Abstract
Objective: ClearVoice is a single-microphone noise reduction algorithm in Advanced Bionics cochlear implant(CI) systems with the aim to improve performance in background noise. The present study investigated a hypothesised increased effect of ClearVoice if combined with a structural increase of maximum comfort stimulation levels (M-levels) in the CI fitting. Design: We tested performance with ClearVoice (Medium) in four conditions, defined by combined settings of ClearVoice off/on and with/without 5% increase of M-levels. The main outcome measures were the Acceptable Noise Level (ANL) and the speech reception threshold in continuous background noise (SRTn). Study sample: Participants were 16 experienced cochlear implant recipients with Advanced Bionics implants and a Naida Q70 processor. Results: The ANL significantly improved by using either ClearVoice or an increase of M-levels. Combining both settings gave the largest improvement in ANL. For the SRTn, we found a small, but significant interaction between ClearVoice and an increase of M-levels, implying that ClearVoice improved speech understanding slightly, but only if combined with a 5% increase of M-levels. Conclusions: Optimal profit from ClearVoice is obtained if combined with a structural 5% increase of M-levels.
Introduction
Cochlear implants (CIs) are an accepted treatment for severe to profound sensorineural hearing loss, with significant improvements in speech perception and quality of life (Gaylor et al. Citation2013). However, understanding speech in background noise is difficult for many CI recipients. In an attempt to improve speech perception in noise, some contemporary sound processors of CI systems contain a single microphone noise reduction algorithm (NRA), among other techniques like directional microphones. Several studies reported that single-microphone NRAs has been able to provide significant speech perception improvements in CI recipients (Buechner et al. Citation2010; Dawson, Mauger, and Hersbach Citation2011; Mauger, Arora, and Dawson Citation2012; Koch et al. Citation2014). The largest improvements were found for steady-state speech weighted noise. For example, Dawson and colleagues reported an improvement of nearly 2 dB in signal-to-noise ratio (SNR) for steady-state speech-weighted noise and around 1 dB for party noise in an adaptive speech test with a target of 50% correct intelligibility.
Furthermore, the noise tolerance of CI recipients, as measured with the acceptable noise level (ANL) test, may be improved due to single-microphone NRAs (Dingemanse and Goedegebure Citation2015). The ANL is a subjective measure that quantifies the individual’s ‘willingness to listen to speech in background noise’ (Nabelek et al. Citation2006). First, the listeners are asked to adjust the loudness level to a level that they perceive as most comfortable (Most Comfortable Level (MCL) for listening to running speech. Second, listeners seek the maximum level of background noise (BNL) that they are willing to put up with while following the running speech presented at their individual MCL (cf Nabelek et al. Citation2006). Subtracting the BNL value from the MCL value yields the ANL measure that indicates a listeners’ noise tolerance. It has been shown that the ANL measure is sensitive for perceptual effects of NRAs (Mueller, Weber, and Hornsby Citation2006; Peeters et al. Citation2009; Pisa, Burk, and Galster Citation2010).
In this study, we focussed on the NRA ClearVoice, a proprietary NRA developed by Advanced Bionics (Stäfa, Switzerland), because for this NRA mixed results were reported for speech-in-noise understanding. The NRA ClearVoice aims to reduce noise by the application of short-term gain reductions, depending on the instantaneous SNR which is obtained by comparing the actual signal level with a long-term estimation of the noise level (Advanced Bionics Citation2012). Some studies reported a significantly better speech understanding in noise with ClearVoice activated (Buechner et al. Citation2010; Noël-Petroff et al. Citation2013; Koch et al. Citation2014), but other investigators did not find a significant effect on speech understanding in noise, at least in most of the tested conditions (Kam et al. Citation2012; Holden et al. Citation2013; Dingemanse and Goedegebure Citation2015). It is remarkable that the studies showing a significant effect of ClearVoice allowed volume control adjustments in the test situation, while the studies that did not find a significant effect did not allow volume adjustments or most subjects did not change the volume. Brendel et al. (Citation2012) suggested that an increase in volume could enhance the effect of ClearVoice. They investigated the effect of ClearVoice in combination with a volume increase of 5% by raising the maximum levels (M-levels) that define the amount of electrical stimulation at the most comfortable level (MCL). They reported that most participants showed an increase in the percent correct score on a sentence-in-noise test with a fixed speech-to-noise ratio (SNR) of 10 dB.
However, several questions may arise with respect to how an increase in volume setting or M-levels may influence speech understanding performance in noise. A first question is if an increase in volume may have impact on speech understanding in noise on its own. As both the noise and speech level are influenced by a volume change, at first glance no substantial differences are expected. However, an increase in volume or equivalently M-levels leads to an increase in the slope of the input output curve. If the SNR is positive, an increase of the slope means that the SNR in the electrical domain becomes more positive, making a positive effect on speech intelligibility in noise conceivable.
A second question is whether a volume increase may cause that stimuli become too loud when the NRA is not active. In the fitting process, maximum comfort levels and threshold levels are usually optimised for situations without background noise. In many daily situations, the amount and type of background noise is varying over time. It is unlikely that CI recipients change the volume setting or the used programme in reaction to every change in background noise level. Therefore, it is important to investigate how an increase of M-level changes the most comfortable level (MCL), and the maximum tolerance level to background noise.
The objective of this study was to answer the following questions:
Does the effect of the NRA ClearVoice on noise tolerance and speech-in-noise understanding increase if combined with raised maximum comfort levels?
What is the effect of an increase of maximum comfort levels without the NRA ClearVoice on MCL, noise tolerance, and speech-in-noise understanding?
Materials and methods
Study design and procedures
This prospective study used a balanced repeated measures design with the factors noise reduction algorithm (NRA) and difference in maximum comfort levels (ΔM-level). M-level is the name for the maximum comfort levels in Advanced Bionics’ software. The M-levels are basic fitting parameters used to define the amount of electrical output at the most comfortable level. Factor NRA had two conditions, NRA-off and NRA-on. Factor ΔM-level had also two conditions, a difference in level of 0% and 5%. A ΔM of 0% means that the unchanged M-levels of the daily-used programme were used. A ΔM of 5% means an 5% increase of the M-levels of the daily used programme. The amount of 5% is chosen based on volume changes reported by Noël-Petroff et al. (Citation2013) and current clinical practice (Hehrmann et al. Citation2012).
Measurements of the Speech Reception Threshold in noise (SRTn) at 50% performance level and noise tolerance as measured with the ANL test were repeated four times within participants for the combinations of conditions of factors NRA and ΔM-level. These combinations were balanced with a balanced 4 × 4 Latin Square over participants. As this type of design has a risk of introducing order effects, like a learning effect or a fatigue effect, we included an evaluation of order effects in the statistical analyses of the results.
The NRA ClearVoice that was investigated in this study is a proprietary single-microphone noise reduction algorithm developed by Advanced Bionics (Stäfa, Switzerland). The NRA has the aim to improve overall signal-to-noise ratio (SNR) by the suppression of frequency channels lacking information useful for understanding speech. The suppression is based on an instant comparison of the current signal level in a channel with an estimation of the background noise level in the channel over the last 1.6 s. In this study, we used the Medium setting of ClearVoice, giving an instant suppression up to −12 dB (Advanced Bionics Citation2012).
The M-levels and T-levels of the daily used programme were used as a starting point to create four experimental programmes, each containing one of the four combinations of NRA and ΔM-level. An audiologist programmed the CI-processor with these four programmes. The experimenter and participants were not informed about the settings in each programme of the CI. The daily used programme was created earlier during a regular clinical appointment. In a clinical appointment, M-levels were set to a most comfortable level for each electrode with an ascending loudness judgment procedure. The threshold levels (T-levels) were set to the threshold levels for each electrode, using an ascending presentation, followed by a standard bracketing procedure. After that, the overall level of the M-level profile was adjusted to make live speech sound comfortable and easily understandable. Additional fine-tuning of the T- and M-level profiles was sometimes applied based on the feedback of the CI user and the professional judgement of the clinical audiologist. In the clinical fitting procedure, no increase of M- or T-level was used if ClearVoice was switched on. During the test session no volume setting adjustments were allowed.
All different test conditions were measured in one test session. First, a practice run of the SRTn test was done to make the participants familiar with the voice and the task and to obtain a first estimate of a participants SRTn. Secondly, a practice condition of the ANL test was done. Then, an SRTn test and an ANL test were performed with each of the CI programmes in the Latin-square balanced order. The SRTn of the practice run was used as starting point for the measurement of the SRTn in the test conditions.
Participants
Sixteen users of an Advanced Bionics cochlear implant (HiRes 90K implant) participated in this study. Participants ranged in age from 43 to 85 years (group mean 70 years; SD = 11.9). All participants used at least 14 active electrodes and HiRes120 sound processing. All participants were unilateral CI users with a group mean of 6.1 (SD 2.1) years of CI use and at least one year of use. All but one used the noise reduction algorithm ClearVoice in their daily programme. The input dynamic range setting was 55 or 60 dB (2 × 55 dB; 14 × 60 dB). Some participants were accustomed to wear a hearing aid in the non-implanted ear, but they did not wear it during the tests. All participants were Dutch native speakers. For inclusion in this study, a phoneme score of at least 70% on clinically used Dutch consonant-vowel-consonant word lists was required. Participants were required to sign a written informed consent form before participating in the study. The Erasmus Medical Centre Ethics Committee approved the study protocol for use with CI recipients.
Speech-in-noise test
Speech understanding in noise was measured with Dutch female-spoken, unrelated sentences in steady-state speech spectrum noise (Versfeld et al. Citation2000). The presentation level of the sentences was fixed at 70 dB (SPL). This speech level is often reached in noisy situations (Pearsons, Bennett, and Fidell Citation1977). The noise started 3 s before the speech and ended 0.5 s after the speech. The noise level was varied following an adaptive procedure to estimate the Speech Reception Threshold in noise (SRTn), the signal-to-noise ratio that yields 50% of correctly understood words, using 26 sentences (Dingemanse and Goedegebure Citation2015). The SRTn was defined as the average SNR over the last 23 presentation levels. (the 27th level was calculated from the response on the 26th sentence).
Acceptable noise level test
The ANL is the difference between the measured most comfortable level (MCL) for running speech and the maximum tolerated background noise level (BNL) while listening to speech. The running speech consisted of connected unrelated sentences of the speech-in-noise lists, with intervals of 500 ms of silence between them. The noise was steady-state speech spectrum noise. The listeners were given oral and written instructions, which were Dutch translations of the instructions in Nabelek et al. (Citation2006). The participants had to find their MCL in three steps. First they were asked to turn up the speech level until it was too loud, and after that to turn it down until it was too soft. In the final step the participant had to select the MCL. The BNL was measured in a similar manner. After listing to a high noise level and a low noise level, the participants’ task was to select the maximum BNL that he/she was willing to accept while following the speech. For each test condition the MCL and BNL procedures were repeated 3 times and the mean values were used for calculation of the ANL.
Equipment
All testing was performed in a sound-treated room. Participants sat one metre in front of a Westra 251 loudspeaker that was connected to a Madsen OB822 audiometer, a MOTU UltraLite mk3 Hybrid soundcard, and a Macbook pro notebook. All participants were tested with the same new Naida Q70 processor and a new T-mic (Advanced Bionics, Stäfa, Switzerland).
Sample size and data analysis
An a priori power analysis in G*Power software (Faul et al. Citation2009) indicated that a sample of 16 people would be needed to detect a clinically significant ANL difference ≥3 dB (Olsen and Brännström Citation2014) and a clinically significant difference of 10 percentage points in the word score on a speech-in-noise test, with 80% power, using a repeated-measures model with four repeated measures and alpha at .05. The calculation was based on within-group standard deviations (ANL: SD = 6.6 dB, SRTn: SD = 4.2 dB) and correlations between repeated measurements of 0.73 for ANL and 0.9 for SRTn. These numbers were based on previous research (Dingemanse and Goedegebure Citation2015).
For research questions 1 and 2, a repeated measures ANOVA was used with the factors NRA and ΔM for MCL, ANL and SRTn tests.
Results
Acceptable noise levels
A normality check revealed that the ANL data was normally distributed for each condition. shows the group mean ANL values for the four conditions, with subsequent better noise tolerance (lower ANL values) for ΔM5%, NRA-on and the combination of ΔM5% and NRA-on, respectively. A repeated measures ANOVA with the factors NRA and ΔM showed that both the factors NRA [F(1,15) = 19.1, MSE = 8.7, p = 0.001, η2p = 0.56] and ΔM [F(1,15) = 5.2, MSE = 12.0, p = .038, η2p = 0.26] had a statistically significant effect on the ANL values. The effect of NRA-on was a decrease of 2.1 dB in ANL, the effect of ΔM5% a decrease of 0.9 dB and the combined effect a decrease of 5.2 dB, which is 2.2 dB more than the summed effect of both factors (3.0 dB). However, the interaction of both factors was not statistically significant [F(1,15) = 1.2, MSE = 16.5, p = 0.27, η2p = 0.07], indicating that the decrease of ANL for the combined application of NRA and ΔM5% is dominated by the summed effect of both factors. The difference between the combined condition (NRA-on, ΔM5%) and the reference condition (NRA-off, ΔM0%) was post hoc tested with a paired t-test, showing that the difference was highly significant and the effect size r was large (t(15) = 5.81, p < 0.0001, r = 0.83). The effect of ΔM5% for NRA-off was 0.9 dB and was not significant on a post hoc paired t-test (t(15) = 0.65, p = 0.53, r = 0.04).
Figure 1. Mean acceptable noise level (ANL) values for the four combinations defined by combined settings of noise reduction algorithm (NRA) off/on and with/without additional 5% increase of M-levels (ΔM). Error bars represent 95% confidence intervals.
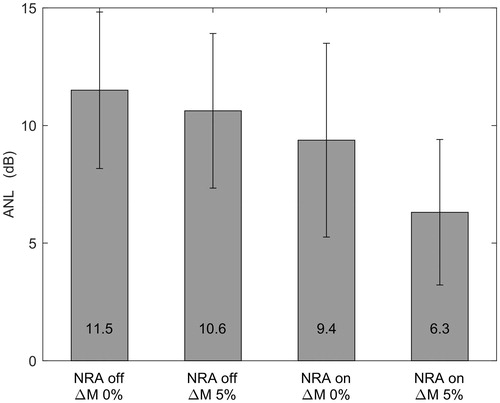
A subsequent analysis with an additional between-subject factor “test sequence” did not change the significance of the findings and none of the interactions of the factors with test sequence reached significance, indicating that the obtained results were not affected by order or fatigue effects.
Participants substantially differed in their noise tolerance. The reference ANL values (from condition NRA-off, ΔM 0%) ranged from 3.3 to 22.7 dB. A significant correlation was found between the ANL baseline score and the ANL improvement due to the combined application of NRA and ΔM5% (r = 0.7, p < 0.002), indicating that participants with high baseline ANL values had the largest improvement of the ANL.
Most comfortable levels
shows the effect of ΔM5% and NRA on the most comfortable levels (MCL) that we measured as part of the ANL procedure. A two-factor ANOVA (NRA, ΔM) showed that the MCL values decreased significantly for the conditions with ΔM5% [F(1,15) = 22.9, MSE = 4.7, p < 0.001, η2p = 0.60], with a mean decrease in 2.6 dB. Neither the NRA factor [F(1,15) = 1.2, MSE = 7.4, p = 0.29, η2p = 0.075] nor the interaction [F(1,15) = 0.054, MSE = 10.5, p = 0.82, η2p = 0.004] had statistically significant impact on MCL values.
Speech-in-noise thresholds
A normality check revealed that the SRTn data were normally distributed for each condition. presents the group mean SRTn values for the four conditions, showing that the SRTn values were not decreased due to ΔM5% or NRA-on alone, but the combination of both factors gave the best SRTn, although the differences between conditions were small. A repeated measures ANOVA with the factors NRA and ΔM, showed that neither the NRA factor [F(1,15) = 0.23, MSE = 2.6, p = 0.63, η2p = 0.015] nor the ΔM factor [F(1,15) = 1.0, MSE = 1.9, p = 0.33, η2p = 0.063] had a statistically significant impact on SRTn values, but the interaction of both factors was statistically significant [F(1,15) = 0.93, MSE = 1.3, p = 0.01, η2p = 0.35]. The difference between the combined condition (NRA-on, ΔM5%) and the reference condition (NRA-off, ΔM0%) was post hoc tested with a paired t-test. No significant difference was found (t(15) = 1.07, p = 0.3, r = 0.27).
Figure 3. Mean speech reception thresholds in noise (SRTn) values for the four combinations defined by combined settings of noise reduction algorithm (NRA) off/on and with/without additional 5% increase of M-levels (ΔM). Error bars represent 95% confidence intervals.
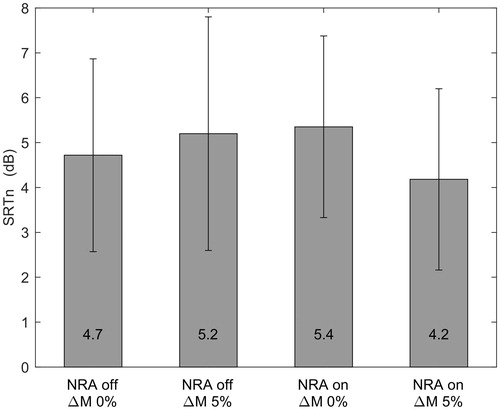
A subsequent analysis with the additional between-subject factor “test sequence” did not change the significance of the findings and none of the interactions of the factors with test sequence reached significance, indicating that the obtained results were not affected by order or fatigue effects.
Participants substantially differed in their SRTn value. The reference SRTn values (from condition NRA-off, ΔM 0%) ranged from −0.9 through to 12.7 dB. The SRTn improvement due to the combined application of NRA and ΔM5% was not significantly correlated with the reference SRTn values (r = 0.36, p < 0.17).
Discussion
Influence of M-level increase on the effect of ClearVoice
This study has demonstrated that NRA ClearVoice is more effective for noise tolerance and speech understanding in noise when combined with a 5% raise of M-levels.
First, raising the M-levels with 5% resulted in an extra effect of the NRA on noise tolerance as measured by the acceptable noise level (ANL). The NRA significantly improved noise tolerance on its own, in accordance with the findings of our previous study (Dingemanse and Goedegebure Citation2015). But if combined with an increase of M-levels with 5% the effect is even larger. The results showed a 2.2 dB more increase in noise tolerance for the combination of the NRA and a 5% raise of M-levels than the sum of the effect of both factors apart. Nevertheless, the interaction between the NRA and ΔM-level was not statistically significant. This is in contrast with our expectations. Possibly the lack of statistical significance is due to a relatively limited test precision in the ANL test (Olsen and Brännström Citation2014; Koch et al. Citation2016).
Secondly, for speech understanding in noise, a significant interaction was found between the factors NRA and ΔM5%. This indicates that it is valuable to combine the NRA ClearVoice with and M-level increase, although the observed effect was small. The improvement in SRTn between the combined condition (NRA-on, ΔM5%) and the reference condition (NRA-off, ΔM0%) was only 0.5 dB and not statistically significant, most probably due to a lack of statistical power for this comparison, that uses only two of the four conditions. In the interaction term the data of all the conditions is included, giving more statistical power, than in the case of comparison of two conditions. Given the small difference of 0.5 dB, the clinical relevance for speech understanding in noise is limited.
The results indicate that participants perceived an increase in SNR if the NRA was on, especially if combined with an increase of M-levels, but this perceived improvement was not enough to increase the intelligibility substantially. One explanation is that the listener perceived an increase in SNR mainly due to the maximum noise reduction during gaps between utterances of the words in a sentence and between sentences, while noise reduction is less during words, yielding less benefit regarding actual intelligibility. Another possibility is that the perceived SNR-increase was counteracted by a small decreasing effect of the NRA on speech intelligibility in noise. The NRA removed sound energy, that may have given a small decreasing effect on speech intelligibility in noise, or alternatively, the NRA may have introduced some distortion of the speech signal.
A possible explanation for the combined effect of the NRA and a 5% increase in M-level is that raised M-levels lead to a steeper slope of the input/output mapping function, giving a further enhancement of the speech-dominated peaks, a restoration of the perceived volume and an increase of positive SNRs in the electrical domain.
The effect of the combined application of ClearVoice and a 5% increase in M-level was significantly correlated to ANL baseline scores (from condition NRA-off, ΔM 0%) indicating that participants with high baseline ANL values had the largest improvement of the ANL, but this was not the case for SRTn baselines. An explanation for this difference is that both measures are obtained at different SNR levels. The mean SNR in the ANL-test was around 11 dB at 61.5 dB (SPL), but the mean SNR in the SRTn test was 5.0 dB at 70 dB (SPL). This suggests that the NRA ClearVoice in combination with a 5% M-level increase may be more effective at higher SNR-levels or lower speech levels.
Influence of M-level increase alone
An increase of M-levels without the NRA ClearVoice significantly lowered the MCL of the presented speech but did not significantly change noise tolerance or speech understanding in noise. The structural increase of M-levels had the goal to compensate for reduced signal volume due to attenuation caused by the NRA. This holds for situations with background noise, but not for quiet situations. Although the difference in MCL was only 2,6 dB due to the 5% increase of M-levels, it cannot be ruled out that this difference may cause some loudness discomfort for speech or other transient sounds in quiet, especially at higher input levels. As a consequence, CI users may choose to use a lower volume setting in general, which may diminish the positive effect of the 5% M-level increase in noise. A limitation of this study is that subjective rating of loudness was not included to answer this question of loudness discomfort.
Clinical consequences
The combined result of speech in noise and ANL suggest that NRA ClearVoice becomes more effective by increasing the M-levels. Although it does not result in a clinically relevant effect on speech intelligibility, it may contribute to a general optimisation of the effects of ClearVoice for a broad range of CI-users and listening conditions. Therefore, our findings suggest to always apply a 5% M-level increase when activating ClearVoice. This should be part of the clinical guidelines of Advanced Bionics. If CI users tend to lower the volume for conditions without background noise, it might be helpful to provide them with a separate programme for noisy conditions. It would be even better to include the increase in M-levels in a next version of the NRA ClearVoice. In general, our findings demonstrate that CI-fitting performed in the clinic may not always provide the optimal results for everyday-life conditions with background noise. Manufacturers and clinicians should be aware of this, and efforts should be made to optimise clinical fitting guidelines when introducing new noise reduction algorithms.
Conclusions
We conclude that optimal profit from the NRA ClearVoice is obtained if combined with a structural 5% increase of M-levels. The increase of M-levels alone gave no significant change in noise tolerance or speech understanding in noise.
Abbreviations | ||
ANL | = | acceptable noise level |
ANOVA | = | analysis of variance |
BNL | = | background noise level |
CI | = | Cochlear implant |
MCL | = | most comfortable level |
M-level | = | maximum comfort level or upper stimulation level linked to MCL |
NRA | = | noise reduction algorithm |
SNR | = | signal-to-noise ratio or speech-to-noise ratio |
SRTn | = | speech reception threshold in noise at 50% intelligibility |
T-level | = | stimulation level at hearing threshold |
Declaration of interest: This work was supported by Advanced Bionics.
Acknowledgements
The authors gratefully acknowledge the participants and they thank Romanna Delauw for her help with data collection.
References
- Advanced Bionics. 2012. ClearVoice. Technical Facts: Advanced Bionics, Valencia CA.
- Brendel, M., T. Rottmann, C. Frohne-Buechner, A. Buechner, and T. Lenarz. 2012. “Benefit of the Noise Reduction Algorithm ClearVoice for experienced Cochlear Implant Users." Paper presented at the 12th International Conference on Cochlear Implants and Other Implantable Auditory Technologies. Baltimore, MD.
- Buechner, A., M. Brendel, H. Saalfeld, L. Litvak, C. Frohne-Buechner, and T. Lenarz. 2010. “Results of a Pilot Study with a Signal Enhancement Algorithm for HiRes 120 Cochlear Implant Users.” Otology Neurotology 31: 1386–1390.
- Dawson, P. W., S. J. Mauger, and A. A. Hersbach. 2011. “Clinical Evaluation of Signal-to-Noise Ratio-Based Noise Reduction in Nucleus(R) Cochlear Implant Recipients.” Ear Hear 32: 382–390.
- Dingemanse, J. G., and A. Goedegebure. 2015. “Application of Noise Reduction Algorithm ClearVoice in Cochlear Implant Processing: Effects on Noise Tolerance and Speech Intelligibility in Noise in Relation to Spectral Resolution.” Ear and Hearing 36: 357–367.
- Faul, F., E. Erdfelder, A. Buchner, and A. -G. Lang. 2009. “Statistical Power Analyses Using G* Power 3.1: Tests for Correlation and Regression Analyses.” Behavior Research Methods 41: 1149–1160.
- Gaylor, J. M., G. Raman, M. Chung, J. Lee, M. Rao, J. Lau, and D. S. Poe. 2013. “Cochlear Implantation in Adults: A Systematic Review and Meta-Analysis.” JAMA Otolaryngology- Head and Neck Surgery 139: 265–272.
- Hehrmann, P., S. Fredelake, V. Hamacher, K. H. Dyballa, and A. Büchner. 2012. “Improved speech intelligibility with cochlear implants using state-of-the-art noise reduction algorithms." Paper presented at ITG Symposium Speech Communication (VDE), pp. 1–3.
- Holden, L. K., C. Brenner, R. M. Reeder, and J. B. Firszt. 2013. “Postlingual Adult Performance in Noise with HiRes 120 and ClearVoice Low, Medium, and High.” Cochlear Implants International 14: 276–286.
- Kam, A. C., I. H. Ng, M. M. Cheng, T. K. Wong, and M. C. Tong. 2012. “Evaluation of the ClearVoice Strategy in Adults Using HiResolution Fidelity 120 Sound Processing.” Clinical and Experimental Otorhinolaryngology 5: S89–S92.
- Koch, D. B., A. Quick, M. J. Osberger, A. Saoji, and L. Litvak. 2014. “Enhanced Hearing in Noise for Cochlear Implant Recipients: Clinical Trial Results for a Commercially Available Speech-Enhancement Strategy.” Otology Neurotology 35: 803–809.
- Koch, X., G. Dingemanse, A. Goedegebure, and E. Janse. 2016. “Type of Speech Material Affects Acceptable Noise Level Test Outcome.” Frontiers in Psychology 7: 186.
- Mauger, S. J., K. Arora, and P. W. Dawson. 2012. “Cochlear Implant Optimized Noise Reduction.” Journal of Neural Engineering 9: 065007.
- Mueller, H. G., J. Weber, and B. W. Hornsby. 2006. “The Effects of Digital Noise Reduction on the Acceptance of Background Noise.” Trends in Amplification 10: 83–93.
- Nabelek, A. K., M. C. Freyaldenhoven, J. W. Tampas, S. B. Burchfiel, and R. A. Muenchen. 2006. “Acceptable Noise Level as a Predictor of Hearing Aid Use.” Journal of the American Academy of Audiology 17: 626–639.
- Noël-Petroff, N., N. Mathias, C. Ulmann, and V. T. Den Abbeele 2013. “Pediatric Evaluation of the ClearVoice™ Speech Enhancement Algorithm in Everyday Life.” Audiology Research 3: e9.
- Olsen, S. Ø., and K. J. Brännström. 2014. “Does the Acceptable Noise Level (ANL) Predict Hearing-Aid Use?” International Journal of Audiology 53: 2–20.
- Pearsons, K. S., R. L. Bennett, and S. Fidell. 1977. Speech Levels in Various Noise Environments. Washington, DC: U.S. Environmental Protection Agency.
- Peeters, H., F. Kuk, C. C. Lau, and D. Keenan. 2009. “Subjective and Objective Evaluation of Noise Management Algorithms.” Journal of the American Academy of Audiology 20: 89–98.
- Pisa, J., M. Burk, and E. Galster. 2010. “Evidence-Based Design of a Noise-Management Algorithm.” The Hearing Journal 63: 42–44.
- Versfeld, N. J., L. Daalder, J. M. Festen, and T. Houtgast. 2000. “Method for the Selection of Sentence Materials for Efficient Measurement of the Speech Reception Threshold.” Journal of Acoustical Society of America 107: 1671–1684.