ABSTRACT
The forestry sector is constantly looking for ways for making data-driven decisions and improving efficiency. The application of Data Envelopment Analysis (DEA) and Stochastic Frontier Analysis (SFA) allow the users to go beyond looking at simple key performance indicators. Benchmarking is one of the most common tools in business for improving efficiency and competitiveness. This study searched for benchmarking studies in Web of Science until December 2020. It reviewed 56 benchmarking studies in forestry and discusses the potential advantages of using benchmarking in forestry. More than 80% of the studies apply DEA. This review found that almost half of the benchmarking studies in forestry have attempted to estimate the efficiency of forest management organizations at regional scale, mostly being public or state-owned forest districts. A bit more than one-third of the studies have focused on benchmarking forest industries and one-fifth, benchmarking of forest operations. Forest management organizations mainly applied benchmarking for internal comparison and forest industries entirely focused on competitive benchmarking. Surprisingly, in most cases the studies do not necessarily overlap geographically with forest rich countries (e.g., Russian Federation or Brazil). A number of studies address multiple criteria. The future potential for applying automatic data transfer from harvest machines to interactive benchmarking systems are discussed. Finally, the paper discusses the advantages and weaknesses of benchmarking and future research on improving usefulness and usability of benchmarking in forest businesses.
Introduction
Improving performance and efficiency is a fundamental goal across all sectorss including forestry. Traditionally, key performance indicators (KPIs) have been applied in the forestry sector to track business performance and efficiency (Rafiei et al. Citation2014; Proto and Zimbalatti Citation2016). Depending on the goal, specific KPIs are often expressed as the ratio of an output to an input where naturally the higher KPI the better. Each KPI is then a partial measure of performance. However, KPIs have limitations because they are based on several explicit assumptions, e.g., input and output can be scaled proportionally (i.e., constant returns-to-scale). This means that production does not depend on the size of the production unit, and that a small production unit can generate the same income per unit produced as do larger units. Furthermore, KPIs usually do not capture relationships associated with the substitution of inputs and outputs. In order to address this limitation of KPIs, other benchmarking approaches have been developed. In modern benchmarking the two main approaches are Data Envelopment Analysis (DEA) and Stochastic Frontier Analysis (SFA). DEA has the advantage that it is non-parametric, it does not require a functional form specification and it is easy to compute using linear programming. Furthermore, it directly allows for learning from dominating peers. SFA usually requires a parametric functional form specification and distributional assumptions on the noise and inefficiency term, but has the advantage that it naturally handles noise in the data (Kumbhakar and Lovell Citation2003; Bogetoft and Otto Citation2011). The potential of such applications of benchmarking has so far merely been discussed in the research community (Aalmo and Baardsen Citation2015; Wang et al. Citation2019; Obi and Visser Citation2020; Aalmo et al. Citation2020).
The main aim of this paper is to review the applications of DEA and SFA for benchmarking in the forestry sector. Earlier literature reviews on their applications can be found in Salehirad and Sowlati (Citation2005) and Sowlati (Citation2005). Since then a number of studies applying SFA and DEA in the forestry sector have been published. The review is followed by a discussion of the potential for operators, machine owners and forest owners to make use of the vast amount of data modern harvesters store from harvest operations for benchmarking. However, most often benchmarking analysis is made on a static data set not allowing for more frequent updating of the data which forms the production set (Agrell and Bogetoft Citation2017). Therefore, we discuss the potential of automated data capture from machines, online monitoring of productivity and interactive benchmarking as a tool for improving productivity in forest operations.
Benchmarking with DEA and SFA
Benchmarking has been applied as a management tool to improve performance by identifying and applying best documented practices. Managers compare the performance of their respective organizations, products and processes externally with competitors and best-in-class companies and internally with other operations within their own firms that perform similar activities.
In contrast to KPI’s, the starting point of benchmarking is the “production process” describing the physical relationship between resources (called “inputs”) and products/outcomes (called “outputs”). The granularity of this specification can be very high (i.e. all inputs contribute in production of all outputs), but can also be more refined e.g., (i) one can specify different sub-processes with their own process-specific inputs, shared inputs and (intermediate) outputs; (ii) one can specify an output-specific production process for every output with its own output-specific inputs and joint inputs. The granularity of this specification mostly depends on the information available to the analyst, who also needs to consider a sufficient level of discrimination (Dyson et al. Citation2001).
Together, the inputs and outputs form a production set on which a number of plausible assumptions in the form of mathematical axioms are postulated. Together with the observations, these assumptions serve to approximate the production set. The efficient frontier of the defined production set represents all the “technically efficient” input-output combinations. This frontier gives the maximal outputs that can be produced for some given underlying inputs, or the minimum input required to produce a given output (Bogetoft Citation2012, p. 8). One of the first DEA models (named DEA-CCR), suggested by Charnes et al. (Citation1978) assumes a constant returns to scale between inputs and outputs. A second and frequently applied model is the DEA-BCC model, which assumes variable returns to scale (Banker et al. Citation1984). Often technical efficiency from DEA-CCR and DEA-BCC models are applied to estimate the scale inefficiency due to increasing or decreasing returns to scale (Cooper et al. Citation2000). The actual benchmarking is done by comparing the observation under evaluation against the efficient frontier by measuring the distance between the evaluated observation and the frontier using a distance function. Depending on the specification, this distance function effectively gauges for potential improvements in (i) inputs keeping outputs constant, (ii) outputs keeping inputs constant or (iii) both. Approximation of the specific production set can be done using either DEA or SFA. Productivity may change over time and to capture such changes in efficiency analysis the most popular method has been the Malmquist index, which measures how much a firm improves from one period to the next (Färe et al. Citation1994).
Materials and methods
Drawing upon the literature and practice of systematic reviews in social sciences (Gough et al. Citation2012; Petticrew and Roberts Citation2012), a systematic review methodology was applied to identify and collect relevant studies. The systematic review process consisted of identification of scope of the review and research questions, development of the search strategy, literature search and selection of relevant publications and data extraction and synthesis. The scoping focused on identifying all applications of DEA and SFA studies in forestry. Based on this we developed a search for relevant DEA and SFA studies in Web of Science (December 2020) using Term Searched = TS = ((forest* OR wood OR sawmill OR “wood industr*“ OR “forest sector*“) AND (”stochastic frontier analysis” OR SFA OR DEA OR ”data envelopment analysis”)) as keywords. This stage of the search revealed 360 references, and we extracted a final set of references by excluding conference proceedings, theoretical or methods papers without case applications, and papers mentioning DEA or SFA studies but not implementing an efficiency analysis on a forestry relevant case. We synthesized these studies’ by their general characteristics, country case, methodological approaches and data. Furthermore, the studies were assigned a benchmarking type according to whether they were performance, process or strategic benchmarking, and if they were applied for internal or competitive benchmarking (Andersen and Pettersen Citation1996, p. 7). Performance benchmarking determines how well a company compares to others. Process benchmarking is a comparison of methods and practices to learn and improve a company’s processes. Strategic benchmarking is a comparison of the strategic choices and disposition made by other companies to improve a company’s own strategic planning. Internal benchmarking is a comparison within the same company or organization, and competitive benchmarking compares a company with competitors (ibid.).
Results
The final selection included 56 studies that were classified as to whether they applied DEA, SFA or both, together with a brief description of the subject they analyzed. Furthermore, we noted the returns to scale assumptions of the model (technology), efficiency measures, benchmarking types, inputs, outputs, country case, and data sources (see and Supplementary Information Table S1).
Figure 1. Distribution of the 56 reviewed studies according to focus of the benchmarking (panel A), benchmarking with DEA, SFA or both (panel B), type of efficiency measure (panel C), ratio between number of Decision Making Units (DMUs) and three times the sum of input and output variables (panel D), and internal versus competetive benchmarking studies (Andersen and Pettersen Citation1996) according to focus of the benchmarking (panel E)
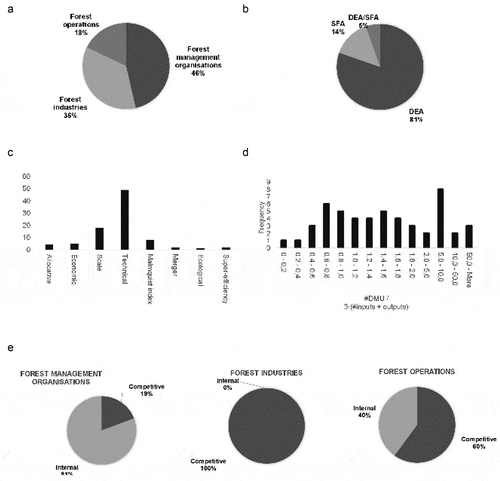
Case countries and focus area of the benchmarking
We identified the case countries and categorized the studies according to the focus area of the benchmarking.
DEA and SFA studies in forestry have been conducted in many countries. However, the most numerous investigations have been applied in United States, Canada, Taiwan, China, and Norway. The results indicate that the applications of benchmarking approaches such as DEA and SFA in forestry are concentrated on a few countries (). Most of the studies, like Kao and Yang (Citation1991) and LeBel (Citation1996) verify the viability of DEA within forestry. The study subjects range from the operational assessment of forest workers’ performance to the efficiency of sawmills and forest management organizations. These studies use DEA or SFA as methods to measure efficiency and establish a foundation for comparison. The efficiency assessment becomes the first step for subsequent analysis. Examples include the identification of potential mergers (Bogetoft et al. Citation2003), the identification of scale efficiency (Nyrud and Bergseng Citation2002) and statistical analysis and verification (Viitala and Hänninen Citation1998). To be able to better distinguish between these studies, they have been categorized according to the subjects they address, which is the efficiency estimation of three main focus areas: benchmarking of forest management organizations, benchmarking of forest industries, and benchmarking of forest operations (, panel A). More than 46% of the 56 studies attempted to estimate the efficiency of forest management organizations, with the majority addressing public or state-owned forest districts (Korkmaz Citation2011; Lin and Ge Citation2019; Zadmirzaei et al. Citation2019). A relative large number of studies (36%) have investigated the application of DEA within the forest industry. These studies have been conducted in a highly competitive environment, with marginal profit margins (Salehirad and Sowlati Citation2005). The primary focus of these studies was the productivity of individual pulp and paper plants and sawmills that produced a range of different products. For such reasons a narrow focus on input and outputs, which best describe a cost efficient production, was applied. For example Yin (Citation1998) investigated the application of DEA to measure the productivity efficiency of 44 mills. This study found that most of the mills were technically efficient, but not allocative efficient. Similar performance studies were conducted by Nyrud and Bergseng (Citation2002) and Nyrud and Baardsen (Citation2003), each using DEA as a part of an analysis to estimate productivity growth in technical and scale efficiency over multiple periods for a large number of sawmills. Finally, a study conducted by Penfield et al. (Citation2014) combined DEA analysis with a survey of 15 sawmills, where the DEA was used to verify the results of the questionnaire. Approximately 18% of the 56 studies estimated the efficiency of operational forest management and logging. A few studies have applied DEA to investigate forest contractors’ efficiency (LeBel Citation1996; Obi and Visser Citation2018). LeBel (Citation1996) made a very comprehensive study of 23 competing logging contractors, applying DEA to evaluate annual performance over 6 years. They also studied the factors determining efficiency, like how local conditions affected productivity. Šporčić et al. (Citation2009b) applied DEA to research the maintenance of 13 mechanized forestry units that executed forest operations in the Croatian State Forest. They assessed the mechanized units’ efficiency to operate when waste (waste tyres, solid waste, and waste oil) from the mechanized units was taken into account, along with financial measures. Their results show potential for the different mechanized units to minimize waste compared to the best performers. They concluded that DEA was applicable in a context where the ecological and financial efficiency of organizational units were aggregated. This approach could be used as the first part of a process to identify the best performing units, while another conclusion was that the method was highly sensitive to outliers and that DEA can identify best performance, but not provide the answers. More than 81% of the 56 studies applied DEA, 14% SFA, and the remaining 5% both approaches for studying efficiency (, panel B).
Table 1. Rank, countries/regions, records, and percentage of the 56 forestry relevant DEA and SFA records found in Web of Science (December 2020)
Efficiency measures
The focus of the majority of the studies is on evaluating technical efficiency (, panel C). Almost 20 studies investigated scale efficiency (Viitala and Hänninen Citation1998; Yin Citation1998, Citation2000; Nyrud and Bergseng Citation2002; Bogetoft et al. Citation2003; Salehirad and Sowlati Citation2005). For example, Kao and Yang (Citation1991) estimated the technical and scale efficiency of 13 national forest districts based on their ability to produce wood, their recreational value and their ability to prevent soil erosion. They showed that, by disaggregating efficiency into technical and scale efficiency, forest managers can identify inefficiency and propose policies that improve efficiency of the forest districts. Martinic et al. (2009) conducted a similar study using a much larger dataset. The authors grouped the forest offices by basic structural characteristics, like region, number of hectares and growing stock. Results showed clear differences between technical and scale efficiency amongst groupings. Shiba (Citation1997) also conducted a technical efficiency study of a forest owners association. The author made three partial models, each using several combinations of inputs and outputs, to test their effect on efficiency score. This study concluded that a key component in DEA is to choose the right inputs and outputs that best describe efficiency, and that DEA allows for the identification of Decision Making Units (DMUs) with the highest efficiency scores to gain further information about how to achieve the same performance. The same conclusions were reached by Korkmaz (Citation2011) by applying three partial models, and a fourth model that included all inputs and outputs. Viitala and Hänninen (Citation1998) conducted similar studies of 19 public forestry boards within the same organization. Because the managers in each forestry board could allocate resources freely to each input, the estimation of efficiency and scale efficiency where done so that each input that was specified in costs and regarded as an activity had its own efficiency and scale efficiency score. Their conclusion was that because of increasing returns to scale, it would be feasible to merge some of the units to improve efficiency. Furthermore, they concluded that DEA proved useful but that it is prone to extreme values, so that verification of results is necessary. Similar studies assessing organizational efficiency and scale efficiency for eventual merger propositions based on increasing returns to scale were done by Kao and Yang (Citation1991). Bogetoft et al. (Citation2003) estimated the technical efficiency of 14 private forests cooperatives. Their efficiency assessment led them to propose a potential merger of specific districts based upon further decomposition of the efficiency measure, so that increases in efficiency can be based upon changes in returns to scale, technology and increased knowledge about the organization.
LeBel (Citation1996) applied cost information for the input and could compute the cost efficiency. Other studies that considered contractors applied SFA to estimate technical efficiency, such as Aalmo and Baardsen (Citation2015) and Bonds and Hughes (Citation2007). Aalmo and Baardsen (Citation2015) conducted an operational study that used the SFA approach to estimate the technical efficiency of 11 harvesting crews, to identify sources of inefficiency among environmental parameters such as terrain roughness, steepness, weather conditions and snow-depth that influenced the productivity of steep terrain harvesting. Their conclusion was that the SFA method could be successfully applied to measure inefficiencies in these logging operations. Carter and Cubbage (Citation1995) conducted a related study where SFA was used to estimate technical efficiency and sources of inefficiency within the Southern U.S. pulpwood timber harvesting industry by assessing efficiency of forest contractors on a crew level. In the forest management organization studies where the efficiency of forest districts was assessed, technical efficiency was the main efficiency measure applied, one example is the study by Šporčić (Citation2009a). In studies assessing the efficiency of individual sawmills, technical efficiency was the main efficiency measure applied (Nyrud and Bergseng Citation2002; Penfield et al. Citation2014). Yin (Citation1998, Citation2000) were able to obtain technical and cost information to decompose efficiency into technical and allocative efficiency. As such, these studies were able to estimate the cost and allocative efficiency of different sawmills. We found it is rather common to apply panel data to study productivity change over time. More than 16 studies estimate and compare the efficiency scores of several years or periods (Nyrud and Bergseng Citation2002; Bogetoft et al. Citation2003; Nyrud and Baardsen Citation2003; Vahid and Sowlati Citation2007; Helvoigt and Adams Citation2008, Citation2009; Kao Citation2013; Zadmirzaei et al. Citation2015; Obi and Visser Citation2017a; Xiong et al. Citation2018; Zhang et al. Citation2018; Kropivšek and Grošelj Citation2019; Shahi and Dia Citation2019; Wang et al. Citation2019; Chen et al. Citation2020; Toma et al. Citation2020). Six studies applied Malmquist indices to evaluate the changes in productivity over time (Kao Citation2000; Sowlati and Vahid Citation2006; Salehirad and Sowlati Citation2007; Lin and Ge Citation2019; Kropivšek et al. Citation2019; Obi and Visser Citation2020). To reduce the risk of using extreme values from a particular year some studies use data from several years to estimate average values (Kovalčík Citation2018, Citation2020).
Returns to scale
Depending on the scope of the intended research, studies in the forestry sector have proposed different approaches for applying DEA and SFA. All of the DEA studies applied the Constant Returns to Scale (CRS) and Variable Returns to Scale (VRS) technologies, with the exception of Shiba (Citation1997) and Penfield et al. (Citation2014), which only applied one of them. The efficiency measures produced by the CRS and VRS technologies are often compared in the studies and different types of sensitivity analyses are conducted to verify and compare the efficiency from these two technologies, as in Bogetoft et al. (Citation2003). The choice of scale assumption is crucial in setting up a model.
Choice of DMUs and inputs and outputs
As mentioned by Bogetoft (Citation2012), a major issue to consider when applying DEA is the choice of DMUs and appropriate inputs and outputs. These must have relevance and the DMU should have a certain managerial control. An example of this is Viitala and Hänninen (Citation1998), where each manager on the forest board could distribute resources freely amongst activities (outputs). In this example, each forest board is regarded as a DMU. The same can be seen in the sawmill studies, where each sawmill is considered a DMU (Yin Citation1998). Another common approach is to pool data for the same districts or units over a number of years, so the number of DMUs increases (Diaz-Balteiro et al. Citation2006; Kovalčík Citation2020; Gutiérrez and Lozano Citation2020). Bogetoft et al. (Citation2003) and LeBel (Citation1996) pooled data over 3 and 6 years, respectively, so that a contractor’s annual performance represented a DMU. While there is no fixed rule, an often encountered rule-of-thumb is that the number of DMUs relative to 3 times the sum of input and output variables should be at least 1 (Dyson et al. Citation2001). It was found that more than 72% of the studies complied with this criterion (, panel D).
Benchmarking categories
According to the classification developed by Andersen and Pettersen (Citation1996), every study can be categorized as performance benchmarking because they all assess efficiency, to identify the best performers. Studies of the sawmill industry can be categorized as competitive benchmarking, as data from several companies was included in each study. It was not possible to categorize any of the studies as process benchmarking or strategic benchmarking. The majority (81%) of forest management organization studies can be characterized as internal benchmarking, because the different units are compared within the same organization (, panel E). All studies concerned government or public organizations, with the exception of Bogetoft et al. (Citation2003), which investigated a private cooperative. Data used in these forest management organizations studies was mostly obtained directly from forest bureaus and district offices (Shiba Citation1997; Kao Citation2000; Korkmaz Citation2011). LeBel's (Citation1996) study of forest contractors can be characterized as competitor benchmarking. Besides the assessment of performance, field inspections were conducted to assess equipment and the working process, like the sorting of timber. According to Andersen and Pettersen (Citation1996) framework, this study could potentially also be categorized as process benchmarking. All benchmarking studies of forest industries were assessed as competitive. Benchmarking studies on forest operations were assessed as 40% internal and 60% competitive (, panel E).
Information sources
Data applied in the forest management organization studies was primarily based on data from forest districts, while the forest industrial studies mainly applied data from national statistics (Yin Citation1998; Nyrud and Baardsen Citation2003), consultancies or directly from sawmills (Penfield et al. Citation2014). The data obtained for the forest contractors came from harvest operations. The number of inputs and outputs ranged from two to more than nine. Data entered as cost or revenues or biophysical quantities (e.g., volume of forest various forest products, volume stock, numbers of workers). A limited number of studies include environmental variables which may affect productivity (Aalmo and Baardsen Citation2015; Aalmo et al. Citation2020) (see Supplementary Information Table S1).
Discussion
General discussion of review results
Focus of the benchmarking studies
The forestry sector strives for increased efficiency in production and environmental performance. It is crucial for the sector that it is continuously able to increase competitiveness with reduced costs and lower environmental impacts. One approach to understand what drives production efficiency is applying advanced benchmarking. The current study reviews the applications of Data Envelopment Analysis and Stochastic Frontier Analysis in forestry. More than 81% of the studies apply DEA only, 5% apply SFA only, and 14% both DEA and SFA. Therefore, the scope of this discussion is mainly focused on DEA. We found that the majority of studies (about half) have investigated the efficiency of forest management organizations at a regional or national scale with the main part addressing public or state-owned forest districts. More than one-third of the studies focused on benchmarking forest industries and one fifth benchmarked forest operations. This may also reflect that benchmarking is one of the most common tools applied by business for comparing their competitiveness and making strategic decisions about organizational development (Bain & Company Citation2018). We found that the number of studies focusing on internal benchmarking was comparable to the number of studies focusing on competitive benchmarking, but it varied between three categories of focus areas of benchmarking. Forest management organization studies addressed internal benchmarking more frequently in evaluating the performance within their organization or company. Benchmarking of forest industries was often framed as competitive benchmarking, as data from several companies was included in each study. Internal benchmarking is useful for initiating a process of identifying, sharing, and using the knowledge and practices inside an organization and to learn how the organization may improve its performance (O’Dell and Grayson Citation1998). Competitive (external) benchmarking is important for managers and boards to compare the performance of their respective organizations with competitors and best-in-class companies. Selection criteria for when to implement either have been discussed by Southard and Parente (Citation2007).
DEA and SFA applications in forestry compared to other domains
We found 56 applications of DEA or SFA in the forestry domain. However, a similar Web of Science search, which only included the search term TS = (“stochastic frontier analysis“ OR SFA OR DEA OR ”data envelopment analysis”) resulted in 33,113 records. The major categories included operations research and management science (4657 records), economics (4268 records), management (4107 records) and environmental science (2565 records). This may indicate that benchmarking studies within the forestry sector are less frequent than in other sectors and research domains.
We found 56 studies, which complied with our inclusion criteria. However, a total of 96 studies (of 33,113 records) in Web of Science, which use the terms of stochastic frontier analysis, data envelopment analysis, SFA OR DEA have been classified as belonging to the forestry research field. The difference is mainly caused by the inclusion criteria that the study should include an application of DEA or SFA on forest-related data. Not surprisingly, research fields such as business economics (25.8%), engineering (17.3%), operations research management science (14.2%), and computer science (9.5%) dominate the literature. Interestingly, agriculture represents 6% of all studies compared to only 0.3% presenting evaluations in forestry. A recent study on dynamic DEA found that most studies address efficiency analysis in the agriculture and farming sector, followed by banking and energy sectors (Mariz et al. Citation2018). This may indicate that the uptake of modern benchmarking may also be higher in the agricultural sector than in the forestry sector. Examples document that benchmarking has been applied extensively in agricultural practice. One such example is the UK Farm Business Survey (farmbusinessurvey.co.uk) which provides online information on the physical and economic performance of farm businesses in England and Wales. Farmbench (ahdb.org.uk/farmbench) is another online tool which farmers can anonymously sign up to and compare key performance indicators (KPI) with neighboring, local or national farmers.
Geographical distribution of studies in forestry and other domains
The geographical distribution of the 33,113 records is presented in . Comparing this with we see overlaps with some countries, e.g. United States, China, Canada, and Iran. However, it is also clear that some continents and a lot of countries are not covered in the forestry literature. Missing countries include, e.g., the Russian Federation and Brazil, which account for more than 32% of the world’s forest (FAO Citation2020) and a forestry sector, which is important for their national economies. This could also indicate a mismatch between supply of benchmarking studies and demand for improving efficiency analysis in the forestry sector. Such geographical mismatches have been studied in other research domains, e.g. climate change (Pasgaard and Strange Citation2013; Pasgaard et al. 2015). It should be noted that the Web of Science country classification is based on a search in the affiliation field. This means for some of the records the author addresses may not reflect the case country of the research. However, all 56 applications of DEA or SFA in forestry did include author addresses from the case country. We applied English search terms, which may cause a language bias and lead to an underestimation of publication written in non-English languages.
Table 2. Rank, countries/regions, records, and percentage of all DEA and SFA records found in Web of Science (December 2020)
Identifying relevant input, output, and environmental variables
The studies presented a large range of input and output variables as well as environmental variables. Selecting the best possible variables and aggregation of data may be facilitated through discussions with various stakeholders who understand the relevance of various decision-making units (DMUs) and the production process one wants to model. Expert knowledge may also be necessary to select potential useful variables for the benchmarking analysis. DMUs can be identified at multiple levels: from a highly aggregated level (e.g., harvester operator organization) to a very low level (e.g., individual harvester operators). Furthermore, the DMUs must be comparable in the sense that they perform similar tasks and transform the same types of inputs to the same types of outputs, cf. also Golany and Roll (Citation1989).
Ideally, the selection of input and output variables should characterize the technology, which describes the production. However, sometimes the criteria for the selection of inputs and outputs are primarily based on availability of data in databases and relevant literature (Obi and Visser Citation2017b). Bogetoft (Citation2012) recommends that the selection should be comprehensive and suggest organizing the variables into a hierarchy, where higher at the top we have the overall inputs and outputs level variables reflecting the properties of lower level ones. If only lower level variables are available, one should assure that all facets of the overall variables are taken into account when selecting candidate variables. Generally, the studies apply inputs (e.g., labor, machine time, fuel) which represent the resources used in the production process and outputs (e.g., cubic meters of harvested wood of different assortments) which represent the resulting outcomes. The underlying assumption is that more inputs lead to more outputs and thus a positive correlation between inputs and outputs. Environmental variables (e.g., terrain ruggedness (Aalmo et al. Citation2020)) represent operating conditions that directly affect the production process, but which are beyond the control of the DMUs. In the modeling, environmental variables can sometimes be dealt with as non-controllable inputs (if this facilitates the production) or outputs (if they complicate the production), while in other cases one must specifically account for these environmental conditions via the potential peer units such that DMUs are only benchmarked against other DMUs operating in similar or worse environments. Related studies have applied DEA to investigate the environmental efficiency of the forestry sector, recognizing that forest production also involves the production of environmental externalities (Lin and Ge Citation2019).
There are a number of issues to consider in the selection of variables: (i) the number of variables relative to the number of DMUs; (ii) factor measurement and (iii) correlation of variables. The first issue is particularly relevant in DEA. If the number of inputs and outputs is large relative to the number of DMUs then DEA may lose discriminatory power. We found when applying a rule of thumb that the ratio between number of DMUs and 3 times the sum of inputs and outputs should be higher than 1 (Dyson et al. Citation2001), that more than 72% of the studies did meet this criteria. If the ratio is smaller than 1 the risk is that the discriminatory power is too small. Some of the studies suggest alternative procedures to overcome too few DMUs, e.g., by using overlapping windows of data and window analysis (Helvoigt and Adams Citation2008; Kropivšek and Grošelj Citation2019). A number of studies emphasized the need to ensure a sufficient number of DMUs compared to the number of input and output variables (Diaz-Balteiro et al. Citation2006; Šporčić et al. Citation2009a). The second issue is that one must be careful not to mix indices, percentages, or ratios with absolute numbers. Improper mixing can result in incorrect efficiency results. All studies complied with this criteria except Kropivšek and Grošelj (Citation2019) which uses leverage and liquidity ratios mixed with e.g. size of assets, capital, number of employees, net sales, gross profit, and gross value added. Third, in an effort to reduce the number of variables in order to increase discriminatory power one might be tempted to remove highly correlated inputs (outputs). Dyson et al. (Citation2001) warn against this practice as removing highly correlated inputs (outputs) can substantially alter the efficiency analysis. One can only safely remove one of two highly correlated inputs (outputs) if one is a scalar multiple of the other.
Selection of DEA models
An important property of a benchmarking approach is its ability to reflect and respect the characteristics of the technology that we aim at modeling (Bogetoft Citation2012). One weakness of DEA is that it does not include any statistical tests to help the user to select the most appropriate model. Use of stakeholders and expert knowledge may be insufficient to select the most robust models. It is generally recommended to use a flexible model in the sense that its structure can adapt to data, and cope with changes in the number of variables and range sizes (including procedures for excluding outliers), covariance between input variables or output variables (Smith Citation1997). Several accompanying methods have been developed to assist in the identification of more robust models. They include the F-tests, Principal Component Analysis, regression-based tests and bootstrapping approaches which are applied to evaluate the inclusion and exclusion of input/output variables (Pastor et al. Citation2002). Others point at applying probabilistic/combinatorial approaches that provide DEA efficiency scores which are robust against the selection of inputs/outputs to be included in the model (Landete et al. Citation2017). None of the studies included in this review report selection procedures and test for robustness.
Potential for making benchmarking useful in forestry
This review revealed that the majority of studies used data from either public statistics, reports from forest or company offices, surveys or field plots and harvest operations. While this type of analysis allows the analyst to investigate a range of operational, tactical or strategic decisions, the analysis is based on historical and potentially outdated information. Furthermore, the studies rarely involve industrial partners or report any stakeholder interaction. Only a few examples of the reviewed studies involve authors with affiliations from the private sector parties, e.g., consultancies (Helvoigt and Adams Citation2008, Citation2009) or the bank sector (Zhang et al. Citation2018). Reviewing the 56 studies only revealed that Obi and Visser (Citation2017a, Citation2017b, Citation2018, Citation2020), Shahi and Dia (Citation2019), Aalmo and Baardsen (Citation2015), and Gutiérrez and Lozano (Citation2020) discuss how their studies could provide some useful decision support to forest companies, forest industries policymakers, and general industry stakeholders involved in the measurement and overall improvement of performance. This may indicate that DEA and SFA studies are mainly prepared by specialists with high technological skills. Even though most managers have experience with key performance indicators, DEA and SFA may be difficult to comprehend and implement for managers. The potential gain from using benchmarking is then constrained by both applying outdated information and users/decision makers having insufficient technical and theoretical knowledge to run the analysis on their own. However, taking benchmarking to the next level, the forestry sector may benefit significantly from developing software solutions which combine state-of-the-art benchmarking methods with an easy and intuitive user interface (Bogetoft Citation2012). Digital and technological progress has created new opportunities for the forestry sector (Müller et al. Citation2019). Today data on harvested volumes, assortment and tree species distributions, log sizes, fuel consumption and other types of information are stored by on board computers in harvesters. Data that are highly relevant for characterizing the productivity (Olivera et al. Citation2016) of forest operations. Transmission of such data to databases may allow for day-to-day benchmarking analysis of forest operations, a daily comparison of performance against past operations. Such data capture and integration with modern benchmarking software would make it possible to create learning labs for the machine operator or owner who can explore operational, tactical and strategic decisions (Bogetoft Citation2012). They could serve as online monitoring and interactive learning tools and allow the user to explore various features of a firm (Post and Spronk Citation1999; Bogetoft et al. Citation2006). Interactive benchmarking would allow the user to ask a lot of what-if questions. This may include selecting and testing various data sets, the KPIs of the firm, choosing a model and deciding who to be compared to, identifying peers in the sector or inside the firm, evaluating dynamic development over time, and extracting reports which may form part of the information needed to make long-term strategic decisions. Comparisons to other sectors, e.g., agriculture, and observations of tremendous gains across sectors in efficiency due to digitization document large potentials for advancing modern benchmarking in the forestry sector.
Another potential future application of benchmarking may be to facilitate incentive provision. The operator may also be the machine owner, however often the machine owner may have several operators in the team or sub-contract with other machine owners. Even if a benchmarking proves that efficiency gains could be obtained by the machine operators and this information is part of the operational plan, there are no guarantees that the operators will follow the plan. The operators involved in the plan must also have incentives to implement it, and the incentives must be attractive enough to make the operators participate. The benchmarking literature describes this relationship between the benchmarking model and the motivational aspect as either implicit or explicit. An implicit or informal relationship is found when the mere existence of a model improves behavior because performance now receives more attention in the minds of the operators. A more explicit and formalized relationship is found when the payment to an operator is made a direct function of the benchmarking results (Bogetoft Citation2012). Benchmarking and so-called yardstick competition has been suggested for regulating such asymmetric incentive structures (Agrell et al. Citation2005, Citation2007), mostly for regulating large monopolistic companies. However, it may be relevant for future work to address how this and other benchmarking approaches could be applied for increasing competition and efficiency within and between organizations or firms in the forestry sector.
Conclusion
The efficiency of the forestry sector has increased for the last 50 years, mainly as a result of technological development and mechanization of processes. Benchmarking may be an important part of exploring new opportunities for increasing efficiency in the forestry sector. This review found that almost half of the benchmarking studies in forestry have attempted to estimate the efficiency of forest management organizations at a regional scale, mostly being public or state-owned forest districts. A bit more than one-third of the studies have focused on benchmarking forest industries and one-fifth benchmarking of forest operations. Surprisingly, the studies are in most cases not geographically overlapping with forest rich countries. We also found that almost half of the studies evaluate the productivity change over time. Most often, the productivity data is based on annual reports or longer periods (months to several years). We suggest that the forestry sector may benefit further from the potential advantages of digitalization and use of interactive benchmarking based on frequently updated performance data automatically captured from harvesters and forwarders. The challenge is to establish incentives and make the benchmarking tools user-friendly and interesting learning labs for the potential users in the forestry sector.
Supplemental Material
Download MS Word (493.9 KB)Acknowledgements
This work has received funding from the Bio-Based Industries Joint Undertaking under the European Union’s Horizon 2020 research and innovation programme under grant agreement No 720757.
Disclosure statement
No potential conflict of interest was reported by the author(s).
Supplementary material
Supplemental data for this article can be accessed on the publisher’s website.
Additional information
Funding
References
- Aalmo GO, Baardsen S. 2015. Environmental factors affecting technical efficiency in Norwegian steep terrain logging crews: a stochastic frontier analysis. J For Res [Internet]. 20(1):18–23. doi:https://doi.org/10.1007/s10310-014-0466-x.
- Aalmo GO, Kerstens PJ, Belbo H, Bogetoft P, Talbot B, Strange N. 2020. Efficiency drivers in harvesting operations in mixed Boreal stands: a Norwegian case study. Int J For Eng [Internet]. 1–13. doi:https://doi.org/10.1080/14942119.2020.1778980
- Agrell PJ, Bogetoft P. 2017. Regulatory benchmarking: models, analyses and applications. Data Envel Anal J [Internet]. 3(1–2):49–91. doi:https://doi.org/10.1561/103.00000017.
- Agrell PJ, Bogetoft P, Halbersma R, Mikkers MC. 2007. Yardstick Competition for Multi-Product Hospitals - An Analysis of the Proposed Dutch Yardstick Mechanism. Utrecht, The Netherlands. https://www.econstor.eu/handle/10419/208533http://www.ssrn.com/abstract=1816288
- Agrell PJ, Bogetoft P, Tind J. 2005. DEA and dynamic yardstick competition in scandinavian electricity distribution. J Product Anal [Internet]. 23(2):173–201. doi:https://doi.org/10.1007/s11123-005-1327-6.
- Andersen B, Pettersen PG. 1996. The benchmarking handbook. London: Chapmann and Hall.
- Bain & Company. 2018. Management Tools & Trends. Brief [Internet]. [accessed 2021 Apr 27]. https://www.bain.com/insights/management-tools-and-trends-2017/.https://www.bain.com/insights/management-tools-and-trends-2017/
- Banker R, Charnes A, Cooper W. 1984. Models for estimation of technical and scale inefficiencies in data envelopment analysis. Manage Sci. 30(9):1078–1092. doi:https://doi.org/10.1287/mnsc.30.9.1078.
- Bogetoft P. 2012. Performance benchmarking. Boston (MA): Springer US.
- Bogetoft P, Bramsen JM, Nielsen K. 2006. Balanced benchmarking. Int J Bus Perform Manag [Internet]. 8(4):274–289. doi:https://doi.org/10.1504/IJBPM.2006.009609.
- Bogetoft P, Otto L. 2011. Benchmarking with DEA, SFA, and R. [place unknown]: Springer Science+Business Media.
- Bogetoft P, Thorsen BJ, Strange N. 2003. Efficiency and merger gains in the Danish forestry extension service. For Sci. 49(4):585–595.
- Bonds MH, Hughes DR. 2007. On the productivity of public forests: a stochastic frontier analysis of Mississippi school trust timber production. Can J Agric Econ. 55(2):171–183. doi:https://doi.org/10.1111/j.1744-7976.2007.00086.x.
- Carter DR, Cubbage FW. 1995. Stochastic frontier estimation and sources of technical efficiency in southern timber harvesting. For Sci. 41(3):576–593.
- Charnes A, Cooper WW, Rhodes E. 1978. Measuring the efficiency of decision making units. Eur J Oper Res [Internet]. 2(6):429–444. doi:https://doi.org/10.1016/0377-2217(78)90138-8.
- Chen N, Qin F, Zhai Y, Cao H, Zhang R, Cao F. 2020. Evaluation of coordinated development of forestry management efficiency and forest ecological security: a spatiotemporal empirical study based on China’s provinces. J Clean Prod [Internet]. 260:121042. doi:https://doi.org/10.1016/j.jclepro.2020.121042.
- Cooper WW, Seiford LM, Tone K. 2000. Data envelopment analysis. Boston (MA): Kluwer Academic Publishers.
- Diaz-Balteiro L, Casimiro Herruzo A, Martinez M, González-Pachón J. 2006. An analysis of productive efficiency and innovation activity using DEA: an application to Spain’s wood-based industry. For Policy Econ [Internet]. 8(7):762–773. doi:https://doi.org/10.1016/j.forpol.2005.06.004.
- Dyson RG, Allen R, Camanho AS, Podinovski VV, Sarrico CS, Shale EA. 2001. Pitfalls and protocols in DEA. Eur J Oper Res [Internet]. 132(2):245–259. doi:https://doi.org/10.1016/S0377-2217(00)00149-1.
- FAO. 2020. Global forest resources assessment 2020: main report. Rome: FAO. http://www.fao.org/documents/card/en/c/ca9825en.
- Färe R, Grosskopf S, Norris M, Zhongyang Z. 1994. Productivity growth, technical progress, and efficiency change in industrialized countries. Am Econ Rev [Internet]. 84(1):66–83. https://www.jstor.org/stable/2117971
- Golany B, Roll Y. 1989. An application procedure for DEA. Omega [Internet]. 17(3):237–250. https://linkinghub.elsevier.com/retrieve/pii/0305048389900297
- Gough D, Oliver S, Thomas J. 2012. An introduction to systematic reviews. London: SAGE Publications.
- Gutiérrez E, Lozano S. 2020. Cross-country comparison of the efficiency of the European forest sector and second stage DEA approach. Ann Oper Res [Internet]. doi:https://doi.org/10.1007/s10479-020-03756-9
- Helvoigt TL, Adams DM. 2008. Data envelopment analysis of technical efficiency and productivity growth in the US Pacific Northwest sawmill industry. Can J For Res [Internet]. 38(10):2553–2565. doi:https://doi.org/10.1139/X08-107.
- Helvoigt TL, Adams DM. 2009. A stochastic frontier analysis of technical progress, efficiency change and productivity growth in the Pacific Northwest sawmill industry. For Policy Econ [Internet]. 11(4):280–287. doi:https://doi.org/10.1016/j.forpol.2009.04.006.
- Kao C. 2000. Measuring the performance improvement of Taiwan forests after reorganization. For Sci. 46(4):577–584.
- Kao C. 2013. Dynamic data envelopment analysis: a relational analysis. Eur J Oper Res [Internet]. 227(2):325–330. doi:https://doi.org/10.1016/j.ejor.2012.12.012.
- Kao C, Yang YC. 1991. Measuring the efficiency of forest management. For Sci. 37(5):1239–1252.
- Korkmaz M. 2011. Measuring the productive efficiency of forest enterprises in Mediterranean Region of Turkey using data envelopment analysis. African J Agric Res. 6(19):4522–4532.
- Kovalčík M. 2018. Efficiency of the Slovak forestry in comparison to other European countries: an application of data envelopment analysis. Cent Eur For J [Internet]. 64(1):46–54. http://content.sciendo.com/view/journals/forj/64/1/article-p46.xml
- Kovalčík M. 2020. Profitability and efficiency of forest contractors in Slovakia—comparison of mountain and lowland regions. Forests [Internet]. 11(4):370. doi:https://doi.org/10.3390/f11040370.
- Kropivšek J, Grošelj P. 2019. Long-term financial analysis of the Slovenian wood industry using DEA. Drv Ind [Internet]. 70(1):61–70. doi:https://doi.org/10.5552/drvind.2019.1810.
- Kropivšek J, Perić I, Pirc Barčić A, Grošelj P, Motik D, Jošt M. 2019. A comparative evaluation of operational efficiency of wood industry using data envelopment analysis and Malmquist productivity index. Drv Ind [Internet]. 70(3):287–298. doi:https://doi.org/10.5552/drvind.2019.1937.
- Kumbhakar SC, Lovell CK. 2003. Stochastic frontier analysis. [place unknown]: Cambridge University Press.
- Landete M, Monge JF, Ruiz JL. 2017. Robust DEA efficiency scores: a probabilistic/combinatorial approach. Expert Syst Appl [Internet]. 86:145–154. doi:https://doi.org/10.1016/j.eswa.2017.05.072.
- LeBel L 1996. Performance and efficiency evaluation of logging contractors using Data Envelopment Analysis. PhD Thesis [Internet]. Virginia: VirginiaTech and Virginia State University. https://vtechworks.lib.vt.edu/handle/10919/27962?show=full
- Lin B, Ge J. 2019. Carbon sinks and output of China’s forestry sector: an ecological economic development perspective. Sci Total Environ [Internet]. 655:1169–1180. doi:https://doi.org/10.1016/j.scitotenv.2018.11.219.
- Mariz FBAR, Almeida MR, Aloise D. 2018. A review of dynamic data envelopment analysis: state of the art and applications. Int Trans Oper Res [Internet]. 25(2):469–505. doi:https://doi.org/10.1111/itor.12468.
- Müller F, Jaeger D, Hanewinkel M. 2019. Digitization in wood supply – a review on how Industry 4.0 will change the forest value chain. Comput Electron Agric [Internet]. 162:206–218. doi:https://doi.org/10.1016/j.compag.2019.04.002.
- Nyrud AQ, Baardsen S. 2003. Production efficiency and productivity growth in Norwegian sawmilling. For Sci. 49(1):89–97.
- Nyrud AQ, Bergseng ER. 2002. Production efficiency and size in Norwegian sawmilling. Scand J For Res [Internet]. 17(6):566–575. doi:https://doi.org/10.1080/02827580260417224.
- O’Dell C, Grayson CJ. 1998. If only we knew what we know: identification and transfer of internal best practices. Calif Manage Rev [Internet]. 40(3):154–174. doi:https://doi.org/10.2307/41165948.
- Obi OF, Visser R. 2017a. Operational efficiency analysis of New Zealand timber harvesting contractors using data envelopment analysis. Int J For Eng [Internet]. 28(2):85–93. doi:https://doi.org/10.1080/14942119.2017.1313489.
- Obi OF, Visser R. 2017b. Influence of the operating environment on the technical efficiency of forest harvesting operations. Int J For Eng [Internet]. 28(3):140–147. doi:https://doi.org/10.1080/14942119.2017.1357391.
- Obi OF, Visser R. 2018. Including exogenous factors in the evaluation of harvesting crew technical efficiency using a multi-step data envelopment analysis procedure. Croat J For Eng. 39(2):153–162.
- Obi OF, Visser R. 2020. Productivity measurement of New Zealand forest harvesting sector using the DEA-Malmquist index. Int J For Eng [Internet]. 1–9. doi:https://doi.org/10.1080/14942119.2020.1770566
- Olivera A, Visser R, Acuna M, Morgenroth J. 2016. Automatic GNSS-enabled harvester data collection as a tool to evaluate factors affecting harvester productivity in a Eucalyptus spp. harvesting operation in Uruguay. Int J For Eng [Internet]. 27(1):15–28. doi:https://doi.org/10.1080/14942119.2015.1099775.
- Pasgaard M, Strange N. 2013. A quantitative analysis of the causes of the global climate change research distribution. Glob Environ Chang. 23(6):1684–1693. doi:https://doi.org/10.1016/j.gloenvcha.2013.08.013.
- Pastor JT, Ruiz JL, Sirvent I. 2002. A statistical test for nested radial dea models. Oper Res [Internet]. 50(4):728–735. doi:https://doi.org/10.1287/opre.50.4.728.2866.
- Penfield PC, Germain R, Smith W. 2014. Assessing the supply chain efficiency of hardwood sawmills in New York state through case study analysis and data envelopment analysis modeling. For Prod J [Internet]. 64(3–4):90–96. doi:https://doi.org/10.13073/FPJ-D-13-00080.
- Petticrew M, Roberts H. 2012. Systematic reviews in the social sciences. A practical guide. Oxford: Blackwell Publishing.
- Post T, Spronk J. 1999. Performance benchmarking using interactive data envelopment analysis. Eur J Oper Res. 115(3):472–487. doi:https://doi.org/10.1016/S0377-2217(98)00022-8.
- Proto AR, Zimbalatti G. 2016. Firewood cable extraction in the southern Mediterranean area of Italy. Forest Sci Technol [Internet]. 12(1):16–23. doi:https://doi.org/10.1080/21580103.2015.1018961.
- Rafiei R, Nourelfath M, Gaudreault J, Santa-Eulalia LA, Bouchard M. 2014. A periodic re-planning approach for demand-driven wood remanufacturing industry: a real-scale application. Int J Prod Res [Internet]. 52(14):4198–4215. doi:https://doi.org/10.1080/00207543.2013.869631.
- Salehirad N, Sowlati T. 2005. Performance analysis of primary wood producers in British Columbia using data envelopment analysis. Can J For Res. 35(2):285–294. doi:https://doi.org/10.1139/x04-154.
- Salehirad N, Sowlati T. 2007. Dynamic efficiency analysis of primary wood producers in British Columbia. Math Comput Model [Internet]. 45(9–10):1179–1188. doi:https://doi.org/10.1016/j.mcm.2006.10.003.
- Shahi SK, Dia M. 2019. Efficiency measurement of Ontario’s sawmills using bootstrap data envelopment analysis. J Multi-Criteria Decis Anal [Internet]. 26(5–6):272–295. doi:https://doi.org/10.1002/mcda.1695.
- Shiba M. 1997. Measuring the efficiency of managerial and technical performances in forestry activities by means of Data Envelopment Analysis (DEA). J For Eng. 8(1):7–19.
- Šporčić M, Martinić I, Landekić M, Lovrić M. 2009a. Measuring efficiency of organisational units in Forestry by nonparametric model. Croat J For Eng. 30(1):1–13.
- Šporčić M, Martinić I, Segotic K. 2009b. Application of Data Envelopment Analysis in ecological research of maintenance of forestry mechanisation. Strojniški Vestn. 55(10):599–608.
- Smith P. 1997. Model misspecification in Data Envelopment Analysis. Ann Oper Res. 73:233–252.
- Southard PB, Parente DH. 2007. A model for internal benchmarking: when and how? Benchmarking An Int J [Internet]. 14(2):161–171. doi:https://doi.org/10.1108/14635770710740369/full/html.
- Sowlati T. 2005. Efficiency studies in forestry using data envelopment analysis. For Prod J. 55(1):49–57.
- Sowlati T, Vahid S. 2006. Malmquist productivity index of the manufacturing sector in Canada from 1994 to 2002, with a focus on the wood manufacturing sector. Scand J For Res [Internet]. 21(5):424–433. doi:https://doi.org/10.1080/02827580600917304.
- Toma P, Miglietta PP, Morrone D, Porrini D. 2020. Environmental risks and efficiency performances: the vulnerability of Italian forestry firms. Corp Soc Responsib Environ Manag [Internet]. 27(6):2793–2803. doi:https://doi.org/10.1002/csr.2002.
- Vahid S, Sowlati T. 2007. Efficiency analysis of the Canadian wood-product manufacturing subsectors: a DEA approach. For Prod J. 57(1–2):71–77.
- Viitala EJ, Hänninen H. 1998. Measuring the efficiency of public forestry organizations. For Sci. 44(2):298–307.
- Wang C, Chu X, Zhan J, Wang P, Zhang F, Xin Z. 2019. Factors contributing to efficient forest production in the region of the three-north shelter forest program, China. Sustainability [Internet]. 12(1):302. doi:https://doi.org/10.3390/su12010302.
- Xiong L, Wang F, Cheng B, Yu C. 2018. Identifying factors influencing the forestry production efficiency in Northwest China. Resour Conserv Recycl [Internet]. 130:12–19. doi:https://doi.org/10.1016/j.resconrec.2017.11.009.
- Yin R. 1998. DEA: a new methodology for evaluating the performance of forest products producers. For Prod J. 48(1):29–34.
- Yin R. 2000. Alternative measurements of productive efficiency in the global bleached softwood pulp sector. For Sci. 46(4):558–569.
- Zadmirzaei M, Limaei SM, Amirteimoori A. 2015. Efficiency analysis of paper mill using data envelopment analysis models (Case study: mazandaran wood and paper company in Iran). J Agric Sci Technol [Internet]. 17(6):1381–1391. http://jast.modares.ac.ir/article-23-3513-en.html
- Zadmirzaei M, Mohammadi Limaei S, Amirteimoori A, Olsson L. 2019. Measuring the relative performance of forest management units: a chance-constrained DEA model in the presence of the nondiscretionary factor. Can J For Res [Internet]. 49(7):788–801. doi:https://doi.org/10.1139/cjfr-2018-0229.
- Zhang K, Yuan B, Li Y. 2018. Efficiency analysis of wood processing industry in China during 2006-2015. IOP Conf Ser Mater Sci Eng [Internet]. 322:52062. doi:https://doi.org/10.1088/1757-899X/322/5/052062