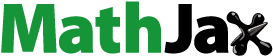
ABSTRACT
We examine the extent to which spatial interactions affect the labour market matching process at the regional level using three frameworks: random, stock-flow, and job-queuing. We study the underexplored, regionally diversified former transition country of Poland at the LAU-1 level over the 2003–2014 time period using registered monthly data. We employ a Durbin panel model with spatial error. Results prove that spatial interactions affect matching and that the stock-flow model is in some respects superior to others in explaining the job creation process. Unemployment affects matching more than vacancies. The newly unemployed may seek jobs in adjacent regions and can cause congestion there, but vacancies tend to attract workers from adjacent markets and exert positive externalities. Policy actions should be aimed at exploiting spatial interdependencies to improve matching at the regional level by increasing the number of available job offers, improving information, and increasing labour force mobility.
1. Introduction
Spatial disparities in unemployment figures across regions are persistent in many economies (e.g., Arntz & Wilke, Citation2009; Bradley & Taylor, Citation1997; Clark & Maas, Citation2015). This pattern seems to contradict the theoretical implications of migration behaviour, but is more in line with observed patterns of mobility mismatch. According to migration theory, workers will move to the regions that offer highest real wages, but the relocation costs depend on factors such as distance, housing market conditions, (un)employment rates, and family relationships. These factors may prevent workers from migrating on a large scale. The presence of a more attractive labour force can attract more investment, which may in turn reinforce and prolong development disparities between regions (e.g., Kunz, Citation2012; Wajdi, Adioetomo, & Mulder, Citation2017). Commuting behaviour (interregional spatial mobility) seems to provide an intermediate solution, especially if developed regions with low unemployment rates are located next to regions with high unemployment rates (Clemente, Larramona, & Olmos, Citation2016; Kosfeld, Citation2007; Rijnks, Koster, & McCann, Citation2016). Commuting is less costly than moving and increases labour force mobility, and thus enhances the job creation process from a spatial perspective. But to what extent does commuting help reduce the disparities between local labour markets if the workers in these markets are not mobile enough to migrate?
In this study, we analyse to what extent agents in regional labour markets, unemployed workers, and vacancies affect the job matching process in adjacent regions. We examine the regional job creation process by comparing various matching frameworks: namely, random, stock-flow, and job-queuing models. These frameworks are based on different matching schemes that will give us structural information about commuters’ matching behaviour. In a random (stock-stock) model (see, e.g., Blanchard & Diamond, Citation1994), a match occurs once a job seeker is assigned to a particular job. Vacancies and unemployment coexist due to coordination failure among agents, even if demand equals supply. The stock-flow model (see, e.g., Coles & Smith, Citation1998) assumes that agents have perfect information. This model posits that agents first consider numerous advertisements before applying for selected job offers, and that after they have rejected an offer, they are less likely to reapply than to search for new vacancies. It further assumes that agents who remain in the job market lack a proper partner, as all trade options have been exploited. In the equilibrium, the stock trades with the inflow (i.e., the unemployment stock trades with the vacancy inflow and the vacancy stock trades with the unemployment inflow). The job-queuing model (see, e.g., Shapiro & Stiglitz, Citation1984, matching takes place randomly) is formulated to reflect large discrepancies between demand and supply. According to this model, the short side of the market clears in each period, but an insufficient number of vacancies mean that workers must wait for new job postings. Thus, in the equilibrium, the unemployment stock matches the vacancy inflow. In our research, we try to disentangle the impact of inflow variables (unemployment and vacancy inflow) from the impact of stock variables (unemployment and vacancy stock) in generating job matches in focal and adjacent markets at the regional level. We argue that spatial interactions matter in disaggregated labour markets, and should therefore be included in the quantitative analysis. Regional labour markets are considered neither independent nor homogenous, and worker flows between regions are seen as indicating spatial dependence, which generates spatial externalities. The spatial analysis should help us gain a better understanding of the spatial interdependencies that affect the labour market matching process at the regional level. Our findings regarding the characteristics of the regional job matching process can be useful when formulating policy recommendations for medium-sized, regionally diversified economies in particular.
In this study, we focus on the understudied case of the labour market in Poland. Compared to the UE-28 average, this former transition country currently has a lower employment rate (in 2016, 69.3% in Poland and 71.1% in the UE-28), but it also has a lower unemploymentFootnote1 rate (in 2016, 6.2% in Poland and 8.6% in the UE-28). This pattern is attributable to the relatively low labour market participation rates that remained after the transition period. The shift from a centrally planned to a market-oriented economy occurred at the expense of adjustments in the labour market. Large shares of workers who could not adapt to the new conditions dropped out of the labour force, which resulted in relatively low labour force participation rates (Lehmann, Citation2012). Moreover, the labour market in Poland suffers from mismatches in skills, qualifications, and the regional distribution of the labour force (European Centre for the Development of Vocational Training, Citation2014; Organisation for Economic Co-operation and Development, Citation2014). From a regional perspective, Poland is characterised by large distortions in unemployment and vacancy rates.Footnote2 While labour market policy is implemented at the first level of Local Administrative Units (LAU-1), many Poles commute to work in another city, which is often located in another unit (Central Statistical Office, Citation2014). Because of these features, we see Poland as an exceptionally interesting case. In our research, we focus on public employment offices’ intermediation in Poland, and use the data for LAU-1 (379 regions) in the 2003–2014 period.
The regional job matching process combines the problem of spatial aggregation and spatial interdependencies. Many previous analyses of this process simply aggregated the number of agents across space to explain matching in a labour market of a given size. This perspective neglects the distortions in the distribution of unemployed workers and vacancies across regions, and it fails to account for the impact of the workers and the vacancy flows on the job creation process in focal and adjacent labour markets. In the outcome, the character of the returns to scale may be affected if spatial interactions in regional job matching process are neglected (Petrongolo & Pissarides, Citation2001). Burda and Profit (Citation1996) were the first to test spatial explanations for geographic instability in the matching function. They found that “foreign” unemployment affected local matching processes. The sign and the strength of this effect were distance-dependent; shorter distances produced positive externalities, while longer distances produced negative externalities. Burgess and Profit (Citation2001) extended the analysis of Burda and Profit (Citation1996), and explored the impact of unemployment and vacancy inflows on the matching process. Burgess and Profit (Citation2001) proved that high unemployment levels in neighbouring areas increased the number of filled vacancies in a given (local) area, but decreased the local outflow from unemployment; high vacancy levels in neighbouring areas raised the local outflow from unemployment and the local outflow of filled vacancies. Similar findings were obtained by Ilmakunnas and Pesola (Citation2003) and Kosfeld (Citation2007). Ilmakunnas and Pesola (Citation2003) showed that unemployment figures in the surrounding areas exerted a negative effect on local labour markets, and that vacancies exerted a positive effect. Kosfeld (Citation2007) found that the strength of these effects was not stable across space. Hynninen (Citation2005) proved that the congestion effect arose among job seekers in local labour markets, and was strengthened by spatial spillovers. Job seekers from neighbouring areas caused additional heterogeneity in the matching process in densely populated areas, and matching efficiency decreased in these places. So far, no study has analysed which matching framework best describes the behaviour of commuters.
In our research, we employ a spatial Durbin panel fixed effects model with spatial error. According to the literature, this is the only model that produces unbiased estimates of parameters and correct standard errors (Cook, Hays, & Franzese, Citation2015; Elhorst, Citation2010; Franzese & Hays, Citation2014). This model allows for regional heterogeneity in the data; avoids bias in the non-spatial effects; and produces estimates of local spatial (spillover) effects and spatial effects in unobservables for particular matching functions. We have come across a few papers that used this method, but none of these studies referred to the Polish labour market. Hujer, Rodrigues, and Wolf (Citation2009) estimated the augmented matching function that accounted for spatial interactions. They used a dynamic panel estimator and examined various weighting matrices. Stops (Citation2011) used a restricted version of the spatial Durbin panel that included only the “spatial” lags for covariates with fixed effects and random effects to analyse matching processes in occupational labour markets in Germany.Footnote3 Agovino (Citation2013) used static and dynamic versions of the model to investigate the spatial matching function for disabled workers in Italy. He investigated the importance of new matches, vacancy stocks, and unemployment stocks. Haller and Heuermann (Citation2016) examined the job matching process in local labour markets (NUTS-3) in Germany in the 2000–2010 period. They argued that spillovers were best approximated by geographical distance, rather than by the present or the past infrastructure or by commuter numbers.
We contribute to the literature by examining spatial interdependencies in the regional job matching process using three matching frameworks: random, stock-flow, and job-queuing. We use detailed monthly data for public employment intermediation in the relatively unexplored, yet interesting case of Poland for the 2003–2014 period. We use a spatial econometric method that produces robust results. We find spatial interactions in all of the labour market matching frameworks. Workers commute to surrounding local markets, but many of these flows take place across a single administrative border. We find that vacancies in contiguous local labour markets exert positive externalities on focal markets, and increase the matching rate there. However, we also find that unemployed individuals generate negative externalities, as most workers who seek work and cause congestion in adjacent areas are newly unemployed, whereas individuals from the unemployment stock are less likely to engage in a matching process in adjacent areas. These findings confirm our assumption that workers compete for scarce job opportunities in focal and adjacent areas. The results of the stock-flow model indicate that the unemployment stock affects matching more than the unemployment inflow, but that the vacancy inflow affects matching more than the vacancy stock. Based on these findings, we argue that policy actions should be aimed at exploiting spatial interdependencies to improve labour market matching at the regional level by, for example, increasing the number of available job offers (especially the magnitude of the vacancy inflow), improving information about available matching partners in the labour market, and increasing labour force mobility to take advantage of matching possibilities in the adjacent areas.
2. Spatial interactions in the labour market in Poland
We examined the commuting behaviour of workers at the LAU-1 level to check to what extent spatial interactions took place in the Polish labour market. We used data from income tax records and from social and agricultural insurance records that refer to the year 2011 (Central Statistical Office, Citation2014). We identified individuals who commuted from their place of residence to a workplace.Footnote4 In , we present the distribution of the regions that experienced the highest flows of incoming/outgoing commuters from a relative perspective. In 2011, the net commuting flow was negative in 87% of the LAU-1 units, which means that more workers went to work in other units than came to the given region. Moreover, in the 2006–2011 period, almost every third worker commuted to another region to work.
Figure 1. Distribution of the regions that attract and produce the most commuters.
Note: Data for 2011.Source: Own elaborations based on CSO data.
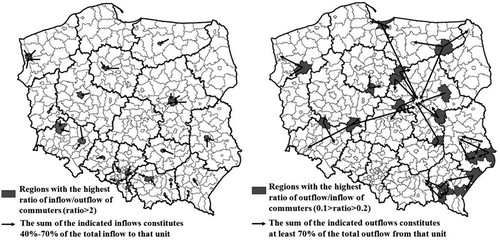
Based on commuting behaviour data, we built spatial weight matrices to determine the intensity of spatial interactions. We found that up to 50% of the commuting flows were across just one administrative border (e.g., from one region to another) and that the first-order contiguity inflow of commuters to the region produced the strongest impact on the employment in that unit ().Footnote5
Table 1. Correlation between the inflow of commuters to the region (up to 11 orders of contiguity) and the employment in that unit.
3. The data
Poland is divided into three regional classification levels (NUTS) and two levels of LAU-1 and LAU-2. There are (status as of 1 January 2016) 6 regions (made up of provinces, NUTS-1), 16 provinces (NUTS-2), 72 sub-regions (made up of districts, NUTS-3), 380 districtsFootnote6 (LAU-1), and 2478 communes (LAU-2). We focus on LAU-1 level, as these are the smallest geographical units for which data are available. The average area of a LAU-1 region is 825 km2 (0.3% of the total area of Poland). The average population of each region is 101,013 people (also 0.3% of the total population of Poland). We based our analysis on registered unemployment data from public employment offices (i.e., the data collected at the LAU-1). We analysed the 2003–2014 period using monthly data on the outflow from unemployment to employment, the vacancy and unemployment stocks, and the vacancy and unemployment inflows. displays the summary statistics of the data.
Table 2. Summary statistics (mean values, 2003–2014) of unemployment, vacancy stocks and inflows, and outflow from unemployment to employment.
We computed two labour market indicesFootnote7: the ratio of the vacancy inflow to the unemployment stock, and the ratio of the vacancy stock to the unemployment stock. These indices are presented in , and the exit rate from unemployment is presented in . The values of the indices indicate the relative difficulty of finding work for job seekers, and the relative ease of finding workers for companies. In the most favourable regions, an average of just nine workers competed for each new vacancy; whereas in the least favourable regions, as many as 70 individuals competed for each new vacancy. The spatial units with large labour market indices based on the vacancy inflow usually had high labour market indices based on the vacancy stock. It was often the case that a tight labour market was located next to a market that was less tight. Still, the south-western part of the country had the highest number of vacancies per unemployed person (Opolskie, Śląskie, Wielkopolskie, and Lubuskie). The Moran’s I indices showed that adjacent districts tended to cluster according to the vacancy stock and inflow, but that the polarisation could have occurred in terms of the unemployment stock or inflow. Certain values fluctuated over time, and the changes had no clear pattern. The mean exit rates differed substantially in the western and the eastern regions of the country. The largest exit rates were observed among the districts that had the tightest labour markets, especially in terms of the vacancy inflow. This pattern was visible primarily in the surroundings of large urban agglomerations in Łódzkie, Wielkopolskie, and Lubuskie.
Figure 2. Vacancy inflow to unemployment stock ratio (a), and vacancy stock to unemployment stock ratio (b), LAU-1, 2003–2014 mean value.
Note: Labour market tightness indices: vacancy inflow to unemployment stock (on the left) and vacancy stock to unemployment stock (on the right). The labour market tightness index reflects how many job offers there are per worker. The darker the district, the tighter the labour market.Source: Own elaborations based on public employment offices data.
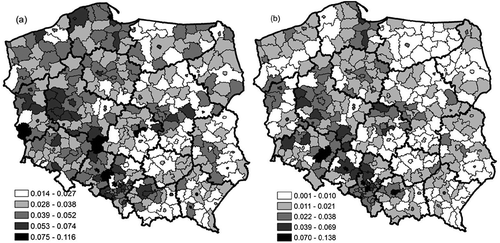
4. Matching function as a spatial Durbin panel model
Spatial Durbin panel models account for spatial auto-regression, autocorrelation, and cross-regression effects (i.e., the impact of spatially non-lagged and lagged exogenous variables). These models explain the differences in the levels of variables between objects – here, between regions – in a given period, and the differences in the levels of variables in selected objects during the period (Anselin, Le Gallo, & Jayet, Citation2008; Elhorst, Citation2003). Spatial interactions in panel Durbin models can be addressed in a few ways. The possible approaches include both spatial endogenous and exogenous interaction effects (SDM, spatial Durbin model); exogenous interaction effects among the explanatory variables (SLX, spatial lag of X model); autocorrelation of the random element and spatial exogenous interaction effects (SDEM, spatial Durbin error model), and the three-source model of the spatial endogenous, exogenous, and random elements (GNS, general nesting spatial model) (Elhorst & Vega, Citation2013; Vega & Elhorst, Citation2015). Spatial heterogeneity (spatial structure, diversification) can be represented by fixed or random effects.
Elhorst (Citation2010) provided an overview of the spatial panel econometric models, and argued that the SDM is the only model that produces unbiased estimates of parameters and correct standard errors. We chose a spatial Durbin panel fixed effects model with spatial error, as it allows for regional heterogeneity in the data (fixed effects), avoids bias in the non-spatial effects, and produces estimates of local spatial (spillover) effects and spatial effects in unobservables for particular models of a matching function.
We applied a spatial Durbin panel fixed effects model with spatial error to three labour market matching technologies: random, stock-flow, and job-queuing. Particular models are usually formalised by assuming the Cobb–Douglas matching function. The stock-stock model is , the job-queuing model is
, and the stock-flow model is
(Blanchard & Diamond, Citation1994; Coles & Smith, Citation1998; Gregg & Petrongolo, Citation2005); where
is the unemployment stock,
is the vacancy stock,
is the unemployment inflow, and
is the vacancy inflow. The investigated equations took the following form, for the random stock-stock model:
and the stock-flow model:
and the job-queuing model:
where is the outflow from unemployment to employment,
and
are the vacancy and unemployment stocks at the beginning of the month, and
and
are the vacancy and unemployment inflows during the month.
denotes a region,
denotes time, and
denotes a spatial weights matrix. All of the variables were expressed in natural logarithms.
We included in the estimations the first order of contiguity matrix . Our choice resulted from the initial analysis of the character of spatial interactions in the labour market. We constructed the first order of contiguity matrix using the queen criterion. The neighbours in the queen criterion are the units that have at least one point in common, including borders and corners. The dimension of the binary matrix
is equal to the number of units. The value of the matrix is one when units
and
are neighbours, and is otherwise zero. The diagonal elements of the matrix are set to zero, by assumption. Next, the binary contiguity matrix is transformed into a row standardised spatial weights matrix. Each element in the
-th row is divided by the row’s sum. The elements of the row standardised matrix take values between zero and one. The sum of the row values is always one.
displays the results for the random, stock-flow, and job-queuing models. The estimates proved that both the stock and the inflow of agents took part in a job creation process. Certain models indicated that the matching process was complicated, and various frameworks described the matching mechanism. Nevertheless, the log-likelihood results implied that the stock-flow model prevailed to some degree. The spatial Chow and log-likelihood ratio tests proved that the spatial regimes were significant, and that the spatial models could not be simplified to the non-spatial models (Anselin Citation1988). Moran’s I showed that by allowing the error terms to be spatially correlated , we improved the models’ goodness of fit and accounted for spatial effects in the modelling.
Table 3. Spatial model estimates, balanced panel: n = 379, T = 144, N = 54,576.
The non-weighed parameter estimates, for
, reflect the mean non-spatial direct effect, which captures the effect of a unit change in an explanatory variable in a focal district on the dependent variable in that district. The spatially weighed parameter estimates,
for
, reflect the mean indirect (spillover) effect, which is the effect of a unit change in an explanatory variable in the neighbouring districts on the dependent variable in a focal district (Elhorst, Citation2014; LeSage & Pace, Citation2009; Seldadyo, Elhorst, & De Haan, Citation2010).
All of the frameworks produced consistent (with respect to a sign) non-spatial direct and spatial indirect effects for particular variables. The unemployed individuals affected more matching than vacancies in a given district; but the unemployment stock mattered more than the unemployment inflow, whereas the vacancy inflow mattered more than the vacancy stock. In particular, a 1% increase in the unemployment stock increased the matching from 0.51% to 0.63% (depending on the model); but if the unemployment inflow increased by 1%, the outflow from unemployment to employment increased by 0.25%. The 1% increase in vacancies raised the matching rate by 0.07–0.08% (vacancy inflow) and 0.01% (vacancy stock). The weighed vacancies increased the outflow rate from unemployment to employment in a given district, and as in a direct effect, the vacancy inflow mattered more than the vacancy stock. The unemployed individuals from surrounding local labour markets decreased the matching rate in a given market, and the newly unemployed individuals competed more (a more negative parameter estimate) than the individuals from the unemployment stock. For example, a 1% increase in the unemployment inflow in the surrounding regions decreased the matching rate in a focal unit by 0.20%, whereas a 1% increase in the vacancy inflow in the adjacent markets increased the outflow from unemployment to employment in a focal market by 0.07–0.08%. The spatial error term parameters were significant and had positive signs. This means that a random shock that occurred in a given district was affected by unobservable effects in the neighbouring regions (compare to: Agovino, Citation2013; Haller & Heuermann, Citation2016; Rey & Montouri, Citation1999).
5. Discussion
The results of all three matching function models proved that over the period studied, spatial interactions existed and affected the labour market matching in Poland at the administrative units level (LAU-1). The non-weighed and weighed parameter estimates indicate that unemployed individuals affected the matching more than vacancies. The matching rate in a focal district depended more on the unemployment stock than on the unemployment inflow, but the vacancy inflow had greater elasticity than the stock counterpart. These results indicate that the job creation process predominantly originated from matches between “old” unemployed individuals and “new” vacancies. Job seekers registered with a public employment office in a given district, waited for new offers to appear in the market, and then formed a match. The weighed variables parameter estimates, in turn, indicated that unemployed individuals exerted negative externalities in adjacent local labour markets. As newly unemployed workers were looking for work in focal and contiguous markets, they were competing for scarce job opportunities with individuals who were registered as unemployed in those markets. Vacancies could not move freely between local labour markets after being registered with a public employment office. But because these vacancies attracted unemployed individuals from surrounding markets, the exit rate from unemployment to employment increased in the markets from which unemployed individuals originated. Interestingly, the negative impact of unemployment inflows from adjacent markets was much greater than the positive impact of vacancies from surrounding markets. The elasticity of the outflow rate on the unemployment inflow was 2.5 times higher than the elasticity of the outflow rate on the vacancy inflow in adjacent markets. Thus, while vacancies attracted mobile workers from surrounding areas, the number of vacancies was low compared to the number of job seekers. By competing heavily for job opportunities in adjacent markets, these job seekers caused congestion for the unemployed individuals who job-searched locally. Our results are in line with previous findings of positive and negative externalities exerted by vacancies and the unemployed (compare, e.g., Agovino, Citation2013; Ilmakunnas & Pesola, Citation2003; Stops, Citation2011).
For a robustness check of the results, we used regular panel models to compare the results to the findings of spatial models (see ). As the non-spatial panels produced greater elasticities on vacancies and smaller elasticities on unemployment, we can conclude that the lack of spatial interactions assumption underestimated the role of job seekers and overestimated the role of vacanciesFootnote8 in the labour market matching process at the regional level (compare Stops, Citation2014; Stops & Fedorets, Citation2015).
Table 4. Random matching, stock-flow matching, and job-queuing model estimates panel non-spatial models.
We tested the spatial autocorrelation in the residuals, and found that the global Moran’s I statistics were positive and statistically significant. This finding suggests that there is spatial dependency in the data. Thus, if we had neglected the observed and unobserved spatial interactions between the local labour markets, the results would have been biased. We also ran some comparison tests to determine which of the spatial models (SLX, SDM, GNS, or SDEM for fixed effects) is best suited to describing the data-generating process in the context of regional matching functions. In particular, we checked whether spatial autocorrelation in the outcome was caused by observable or unobservable effects. We found that the spatial-autoregressive lag model (which we did not apply) could be re-expressed as a higher-order variation of the SDEM (consistent with Franzese and Hays (Citation2014) or Cook et al. (Citation2015)). Moreover, we calculated the means of the log-likelihood, the log-likelihood ratio, and the Moran’s I of residuals, as recommended in LeSage (Citation2014); Cook et al. (Citation2015); and Vega and Elhorst (Citation2015). All of the tests confirmed that the SDEM suits the context of regional matching functions better than the SLX, GNS, and SDM (see ).
Table 5. Comparison tests for spatial models, balanced panel: n = 379, T = 144, N = 54,576.
The highest exit rates were observed in the regions that had the tightest labour markets, especially in terms of the vacancy inflow. Moreover, the largest exit rates were primarily visible in the surroundings of large urban agglomerations in Łódzkie, Wielkopolskie, Lubuskie, and northern parts of Poland. The fixed effects coefficientsFootnote9 produced a similar outcome (i.e., that larger markets facilitated the outflow from unemployment to employment by generating more vacancies) ().
Figure 4. Local specific influence on the outflow from unemployment to employment (fixed effects).
Note: The darker the region, the stronger the influence on the outflow from unemployment to employment.Source: Own elaborations based on public employment offices data.
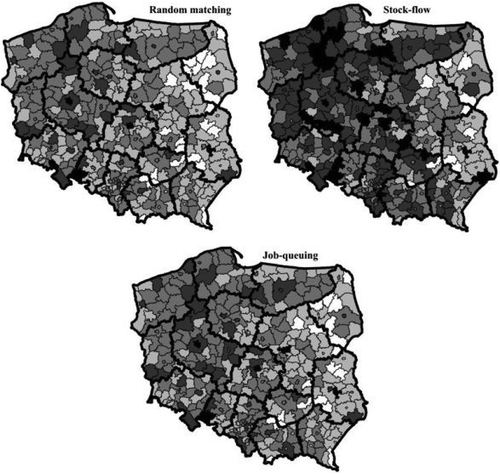
We found decreasing returns to scale, which suggests that local markets were insufficient to generate a proportional increase in the number of matches after the number of agents had increased. This finding may suggest that heterogeneity between local markets reflects a mismatch, and that workers look for work in adjacent areas to find suitable job offers.
We are aware of some limitations of our study. We built a simple spatial weight matrix of the first order using queen contiguity.Footnote10 This matrix defines neighbouring districts as districts that have at least one point in common (a border or a corner). Haller and Heuermann (Citation2016) constructed nine different spatial weight matrices based on distance, commuter behaviour, and infrastructure. They argued that using more complex spatial weights to model regional dependencies in a more realistic way adds only a small amount of information to regional matching models, and that the size of the direct and indirect effects hinges on the definition of the spatial weight matrix W. We chose the first-order contiguity matrix because the data indicated that up to 50% of the commuting flows were across just oneFootnote11 administrative border. Thus, we decided to include the first-order matrix in the explanatory spatial data analysis and the modelling. The estimation results showed that our W matrix produced the strongest spatial autocorrelation, and allowed for a more precise identification of spillover effects (compare also Agovino, Citation2013 on the justification for using commuting data to build a matrix).
Unlike previous studies that analysed similar questions, we estimated a Durbin model for panel data with fixed effects and spatial error term. The studies that used approaches relatively close to our own are Stops (Citation2011) and Haller and Heuermann (Citation2016). But unlike Stops, we investigated how the mobility of job seekers affected the labour market matching process at the LAU-1 (by contrast, he examined whether new hires were influenced by exogenous regressors in other occupation groups). Moreover, unlike Haller and Heuermann, we extended our analysis to account for inflow variables and to estimate stock-flow and job-queuing models. We compared non-spatial estimates with spatial estimates to identify the potential advantages of the spatial analysis in the labour market matching process. No previous study has looked at spatial interactions in the Polish labour market from a matching perspective.
We used registered unemployment data, and only referred to information on public employment intermediation. These data are useful for a number of reasons. They provide consecutive time series of the necessary stocks and flows of unemployment and vacancies, and the job offers in the data are directed at the individuals who have registered as unemployed. In Poland, an individual can register as unemployed, or as a non-unemployed job seeker. A worker is obliged to make periodic (monthly) visits to a public employment office to update her status, and to declare whether she remains ready and willing to work. If she fails to meet these requirements, she is removed from the registry. Unemployed workers are supposed to register with a public employment office to receive support in finding a job, but there are other incentives to register as well. For example, for non-employed workers, registration is a prerequisite for obtaining free health insurance. Hence, there are individuals who are registered as unemployed but who are not actively seeking employment. Many of these individuals are working in the shadow economy or are working abroad. Job seekers use various job search methods, and companies use various recruitment methods. Enterprises are supposed to publish every job vacancy at a public employment office, but because this regulation is frequently disregarded, public employment offices list only a fraction of all vacancies available in the economy. While there are alternative data from a representative survey on labour demand, these data are published on a quarterly basis only. An analysis of these representative survey data showed that at the end of 2014, the stock of job offers from public employment offices represented 93% of all vacancies (a stock). This ratio appears to have fluctuated over time, and has not shown any systematic trends. We decided to use the public employment offices’ measure of vacancies in order to ensure consistency with unemployment measures, and given the availability of stock and inflow variables. While better paid white-collar jobs are underrepresented in our measure of vacancies, job seekers with higher skills are also less likely than job seekers with lower skills to have registered unemployment spells. These highly skilled individuals tend to search for jobs using other methods, and often do not register with public employment offices. Moreover, individuals who are registered as unemployed tend to use vacancies from public employment offices, and registered vacancies can only be filled by workers who are registered as unemployed. As a result, we cannot equate the unemployment-to-employment flow with public employment intermediation based on the common legal conditions (at the LAU-1). Since there is no information on regional diversification in the use of public employment offices, we assume that the use of these offices does not differ across regions. Thus, we do not expect to find any systematic regional bias.
Looking to the future, we plan to extend our analysis in several ways. To address the drawbacks of our analysis, we plan to construct the spatial weight matrix differently to examine whether there are any non-linearities or asymmetries in the externalities in more distant spatial interactions. We also think that accounting for the determinants of the efficiency of the matching process can enrich the analysis. Thus, we will try to estimate the augmented matching function. We also plan to examine how the data spatial aggregation interacts with the data temporal aggregation, and how these aggregations jointly affect the matching function elasticities.
6. Concluding remarks
In this study, we analysed how spatial interactions affect labour market matching. We based our analysis on Polish regional data at the administrative units level (LAU-1). We used monthly registered unemployment data and estimated matching function models (stock-stock, stock-flow, and job-queuing) using a spatial Durbin panel fixed effects model with spatial error.
Our findings confirm our assumption that spatial interactions existed and affected the matching process in the local labour markets studied. We found heterogeneity among the LAU-1 labour markets, and some indications of both clustering and polarisation processes. The spatial panel Durbin model estimates indicated that unemployed individuals looked for work in focal and adjacent markets, which caused congestion in surrounding areas (negative externalities). The unemployed individuals competed for scarce job offers. Vacancies increased the job creation rate (in both the focal and the adjacent markets), and exerted positive externalities in the surrounding areas (by attracting unemployed individuals). Thus, the outflow from unemployment to employment could increase if more job offers were created.
In addition, our findings show the usefulness of exploiting spatial interdependencies to improve labour market matching from a regional perspective. The labour market policy measures should be directed at improving the information in the labour market about the available matching partners. If unemployed individuals have better information about available job offers, they can form more matches. The unemployed individuals generate negative externalities in contiguous markets, but improved information and labour force mobility should decrease the number of mismatches and increase the number of matches. The explanatory power of the stock-flow model proves that inflows engage in a matching process. Thus, policy actions aimed at increasing the number of available job seekers and the number of vacancies should also result in an increase in the number of matches.
Acknowledgements
The article was prepared within a project financed by the CERGE-EI in the 15th Global Development Network Regional Research Competition (RRC-15). We wish to thank the Ministry of Labour and Social Policy in Poland for sharing their database.
Disclosure statement
No potential conflict of interest was reported by the authors.
Additional information
Funding
Notes on contributors
Elżbieta Antczak
Elżbieta Antczak is Associate professor at the Department of Spatial Econometrics, Faculty of Economics and Sociology at University of Lodz. She implements spatial statistics, econometric (also spatial panel data models) and geostatistics tools for socio-economic and sustainable development analysis. She is the co-author and author of three books. In 2018-2020 she is an expert in the project: Analysis of the processes on the Polish labor market and in the area of social integration in the context of conducted economic policy implemented by the Polish Institute of Labor and Social Studies. In 2018-2019 she is the manager of scientific project: Municipal Waste in Communes. Analysis of Spatial Diversity of Socio-economic Determinants by the National Science Centre of Poland.
Ewa Gałecka-Burdziak
Ewa Gałecka-Burdziak received PhD in economics from Warsaw School of Economics in 2013. Previously, she graduated from University of Łódź. Principal Investigator of the projects financed by National Science Centre, Poland and CERGE-EI Foundation. She has received the START scholarship founded by Foundation for Polish Science. Her research interests focus on labour economics.
Robert Pater
Robert Pater received PhD in Economics, obtained in The Collegium of Economic Analysis at Warsaw School of Economics. Currently holds the position of the Head of Department of Macroeconomics at University of Information Technology and Management in Rzeszów, Poland. Specializes in macroeconomics and econometrics with particular application to business cycle, vacancy market and policy evaluation. Author or co-author of 50 scientific articles and over 100 reports. Participated in several labour market research projects. Conducts regular research on the vacancy market in Poland. A member of the European Association of Labour Economists (EALE). Cooperates with the Educational Research Institute, and Bureau for Investments and Economic Cycles in Warsaw. Polish expert of Institut fur Wirtschaftforschung (IFO) in Munich in a worldwide survey on economic tendencies.
Notes
1 At the same time, the vacancy rate is also relatively low: 0.8% in Poland compared to 1.8% in the EU-28.
2 We use the Local Administrative Unit (LAU) classification of the European Union regions at a detailed level. LAU-1 level refers to districts, whereas LAU-2 is the lowest local administrative unit – a commune in Poland) At the LAU-1 level, the unemployment rate in 2014 ranged from 3.1% in certain urban areas (e.g., Poznań, Warsaw, and Wrocław, and rural areas of western Poland) to 34.4% in some rural districts (the Szydłowiecki district in Masovia and rural areas of north-eastern Poland). The vacancy rate ranged from 0% in a few rural areas (e.g., Białobrzeski, Przysuski, and Zwoleński in south-west Mazovia) to 2.7% in Świętochłowice city in Upper Silesia (Śląskie).
3 Stops (Citation2014) and Stops and Fedorets (Citation2015) also analysed the matching function (and augmented matching function). They accounted for spatial dependence, but employed other methods.
4 The data did not contain information on the means of transportation, the frequency of the commute, or the travel time.
5 Nonetheless, the data indicated that there were statistically significant spatial interactions up to the 11th row of contiguity, but that less than 5% of the worker flows occurred in the units from the seventh to the 11th degree of contiguity.
6 Throughout the paper, we use the term region(s) to refer to district(s). We included in our research a total of 379 regions (out of 380). As of 1 January 2013, the city of Wałbrzych was distinguished from the Wałbrzyski region as a separate district. We added the values for both regions (as it was provided in the data before 2013) for 2013–2014 period.
7 The labour market tightness index is usually based on stock variables. We argue that the vacancy inflow may be a more accurate measure of the number of available job offers, especially if the vacancies are filled quickly, and thus do not appear in the end-of-the-period stocks.
8 Hujer et al. (Citation2009) also obtained in the spatial model a higher elasticity on unemployment than vacancies, and attributed this finding to the underreporting of vacancies to the local employment agencies (compare also to Haller & Heuermann, Citation2016).
9 By introducing fixed effects, we have greatly reduced the threat of omitted variable bias.
10 Contiguity-based relations are mostly used in the presence of irregular polygons with varying shapes and surfaces, since contiguity ignores distance, and instead focuses on the location of an area.
11 We also tested more distant contiguity matrices, which indicated that higher-order neighbours are useful when looking at more global spatial autocorrelation (available by e-mail).
References
- Agovino, M. (2013). Estimating a spatial matching function for disabled people: Evidence from Italy. Working Paper, Retrieved January 23, 2017, from http://www.aiel.it/Old/bacheca/LUISS/papers/agovino.pdf.
- Anselin, L. (1988). Spatial econometrics: Methods and models. Dordrecht: Kluwer Academic Publishers.
- Anselin, L., Le Gallo, J., & Jayet, H. (2008). Spatial panel econometrics. Chapter 19. In M. László & S. Patrick (Eds.), The econometrics of panel data: Fundamentals and recent developments in theory and practice. Berlin: Springer: 625–660.
- Arntz, M., & Wilke, R. A. (2009). Unemployment duration in Germany: Individual and regional determinants of local job finding migration and subsidized employment. Regional Studies Taylor & Francis (Routledge), 43(01), 43–61.
- Blanchard, O., & Diamond, P. (1994). Ranking, unemployment duration, and wages. The Review of Economic Studies, 61, 417–434.
- Bradley, S., & Taylor, J. (1997). Unemployment in Europe: A comparative analysis of regional disparities in Germany, Italy and the UK. Kyklos, 50(2), 221–245.
- Burda, M. C., & Profit, S. (1996). Matching across space: Evidence on mobility in the Czech Republic. Labour Economics, 3, 255–278.
- Burgess, S., & Profit, S. (2001). Externalities in the matching of workers and firms in Britain. Labour Economics, 8, 313–333.
- Central Statistical Office. (2014). Commuting to work in Poland – Results of the national census of population and housing 2011, Warsaw. Retrieved January 16, 2017, from http://stat.gov.pl/spisy-powszechne/nsp-2011/nsp-2011-wyniki/dojazdy-do-pracy-nsp-2011,7,1.html
- Clark, W., & Maas, R. (2015). Spatial mobility and opportunity in Australia: Residential selection and neighbourhood connections. Urban Studies, 53(6), 1317–1331.
- Clemente, J., Larramona, G., & Olmos, L. (2016). Interregional migration and thresholds: Evidence from Spain. Spatial Economic Analysis, 11(3), 276–293.
- Coles, D. M., & Smith, J. E. (1998). Marketplace and matching. International Economic Review, 39, 239–254.
- Cook, S. J., Hays, J. C., & Franzese, R. J. (2015). Model specification and spatial interdependence. Retrieved October 24, 2017, from http://www.sas.rochester.edu/psc/polmeth/papers/Cook_Hays_Franzese.pdf
- Elhorst, P. J. (2003). Specification and estimation of spatial panel data models. International Regional Science Review, 26, 244–268.
- Elhorst, P. J. (2010). Spatial panel data models. In M. M. Fischer & A. Getis (Eds.), Handbook of applied spatial analysis Berlin (pp. 77–407). Heidelberg and New York: Springer.
- Elhorst, P. J. (2014). Matlab software for spatial panels. International Regional Science Review, 37, 389–405.
- Elhorst, P. J., & Vega, S. H. (2013). On spatial econometric models, spillover effects, and W. ERSA conference papers ersa13p222. European Regional Science Association. Retrieved January 4, 2017, from http://www-sre.wu.ac.at/ersa/ersaconfs/ersa13/ERSA2013_paper_00222.pdf
- European Centre for the Development of Vocational Training (2014). Briefing note – Skill mismatch: More than meets the eye. Retrieved December 11, 2016, from http://www.cedefop.europa.eu/en/publications-and-resources/publications/9087 doi:10.2801/57257
- Franzese, R. J., & Hays, J. C. (2014). Testing for spatial-autoregressive lag versus (Unobserved) spatially correlated error-components. Retrieved October 24, 2017, from http://myweb.uiowa.edu/fboehmke/shambaugh2014/papers/Franzese_Hays_IOWA14.pdf
- Gregg, P., & Petrongolo, B. (2005). Stock-flow matching and the performance of the labor market. European Economic Review, 49, 1987–2011.
- Hujer, R., Rodrigues, P. J. M., & Wolf, K. (2009). Estimating the macroeconomic effects of active labour market policies using spatial econometric methods. International Journal of Manpower, 30, 648–671.
- Hynninen, S.-M. (2005). Matching across space: Evidence from Finland. Labour, 19, 749–765.
- Ilmakunnas, P., & Pesola, H. (2003). Regional labour market matching functions and efficiency analysis. Labour, 17, 413–437.
- Kosfeld, R. (2007). Regional spillovers and spatial heterogeneity in matching workers and employers in Germany. Jahrbücher Für Nationalökonomie Und Statistik, 227, 236–253.
- Kunz, M. (2012). Regional unemployment disparities in Germany: An empirical analysis of the determinants and adjustment paths on a small regional level. Bielefeld: Bertelsmann.
- Lehmann, H. (2012), The Polish growth miracle: Outcome of persistent reform efforts, IZA Policy Paper No. 40, Retrieved November 4, 2017. http://ftp.iza.org/pp40.pdf
- LeSage, J. P. J. (2014). What regional scientists need to know about spatial econometrics. Retrieved (October 22, 2017, from http://citeseerx.ist.psu.edu/viewdoc/download?doi=10.1.1.722.8111&rep=rep1&type=pdf
- LeSage, J. P. J., & Pace, K. R. (2009). Introduction to spatial econometrics. Boca Raton: Chapman & Hall.
- Organisation for Economic Co-operation and Development. (2014). OECD economic surveys: Poland 2014. Paris: OECD Publishing.
- Haller, P., & Heuermann, D. F. (2016). Job search and hiring in local labor markets: Spillovers in regional matching functions. Regional Science and Urban Economics, 60, 125–138.
- Petrongolo, B., & Pissarides, C. A. (2001). Looking into the black box: A survey of the matching function. Journal of Economic Literature, 39, 390–431.
- Rey, S. J., & Montouri, B. D. (1999). US regional income convergence: A spatial econometric perspective. Regional Studies, 33, 143–156.
- Rijnks, R. H., Koster, S., & McCann, P. (2016). Spatial heterogeneity in amenity and labor market migration. International Regional Science Review. doi:10.1177/0160017616672516
- Seldadyo, H., Elhorst, P. J., & De Haan, J. (2010). Geography and governance: Does space matter? Papers in Regional Science, 89, 625–640.
- Shapiro, C., & Stiglitz, J. E. (1984). Equilibrium unemployment as a worker discipline device. The American Economic Review, 74, 433–444.
- Stops, M. (2011). Job matching on non-separated occupational labour markets. Retrieved November 30, 2016, from http://www-sre.wu.ac.at/ersa/ersaconfs/ersa11/e110830aFinal00372.pdf
- Stops, M. (2014). Job matching across occupational labour markets. Oxford Economic Papers, 66, 940–958.
- Stops, M., & Fedorets, A. (2015). Job matching on connected occupational and regional labor markets. Retrieved November 11, 2016, https://www.diw.de/documents/vortragsdokumente/220/diw_01.c.512184.de/v_2015_fedorets_job_eea.pdf
- Vega, S. H., & Elhorst, P. (2015). The SLX model. Journal Of Regional Science, 55(3), 339–363.
- Wajdi, N., Adioetomo, S. M., & Mulder, C. H. (2017). Gravity models of interregional migration in Indonesia. Bulletin of Indonesian Economic Studies, 53, 309–332.