCR, independent of the HER2 inhibitor modality. LCM PTM HER2 activation status has significant advantages compared to previous predictors because the test is conducted on the pretreatment biopsy and directly evaluates the molecular target of the major classes of HER2 neoadjuvant therapies.
Figure 3. Phosphorylated epitopes indicative of ERBB2 (HER2) family signal activity are correlated with pathologic complete response (pCR). Dimerization (homotypic or heterotypic) following ligand engagement triggers autophosphorylation. The phosphorylated sites on the cytoplasmic domain dock with downstream pathways indicated in green and yellow. Receptor kinase function, and downstream signaling driving the cancer cell can be blocked by Pertuzumab, T-DM-1, or Trastuzumab, on the cell surface domain, or by kinase inhibitors Neratinib and Lapatinib that act at the level of the cytoplasmic domain(s). Unblinding of the RPPA data revealed that the most significant phosphorylated epitopes predictive of pCR were located in the distal cytoplasmic domain of the ERBB1 receptor (outlined in the red box) that a) is the known target of Neratinib, and b) are docking sites for triggering downstream growth and survival pathways driving the breast cancer cell. Insert: 2D scatterplot indicating clear separation of pCR yes(green)/no(red). from Wulkuhle et al [Citation31]
![Figure 3. Phosphorylated epitopes indicative of ERBB2 (HER2) family signal activity are correlated with pathologic complete response (pCR). Dimerization (homotypic or heterotypic) following ligand engagement triggers autophosphorylation. The phosphorylated sites on the cytoplasmic domain dock with downstream pathways indicated in green and yellow. Receptor kinase function, and downstream signaling driving the cancer cell can be blocked by Pertuzumab, T-DM-1, or Trastuzumab, on the cell surface domain, or by kinase inhibitors Neratinib and Lapatinib that act at the level of the cytoplasmic domain(s). Unblinding of the RPPA data revealed that the most significant phosphorylated epitopes predictive of pCR were located in the distal cytoplasmic domain of the ERBB1 receptor (outlined in the red box) that a) is the known target of Neratinib, and b) are docking sites for triggering downstream growth and survival pathways driving the breast cancer cell. Insert: 2D scatterplot indicating clear separation of pCR yes(green)/no(red). from Wulkuhle et al [Citation31]](/cms/asset/377fdcd1-953e-411b-9366-74db4bb569a2/ieru_a_1984886_f0003_oc.jpg)
The I-SPY series of clinical research studies, designed by Barry and Esserman, is an exemplary example of how protein biomarkers associated with ERBB amplification or activation can be discovered that are predictive of therapeutic success for patients undergoing therapy with experimental HER2 directed neoadjuvant therapy agents. Quantitative multiplex LCM RPPA-based phosphoprotein analysis of LCM-enriched breast carcinoma cells from pre-treatment core needle biopsy material from patients treated with Neratinib in the I-SPY2 TRIAL (Investigation of Serial Studies to Predict Your Therapeutic Response With Imaging and Molecular Analysis; Clinical Trials.gov identifier: NCT01042379); revealed kinase pathway biomarkers strongly correlated with pCR and predictive of a high probability of future phase III trial success [Citation31,Citation32]. The I-SPY 2 TRIAL (Investigation of Serial Studies to Predict Your Therapeutic Response With Imaging and Molecular Analysis 2; Clinical Trials.gov identifier: NCT01042379) is a phase II, adaptive neoadjuvant therapy trial [Citation35,Citation37]. The goal of ISPY2 is to identify improved treatment regimens for patient subsets on the basis of molecular characteristics (biomarker signatures) of their disease. Regimens of new agents that show a high Bayesian predictive probability of being more effective than standard therapy will graduate from the trial with their corresponding biomarker signature(s). Regimens of New Agents are dropped if they show a low probability of improved efficacy with any biomarker signature. The major proteomic biomarkers evaluated in ISPY 2 are measured using RPPA 16 by the investigator team.
Over the past 10 years, it has been shown that quantitative measurements of the phosphorylation status of HER family intracellular receptor domains using RPPA technology or immunohistochemistry, along with coincident phosphorylation of downstream signaling proteins, are indicative of an active state of signaling flux driving downstream pro-survival, growth, and invasion [Citation87–91]. Clinical data supporting this hypothesis and ISPY trial clinical verification data [Citation8,Citation30,Citation92,Citation93] indicate that coordinated phosphorylation (following dimerization [Citation94] of specific intracellular HER family receptor domains occurring at the same time, namely, HER2 and EGFR activation) is strongly associated with HER2 neoadjuvant therapy pCR for a variety of HER2-targeted agents: (neratinib, TDM-1, trastuzumab, and pertuzumab); both intracellular tyrosine kinase inhibitors and extracellular mAb anti-HER2 therapies. Moreover, quantitative analysis of LCM RPPA proteomics shows a lack of significant association (p < 0.68) of proliferation index measured by Ki67 levels with pCR, providing rationale for measuring the activation status of the HER family drug targets as a novel marker that does not simply track with growth rate. The generalization of this logic is in keeping with Gordon et al. [Citation94] showing that HER2 activation (phosphorylation) status is potentially predictive of the clinical activity of pertuzumab, a HER dimerization inhibitor, for advanced HER2+ ovarian cancer.
Breast cancer cells, which are phenotypically and functionally driven by HER2 pathway activation, as measured by HER2 phosphorylation and dimerization to EGFR (leading to EGFR phosphorylation), are selectively sensitive to HER2-targeted inhibitors that act to shut down this same pathway, whether or not the inhibitor acts on the extracellular or cytoplasmic domains of HER2 and its dimerization (EGFR/HER1 and HER3) partners. Phosphorylation of HER2 cytoplasmic domain kinase substrate epitopes, and phosphorylation of biochemically linked signaling proteins participating in downstream growth, survival or invasion, has been well established for a decade as a means of judging the level of signaling flux in tumor cells expressing members of the HER family [Citation95]. HER2 cooperatively dimerizes with non-HER2 receptor monomers, such as EGFR and HER3. Consequently, the phosphorylation state of multiple members of the HER family is relevant to judging the activity of HER2 signaling. In order to explore the hypothetical link between HER2 pathway activation and HER2-directed treatment response, an LCM PTM quantitative assay for breast cancer cells requires only 4–5 eight micron sections of a core needle biopsy, or a surgical tissue block to measure 300 end points. The assay uses LCM followed by RPPA to measure a selected and focused panel of analytically validated phosphorylation epitopes on members of the HER family and downstream pathways. The calibrated assay was developed under CAP/CLIA compliance [Citation6,Citation34,Citation42] (CAP: 7223012/CLIA: 49D2002076) laboratory. The assay of and 4 was verified from blinded outcome studies using both frozen tumor cases derived from the I-SPY trials as 16 well as FFPE surgical and core needle biopsy material. Independent LCM RPPA investigators throughout the world (RPPA international conference report RPPA guidelines) [Citation31,Citation34,Citation55] have used the same RPPA-based phosphoprotein assay to map tumor pathway signaling and correlate the results with genomic analysis or tumor phenotype [Citation36,Citation55,Citation96], reporting high resolution, high sensitivity, and large multiplex capability.
2.3. LCM RPPA HER2 Pathway activation predicts pCR following Neratinib therapy
Neratinib, a pan-erythroblastic oncogene B (ERBB) inhibitor, was available to patients with all tumor subtypes in the I-SPY 2 TRIAL. Neratinib (HKI-272) [Citation81,Citation97,Citation98] is a low molecular weight orally administered irreversible pan-HER tyrosine kinase inhibitor of HER1, HER2, and HER4. The inhibitor binds covalently to the intracellular tyrosine kinase domain and inhibits autophosphorylation and subsequent downstream signaling (). Because neratinib was available to all patients in the trial, this study provided an opportunity to test the efficacy of the drug in patients with HER2-negative tumors. Neratinib graduated in the HR negative/HER2-positive signature. On the basis of the mechanism of action of neratinib as a potent HER family kinase inhibitor combined with observations of I-SPY 1 TRIAL results, Wulfkuhle et al. [Citation31–33] compare the following measurements on the same cases: (1) assessment of selected mutation/copy number alterations by exome sequencing, (2) mRNA expression levels by expression microarrays, and analysis of protein/phosphoprotein levels by LCM RPPA as specific biomarkers of neratinib response. LCM RPPA analysis assessed the ability of 18 protein/phosphoproteins that comprise the known drug targets of neratinib (EGFR, HER2) and downstream effector molecules, such as SHC transforming protein 1 (SHC) and mammalian target of rapamycin (mTOR)/AK-thymoma (AKT) signaling components to predict complete pathologic response (pCR) to neratinib (). This study is the most comprehensive multi-omic investigation of the HER family pathway activation status and its relationship to HER family–targeted neoadjuvant therapy response in patients with early-stage breast cancer to date. Cell line–based preclinical studies have implicated alterations in HER/AKT/mTOR family genes on the DNA, mRNA, or protein level as predictive of neratinib response [Citation97–99]. As shown in LCM + RPPA reveals phosphorylation of the HER/PI3K/AKT pathway components as a predictive marker of Pathologic Complete Response in Patients with Breast Cancer Treated with Neratinib in the I-SPY 2 TRIAL. Following the unblinding of the data for a cohort of Neratinib treated (n = 193) and concurrent control patients (n = 78), six RPPA proteins measured prior to treatment were found to be highly predictive of pathologic complete response (pCR). All the highly predictive phosphoproteins were directly involved in downstream signaling activity emanating from the intracytoplasmic HER2 receptor domain, particularly at the points where signaling proteins docked with the receptor to control growth, survival, and invasion. As shown in the predictive phosphorylated epitopes are directly in line with the C-terminus end of the TK receptor, the known target of Neratinib. Importantly, as a single biomarker, dichotomized in an exploratory analysis using data from this trial, EGFR Y1173 appears to have a 93% probability of Phase III success for Neratinib, which exceeds that of the HR-/HER2+ graduating signature .
3. Imaging-guided LCM for multicellular and single cell spatial molecular profiling
The advantages of LCM are that it can sample and extract the biomolecules in situ within their native context for the full thickness of the tissue cells targeted for analysis, resulting in high sensitivity and high yield for precise microscopic regions down to a few microns. This advantage has made it possible, for example, in transcriptomics to interrogate moderate and low abundance transcripts comprising the bulk of the transcriptome for deep genomic analysis with next-generation sequencing (NGS) as a ground truth method in the Kidney Precision Medicine Project (KPMP) from single digit to less than one nanogram input of total microdissected mRNA for 20,000 genes coverage [Citation100]*; 100, 10, and single cell transcriptomic profiling for LCM-Seq [Citation43]; and 10 cell pooling (LCM 10 c-RNA-Seq) with inferred single-cell transcriptomics for biological insights into regulatory cell-states [Citation5, Citation43,Citation100, Citation101–103]. The high sensitivity of LCM makes it possible in proteomics to examine more than 300 kinase signaling pathway phosphoproteins within a cancer cell subpopulation comprising only 25% of a typical core needle biopsy while comparing the cancer cells with the host stroma and immune cell population. LCM-guided mass spectrometry (MS) methods are rapidly advancing for discovery applications from region of interest to single-cell resolution; and MS experts are beginning to realize the dream of robust high-yield LCM single-cell tissue proteomics from either the same thin-tissue section or precision registered serial sections from a variety of tissue types [Citation49,Citation104–113], high yield single-cell transfer to nanochip [Citation105], subcellular precision, and high throughput with ML assisted LCM and AI-driven [Citation106] deep visual proteomics with high sensitivity single-cell LCM and high-sensitivity LC MS [Citation49,Citation107–110].
While this review is about the exploding field of Laser Capture Proteomics (LCP), nowadays, LCP spatial profiling is optimal when integrated with orthogonal multiomics (e.g. epigenomics, genomics, and transcriptomics) and high-dimensional data analytics hereafter ‘LCM genomics’ (LCG) to profile mutational and transcriptomic data with proteomic and phosphoproteomic information in the same tissue subpopulation [Citation104,Citation111,Citation114] (See ). For example, a recent LCM study for spatioimageomics (SIO) integrates comparisons of gene regulatory pathways and high-plex, high-dimensional protein expression with Imaging Mass Cytometry (IMC) for prognostic biomarker analysis in high and low survival groups in ovarian cancer [Citation104]. Furthermore, LCG-based transcriptomics based on statistical methods of random sampling of 10 cell pools for RNA-Seq (10cRNA-Seq) with stochastic profiling unlocks inferred single-cell resolution for cell-state regulatory heterogeneity in cancer in situ without the dissociative artifacts of single-cell sequencing methods [101–103,115–117,121*]. LCM-based single-cell spatial genomic methods provide direct evidence, at the single-cell exome level, that subclonal populations of tumor cells preexist within the in-situ lesion of breast cancer that are the precursors of the invasive and metastatic subpopulations during the transition from in-situ to invasive cancer [Citation39]. LCM can be used to topographically map the mutations in subclonal populations in situ by individual clonal lineage most directly associated with a functional genomic driver gene at single-cell resolution. Making a functional connection between genome, transcriptome, and protein cell signaling, at multicellular and single-cell resolution, may have implications for improving precision medicine, regarding subclonal origins, precancer, cancer progression and metastasis or cellular signaling, and drug resistance [Citation5,Citation39,Citation43,Citation104,Citation114,Citation115].
Table 1. Highlight references that are driving the rapidly emerging field of LCM-based single-cell or 10-cell proteomics and multi-omics discoveries using microdissection tissue spatial profiling combined with specialized imaging and analysis software, Artificial Intelligence (AI), and advanced open-source protocols
3.1. Immuno-LCM
Immuno-LCM (Immuno-guided-LCM or label-assisted LCM) represents the use of a label or probe, typically an antibody, cocktail of antibodies (or oligonucleotide) that binds to a single antigen or multiple (multiplex) antigens (or hybridizes to) RNA, DNA, protein, or other target on the tissue surface to guide precision and accuracy of LCM usually for single-cells, sparse cells, or small cell populations with immunohistochemistry (IHC), immunofluorescence (IF), or in situ hybridization (ISH) in first-generation methods [Citation5,Citation49,Citation116]. Typically, a 5–20 um thin-tissue section mounted on a slide or membrane is labeled or probed (in lieu of histopathological staining or after de-staining) and imaged. A same-slide tissue section (the imaged reference slide) is often desired for molecular analysis, especially for single-cells, as serial sections can vary in thickness depending on the downstream molecular application. Today, high precision registration algorithms are available to align serial (adjacent) slides when dyes or labels interfere with downstream molecular analysis (e.g. proteomics studies) or if antigen retrieval steps for binding antibody degrade the quality of biomacromolecules or if insufficient tissue exists for molecular analysis. Custom protocols for antigen retrieval steps and preanalytical tissue processing steps for Immuno-LCM and other immuno-profiling spatial techniques are important to limit the percentage decline of biomacromolecules for DNA, RNA in the same slide tissue section [Citation43,Citation116], while precision registration algorithms in serial (adjacent) sections may be better suited for different applications in FFPEfor example, when prolonged antigen retrieval steps are required.
As these technical challenges are still being addressed for pairing immunostaining with LCM there is a unique opportunity on the horizon to graduate from first generation single antigen Immuno-LCM to second generation multiplex high-dimensional analysis for more sophisticated and intelligent targeting and sampling of tissue with immuno-LCM to correlate deep molecular omics and phosphoproteomic analysis [Citation25,Citation48,Citation106,Citation117]. AI-driven multiplex single-cell LCM with downstream high-sensitivity LC sample processing and MS in Deep Visual Proteomics (DVP) represents a recent demonstration of this advanced approach [Citation106]. Similarly, RareCyte Inc has introduced an automated mechanical microdissection using a 40-micron resolution robotic needle for downstream molecular analysis in conjunction with multiplex or high-plex immunofluorescence cyclical in situ hybridization with high parameter data analytics [Citation3,Citation58]. This integrated multiplex mechanical Immuno-microdissection model, similar to the advanced paradigm of LCM-based DVP [Citation106], demonstrates the feasibility and potential of emergent multiplex Immuno microdissection systems along with several popular commercial molecular spatial profiling (MSP) systems applying high-plex single-molecule imaging or in situ sequencing with integrated single-cell sequencing methods for in situ transcriptomic and proteomic expression profiling[3].
3.2. Coupling LCM proteomics with LCM genomics at the single cell level
Spatial Omics methods for DNA, RNA or proteins (e.g. epigenomics, spatial transcriptomics, and proteomics) measure biomolecules in thin-tissue sections are becoming highly sophisticated. 3D reconstruction methods seek to acquire in situ cell lineage diversity and regulatory cell states with multicellular, single, and subcellular resolution [Citation3,Citation45,Citation52–54,Citation101]. High sensitivity (e.g. depth of overall gene coverage and read alignment for exome or transcriptome NGS) and specificity (e.g. lack of false positives from amplification bias) within small multicellular populations and single-cells also represent a critical goal for accurately developing cell atlases or exploring deep regulatory cell states [Citation101–103]. Determining a spatial imaging method beyond performance (breadth and depth) also includes cellular throughput, destructive vs. nondestructive analysis (imaging or molecular analysis steps), cost, and turnaround time [Citation3,Citation45]. As numerous spatial methods are coevolving, tradeoffs exist for establishing the required breadth and depth of spatial interrogation for an appropriately powered study with high accuracy and reduction of pre-analytical variability to determine lineage and/or regulatory cell state heterogeneity (e.g. cancer cell states) [Citation101–103].
Laser Capture Microdissection (LCM) has been applied for the spatial analysis of both morphologically (e.g. H&E) or phenotypically (e.g. Immuno-LCM; see Immuno-LCM) homogeneous multicellular populations from thin-tissue sections and, to greater effectiveness over time, for single cell analysis[Citation43,Citation47,Citation52,Citation101,Citation102,Citation116]. Despite the microscopic appearance of homogeneous cells, at the single-cell level molecular expression patterns can be heterogeneous [Citation101,Citation102,Citation116,Citation118,Citation119]. This biologic truth has technical implications as the averaged ‘bulk’ genomic tissue analysis becomes the new average ‘bulk’ cellular genomic analysis. Therefore, maximizing the yield of DNA or RNA from single-cells or small groups of cells will be critical for achieving analytical accuracy and reproducibility of single-cell analysis with NGS methods. [Citation5,Citation43,Citation116]. Single-cell libraries for LCM-SEQ are a cost effective in situ and extraction free method for successful single cell transcriptomics with high accuracy and reproducibility in frozen tissue, are not as efficient as 10 cell populations, but reveal insights as shown in studies of Spinal Muscular Atrophy [Citation43,Citation120,Citation121]. LCM-Based methods have recently joined the race for single-cell spatial omics as single-cell resolution for genomics and transcriptomics bring the potential for elucidating single-cell heterogeneity of cell states forming an important dimension for understanding the basis of disease origins [Citation39,Citation43,Citation45,Citation103,Citation116]. LCM and Immuno-LCM of in situ microdissected 10 cell populations and random sampling of tissue with RNA-Seq (10cRNA-Seq) for spatial transcriptomics with stochastic profiling can provide deep overall gene coverage with inferred single-cell resolution for regulatory heterogeneities identifying low level expressed genes [Citation44,Citation101–103,Citation116,Citation118]. This is important due to the technical limitations of sequencing fewer than 10 cells using dissociative tissue-based single-cell sequencing (e.g. sparse data from RNA molecule dropout and low capture yield) compared to LCM-based 10cRNA-Seq with stochastic profiling for detection of inferred rare transcript expression of less than 2-3% of the randomly sampled population [Citation116,Citation117] [Citation103]. LCM-based 10cRNA-Seq with stochastic profiling has revealed novel genomic insights into regulatory heterogeneities in early breast, lung, and brain cancer cell states [Citation116,Citation118,Citation122–125]. The immediate tradeoff for stochastic profiling is lower throughput despite high transcriptomic accuracy for tissue sampling two-state heterogeneity within a cell lineage [Citation101,Citation102,Citation103,Citation108,Citation119,Citation120].
LCM-based spatial methods, including Topographic Single Cell Sequencing (TSCS) [Citation5,Citation39,Citation43,Citation45,Citation115] and Geo-seq have been recently developed for single-cell genomic and transcriptomic mapping of thin-tissue sections for 3D multi-omics profiling but lack very high throughput (10 s, 100 s, and 1000 s of thousands of cells) or rapid (hours vs. days) turnaround time for molecular and data analysis. A next step for high throughput single-cell mapping with LCM may be LCM-based semiautomated downstream microfluidics (LCM microfluidics) combining NGS and genomics on-chip methods (e.g. integrated fluidic circuits (IFCs) [Citation54,Citation126–128] [Citation100,Citation111,Citation114,Citation129,Citation130]. While some approaches can be imaging-free and purely computational at the genomics or omics level, it is difficult to translate this to a routine clinical pathologic assay because the pathologist needs to microscopically verify the correspondence of the computed image with an actual stained histologic section [Citation1].
4. Expert commentary
4.1. Laser capture microdissection systems aided by artificial intelligence for digital histopathology and biomarker discovery workflows
Optical, chemical, and molecular imaging systems aided by Artificial Intelligence (AI) and Laser (Capture) Microdissection (LCM) have the potential to transform digital histopathology and accelerate biomarker discovery. Imaging LCM systems represent widespread, often highly specialized hybrid platforms, including variants of label-free infrared (IR) spectroscopy with LCM (IR – LCM) [Citation50,Citation51,Citation112] and emerging Digital and Whole-Slide Imaging systems within the traditional realm of Optical Microscopy to guide downstream molecular analysis [Citation46,Citation48,Citation69,Citation129]. These next-generation hybrid imaging LCM systems have the potential to further enable state-of-the-art co-evolving downstream molecular and cellular analytical discovery platforms including omics, microfluidics, nanotechnology chip-based assays, and analytical chemistry, such as Mass Spectrometry (LCM–MS) in emergent imaging LCM systems. AI and Machine Learning (ML) provide a growing and pivotal role in these emerging imaging LCM systems for high-dimensional image analysis for the identification, segmentation, and classification of cells or region(s) of interest (ROI) of tissue as well as data analysis for downstream analytical applications including temporospatial molecular profiling [Citation46,Citation48,Citation58,Citation104,Citation106–108,Citation111–114,Citation129,Citation130] (). Deep learning (DL) models such as convolutional neural networks (CNNs) and generalized adversarial networks (GANs) can play a profound role in histopathology and molecular imaging systems for image analysis, image translation, and image transformation when integrated with other types of data, such as clinical and molecular data sets and between different images and imaging modalities, respectively. For example, DL algorithms have the potential to translate between types of tissue images including gold standard clinically stained thin-tissue sections or unstained and unlabeled tissue or cellular samples (e.g. Hematoxylin and Eosin (H & E), immunohistochemistry (IHC), immunofluorescence (IF) staining) as well as transformation across imaging modalities (e.g. holographic, quantitative phase imaging (QPI), IR, and Optical imaging). This can be extended to mapping between imaging spectral data or between spectral data and spatial data. For digital pathology, AI/ML algorithms are assisting histopathology for diagnostic, and prognostic applications with the potential to display subsequent spatially mapped omic data [Citation131]. Spatial imaging systems aided by DL/ML for segmentation and classification of single cell proteomic interrogation within the tissue microenvironmental data will utilize precise co-registration to retain the exact regions of interest mapped to the original histology [Citation131] (). Open-source AI and image analysis software compatible with remote LCM may enable widespread clinical and research multimodal imaging systems integrated with omics and data analytics in the cloud for digital diagnostic histopathology and biomarker discovery workflows ().
4.2. Digital pathology, the evolution of LCM tissue proteomics, and the translation to routine clinical utility
LCM and its sibling technologies continue to evolve closer to routine use in the clinic. As depicted in , near-term technologic advances emerging over the next few years will overcome many of the existing drawbacks of LCM (see section III). Chemistries for creating a liquid coverslip, followed by digital image capture, will overcome the poor resolution of viewing an open-faced tissue section necessary to acquire a full color high-resolution image of the exact same tissue that is being sampled. Instead of laboriously working under the microscope, a high-resolution full-section scanned histologic image will be automatically captured and registered in the Cloud. The operator will then view this image remotely on their desktop, tablet, or portable device. Expert system ML image analysis guidance can complement and supplement the digital image when it is viewed on-screen for marking up the regions of interest. After on-screen marking the areas of interest in interrogation, perhaps in further partnership with the AI image assistant, the operator will simply click ‘send’ and the selected regions will be automatically and remotely microdissected in a laboratory that may be across the building, or across the world. After a complete analysis of the procured regions of interest in proteomics and genomics in the remote laboratory, the encrypted results through the cloud will be displayed back on the digital screen image directly within the selected histologic regions of interest. Using cloud computing, artificial intelligence systems, digital histologic image capture, and on-screen reporting can improve turn-around time, engage expert pathologists, and capture spatially registered data that may be crucially important for personalized medicine of the future.
Figure 5. Digital pathology vision of the future. A diagnostic biopsy pathologic tissue section histologic image is digitally captured at high resolution, sent to the cloud, and then marked up remotely on a scientist or clinician’s computer screen (left panel). Once the specific histopathology regions are marked for interrogation on-screen they are remotely and automatically microdissected (right panel) and analyzed. Improved liquid cover slip chemistry and high resolution UV capture permit capture and analysis of the same tissue cells that are imaged at the microscopic level. Data from the molecular analysis of each selected region is then ported back through the cloud and can be viewed on-screen (left panel)
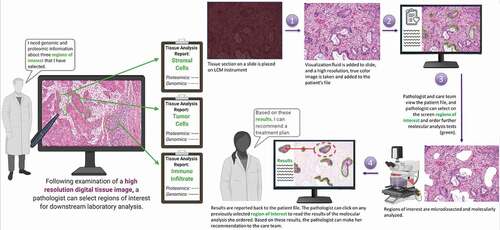
5. Expert opinion
5.1. Multiplexes tissue spatial molecular profiling of tissue will become an integral component of personalized medicine
Individualized therapy based on multiplex molecular profiling of biopsy tissue and cytology specimens is becoming the guiding principle of modern molecular oncology. Recognition of the limitations of whole-tissue extraction profiling has driven a rapidly expanding field of investigators who are creating technology, software, and analytical tools to explore the tissue cellular spatial interactome. Among the many existing technologies, Laser Capture Microdissection (LCM) is a reliable established tool, with many instrument types, and downstream analysis methods, to choose from. LCM is widely used to study the spatial proteomic signatures of individual cell populations within a complex heterogeneous tissue biopsy. The advantage of LCM is that it can sample and extract the molecules within the full thickness of the tissue cells targeted for analysis resulting in high sensitivity and high yield for precise microscopic regions down to a few microns.
A particular strength of LCM is the spatially resolved quantitative multiplex measurement of protein post-translational modifications (PTMs) revealing the activated functional state of tissue cell signaling pathways and proteins, such as tumor cell MHC-II [Citation132] that cannot be discerned by transcriptomics [Citation133]. As cancer therapies shift beyond those that directly target the tumor cells, and immunotherapy approaches continue to grow, specific molecular interrogation of tissue immune cells and classes of host stroma cells will be required for selecting the right treatment for each patient. More than 300 kinase signaling pathway phosphoproteins can quantified within a cancer cell subpopulation comprising only 25% of a typical core needle biopsy, while comparing the cancer cells with the adjacent host stroma and immune cell population. LCM proteomics combined with genomics have been used to study the same subpopulation of tissue cells to explore the genetic drivers of the tissue cellular phenotype. This advantage of LCM combined ‘omics’ has made it possible, for example, to deeply interrogate low abundance signal proteins and low abundance transcripts comprising the bulk of the transcriptome with inferred single-cell resolution to assess novel insights into gene regulatory cell-state heterogeneities. LCM combined with multiplex protein PTM quantification has led to the discovery of a small number of key phosphoproteins that are integral to the function of kinase networks (e.g. ERBB2 growth and Akt pro-survival) that are important therapeutic targets for kinase inhibitors. LCM combined with PTM phosphoprotein epitope measurement conducted in microdissected HER2 positive cancer cells is highly predictive of pathologic complete remission (pCR), independent of the HER2 inhibitor modality. LCM PTM HER2 activation status has significant advantages compared to previous predictors because the test is conducted on the pretreatment biopsy and directly evaluates the molecular target of the major classes of HER2 neoadjuvant therapies.
5.2. Overcoming challenges for future routine clinical Implementation
There are many challenges and questions to overcome if we hope to realize the vision we have presented above: 1) How can multi-omics special tissue data be generated in a timely manner and presented to the treating physician in a meaningful way? 2) How do we design clinical trials to test the superiority of the new technology compared to existing molecular pathology workflows? 3) How do we ensure that the tissue being analyzed is an accurate representative of the lesion? 4) How can we standardize and normalize cutoff levels for proteomic, and genomic results in a manner that can be shared across clinical labs? 5) How do we educate researchers about the requirements needed for full implementation of a proteomic or genomic test under CAP (College of American Pathologists) CLIA laboratory certification?
While there are many positive attributes of tissue spatial profiling technologies, including LCM, these technologies will remain as research tools until they can be shown to be rapid, easy to use, reliable, and sensitive, in the busy clinical arena. Nevertheless, even as sensitivity, speed, and accuracy improve, it will be increasingly important to show utility for key clinical decision-making that can benefit a therapeutic outcome or reduce unwarranted toxicity for a defined patient's intended population.
As investigators attempt to address these challenges for future translational research, they have to keep several principles in mind. A first guiding principle is to develop the processing protocols, blinded validation study sets, proficiency assurance plan, and patient report format under CAP compliance and certification. A second guiding principle is the engagement of the diagnostic pathologist up-front to select the tissue section(s) for molecular profiling that is most representative of the lesion. A third guiding principle is independent blinded validation of cut-points between the bioinformatic core experts of different academic/clinical hospital institutions. This is currently a component of several ongoing collaborations between academic centers who are specifically studying LCM RPPA phosphoprotein predictors of neoadjuvant therapies that achieve a complete pathologic remission. A final critical guiding principle is clear and concise communication to the treating physician. Fortunately, CAP certified diagnostic companies, have adopted the LCM RPPA technology to offer a panel of phosphoprotein signal pathway measurements from microdissected breast cancer cells. For early clinical adopters, the reporting format scales the individual marker levels in a simple chart that compares the patient data to the reference population for the relevant intended use. Regarding blinded clinical trial validation and verification, the ongoing I-SPY breast cancer trials currently employ LCM RPPA proteomics and genomics to identify neoadjuvant therapy biomarkers, and rigorously statistically assess endpoint cut points, for potential predictors of therapeutic outcome [Citation31,Citation33,Citation37]. The I-SPY trials, a model for biomarker verification, have reported that LCM RPPA measurements of breast cancer biopsies can be turned-around in less than 14 days.
Digital pathology in the future can incorporate emerging generations of LCM and other spatial tissue profiling technologies. A diagnostic biopsy pathologic tissue section histologic image is digitally captured at high resolution, sent to the internet cloud, and then marked up remotely on a scientist or clinician’s computer screen. Once the specific histopathology regions are marked for interrogation on-screen (including groups of cells, single cells, immune cells, stromal cells, etc.), these regions are automatically and remotely microdissected, followed by proteomic, mutational, and transcriptomic evaluation. The data from the molecular analysis of each selected region is then ported back through the cloud and can be viewed on-screen as a separate laboratory report for each individual histologic region that is highlighted.
Article highlights
Laser Capture Microdissection (LCM) is a reliable tool, with many instrument types, and downstream analysis methods, to choose from. It is widely used to study the spatial molecular signatures of individual cell populations within a complex heterogeneous tissue biopsy. The choice of cell types to be captured and analyzed is usually done by direct visual microscopic histologic analysis. Nevertheless, digital image capture, A.I./machine learning, telepathology, and continued automation will play a future role in making LCM more generally acceptable to both basic scientists and anatomic clinical pathologists. This will include remote viewing of the histologic image with increasingly sophisticated AI-driven H&E and immuno-guided LCM for deep tissue and cellular genomic, transcriptomic, and proteomic single-cell resolution and 2D/3D/4D mapping.
The advantage of LCM is that it can sample and extract the molecules within the full thickness of the tissue cells targeted for analysis, resulting in high sensitivity and high yield for precise microscopic regions down to a few microns. A particular strength of LCM is the spatially resolved quantitative multiplex measurement of protein post-translational modifications (PTMs) revealing the activated functional state of tissue cell signaling pathways. More than 300 kinase signaling pathway phosphoproteins can quantify within a cancer cell subpopulation comprising only 25% of a typical core needle biopsy while comparing the cancer cells with the adjacent host stroma and immune cell population. LCM combined with protein microarrays (RPPA: Reverse Phase Protein Microarrays) can reliably and reproducibly map genomic and transcriptomic alterations and the activated state of entire kinase signaling pathways, from the cell receptor to the nucleus, within tumor, or host, tissue cell subpopulations, and single cells, inside a typical cancer biopsy. This approach has led to the discovery of a small number of key phosphoproteins that are integral to the function of kinase networks (e.g. ERBB2 growth and Akt pro-survival) that are important therapeutic targets for kinase inhibitors.
LCM proteomics combined with genomics have been used to study the same subpopulation of tissue cells to explore the genetic drivers of the tissue cellular phenotype. This advantage of LCM combined ‘omics’ has made it possible, for example, to deeply interrogate low abundance signal proteins and low abundance transcripts comprising the bulk of the transcriptome with inferred single-cell resolution to assess novel insights into gene regulatory cell-state heterogeneities. LCM-guided mass spectrometry (MS) methods are rapidly advancing for discovery applications from region of interest to single-cell resolution; and MS experts are predicting that the dream of robust high-yield LCM single-cell tissue proteomics will be realized from the same section thin-tissue section and from a variety of tissue types.
We envisage near-term future workflows for the technology in which a diagnostic biopsy pathologic tissue section histologic image is digitally captured at high resolution, sent to the internet cloud, and then marked up remotely on a scientist or clinician’s computer screen. Once the specific histopathology regions are marked for interrogation on-screen (including groups of cells, single cells, immune cells, stromal cells, etc.), these regions are automatically and remotely microdissected by LCM, followed by proteomic, genome sequencing, and mRNA transcriptomic evaluation. The data from the molecular analysis of each selected region is then ported back through the cloud and can be viewed on-screen as a separate laboratory report for each individual histologic region that is highlighted, for integrated clinical and molecular data analysis.
Author contributions
All authors have made substantial contribution to the writing, editing, data production, or revision of this article. Authors’ specific contributions are delineated below.
Liotta: conception, data analysis, figure design, writing of the manuscript
Pappalardo: literature synthesis, conception, writing of the manuscript
Carpino: LCM technology history and future vendor products
Haymond: personalized medicine conception and generation of figures
Pierobon: LCM applied to tumor immunotherapy proteins
Howard: digital LCM and protein microarrays
Espina: LCM protocols and translation to CAP CLIA
Wulfkuhle: LCM phosphoprotein profiling to guide breast cancer neoadjuvant therapy
Petricoin: ISPY trial and personalized medicine based on LCM phosphoproteomics
Declaration of interests
E. Petricoin, L. Liotta, and V. Espina provide consulting advice to Theralink Technologies Inc
L. Liotta, V. Espina, E. Petricoin, M. Howard, P.A. Pappalardo, A. Haymond, M. Pierobon and J.D. Wulfkuhle can receive patent royalties related to the topics in this article, exclusively from NIH-owned and University-owned patents.
A. Carpino is an employee of Fluidigm.
The authors have no other relevant affiliations or financial involvement with any organization or entity with a financial interest in or financial conflict with the subject matter or materials discussed in the manuscript apart from those disclosed.
Additional information
Funding
References
- Schede HH, Schneider CG, Stergiadou J, et al. Spatial tissue profiling by imaging-free molecular tomography. bioRxiv. 2020.DOI: https://doi.org/10.1101/2020.08.04.235655 BIORxiv
- Bassiouni R, Gibbs LD, Craig DW, et al. Applicability of spatial transcriptional profiling to cancer research. Mol Cell. 2021;81(8):1631–1639.
- Asp M, Bergenstråhle J, Lundeberg J. Spatially resolved transcriptomes—Next generation tools for tissue exploration. BioEssays. 2020;42(10):1900221.
- Herrera JA, Mallikarjun V, Rosini S, et al. Laser capture microdissection coupled mass spectrometry (LCM-MS) for spatially resolved analysis of formalin-fixed and stained human lung tissues. Clin Proteomics. 2020;17(1):24.
- Nichterwitz S, Benitez JA, Hoogstraaten R, et al. LCM-Seq: a method for spatial transcriptomic profiling using laser capture microdissection coupled with PolyA-Based RNA sequencing.In: Gaspar I, editor. RNA Detect [Internet]. New York: Springer New York; 2018 [cited 2021 Jun 14]. p. 95–110. Available from:
- Emmert-Buck MR, Bonner RF, Smith PD, et al. Laser Capture Microdissection. Science. 1996;274(5289):998–1001.
- Pin E, Stratton S, Belluco C, et al. A pilot study exploring the molecular architecture of the tumor microenvironment in human prostate cancer using laser capture microdissection and reverse phase protein microarray. Mol Oncol. 2016;10(10):1585–1594.
- Chiechi A, Novello C, Magagnoli G, et al. Elevated TNFR1 and serotonin in bone metastasis are correlated with poor survival following bone metastasis diagnosis for both Carcinoma and sarcoma primary tumors. Clin Cancer Res. 2013;19(9):2473–2485.
- Einspahr JG, Calvert V, Alberts DS, et al. Functional protein pathway activation mapping of the progression of normal skin to squamous cell Carcinoma. Cancer Prev Res (Phila). 2012;5(3):403–413.
- Munsamy T, Bouwer G. Determination of the virulence of single nucleopolyhedrovirus occlusion bodies using a novel laser capture microdissection method. J Gen Virol. 2020;101(12):1300–1304.
- George P, Sharma A, Sharakhov IV. 2D and 3D chromosome painting in malaria mosquitoes. J Vis Exp. 2014;83:51173. DOI: https://doi.org/10.3791/51173
- Iyer EPR, Cox DN. Laser Capture Microdissection of drosophila peripheral neurons. J Vis Exp. 2010;39:2016. DOI: https://doi.org/10.3791/2016
- Ando A, Kirkbride RC, Jones DC, et al. LCM and RNA-seq analyses revealed roles of cell cycle and translational regulation and homoeolog expression bias in cotton fiber cell initiation. BMC Genomics. 2021;22(1):309.
- Gautam V, Chatterjee S, Sarkar AK. Single cell type specific RNA isolation and gene expression analysis in rice using Laser Capture Microdissection (LCM)-Based method. In Bandyopadhyay A, Thilmony R, editors. Rice Genome Eng Gene Ed [Internet]. New York (NY): Springer US; 2021 [cited 2021 Aug 3]. p. 275–283. Available from:
- Santegoeds RGC, Yakkioui Y, Jahanshahi A, et al. Notochord isolation using laser capture microdissection. J Chem Neuroanat. 2017;80:37–43.
- Greene RM, Smolenkova I, Pisano M. Laser capture microdissection of Murine Embryonic neural crest cells. In: Schwarz Q, Wiszniak S, editors. Neural crest cells [Internet]. New York: Springer New York; 2019 [cited 2021 Jun 14]. p. 121–133. Available from:
- Cefalo CMA, Mezza T, Giaccari A, et al. A systematic comparison of protocols for recovery of high-quality RNA from human islets extracted by Laser Capture Microdissection. Biomolecules. 2021;11(5):625.
- Nyalwidhe JO, Grzesik WJ, Burch TC, et al. Comparative quantitative proteomic analysis of disease stratified laser captured microdissected human islets identifies proteins and pathways potentially related to type 1 diabetes. PLOS ONE. 2017;12(9):e0183908. In Pietropaolo M, editor.
- Zhang L, Lanzoni G, Battarra M, et al. Label-Free LC-MS/MS strategy for comprehensive proteomic profiling of human islets collected using laser capture microdissection from frozen pancreata.In: Wang X, Kuruc M, editors. Funct Proteomics [Internet]. New York: Springer New York; 2019 [cited 2021 Jun 14]. p. 253–264. Available from:
- Mollee P, Boros S, Loo D, et al. Implementation and evaluation of amyloidosis subtyping by laser-capture microdissection and tandem mass spectrometry. Clin Proteomics. 2016;13(1):30.
- Datta S, Malhotra L, Dickerson R, et al. Laser capture microdissection: big data from small samples. Histol Histopathol. 2015;30:1255–1269.
- Macdonald JA, Murugesan N, Pachter JS. Endothelial cell heterogeneity of blood-brain barrier gene expression along the cerebral microvasculature. J Neurosci Res. 2009;NA–NA. DOI: https://doi.org/10.1002/jnr.22316
- Song HW, Foreman KL, Gastfriend BD, et al. Transcriptomic comparison of human and mouse brain microvessels. Sci Rep. 2020;10(1):12358.
- Hudock TA, Kaushal D. A novel microdissection approach to recovering mycobacterium tuberculosis specific transcripts from formalin fixed paraffin embedded lung granulomas. J Vis Exp. 2014;88:51693. DOI: https://doi.org/10.3791/51693.
- Zimmerman M, Blanc L, Chen P-Y, et al. Spatial quantification of drugs in pulmonary tuberculosis Lesions by Laser Capture Microdissection Liquid Chromatography Mass Spectrometry (LCM-LC/MS). J Vis Exp. 2018;88:57402. DOI: https://doi.org/10.3791/51693
- Yang X, Song Y, Liang T, et al. Application of laser capture microdissection and PCR sequencing in the diagnosis of Coccidioides spp. infection: a case report and literature review in China. Emerg Microbes Infect. 2021;10(1):331–341.
- Chae YK, Gagliato D de M, Pai SG, et al. The Association between EGFR and cMET expression and phosphorylation and its prognostic implication in patients with breast cancer. PloS One. 2016;11(4):e0152585.
- Espina V, Edmiston KH, Heiby M, et al. A portrait of tissue phosphoprotein stability in the clinical tissue procurement process. Mol Cell Proteomics MCP. 2008;7(10):1998–2018.
- Mnatsakanyan R, Shema G, Basik M, et al. Detecting post-translational modification signatures as potential biomarkers in clinical mass spectrometry. Expert Rev Proteomics. 2018;15(6):515–535.
- Hunt AL, Bateman NW, Barakat W, Extensive intratumor proteogenomic heterogeneity revealed by multiregion sampling in high-grade serous Ovarian tumor specimens. bioRxiv. 2021;24(7):102757. DOI: https://doi.org/10.1016/j.isci.2021.102757.eCollection 2021 Jul 23.
- Wulfkuhle JD, Yau C, Wolf DM, et al. Evaluation of the HER/PI3K/AKT Family signaling network as a predictive biomarker of pathologic complete response for patients with breast cancer treated with neratinib in the I-SPY 2 TRIAL. JCO Precis Oncol. 2018 Aug 16;2:PO.18.00024. doi:https://doi.org/10.1200/PO.18.00024. eCollection 2018. Online only.
- Wulfkuhle JD, Yau C, Wolf DM, et al. Abstract P3-07-48: prediction of complete pathologic response to veliparib/carboplatin plus standard neoadjuvant therapy in HER2 negative breast cancer: exploratory protein pathway marker results from the I-SPY 2 trial. Cancer Res. 2016;76: P3-P3-07–48.
- Wolf DM, Yau C, Wulfkuhle J, et al. Mechanism of action biomarkers predicting response to AKT inhibition in the I-SPY 2 breast cancer trial. Npj Breast Cancer. 2020;6(1):1–8.
- Pierobon M, Ramos C, Wong S, et al. Enrichment of PI3K-AKT–mTOR pathway activation in hepatic metastases from breast cancer. Clin Cancer Res Off J Am Assoc Cancer Res. 2017;23(16):4919–4928.
- I-SPY 2 TRIAL Investigators, Wolf DM, Yau C, Wulfkuhle J, et al. Mechanism of action biomarkers predicting response to AKT inhibition in the I-SPY 2 breast cancer trial. Npj Breast Cancer. 2020;6(1):48.
- Cheng PF, Dummer R, Levesque MP. Data mining The Cancer Genome Atlas in the era of precision cancer medicine. Swiss Med Wkly. 2015;145:w14183.
- Barker AD, Sigman CC, Kelloff GJ, et al. I-SPY 2: an adaptive breast cancer trial design in the setting of neoadjuvant chemotherapy. Clin Pharmacol Ther. 2009;86(1):97–100.
- Petricoin EF, Bichsel VE, Calvert VS, et al. Mapping molecular networks using proteomics: a vision for patient-tailored combination therapy. J Clin Oncol Off J Am Soc Clin Oncol. 2005;23(15):3614–3621.
- Casasent AK, Schalck A, Gao R, et al. Multiclonal invasion in breast tumors identified by topographic single cell sequencing. Cell. 2018;172(1–2):205–217.e12.
- Bonner RF. CELL SAMPLING: Laser Capture Microdissection: molecular analysis of tissue. Science. 1997;278(5342):1481–1483.
- Simone NL, Bonner RF, Gillespie JW, et al. Laser-capture microdissection: opening the microscopic frontier to molecular analysis. Trends Genet. 1998;14(7):272–276.
- Gallagher RI, Blakely SR, Liotta LA, et al. Laser Capture Microdissection: arcturusxt infrared capture and UV cutting methods.In: Espina V, Liotta LA, editors. Mol profiling [Internet]. Totowa (NJ): Humana Press; 2012 [cited 2021 Jun 14]. p. 157–178. Available from:
- Nichterwitz S, Chen G, Aguila Benitez J, et al. Laser capture microscopy coupled with Smart-seq2 for precise spatial transcriptomic profiling. Nat Commun. 2016;7(1):12139.
- Amrhein L, Fuchs C. stochprofML: stochastic profiling using maximum likelihood estimation in R. BMC Bioinformatics. 2021;22(1):123.
- Andersson A, Bergenstråhle J, Asp M, et al. Single-cell and spatial transcriptomics enables probabilistic inference of cell type topography. Commun Biol. 2020;3(1):565.
- Brasko C, Smith K, Molnar C, et al. Intelligent image-based in situ single-cell isolation. Nat Commun. 2018;9(1):226.
- Angelidis I, Simon LM, Fernandez IE, et al. An atlas of the aging lung mapped by single cell transcriptomics and deep tissue proteomics. Nat Commun. 2019;10(1):963.
- Hipp J, Johann D, Chen Y, et al. Computer-aided laser dissection: a microdissection workflow leveraging image analysis tools. J Pathol Inform. 2018;9(1):45.
- Huang P, Kong Q, Gao W, et al. Spatial proteome profiling by immunohistochemistry-based laser capture microdissection and data-independent acquisition proteomics. Anal Chim Acta. 2020;1127:140–148.
- Großerueschkamp F, Gerwert K. Label-free digital pathology by infrared imaging. Biomed Spectrosc Imaging. 2020;9(1–2):5–12.
- Schnell M, Mittal S, Falahkheirkhah K, et al. All-digital histopathology by infrared-optical hybrid microscopy. Proc Natl Acad Sci. 2020;117(7):3388–3396.
- Wang N, Wang R, Zhang X, et al. Spatially-resolved proteomics and transcriptomics: an emerging digital spatial profiling approach for tumor microenvironment. Vis Cancer Med. 2021;2:1.
- Toki MI, Merritt CR, Wong PF, et al. High-Plex predictive marker discovery for melanoma immunotherapy-treated patients using digital spatial profiling. Clin Cancer Res Off J Am Assoc Cancer Res. 2019;25(18):5503–5512.
- Lamanna J, Scott EY, Edwards HS, et al. Digital microfluidic isolation of single cells for -Omics. Nat Commun. 2020;11(10):5632.
- Akbani R, Becker KF, Carragher N, et al. Realizing the promise of reverse phase protein arrays for clinical, translational, and basic research: a workshop report the RPPA (Reverse Phase Protein Array) Society. Mol Cell Proteomics. 2014;13(7):1625–1643.
- Petricoin E, Wulfkuhle J, Howard M, et al. RPPA: origins, transition to a validated clinical research tool, and next generations of the technology. In: Yamada T, Nishizuka SS, Mills GB, et al., editors. Reverse Phase Protein Arrays [Internet]. Singapore: Springer Singapore; 2019 [cited 2021 Jun 20]. p. 1–19. Available from:
- Martinez-Morilla S, Villarroel-Espindola F, Wong PF, et al. Biomarker discovery in patients with immunotherapy-treated melanoma with imaging mass cytometry. Clin Cancer Res. 2021;27(7):1987–1996.
- Maliga Z, Nirmal AJ, Ericson NG, et al. Micro-region transcriptomics of fixed human tissue using Pick-Seq [Internet]. Cancer Biology; 2021 [cited 2021 Jun 14]. Available from: .
- Negm OH, Muftah AA, Aleskandarany MA, et al. Clinical utility of reverse phase protein array for molecular classification of breast cancer. Breast Cancer Res Treat. 2016;155(1):25–35.
- Schürch CM, Bhate SS, Barlow GL, et al. Coordinated cellular neighborhoods orchestrate antitumoral immunity at the colorectal cancer invasive front. Cell. 2020;182(1341–1359.e19):1341–1359.e19.
- Panda A, Betigeri A, Subramanian K, et al. Identifying a clinically applicable mutational burden threshold as a potential biomarker of response to immune checkpoint therapy in solid tumors. JCO Precis Oncol. 2017;1–13. DOI: https://doi.org/10.1200/PO.17.00146
- Panda A, de Cubas AA, Stein M, et al. Endogenous retrovirus expression is associated with response to immune checkpoint blockade in clear cell renal cell carcinoma. JCI Insight. 2018;3(16). DOI:https://doi.org/10.1172/jci.insight.121522.
- Locy H, de Mey S, de Mey W, et al. Immunomodulation of the tumor microenvironment: Turn foe into friend. Front Immunol. 2018;9:2909.
- Dickinson SE, Khawam M, Kirschnerova V, et al. Increased PD‐L1 expression in human skin acutely and chronically exposed to UV irradiation. Photochem Photobiol. 2021;97(4):778–784.
- Pierobon M, Hodge KA, Baldelli E, et al. Abstract 3129: PD-L1 expression differs across cancer metastatic sites from breast tumors. Tumor Biol [Internet]. American Association for Cancer Research; 2018 [cited 2021 Aug 2]. p. 3129–3129. Available from: .
- Pierobon M, Baldelli E, Hodge KA, et al. Development of a quantitative PD-L1 assay using laser capture microdissection (LCM)-based reverse phase protein microarray (RPPA) workflow: implications for precision medicine. J Clin Oncol. 2018;36(5_suppl): 35–35. DOI:https://doi.org/10.1200/JCO.2018.36.5_suppl.35.
- Baldelli E, Calvert V, Hodge KA, et al. Abstract 5656: quantitative measurement of PDL1 expression across tumor types using laser capture microdissection and reverse phase protein microarray. Immunology [Internet]. American Association for Cancer Research; 2017 [cited 2021 Aug 2]. p. 5656–5656. Available from: .
- Hunt AL, Pierobon M, Baldelli E, et al. The impact of ultraviolet- and infrared-based laser microdissection technology on phosphoprotein detection in the laser microdissection-reverse phase protein array workflow. Clin Proteomics. 2020;17(1):9.
- Balis U, Hipp J, Cheng J, et al. SIVQ-aided laser capture microdissection: a tool for high-throughput expression profiling. J Pathol Inform. 2011;2(1):19.
- Harbeck N. Emerging strategies in neoadjuvant treatment of patients with HER2-positive early breast cancer. Breast Edinb Scotl. 2019;48(Suppl 1):S97–S102.
- Bartsch R, Bergen E, Galid A. Current concepts and future directions in neoadjuvant chemotherapy of breast cancer. Memo. 2018;11(3):199–203.
- Nekljudova V, Loibl S, von Minckwitz G, et al. Trial-level prediction of long-term outcome based on pathologic complete response (pCR) after neoadjuvant chemotherapy for early-stage breast cancer (EBC). Contemp Clin Trials. 2018;71:194–198.
- von Minckwitz G, Huang C-S, Mano MS, et al. Trastuzumab emtansine for residual invasive HER2-Positive breast cancer. N Engl J Med. 2019;380(7):617–628.
- Gianni L, Pienkowski T, Im Y-H, et al. Efficacy and safety of neoadjuvant pertuzumab and trastuzumab in women with locally advanced, inflammatory, or early HER2-positive breast cancer (NeoSphere): a randomised multicentre, open-label, phase 2 trial. Lancet Oncol. 2012;13(1):25–32.
- Ditsch N, Untch M, Kolberg-Liedtke C, et al. AGO recommendations for the diagnosis and treatment of patients with locally advanced and metastatic breast cancer: update 2020. Breast Care. 2020;15(3):294–309.
- Cortazar P, Zhang L, Untch M, et al. Pathological complete response and long-term clinical benefit in breast cancer: the CTNeoBC pooled analysis. Lancet Lond Engl. 2014;384(9938):164–172.
- Schneeweiss A, Chia S, Hickish T, et al. Pertuzumab plus trastuzumab in combination with standard neoadjuvant anthracycline-containing and anthracycline-free chemotherapy regimens in patients with HER2-positive early breast cancer: a randomized phase II cardiac safety study (TRYPHAENA). Ann Oncol Off J Eur Soc Med Oncol. 2013;24(9):2278–2284.
- Gradishar WJ. HER2 therapy — an abundance of riches. N Engl J Med. 2012;366(2):176–178.
- Hayes DF, Phimister EG. HER2 and breast cancer - a phenomenal success story. N Engl J Med. 2019;381(13):1284–1286.
- Goutsouliak K, Veeraraghavan J, Sethunath V, et al. Towards personalized treatment for early stage HER2-positive breast cancer. Nat Rev Clin Oncol. 2020;17(4):233–250.
- Dieci MV, Vernaci G, Guarneri V. Escalation and de-escalation in HER2 positive early breast cancer. Curr Opin Oncol. 2019;31(1):35–42.
- Guarneri V, Dieci MV, Bisagni G, et al. De-escalated therapy for HR+/HER2+ breast cancer patients with Ki67 response after 2-week letrozole: results of the PerELISA neoadjuvant study. Ann Oncol Off J Eur Soc Med Oncol. 2019;30(6):921–926.
- Akimoto E, Kadoya T, Kajitani K, et al. Role of 18 F-PET/CT in predicting prognosis of patients with breast cancer after neoadjuvant chemotherapy. Clin Breast Cancer. 2018;18(1):45–52.
- van Ramshorst MS, Loo CE, Groen EJ, et al. MRI predicts pathologic complete response in HER2-positive breast cancer after neoadjuvant chemotherapy. Breast Cancer Res Treat. 2017;164(1):99–106.
- Gebhart G, Gámez C, Holmes E, et al. 18 F-FDG PET/CT for early prediction of response to neoadjuvant Lapatinib, Trastuzumab, and their combination in HER2-positive breast cancer: results from Neo-ALTTO. J Nucl Med. 2013;54(11):1862–1868.
- Nitz UA, Gluz O, Christgen M, et al. De-escalation strategies in HER2-positive early breast cancer (EBC): final analysis of the WSG-ADAPT HER2+/HR- phase II trial: efficacy, safety, and predictive markers for 12 weeks of neoadjuvant dual blockade with trastuzumab and pertuzumab ± weekly paclitaxel. Ann Oncol Off J Eur Soc Med Oncol. 2017;28:2768–2772.
- DiGiovanna MP, Stern DF, Edgerton SM, et al. Relationship of epidermal growth factor receptor expression to ErbB-2 signaling activity and prognosis in breast cancer patients. J Clin Oncol Off J Am Soc Clin Oncol. 2005;23(6):1152–1160.
- Cicenas J, Urban P, Küng W, et al. Phosphorylation of tyrosine 1248-ERBB2 measured by chemiluminescence-linked immunoassay is an independent predictor of poor prognosis in primary breast cancer patients. Eur J Cancer. 2006;42(5):636–645.
- Frogne T, Laenkholm A-V, Lyng MB, et al. Determination of HER2 phosphorylation at tyrosine 1221/1222 improves prediction of poor survival for breast cancer patients with hormone receptor-positive tumors. Breast Cancer Res. 2009;11(1):R11.
- Thor AD, Liu S, Edgerton S, et al. Activation (tyrosine phosphorylation) of ErbB-2 (HER-2/neu): a study of incidence and correlation with outcome in breast cancer. J Clin Oncol Off J Am Soc Clin Oncol. 2000;18(18):3230–3239.
- Hudelist G, Köstler WJ, Czerwenka K, et al. Her-2/neu and EGFR tyrosine kinase activation predict the efficacy of trastuzumab-based therapy in patients with metastatic breast cancer. Int J Cancer. 2006;118(5):1126–1134.
- Jameson GS, Petricoin EF, Sachdev J, et al. A pilot study utilizing multi-omic molecular profiling to find potential targets and select individualized treatments for patients with previously treated metastatic breast cancer. Breast Cancer Res Treat. 2014;147(3):579–588.
- Sereni MI, Baldelli E, Gambara G, et al. Kinase-driven metabolic signalling as a predictor of response to carboplatin–paclitaxel adjuvant treatment in advanced ovarian cancers. Br J Cancer. 2017;117(4):494–502.
- Gordon MS, Matei D, Aghajanian C, et al. Clinical activity of pertuzumab (rhuMAb 2C4), a HER dimerization inhibitor, in advanced ovarian cancer: potential predictive relationship with tumor HER2 activation status. J Clin Oncol. 2006;24(26):4324–4332.
- Lee AV, Cui X, Oesterreich S. Cross-talk among estrogen receptor, epidermal growth factor, and insulin-like growth factor signaling in breast cancer. Clin Cancer Res Off J Am Assoc Cancer Res. 2001;7:4429s–4435s. discussion 4411s-4412s.
- Ahmad DAJ, Negm OH, Alabdullah ML, et al. Clinicopathological and prognostic significance of mitogen-activated protein kinases (MAPK) in breast cancers. Breast Cancer Res Treat. 2016;159(3):457–467.
- Mullooly M, Conklin D, McGowan PM, et al. Neratinib to inhibit the growth of triple-negative breast cancer cells. J Clin Oncol. 2015;33(15_suppl): 1099–1099. DOI: https://doi.org/10.1200/jco.2015.33.15_suppl.1099.
- Park JW, Liu MC, Yee D, et al. Adaptive randomization of neratinib in early breast cancer. N Engl J Med. 2016;375(1):11–22.
- Bose R, Kavuri SM, Searleman AC, et al. Activating HER2 mutations in HER2 gene amplification negative breast cancer. Cancer Discov. 2013;3(2):224–237.
- Barwinska D, Ferkowicz MJ, Cheng Y-H, et al. Application of laser microdissection to uncover regional transcriptomics in human kidney tissue. J Vis Exp. 2020;61371. DOI: https://doi.org/10.3791/61371.
- Janes KA. Single-cell states versus single-cell atlases — two classes of heterogeneity that differ in meaning and method. Curr Opin Biotechnol. 2016;39:120–125.
- Janes KA, Wang -C-C, Holmberg KJ, et al. Identifying single-cell molecular programs by stochastic profiling. Nat Methods. 2010;7(4):311–317.
- Bajikar SS, Fuchs C, Roller A, et al. Parameterizing cell-to-cell regulatory heterogeneities via stochastic transcriptional profiles. Proc Natl Acad Sci. 2014;111(5):E626–E635.
- Zhu Y, Ferri-Borgogno S, Sheng J, et al. SIO: a spatioimageomics pipeline to identify prognostic biomarkers associated with the ovarian tumor microenvironment. Cancers (Basel). 2021;13(8):1777.
- Zhu Y, Dou M, Piehowski PD, et al. Spatially resolved proteome mapping of Laser Capture Microdissected tissue with automated sample transfer to nanodroplets. Mol Cell Proteomics. 2018;17(9):1864–1874.
- Mund A, Coscia F, Hollandi R, et al. AI-driven deep visual proteomics defines cell identity and heterogeneity [Internet]. Systems Biology; 2021 [cited 2021 Jun 14]. Available from: .
- Ezzoukhry Z, Henriet E, Cordelières FP, et al. Combining laser capture microdissection and proteomics reveals an active translation machinery controlling invadosome formation. Nat Commun. 2018;9(1):2031.
- Dewez F, Martin-Lorenzo M, Herfs M, et al. Precise co-registration of mass spectrometry imaging, histology, and laser microdissection-based omics. Anal Bioanal Chem. 2019;411(22):5647–5653.
- Davis S, Scott C, Ansorge O, et al. Development of a sensitive, scalable method for spatial, cell-type-resolved proteomics of the human brain. J Proteome Res. 2019;18(4):1787–1795.
- Xu R, Tang J, Deng Q, et al. Spatial-Resolution cell type proteome profiling of cancer tissue by fully integrated proteomics technology. Anal Chem. 2018;90(9):5879–5886.
- Sigdel TK, Piehowski PD, Roy S, et al. Near-Single-Cell proteomics profiling of the proximal tubular and glomerulus of the normal human kidney. Front Med. 2020;7:499.
- Großerueschkamp F, Bracht T, Diehl HC, et al. Spatial and molecular resolution of diffuse malignant mesothelioma heterogeneity by integrating label-free FTIR imaging, laser capture microdissection and proteomics. Sci Rep. 2017;7(1):44829.
- Mezger STP, Mingels AMA, Bekers O, et al. Mass spectrometry spatial-omics on a single conductive slide. Anal Chem. 2021;93(4):2527–2533.
- Risom T, Glass DR, Liu CC, et al. Transition to invasive breast cancer is associated with progressive changes in the structure and composition of tumor stroma [Internet]. Cancer Biology; 2021 [cited 2021 Jul 30]. Available from: .
- Chen J, Suo S, Tam PP, et al. Spatial transcriptomic analysis of cryosectioned tissue samples with Geo-seq. Nat Protoc. 2017;12(3):566–580.
- Singh S, Wang L, Schaff DL, et al. In situ 10-cell RNA sequencing in tissue and tumor biopsy samples. Sci Rep. 2019;9(1):4836.
- Paweletz CP, Charboneau L, Bichsel VE, et al. Reverse phase protein microarrays which capture disease progression show activation of pro-survival pathways at the cancer invasion front. Oncogene. 2001;20(16):1981–1989.
- Sutcliffe MD, Galvao RP, Wang L, et al. Premalignant oligodendrocyte precursor cells stall in a heterogeneous state of replication stress prior to gliomagenesis. Cancer Res. 2021;81(7):1868–1882.
- Narayanan M, Martins AJ, Tsang JS. Robust inference of cell-to-cell expression variations from single- and K-Cell profiling. PLOS Comput Biol. 2016;12(7):e1005016. Gottardo R, editor.
- Nichterwitz S, Nijssen J, Storvall H, et al. LCM-seq reveals unique transcriptional adaptation mechanisms of resistant neurons and identifies protective pathways in spinal muscular atrophy. Genome Res. 2020;30(8):1083–1096.
- Foley JW, Zhu C, Jolivet P, et al. Gene expression profiling of single cells from archival tissue with laser-capture microdissection and Smart-3SEQ. Genome Res. 2019;29(11):1816–1825.
- Pereira EJ, Burns JS, Lee CY, et al. Sporadic activation of an oxidative stress–dependent NRF2-p53 signaling network in breast epithelial spheroids and premalignancies. Sci Signal. 2020;13(627):eaba4200.
- Singh S, Sutcliffe MD, Repich K, et al. Pan-Cancer drivers are recurrent transcriptional regulatory heterogeneities in early-stage luminal breast cancer. Cancer Res. 2021;81(7):1840–1852.
- Loganathan J, Pandey R, Ambhore NS, et al. Laser-capture microdissection of murine lung for differential cellular RNA analysis. Cell Tissue Res. 2019;376(3):425–432.
- Schaff DL, Singh S, Kim K-B, et al. Fragmentation of small-cell lung cancer regulatory states in heterotypic microenvironments. Cancer Res. 2021;81(7):1853–1867.
- Kurth F, Wilson RE, Trüssel AJ, et al. Bridging the gap: towards microfluidic single cell analysis of in vivo stimulated cells.:3 17th International Conference on Miniaturized Systems for Chemistry and Life Sciences; 27-31 October 2013. DOI: https://doi.org/10.1002/smll.201903905
- O’Sullivan SJ, Reyes BAS, Vadigepalli R, et al. Combining Laser Capture Microdissection and Microfluidic qPCR to Analyze Transcriptional profiles of single cells: a systems biology approach to opioid dependence. J Vis Exp. 2020;60612. DOI: https://doi.org/10.3791/60612
- Surrette C, Shoudy D, Corwin A, et al. Microfluidic tissue mesodissection in molecular cancer diagnostics. SLAS Technol Transl Life Sci Innov. 2017;22:425–430.
- Coope RJ, Schlosser C, Corbett RD, et al. Whole‐slide laser microdissection for tumour enrichment. J Pathol. 2021;253(2):225–233.
- Au Q, Fu M, Bordwell A, et al. Abstract MultiOmyx TM : a multiplexed immunofluorescent assay capable of profiling protein expression and phosphorylation, in combination with next-generation sequencing from a single FFPE tissue section. Mol Cell Biol [Internet]. American Association for Cancer Research; 2015 [cited 2021 Jul 30]. p. 2014–2014. Available from: .
- Srinidhi CL, Ciga O, Martel AL. Deep neural network models for computational histopathology: a survey. Med Image Anal. 2021;67:101813.
- Gonzalez-Ericsson PI, Wulfkhule JD, Gallagher RI, et al. Tumor-specific major histocompatibility-II expression predicts benefit to anti-PD-1/L1 therapy in patients with HER2-negative primary breast cancer. Clin Cancer Res. 2021; clincanres.CCR-21-0607-A.2021. DOI:https://doi.org/10.1158/1078-0432.CCR-21-0607.
- Maurer C, Holmstrom SR, He J, et al. Experimental microdissection enables functional harmonisation of pancreatic cancer subtypes. Gut. 2019;68(6):1034–1043.