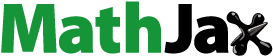
ABSTRACT
Cut-to-length harvesters collect detailed information on the dimensions and characteristics of individual harvested trees. When equipped with global navigation satellite system (GNSS) receivers and motion sensors, the obtained measurements can be linked to locations of single harvested trees, benefitting a range of forest inventory applications. We propose a way of georeferencing harvested trees using a Komatsu 931XC harvester, which measures and records the machine's bearing, crane angle and crane length for each harvested tree. We replaced the harvester’s standard GNSS receiver with a dual-antenna differential GNSS receiver. From the coordinates obtained, rotations calculated from the GNSS receiver and data on crane length, we determined the location of 285 trees harvested in eight final fellings in Norway. We compared the obtained locations to control measurements taken on the corresponding stumps directly after harvest using a differential GNSS receiver. The mean distance between planimetric coordinates of trees measured by the harvester and corresponding control measurements was 0.88 m with a standard deviation of 0.38 m. By correcting the crane lengths for systematic deviations between harvester and control locations, the mean distance was reduced to 0.79 m. This study shows that measurements of single harvested trees can be georeferenced with sub-meter accuracy, by mounting a differential GNSS receiver on a harvester and without installing additional sensors. The results also suggest that the positional accuracy can be further improved by measuring and recording the length of the telescopic boom, and that with minor adjustments, the system could be fully automated.
Introduction
Approximately four billion m3 of industrial roundwood are harvested annually for global timber consumption (Strandell and Wolf Citation2020). The fully mechanized cut-to-length harvesting system is one of the dominant harvesting systems, by which a harvester fells and processes trees in the forest. A forwarder then extracts the logs to a landing at the roadside, from which short-wood trucks transport the timber to the mill. Cut-to-length harvesting was originally developed in Finland and Sweden in the 1970s (Drushka and Konttinen Citation1997), and is now widely used throughout Scandinavia and other parts of Europe (Malinen et al. Citation2016), North America (Gautam et al. Citation2013; Blinn et al. Citation2015; Conrad et al. Citation2017), South America (Mac Donagh et al. Citation2017), Australia (Ghaffariyan et al. Citation2012), New Zealand (Evanson and McConchie Citation1996) and South Africa (Norihiro et al. Citation2018).
Although the basic concept of cut-to-length harvesting has remained unchanged, advances in computing and sensor technologies have greatly enhanced the efficiency of harvesters and their operating systems. Apart from felling, delimbing and bucking logs, harvesters are capable of measuring and storing large amounts of data on a range of production parameters. Log dimensions and volumes are measured by sensors mounted on the harvester head, and each cut is allocated a time stamp and stored on an on-board computer. Tree species and timber assortments are determined and recorded by the operator, providing information on the characteristics and quality of the harvested logs. Additionally, most operating systems can be coupled with Global Navigation Satellite Systems (GNSS, Olivera Citation2016), to track and map the machine’s operational path. The spatial data obtained assist machine operators in terrain navigation and forwarding (Marshall Citation2012). Finally, harvesters are increasingly equipped with motion sensors which monitor the machine bearing, i.e. the compass direction of the machine's front, the crane angle with respect to the machine bearing, and crane length (Westerberg Citation2014; Bhuiyan et al. Citation2016; La Hera and Morales Citation2019). These standardized parameters allow for georeferencing of individual harvested trees. Thus, besides felling and processing timber, harvesters are becoming increasingly efficient in collecting and storing large amounts of detailed data on harvested trees.
Data collected by cut-to-length harvesters are stored by on-board computers following a unified data format, i.e. the standard for forest machine data and communication (StanForD, Arlinger et al. Citation2012). In the newest standard, StanForD 2010, harvest production report (HPR) files are generated, containing data on harvested stems (stem ID, tree species, diameter at breast height, number of logs, time stamp, commercial height, diameters at 10 cm intervals along the stem) and logs produced (log ID, stem ID, assortment, log dimensions and volumes over and under bark). When the GNSS function is enabled, HPR files contain the base machine position (longitude, latitude, altitude), horizontal dilution of precision (HDOP) and number of available satellites. Machines that are equipped with motion sensor hardware in the crane also record the machine’s bearing, the crane angle with respect to the machine bearing and the crane length. These parameters are used to calculate the position of the crane tip when felling trees, and recorded in the HPR files along with the calculated positions (Bhuiyan et al. Citation2016).
When harvester heads are calibrated according to the manufacturers’ specifications, the produced data may benefit a number of forest inventory applications (Murphy et al. Citation2006; Lindroos et al. Citation2015). Forest inventories rely on tree measurements which are labor intensive and expensive to obtain, and harvesters acquire large amounts of such measurements during their operation. Recording data on many of the same characteristics of trees typically recorded in forest inventories (diameter at breast height, height, species), harvesters have been shown to be more precise than manual field work (Murphy et al. Citation2006), performing the measurements automatically and providing full census data for harvested stands. Particularly GNSS-enabled harvester data have been found useful for forest inventory (Olivera Citation2016), because they can be used as a substitute for conventional tree measurements obtained from sample plots (Hauglin et al. Citation2018). There now exists a trend toward increasing spatial resolution where harvester data are increasingly collected at sub-stand level (Lindroos et al. Citation2015). Therein, a reliable estimation of the position of the harvester head relative to the GNSS receiver has been identified as a key stepping stone (Lindroos et al. Citation2015).
The potential benefits of measuring and recording the position of the harvester head relative to the GNSS receiver was recognized long ago (Stendahl and Dahlin Citation2002). Although GNSS systems have been mounted on harvesters since the 1990s (Gellerstedt and Dahlin Citation1999), they have largely been limited to autonomous receivers mounted on the cabin, i.e. receivers which calculate the cabin’s position in real time from satellite data alone. Since then, GNSS-enabled harvester data have been used in various forest inventory applications, however in most cases, the location obtained from the receiver mounted on the cabin has been used directly as the position of harvested trees (Rasinmäki and Melkas Citation2005; Peuhkurinen et al. Citation2008; Bollandsås et al. Citation2011; Holmgren et al. Citation2012; Saukkola et al. Citation2019). Those studies demonstrated a wide range of applications of GNSS-enabled harvester data in forest inventory, however a lack of positional accuracy has hindered their widespread use.
For harvester data to augment or replace conventional field data in forest inventories, precise positioning of harvested trees is required. In forest inventories, forest attributes are commonly estimated using statistical relationships between field data and remotely sensed data (White et al. Citation2016). To develop such relationships in a reliable way, the field data will typically need to be georeferenced with sub-meter accuracy (Lindroos et al. Citation2015). Therefore, field data are commonly georeferenced using differential positioning (Deckert and Bolstad Citation1996; Næsset and Gjevestad Citation2008). Differential positioning implies using multiple GNSS receivers; one rover receiver at an unknown location and one or multiple base receivers at known locations. Because the locations of base receivers are known, the deviation between measured and actual reference positions can be calculated. Rover locations can then be corrected according to the calculated correction of the base receiver, either in real time or during post processing, for satellite ranging and clock errors (Grewal et al. Citation2020).
In two studies assessing the accuracy of differential positioning on a mobile platform, Ringdahl et al. (Citation2011) and Hellström et al. (Citation2009) fitted a differential GNSS on a forwarder as part of a navigation system, and reported path-tracking errors of only centimeters under clear sky conditions. Kaartinen et al. (Citation2015) tested the accuracy of trees georeferenced using a mobile laser scanning device and a differential GNSS mounted on an all-terrain-vehicle, and reported a mean positional error of 0.7 m. Hauglin et al. (Citation2017) were the first to use a cut-to-length harvester for positioning of individual harvested trees. They installed an integrated positioning system on a John Deere 1270E harvester, composed of two differential GNSS receivers, five wireless tilt sensors and two sensors mounted on hydraulic cylinders, which monitored and recorded the position of the harvester head relative to the GNSS receivers. They compared the positions obtained of 73 harvested trees with control measurements and reported a mean positional error of 0.94 m with a standard deviation of 0.58 m. In subsequent studies (Hauglin et al. Citation2018; Maltamo et al. Citation2019), the harvester measurements obtained were highly useful for forest inventory in linking them to remotely sensed data.
In light of further possibilities of automatic and massive georeferenced tree-level data collection using harvesters, it is important to assess how other harvesters and positioning systems perform in comparison to the results presented by Hauglin et al. (Citation2017). The current state-of-the-art of harvester sensor hardware facilitates the use of machine monitoring data directly as opposed to experimentally installing a system of sensors for monitoring the position of the harvester head. The aim of this study was to demonstrate and evaluate a practical way of measuring locations of harvested trees using a cut-to-length harvester. To improve practicality, we used standardized data on crane length, only replacing the harvester’s standard GNSS receiver with a differential GNSS receiver which allowed for correcting the obtained coordinates with base station reference data.
Materials and methods
Study sites
Data were obtained from eight final fellings in Nord-Aurdal municipality (60° 57ʹ N 9° 15ʹ E, 307–1325 m above sea level) and Etnedal municipality (60° 58ʹ N 9° 39ʹ E, 210–1414 m above sea level) in southern Norway (). The harvested stands were mainly composed of Norway spruce (Picea abies (L.) Karst.), with small proportions of Scots pine (Pinus sylvestris L.) and deciduous species; mainly birch (Betula pendula Roth. and Betula pubescens Ehrh.).
Harvester data
Harvester data were obtained between February 2019 and April 2020 using a Komatsu 931XC single-grip harvester equipped with a 230 H crane with a 10 m reach and a C144 harvester head (). As optional equipment supplied by the manufacturer, a pulse sensor measuring the angle between inner and outer boom was mounted on the crane, enabling crane tip positioning (Bhuiyan et al. Citation2016). The crane and cabin are mounted on a rotating platform with automatic leveling (longitudinal 20°/22° and lateral 16°). The MaxiXplorer control system was used (version 3.1.9 and updated to 3.2.1 during the course of data collection) from which output production data were exported as HPR files. The HPR files contained data on species and diameter at breast height of harvested stems, and dimensions and assortments of individual logs. In addition, for each harvested tree, the HPR files included the longitude and latitude values, machine bearing, i.e. the compass direction of the machine's front, the crane angle with respect to the machine bearing, and the crane length (). The machine bearing was measured as the GNSS track angle in degrees and logged in GPRMC format under forward motion. The crane angle with respect to the machine bearing was measured using a rotary sensor mounted on the rotating cabin platform. Although the sensor had a resolution of 0.25 degrees, crane angles were recorded in the HPR files in whole degrees. Measurements of crane length were based on the sensor measuring the angle between the inner and outer boom and the cosine rule (EquationEquation (1))(1)
(1) :
Figure 2. The Komatsu 931XC harvester used in this study, and the industry standard system for positioning harvested stems. The black arrow indicates the machine's bearing, the white arrow indicates the direction of the crane, C indicates the crane angle with respect to the machine bearing, A indicates the angle between the inner and outer boom, ,
and
indicate the inner and outer boom and telescopic extension, respectively, and L indicates the crane length, as calculated by the on-board computer
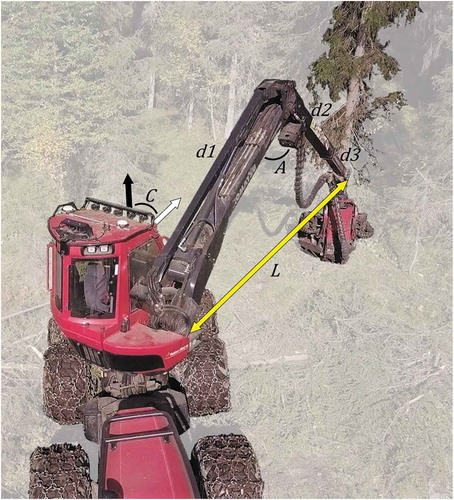
where is the crane length,
is the inner boom of 4.26 m,
is the outer boom of 3.37 m,
is the telescopic boom extension of 2.14 m and
is the angle (in radians) between the inner and outer boom (). Thus, although the length of the telescopic boom ranged between 0 and 2.14 m, it was set to a middle position of 1.07 m in calculating the crane length.
We replaced the harvester’s standard GNSS receiver with a real-time kinematic (RTK) Septentrio AsteRx-U differential GNSS receiver (), from which the longitude and latitude values were recorded in the HPR files. Additionally, the HDOP, number of satellites and rotation (in compass degrees) were recorded for each measurement. The GNSS receiver consisted of two antennas, both of which received pseudorange and carrier phase observables from all available GNSS constellations, i.e. BeiDou, Galileo, GLONASS and GPS. The GNSS receiver has a cellular modem for RTK correction, for which we used SATREF, i.e. a positioning service operated by the Norwegian Mapping Authority based on a nationwide network of geodetic GNSS receivers. Distances to the nearest base station ranged from 11.5 to 17.4 km, and under such circumstances, the system’s horizontal positional accuracy can be expected to be around one centimeter when using SATREF (Myrvang Citation2016).
Figure 3. Overview of the modified system for positioning harvested stems used in this study. White circles indicate the differential GNSS antennas, the white arrow indicates the rotation calculated from the GNSS data and L indicates the crane length calculated by the on-board computer
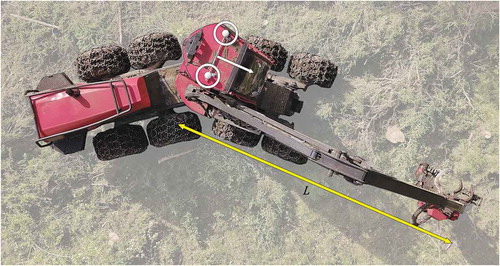
The harvester’s on-board computer only accommodated input from one antenna, locations of which were used as input by the on-board computer in calculating the base machine and crane tip positions. Positions and rotations using also the second antenna were stored in the National Marine Electronics Association (NMEA) format in the Septentrio GNSS at a one second rate. HPR and NMEA files were accessible online through the Komatsu MaxiFleet application and a file transfer protocol (FTP), respectively. An overview of the harvester data is shown in .
Table 1. Summary of harvester data
Control measurements
We determined the locations of a total of 285 control trees using a TOPCON HiPer SR in RTK mode, by measuring the stump center immediately after harvest. The control measurements were collected as part of a master's thesis (Iversen Citation2020; site 6) and our data quality control for the remaining sites. Each stump was measured directly after harvest and before the subsequent tree was harvested, ensuring that the order of control measurements corresponded to the order of harvested trees in the HPR files. All measurements were obtained under clear sky conditions and with RTK fixed solutions. The planimetric error margin of control measurements ranged from 1 to 3 cm with a mean of 2 cm. After measuring the stump of the last control tree during field work, we exported the HPR files from the on-board computer to ensure a link between control trees and stem ID in the HPR files.
Data analysis
From the HPR files, we extracted time stamps of fellings, crane tip positions and values of machine bearing, crane angle and crane length at the time of felling. In this study, the crane tip positions represent coordinates of harvested trees. Because we replaced the standard GNSS with the differential GNSS, the crane tip positions calculated by the on-board computer and recorded in the HPR files were based on differential GNSS measurements. However, we found a number of irregularities in values of machine bearing, where, for example, the bearing changed approximately 180° abruptly between harvested trees, resulting in positional errors >10 m. Therefore, we re-calculated the crane tip positions using rotations calculated from the NMEA data () as described in the following. For each harvested tree, we linked the stem data obtained from the HPR files with the nearest NMEA measurement, and extracted the corresponding NMEA rotation, HDOP and number of satellites. We then calculated the distance between the base machine position and tree location in longitudinal and latitudinal directions, by multiplying the crane length with the sine and cosine of the GNSS rotation, respectively. Finally, we added the distances in longitudinal and latitudinal directions to the base machine coordinates to obtain the coordinates of harvested trees.
We computed positional errors as the distances between planimetric coordinates of control measurements and harvester crane tip measurements. To assess whether the positional accuracy remained stable over time, we compared mean errors obtained for the separate sites using a one-way analysis of variance (ANOVA) test. We further assessed the correlation between the obtained positional errors and the HDOP and number of satellites recorded by the GNSS and the crane length recorded in the HPR files. Additionally, because the length of the telescopic boom was not accounted for in the crane length parameter, we computed all tree locations given adjustments of crane length ranging from −100 cm to 100 cm with 10 cm intervals, to quantify and potentially compensate for systematic deviations between control measurements and harvester tree locations.
Results
The distribution of the positional errors obtained is shown in . Errors ranged from 0.14 to 2.85 m, with a mean of 0.88 m and a standard deviation of 0.38 m. The one-way ANOVA test revealed that mean errors did not differ significantly among sites (F-value = 0.20; p-value = 0.66), i.e. the accuracy of the positioning system remained stable over time (). The mean error obtained for site 6, i.e. the site with the largest number of control measurements, was 0.89 m, with a standard deviation of 0.26 m.
Figure 4. Density plot of positional errors obtained for the 285 harvested trees. The dashed line indicates the mean error of 0.88 m
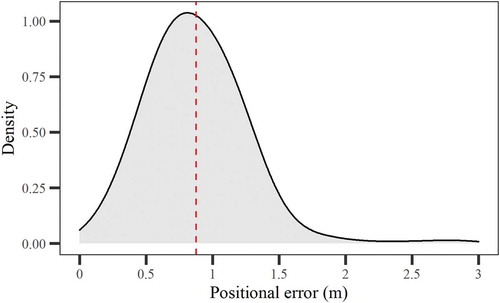
Figure 5. Ridgeline plot of positional errors obtained across sites. Labels indicate the month of data collection and number of observations, the dashed line indicates the mean error obtained for all sites combined
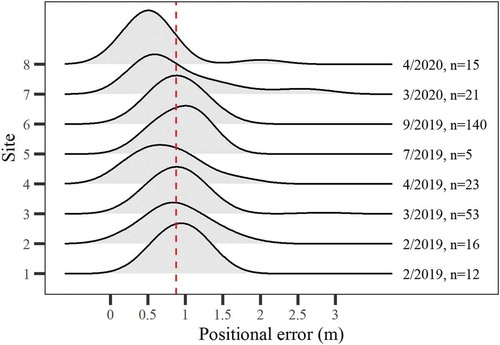
We found only weak correlations between positional errors and values of HDOP, number of satellites and crane length (0.18, −0.14 and −0.16, respectively). We did not find any clear patterns in the distribution of errors across crane angles, however, positional errors were limited to around 1.5 meter when only using measurements obtained with at least 19 observed satellites ().
Figure 6. Positional errors plotted against values of horizontal dilution of precision, number of satellites, crane length and crane angle
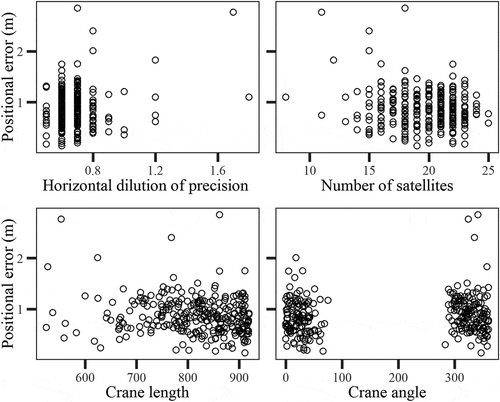
Mean positional errors were reduced by decreasing the crane length, where a crane length reduction of 40 cm minimized the mean positional error, yielding a mean error of 0.79 m (). Given the 40 cm reduction, the positional errors had a standard deviation of 0.36 m, 84% of errors were <1 m, and 95% of the errors were between 0.34 and 1.45 m. The positional accuracy was improved by reducing the crane length with as much as 80 cm. The magnitude of variability in positional errors increased with both negative and positive crane length adjustments.
Discussion
The aim of this study was to demonstrate and evaluate a practical way of measuring locations of harvested trees using a cut-to-length harvester. By coupling a differential GNSS receiver to a conventional harvester operating system and using standardized machine monitoring data on crane length, we were able to position harvested trees with sub-meter accuracy. From a forest inventory perspective, this result is promising because it indicates that large numbers of harvester measurements can be georeferenced relatively easily, which may benefit a range of forest inventory applications (Lindroos et al. Citation2015). Given the level of positional accuracy, the data may potentially augment or replace manual field work in various inventory applications, three main advantages being (1) the level of detail of tree measurements, (2) the large volume of generated data and (3) the fact that the data are generated as a by-product of harvesting operations. Due to irregularities in values of machine bearing, however, we had to post-process the data using rotations calculated from the GNSS receiver. Therefore, the crane tip positions recorded in the HPR files could not be used directly as locations of harvested trees, making the method less practical. However, this issue could be resolved by reprogramming the on-board computer to use rotations calculated from the GNSS data instead of rotations based on values of machine bearing and crane angle.
An obvious potential use of the data obtained lies in linking them to remotely sensed data for modeling and predicting forest attributes, as a substitute for plot-level measurements. For such an application, the positional accuracy obtained in this study would suffice (Lindroos et al. Citation2015). In previous studies, harvester data were shown to be a suitable replacement for manual field work (Hauglin et al. Citation2018), by which plots can be selected anywhere from within a harvested forest area, of any desired size and shape (Maltamo et al. Citation2019). A potential challenge of utilizing harvester data in forest inventories based on remotely sensed data, however, is that the data will not constitute a probability-based sample. Thereby, they are largely limited to mature forests, i.e. at a stage of being ready for commercial harvest. The system could potentially also be used in a younger forest, during thinnings, however under a forest canopy the positional accuracy may degrade substantially. In this study, we only used data collected during clear-felling operations and thus under open-sky conditions. Therefore, further testing is needed to assess the performance of the positioning system under a forest canopy. Nevertheless, field work constitutes one of the main cost components of forest inventories assisted by remotely sensed data (Means et al. Citation2000; Næsset Citation2007), and harvester data may thus prove beneficial in augmenting at least some of the manual field work carried out in forest inventories.
The positional accuracies obtained in this study confirm earlier findings reported by Hauglin et al. (Citation2017), who found that measurements of single harvested trees can be georeferenced with sub-meter accuracy using a harvester. In the aforementioned study, slightly larger positional errors were reported with a mean of 0.94 m and standard deviation of 0.58 m. Two main novel aspects of the current study are that (1) additional equipment mounted on the harvester was limited to a differential GNSS receiver and (2) the crane and cabin of Komatsu cut-to-length harvesters are both mounted on the same automatically leveled platform. While the former aspect mainly benefits practicality, the latter also benefits positional accuracy as both the GNSS receiver and crane are leveled simultaneously when maneuvering uneven terrain, thus eliminating potential orientation errors between the GNSS receiver and crane base. For harvesters on which the crane and cabin are mounted on separate platforms, the use of rotations calculated from the GNSS data may be less practical, and calculations of crane tip positions may alternatively be based on values of machine bearing and crane angle, i.e. the current industry standard. Thus, a range of forest harvesters could potentially be equipped with positioning systems similar to the one used in this study, including other types of harvesters such as feller bunchers (Pan and McDonald Citation2019) and excavator-based harvesters (Ackerman et al. Citation2018).
Because the horizontal positional accuracy of the differential GNSS receiver mounted on the cabin can be expected to be around one centimeter, most of the positional errors obtained should be attributed to other error sources. Primarily, there was no sensor measuring the length of the telescopic boom, which ranged between 0 and 2.14 m. In calculating the crane length, the position of the telescopic boom was set to a constant middle position of 1.07 m, however this introduced a substantial random error which may have caused a large portion of the positional errors obtained in this study. The extent to which the telescopic boom is used may vary across operators, forest types and terrain, whereby the boom may tend to be extended further in steep terrain, degrading the positional accuracy substantially. Visual assessments during control measurements indicated that the majority of control trees were felled with the telescopic boom in an approximate middle position. If the operator would have had a tendency to extent or retract the telescopic boom further during felling, the positional errors would have been larger. Nevertheless, other sources of error should be anticipated. These include varying angles between the crane base and inner boom, orientation errors due to the harvester head being rotated toward either side of the crane tip, varying stem diameters, or resolution errors in crane angle sensors. However, GNSS rotations were recorded with 0.001 degree resolution, and resulting resolution errors were therefore small in comparison to the random error introduced by the telescopic boom, which for any given tree may have been off by a meter in either direction. To further improve the positional accuracy, sensor hardware measuring the length of the telescopic boom is needed to aid in the calculation of crane length.
We did not find any strong correlation between positional errors and values of HDOP, number of satellites and crane length. While Hauglin et al. (Citation2017) reported correlations of 0.51 and −0.21 with values of HDOP and number of satellites, respectively, we obtained values of 0.18 and −0.14. It is generally assumed that HDOP is a good indicator of positional accuracy (Lu and Schnieder Citation2014; Wen et al. Citation2018). However, there was little variation in values of HDOP in this study, where values ranged between 0.5 and 0.9 (, ). Values of HDOP may increase under canopy conditions (Blum et al. Citation2016), and although the data were collected during final fellings, partial canopy coverage may have been present during collection of some of the data used in this study. Although we did not find a strong correlation between positional errors and the number of observed satellites, positional errors were limited to around 1.5 meter when only using measurements with at least 19 observed satellites. Thus, a certain level of positional accuracy may potentially be maintained by only using GNSS positions calculated from a given number of observed satellites, however future studies using more data are needed to confirm this prospect.
Regarding the operational use of georeferenced harvester data in forest inventories, there are several directions for future research which may be pursued to improve accuracy and practicality. First, sensor hardware for measuring the length of the telescopic boom should be investigated, as this was likely the main source of error in this study. Second, sensor hardware for measuring the angle between the crane base and inner boom, as well as the rotation of the harvester head toward either side of the crane tip and dimensions of the harvester heads, may further improve the positional accuracy. Thereby, diameters of harvested stems could be taken into account as well, which are already measured and recorded. Third, practicality may be improved by using the GNSS rotation directly when calculating the coordinates of harvested trees, to avoid re-calculating the coordinates during post-processing. Fourth, the system should be tested under thinning operations, as the presence of a partial or closed canopy may degrade the positional accuracy substantially. Finally, operational applications of sub-meter accurate GNSS-enabled harvester data should be investigated.
Conclusions
Overall, this study showed that measurements of single harvested trees can be georeferenced with sub-meter accuracy by replacing a harvesters’ standard GNSS with a dual antenna differential GNSS. Rotations obtained from the GNSS data and the length of the crane obtained from the on-board computer proved to be highly useful in determining the coordinates of harvested trees. Future research should focus on developing sensor hardware for measuring and recording the length of the harvester’s telescopic boom.
Acknowledgements
This work was funded by the Research Council of Norway under the project PRECISION (NFR Project No. 281140). We would like to extend our thanks to the contractor, Valdres Skog AS, and the machine operator Karl Henning Espeseth for permitting the use of their harvester and data, and the manufacturer Komatsu Forestry for useful information. We are also grateful to the forestry students Ole Marius Tollefsen Moen, Erik Armand Iversen and Mikal Råheim who helped with data collection. Finally, we would like to thank the reviewers for their constructive feedback.
Disclosure statement
No potential conflict of interest was reported by the authors.
Additional information
Funding
References
- Ackerman P, Martin C, Brewer J, Ackerman S. 2018. Effect of slope on productivity and cost of Eucalyptus pulpwood harvesting using single-grip purpose-built and excavator-based harvesters. Int J For Eng. 29(2):74–82.
- Arlinger J, Nordström M, Möller JJ. 2012. StanForD 2010: modern communication with forest machines. Arbetsrapport från Skogforsk. Uppsala, Sweden.Skogforsk. (785).
- Bhuiyan N, Möller J, Hannrup B, Arlinger J. 2016. Automatisk gallringsuppföljning–Arealberäkning samt registrering av kranvinkel för identifiering av stickvägsträd och beräkning av gallringskvot. Arbetsrapport från Skogforsk. Uppsala, Sweden.Skogforsk. (899).
- Blinn CR, O’Hara TJ, Chura DT, Russell MB. 2015. Minnesota’s logging businesses: an assessment of the health and viability of the sector. For Sci. 61(2):381–387. doi:10.5849/forsci.14-013
- Blum R, Bischof R, Sauter UH, Foeller J. 2016. Tests of reception of the combination of GPS and GLONASS signals under and above forest canopy in the Black Forest, Germany, using choke ring antennas. Int J For Eng. 27(1):2–14.
- Bollandsås OM, Maltamo M, Gobakken T, Lien V, Næssset E. 2011. Prediction of timber quality parameters of forest stands by means of small footprint airborne laser scanner data. Int J For Eng. 22(1):14–23
- Conrad JL IV, Vokoun MM, Prisley SP, Bolding MC. 2017. Barriers to logging production and efficiency in Wisconsin. Int J For Eng. 28(1):57–65.
- Deckert C, Bolstad PV. 1996. Forest canopy, terrain, and distance effects on global positioning system point accuracy. Photogramm Eng Remote Sens. 62(3):317–321.
- Drushka K, Konttinen H. 1997. Tracks in the forest: the evolution of logging machinery. Helsinki (Finland): Timberjack group.
- Evanson T, McConchie M. 1996. Productivity measurements of two Waratah 234 hydraulic tree harvesters in radiata pine in New Zealand. Int J For Eng. 7(3):41–52.
- Exclusion of GNSS NLOS receptions caused by dynamic objects in heavy traffic urban scenarios using real-time 3D point cloud: An approach without 3D maps. In: 2018 IEEE/ION Position, Location and Navigation Symposium (PLANS); 2018: IEEE.
- Gautam S, Pulkki R, Shahi C, Leitch M. 2013. Quality assessment of cut-to-length logging residues for bioenergy production in Northwestern Ontario. Int J For Eng. 24(1):53–59.
- Gellerstedt S, Dahlin B. 1999. Cut-to-length: the next decade. J For Eng. 10(2):17–24.
- Ghaffariyan M, Sessions J, Brown M. 2012. Machine productivity and residual harvesting residues associated with a cut-to-length harvest system in southern Tasmania. South For. 74(4):229–235. doi:10.2989/20702620.2012.741770
- Grewal MS, Andrews AP, Bartone CG. 2020. Global Navigation Satellite Systems, Inertial Navigation, and Integration. Hoboken, USA: John Wiley & Sons.
- Hauglin M, Hansen E, Sørngård E, Næsset E, Gobakken T. 2018. Utilizing accurately positioned harvester data: modelling forest volume with airborne laser scanning. Can J For Res. 48(8):913–922. doi:10.1139/cjfr-2017-0467
- Hauglin M, Hansen EH, Næsset E, Busterud BE, Gjevestad JGO, Gobakken T. 2017. Accurate single-tree positions from a harvester: a test of two global satellite-based positioning systems. Scand J For Res. 32(8):774–781. doi:10.1080/02827581.2017.1296967
- Hellström T, Lärkeryd P, Nordfjell T, Ringdahl O. 2009. Autonomous forest vehicles: historic, envisioned, and state-of-the-art. Int J For Eng. 20(1):31–38.
- Holmgren J, Barth A, Larsson H, Olsson H. 2012. Prediction of stem attributes by combining airborne laser scanning and measurements from harvesters. Silva Fenn. 46(2):227–239
- Iversen EA. 2020. Detection of root and butt rot in Norway Spruce (Picea Abies) using airborne hyperspectral images and laser scanning. Ås: Norwegian University of Life Sciences.
- Kaartinen H, Hyyppä J, Vastaranta M, Kukko A, Jaakkola A, Yu X, Pyörälä J, Liang X, Liu J, Wang Y. 2015. Accuracy of kinematic positioning using global satellite navigation systems under forest canopies. Forests. 6(9):3218–3236. doi:10.3390/f6093218
- La Hera P, Morales DO. 2019. What do we observe when we equip a forestry crane with motion sensors? Croat J For Eng. 40(2):259–280. doi:10.5552/crojfe.2019.501
- Lindroos O, Ringdahl O, La Hera P, Hohnloser P, Hellström TH. 2015. Estimating the position of the harvester head–a key step towards the precision forestry of the future? Croat J For Eng. 36(2):147–164.
- Lu D, Schnieder E. 2014. Performance evaluation of GNSS for train localization. IEEE Trans Intell Transp Syst. 16(2):1054–1059.
- Mac Donagh P, Botta G, Schlichter T, Cubbage F. 2017. Harvesting contractor production and costs in forest plantations of Argentina, Brazil, and Uruguay. Int J For Eng. 28(3):157–168.
- Malinen J, Laitila J, Väätäinen K, Viitamäki K. 2016. Variation in age, annual usage and resale price of cut-to-length machinery in different regions of Europe. Int J For Eng. 27(2):95–102.
- Maltamo M, Hauglin M, Næsset E, Gobakken T. 2019. Estimating stand level stem diameter distribution utilizing harvester data and airborne laser scanning. Silva Fenn. 53(3):1–19. doi:10.14214/sf.10075
- Marshall H. 2012. On-board machine stability information system. Harvesting technical note HTN05-01. Rotorua: Future Forests Research Limited.
- Means JE, Acker SA, Fitt BJ, Renslow M, Emerson L, Hendrix CJ. 2000. Predicting forest stand characteristics with airborne scanning lidar. Photogramm Eng Remote Sens. 66(11):1367–1372.
- Murphy G, Wilson I, Barr B. 2006. Developing methods for pre-harvest inventories which use a harvester as the sampling tool. Aust For. 69(1):9–15. doi:10.1080/00049158.2006.10674982
- Myrvang B. 2016. Kvalitetskontroll av CPOS. Ås: Norwegian University of LIfe Sciences.
- Næsset E. 2007. Airborne laser scanning as a method in operational forest inventory: status of accuracy assessments accomplished in Scandinavia. Scand J For Res. 22(5):433–442. doi:10.1080/02827580701672147
- Næsset E, Gjevestad JG. 2008. Performance of GPS precise point positioning under conifer forest canopies. Photogramm Eng Remote Sens. 74(5):661–668. doi:10.14358/PERS.74.5.661
- Norihiro J, Ackerman P, Spong BD, Längin D. 2018. Productivity model for cut-to-length harvester operation in South African Eucalyptus pulpwood plantations. Croat J For Eng. 39(1):1–13.
- Olivera A. 2016. Exploring opportunities for the integration of GNSS with forest harvester data to improve forest management [Doctoral thesis]. Christchurch (New Zealand): University of Canterbury.
- Pan P, McDonald TP. 2019. Tree size estimation from a feller-buncher’s cutting sound. Comput Electron Agric. 159:50–58. doi:10.1016/j.compag.2019.02.021.
- Peuhkurinen, J, Maltamo, M, & Malinen J. 2008. Estimating species-specific diameter distributions and saw log recoveries of boreal forests from airborne laser scanning data and aerial photographs: a distribution-based approach. Silva Fenn. 42(4):625–641
- Rasinmäki J, Melkas T. 2005. A method for estimating tree composition and volume using harvester data. Scand J For Res. 20(1):85–95. doi:10.1080/02827580510008185
- Ringdahl O, Lindroos O, Hellström T, Bergström D, Athanassiadis D, Nordfjell T. 2011. Path tracking in forest terrain by an autonomous forwarder. Scand J For Res. 26(4):350–359. doi:10.1080/02827581.2011.566889
- Saukkola A, Melkas T, Riekki K, Sirparanta S, Peuhkurinen J, Holopainen M, Hyyppä J, Vastaranta M. 2019. Predicting forest inventory attributes using airborne laser scanning, aerial imagery, and harvester data. Remote Sens. 11(7):797
- Stendahl J, Dahlin B. 2002. Possibilities for harvester-based forest inventory in thinnings. Scand J For Res. 17(6):548–555. doi:10.1080/02827580260417206
- Strandell H, Wolf P. 2020. The EU in the world. Luxembourg: European Union.
- Wen, W, Zhang, G, Hsu, LT. 2018. Exclusion of GNSS NLOS receptions caused by dynamic objects in heavy traffic urban scenarios using real-time 3D point cloud: An approach without 3D maps. IEEE/ION Position, Location and Navigation Symposium. 2018:158–165
- Westerberg S. 2014. Semi-automating forestry machines: motion planning, system integration, and human-machine interaction [Doctoral thesis]. Sweden: Umeå Universitet.
- White JC, Coops NC, Wulder MA, Vastaranta M, Hilker T, Tompalski P. 2016. Remote sensing technologies for enhancing forest inventories: a review. Can J Remote Sens. 42(5):619–641. doi:10.1080/07038992.2016.1207484