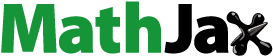
ABSTRACT
We applied the fractional integration approach to measure inflation deviation inertia in an emerging economy under an inflation targeting regime, as well as to control for potential determinants of such a deviation. We did not base our analysis on typical unit root tests, as we identified a long-memory behavior in inflation deviation. Such a non-conventional procedure can be regarded as a contribution to the inflation targeting literature. From ARFIMA and ARFIMAX estimates, we identified that inflation deviation was mainly determined by a strong inertia degree (AR(1)), commodity prices, nominal exchange rate and economic activity over the entire sample. Moreover, we found an increase of the inflation deviation inertia over the last years of our time sample, thereby indicating a deterioration of the inflation targeting regime in such a specific period.
1. Introduction
Inflation targeting regimes are commonly associated with stationarity in the inflation deviation dynamics with a zero mean process in ideal situations (Clarida, Galí, & Gertler, Citation1999; Svensson, Citation1997). The explicit announcement of an inflation target and a commitment of the Central Bank to such a goal can anchor inflationary expectations to the inflation target, thereby enhancing the ability of monetary policy in disinflation episodes (Gonçalves & Carvalho Citation2009). However, there exist some potential factors conditioning the performance of an inflation targeting regime to tame expectations and the observed inflation dynamics. Problems relating to the fiscal dimension and monetary policy credibility are examples (Woodford, Citation2001). Under critical constraints, thus an inflation deviation can only revert slowly to its mean over time, preventing the occurrence of a stationary inflation deviation dynamics.
An expressive group of advanced and emerging economies have performed inflation targeting since 1990, including Brazil, which adopted its particular specification in 1999. However, inflation deviation since then presented persistent positive levels in this country, especially in more recent years, in contrast to the predicted zero mean process or the efficiency of the regime. Several studies have reported controversial international findings with regard to the benefits from adopting inflation targeting regimes, varying from non-statistically significant effects (e.g., Ball & Sheridan, Citation2004) to positive and statistically significant benefits (Gonçalves & Salles Citation2008; Wu, Citation2004).
There is no a significant literature on the properties of inflation deviations in Brazil, but rather on inflation rates in absolute terms. Some examples of the latter are Cysne (Citation1985), Barbosa and McNelis (Citation1989), de Holanda Novaes (Citation1993), Durevall (Citation1998), Gottschalk (Citation2003), Reisen, Cribari-Neto, and Jensen (Citation2003), Cerisola and Gelos (Citation2009), Veloso et al. (Citation2013) and Ponzoni and Zilli (Citation2015). From an economic perspective, the focus of these empirical efforts is to evaluate if inflation rates in Brazil converge to a stable level or not. Given the Brazilian hyperinflation episode from the 60’s until the first half of the 90’s, this subject can be regarded as a natural trend.Footnote1 From a statistical perspective, on the other hand, this literature is mostly based on typical Dickey–Fuller tests to identify unit root in data. One of the problems of such tests is that it can easily overestimate (or underestimate) the integration order of a specific time series (Diebold & Rudebush, Citation1991; Hasler & Wolters Citation1994; Lee & Amsler, Citation1997). That is, the conventional unit root tests, such as Augmented Dickey–Fuller, Phillips–Perron and KPSS tests, among others, do not deal with the problem of long-memory in data. The statistical error of disregarding long-memory is that, for instance, when a time series is assumed as stationary by the conventional tests, but it actually presents long-memory, the resulting autoregressive inference commonly overestimates coefficients. Thus, one way to deal appropriately with long-memory in data is by the fractional integration approach (Baillie, Chung, & Tieslau, Citation1996; Geweke & Porter-Hudak, Citation1983; Reisen, Abraham, & Lopes, Citation2001; Reisen et al., Citation2003; Wei, Citation2006). Basically, this approach allows for transforming a long-memory time series into a short-memory one, so that we can avoid spurious estimates in autoregressive models.
Our study aims at identifying how inflation deviation behaves over time in Brazil, based on the fractional integration approach and focusing on the persistence degree of deviations from the inflation target. The empirical inference was made by dealing with long-memory in data and so can be regarded as a contribution to the inflation targeting literature from a statistical perspective. In studying inflation rate deviation, it departs from most of the related literature for Brazil, previously mentioned, which focuses mainly on the inflation rate per se. Under an inflation target regime, the main variable for evaluating the monetary policy’s consistency must be the inflation deviation and not the inflation rate per se (Barro & Gordon, Citation1983; Clarida et al., Citation1999; Svensson, Citation1997).
Specifically for the inflation targeting period in Brazil (i.e., since 1999), Reisen et al. (Citation2003) applied a long-memory approach in an univariate study, instead of using typical unit root tests, in order to test for inertia in Brazil’s inflation rates. By using fractional differentiation with ARFIMA models, they concluded that the Brazilian inflation did not present inertia in the conventional sense, meaning that there was not an unit root process, in contrast to some previous empirical results. However, as Reisen et al. (Citation2003) assessed only the intrinsic component of the inflationary inertia, i.e., only the role of the own inflation path to determine the inflationary inertia, such an analysis did not regard the role of other macroeconomic variables, such as exchange rate and output (extrinsic components) on the degree of inflationary inertia over time.
It is worth noting that there is a difference of meaning for inflation inertia in the related literature. Some works consider inflation inertia strictly as the existence of a unit root in inflation rates. An example of this specific stream is Reisen et al. (Citation2003). In contrast, the literature mostly regards inertia from a more general viewpoint, that is, as inertia degree. In this perspective, even when there is no unit root in inflation levels, it is possible to speak of its inertia degree, which can be assessed by the autoregressive component of the inflation rate over time.
Figueiredo and Marques (Citation2009) applied an Arfima-Figarch methodology in order to assess the long-memory in the Brazilian inflation rate, regarded as a fractionally integrated process in both the mean and variance values.Footnote2 The data comprised from January/1980 to January/2008 and the inflation rate was measured by the General Price Index of the Fundação Getúlio Vargas (IGP-DI/FGV). The findings demonstrated that the Brazilian inflation rate presented a fractionally integrated process since the Post-Real Plan, that is, after the stabilization policies adopted in Brazil in 1994. Furthermore, the results showed that Brazil’s inflation rate indicated a short-term inertia component, with short-memory, and a long-term inertia component with long-memory in both the first (mean) and second (variance) moments of the inflation rate. Finally, the authors found effects of the inflation rate volatility on the inflation rate mean value over time. In turn, Cerisola and Gelos (Citation2009) found results demonstrating that the expected inflation process was more anchored on inflation targets and that inflation rates in Brazil did not present expressive inertia since the adoption of such a targeting regime.
Finally, Machado and Portugal (Citation2014) investigated the inflation persistence in Brazil based on a Bayesian approach and by decomposing the inflation rate into unobserved components through a Kalman filter analysis for the period from the first quarter of 1995 to the first quarter of 2011. Three sources of inflation persistence were identified: intrinsic, related to the lagged inflation path; extrinsic, related to shifts in output gaps and in the natural rate of interest; and expectations-based, associated with the difference between the public’s perceived inflation target vs. the actual inflation target. The results confirmed the relevant role of the latter source, as well as of shifts in output gaps and natural rate of interests as driving forces of inflation persistence. The authors also found results showing a decrease of the intrinsic component – i.e., the lagged inflation rate path – over the last years of the sample, and pointed out the persistent nature of inflationary expectations as a main source of inflation persistence in Brazil.
All these studies covering the inflation targeting period in Brazil paid special attention to inertia of the inflation rate per se. However, there is still a gap in the related literature with regard to inertia of the inflation deviation, i.e., inertia of the difference between inflation rate and the inflation target. As a country adopts the inflation targeting regime, the analysis of the inflation deviation inertia should be considered as the main issue.
With regard to the international literature, studies for other countries are also based mainly on a conventional approach, i.e., they generally do not regard problems of long-memory in data and are usually concerned with inflation inertia instead of inflation deviation inertia, even for countries under inflation targeting regimes. For example, in a classical work on inflation dynamics for the US economy, Galí and Gertler (Citation1999) performed Generalized Method of Moments estimates based on quarterly data from 1960:1 to 1997:4. Although such a work has been strongly representative of original evidence on the so-called hybrid Phillips curve, thereby reducing the importance of the lagged inflation component in the estimates for inflation dynamics, the authors did not consider the possibility of problems related to long-memory in their sample.
In turn, Melnick and Strohsal (Citation2017) estimated a Phillips Curve for Israel from 1986 to 2015 and found results showing that credibility was built gradually and the adoption of an inflation target was a driver force in lowering inflation rates from 10% to 2% over time. However, this study did not make its analysis with the concept of inflation deviation dynamics or inertia, along with a lack of appropriate tests for identifying the (fractional) integration order of the time series. In this line, Baxa, Plašil, and Vašíček (Citation2015) investigated if the adoption of inflation targeting regimes contributed to stabilize inflation rates in a group of Central European countries, with quarterly data from 1996:1 to 2010:4. The results suggest that the adoption of inflation targets per se do not guarantee desirable outcomes such as a reduction in intrinsic inflation persistence or inflation volatility. Despite the relevance of such a finding, it remains without robustness in our view, especially because the authors did not perform tests for verifying the occurrence of fractional integration in data. Thus, the statistical results on inflation persistence can be misleading.
The remainder of this paper is structured in the following way. The second section makes a brief overview relating the inflation targeting literature and inflation deviation in Brazil. The third section presents the dataset and the methodological strategy for empirical purposes. The fourth section analyzes the estimates obtained in this work. Lastly, we make the concluding remarks.
2. Inflation targeting regime and inflation deviation in Brazil
2.1. A. a theoretical assessment of the inflation targeting regime
Since 1990, several developed and developing economies have performed an inflation targeting regime as a nominal anchor for the Central Bank’s monetary policy. By announcing an explicit inflation target over a long-term horizon and consolidating a credible commitment with such a goal, Central Banks are able to control inflationary expectations, thereby increasing the efficacy of interest rate adjustments (Clarida et al., Citation1999; Svensson, Citation1997).
In general, under inflation targeting regimes monetary policy is performed through a type of the so-called Taylor rule (Taylor, Citation1993). In other words, basic interest rate adjustments are made as a positive reaction to inflationary expectations deviations regarding the inflation target, as well as to output gaps in relation to potential output levels. This strategy of monetary policy is associated with a process of convergence of both expected and observed inflation to the target.
In turn, inflation deviations from the established target are regarded only as transitory events. The theoretical literature on inflation targeting commonly considers possible causes that motivates such temporary inflation deviations as supply shocks, exchange rate movements and the need for policy gradualism (Bernanke & Mishkin, Citation1997; Ball, Citation1999a; Citation1999b; Rudebusch, Citation2006). Thus, if any inflation deviation is due to transitory factors one can predicts a process of stationarity of the inflation deviation, so that this stochastic variable presents a zero mean and a constant variance.
However, some works point out potential factors conditioning the performance of inflation targeting regimes, such as fiscal dynamics and monetary policy credibility (Moreira, Citation2016; Woodford, Citation2001). It means that the simple adoption of an inflation target is not a guarantee of a context marked by inflation stability over time. Therefore, depending on a sample of concomitant factors, one can find an inflation deviation dynamics with a slow reversion to its mean value. In a statistical perspective, this stochastic process potentially presents a problem of long-memory and cannot be considered as a stationary dynamics.
Let us formalize an inflation targeting regime as a framework in which the main long-run goal of the Central Bank is to accomplish the inflation target, that is, to make the inflation rate equal to the inflation target. From the inflation targeting models found in Svensson (Citation1997) and Clarida et al. (Citation1999), among others, we can call the observed inflation rate and the inflation target
and express the inflation rate behavior by:
Call the inflation rate variance δ2Π. Therefore, we can regard (1) as the efficiency condition for the inflation targeting regime. Hence, in such a case the observed inflation rate converges to the inflation target and deviations do not show propensity to change. Besides, in this particular situation the inflationary expectations, are anchored on the inflation target, such that:
We call the inflation deviation :
When the efficiency condition (1) is satisfied, then Otherwise,
, or
, where the persistent deviation k is a positive or negative constant. Therefore, when the inflation targeting regime is inefficient we have:
The inflation rate converges to a level above or below the inflation target, with a persistent deviation equal to k. The consequence of this persistent deviation is a loss of credibility of the inflation target, which thus does not anchor the inflationary expectations. In this case, such expectations present the following behavior:
In this perspective, in order to satisfy the inflation targeting regime’s efficiency condition, and to eliminate the persistent deviation , it is important to identify and test for the potential determinants of this deviation. A multivariate analysis that explains the behavior of k over time is necessary to control its resulting social loss.
2.2. B. A brief contextualization of inflation deviation in Brazil
The Brazilian inflation targeting regime was introduced in 1999 and since then the Central Bank has performed its monetary policy mainly by adjusting the short-term interest rate, i.e., the Selic interest rate, as the instrument to control the observed inflation in relation to the inflation target. In 2003, the president Luiz Inácio Lula da Silva launched his first mandate (2003–2006) under a strong uncertainty regarding what would be the effective direction of the economic policy in the Brazilian economy. As a result of such uncertainty there was an expressive undervaluation of the domestic currency (R$) in relation to the US dollar, thereby rapidly raising inflationary expectations and the observed inflation over that first year.
The government responded to the confidence shock by means of an increase of the consumer inflation target, from 4% per year in 2003 to 5.5% per year in 2004, because the Central Bank argued that such an adjustment of the inflation target would allow the monetary policy to become less aggressive in responding to the augmented inflationary deviation. In turn, the nominal Selic target in February 2003 reached 26.5% per year and gradually decreased to 16% in April 2004, as the expected inflation for 12 months forward dropped from 10.9% per year in the former month to 5.6% in the latter.
In 2005, the consumer inflation target received a new adjustment, from 5.5% to 4.5% per year, which has been the inflation target since then. The Selic target, in turn, has presented a cycle that has mainly responded to inflationary expectations, denoting a forward perspective of the Brazilian monetary policy, although some works on Taylor rules for Brazil disagree regarding such a forward pattern, pointing to an expressive inertial component of the Selic effective rate as the main factor explaining monetary policy behavior in Brazil (Moreira, Citation2015).
Furthermore, the evident declining trend of the Selic target over the sample was not justified if one accounted for the persistent inflation deviation, especially from August 2011 to October 2012, when the Selic target decreased from 12.5% per year to 7.25%, despite a persistent and relatively constant inflation deviation between these two moments. Such a decreasing trend of the Selic would thus be motivated by political and judgmental factors beyond the macroeconomic fundamentals (Barbosa, Camelo, & Joao, Citation2016; Moreira, Citation2015). However, a new augmented inflationary deviation from 2013 was followed by a cycle of positive changes of the Selic target, which reached 14.25% in July 2015 and was kept at this level for 14 consecutive months. In sum, and particularly regarding the performance of the inflation target regime in Brazil, the monetary policy has had non-trivial challenges, as we can infer from the effective inflation deviation over the sample.
In , we observe the behavior of the effective inflation deviation (accumulated in the last 12 months) and the expected inflation deviation (accumulated 12 months forward), both in relation to the inflation target, over the period from May 2004 to December 2015. Although the series show positive and negative values over time, since 2010 the deviations started a positive persistence process. Indeed, the mean of the effective deviation in the whole period was 1.3 percentage points above the inflation target, and the mean of the expected inflation deviation was 0.67 percentage point above the inflation target. In contrast, when we consider only the period from January 2010 to December 2015, those mean values increased to 2.07 and 1.34 percentage points, respectively. Such persistent deviations are contrary to a case of efficiency for the inflation targeting regime (Clarida et al., Citation1999; Svensson, Citation1997). Thus, for monetary policy purposes, it is important to assess the nature of these persistent deviations as well as their determinants over time.
3. Dataset and methodological strategy
3.1. A. Dataset
We adopted monthly macroeconomic time series for Brazil covering the period from May/2004 to Dec/2015. It is important to explain why we selected such a time sample. First, some time series were available only since 2003, such as the economic activity index (Y) of the Brazilian Central Bank. Second, when we set the observed inflation deviation series (DESV_P) from 2003, a strong problem of atypical observations raised, due to the expressive acceleration of Brazil’s inflation rate over 2003, as a consequence of the uncertainties regarding the newly elected president, Luiz Inácio Lula da Silva. In turn, outliers in time series can affect the estimates of autoregressive models (Fajardo et al. Citation2009). Therefore, we chose to work with the time series from May/2004, when the apparent outlier problem of DESV_P is eliminated. We have the following time series for empirical purposes. First, DESV_P, the observed inflation deviation, i.e., the difference between the accumulated inflation rate over 12 months and the inflation target. The inflation rate is based on the Broad Consumer Prices Index (IPCA-IBGE). Second, DESV_EXP_P, the expected inflation deviation, i.e., the difference between the accumulated inflation rate for 12 months forward (based on the IPCA) and the inflation target. Third, E, the nominal exchange rate, i.e., the exchange rate between the Real (R$) and the US dollar (US$). Fourth, Y, the economic activity index of the Brazilian Central Bank, commonly used as a proxy of the GDP in monthly basis. Fifth, DIV, the net domestic public debt ratio to Brazil’s GDP. All the time series were collected from the Brazilian Central Bank. And finally COMM, the commodity prices general index of the International Monetary Fund.
3.2. B. methodological strategy
We divided the analysis of the inflation deviation series (DESV_P) into two steps. First, we applied an univariate analysis of such a variable, based on the method of Geweke and Porter-Hudak (GPH) (Geweke & Porter-Hudak, Citation1983), as well as semiparametric and parametric methods, especially ARFIMA models. Such an analysis allows for identifying the existence of long-memory in time series (Reisen, Citation1994; Reisen et al., Citation2001; Wei, Citation2006). Second, we applied an analysis with explanatory variables in order to indentify the main macroeconomic factors determining inflation deviation in Brazil, as well as to verify if the inertia degree of inflation deviations varied over time. Thus, we estimated three ARFIMAX models, which can be regarded as an extension of the ARFIMA analysis by means of adopting explanatory variables: (i) for the entire sample; (ii) from May/2004 to Dec/2009, and; (iii) from Jan/2010 to Dec/2015. These two latter sub-samples were due to the preliminary intuition we had about inflation deviation persistence, mentioned in Section 2.2 (), and thereby allowed to a more robust result with regard to inflation deviation inertia varying over time. Furthermore, in ARFIMAX estimation, the short memory (AR(1)) and long-memory (d) parameters relative to the dependent variable (DESV_P) are estimated simultaneously.
The general specification of the ARFIMAX models is as follows, where a1–a7 are coefficients and μ is the error term:
From an economic theory viewpoint, we selected such a specification based on the following motivation: (i) DESV_Pt−1: like inflation rates per se, inflation deviations over time also presents an inertia degree, so that we accounted for AR(1) component; (ii) COMMt−1: supply or costs shocks, e.g., shocks to commodity prices; (iii) DESV_EXP_Pt−1: expectations on future inflation deviation; (iv) Yt−1: economic activity dynamics; (v) Et−1: exchange rate levels (pass-through effects on inflation deviation). The factors i-v are considered in studies built on the New-Keynesian Phillips Curve, such as Svensson (Citation1997), Clarida et al. (Citation1999), and Ponzoni and Zilli (Citation2015); (vi) moreover, DIVt−1: fiscal aspects, e.g. public debt ratio to GDP, which are essential to the Fiscal Theory of the Price Level (Woodford, Citation2001). All the regressors were considered in period t−1 due to the assumed lagged effects on inflation deviation, which is consistent with the underlying theoretical works.
It is also worth noting that before applying the ARFIMAX analysis we filtered the long-memory component into the explanatory series with (
, given
for each series) (Reisen, Citation1994), thereby adopting the resulting filtered series,
, i.e., with short-memory. The correct
of each time series was defined based on the GPH method previously mentioned. In contrast, most of the empirical studies regarding inflation rates and targeting in Brazil applied typical Dickey–Fuller tests, which have low power against the fractional alternative (Diebold & Rudebush, Citation1991; Hassler & Wolters, Citation1994). Besides, Lee and Amsler (Citation1997) found that the KPSS test cannot consistently distinguish between a process with nonstationary long-memory
and an unit root process (
). In this perspective, our current effort of using times series based on the fractional integration approach, as well as filtering possible long-memory process in data, can be regarded as an innovative trend in applied macroeconomics.
4. Results
4.1. A. univariate analysis of the inflation deviation series (DESV_P)
According to Brockwell and Davis (Citation2002), the autocorrelation function (ACF) of an autoregressive moving average process (ARMA) in the lag
converges rapidly to zero as
, in the sense that there exists
such that:
Stationary processes with much more slowly decreasing autocorrelation function, known as fractionally integrated ARMA processes, or more precisely as ARFIMA, with
, satisfy difference equations of the form:
where and
are polynomials of degrees
and
, respectively, satisfying
and
, for all z such that
,
is the backward shift operator, and
is a white noise sequence with mean 0 and variance
. The operator
is defined by the binomial expansion:
where and
,
The autocorrelation at lag
of an ARFIMA
process with
has the property:
This implies that, for an ARFIMA process, converges to zero as
at a much slower rate than
for an ARMA process. Consequently, fractionally integrated ARMA processes are said to have “long-memory”. In contrast, stationary processes whose ACF converges to zero rapidly, such as ARMA processes, are said to have “short memory”.
Thus, according to the value of , the ARFIMA
models have the following features: (i) if
, the process is considered to have short memory; if
, the process is stationary with average reversion and long-memory; if
, the series is said to be nonstationary with long-memory, but still shows mean reversion; and, if
, the series is nonstationary and does not present mean reversion.Footnote3
Therefore, the flexibility of the ARFIMA models increases the accuracy of the analysis, as the degree of persistence of the series can be estimated more appropriately. As shown by Reisen et al. (Citation2003), the performance of traditional unit root tests in the presence of long-memory has received much attention in recent years.
To Wei (Citation2006), an ARFIMA process contains several characteristics that, in finite samples, are similar to those of a nonstationary process. For instance, the ACF of an ARFIMA model decays very slowly, a phenomenon similar to the sample ACF of a nonstationary time series (ARIMA). Furthermore, the ARFIMA process and the nonstationary ARIMA models present periodogramsFootnote4
that diverge at the zero frequency. These similarities often lead to model misspecification. For example, a stationary ARFIMA model could be misspecified as a nonstationary ARIMA model. The consequence of this overdifferencing has some undesirable effects on parameter estimation and forecasting.
The sample autocorrelation function presented in does not allow a clear conclusion as to whether or not the series is stationary. Then, in this work, in addition to the ACF function, the following tests were used to verify if the DESV_P series presents an unit root: Augmented Dickey–Fuller – ADF (Dickey & Fuller, Citation1981); Phillips–Perron – PP (Phillips & Perron, Citation1988) and, Kwiatkowski-Phillips–Schmidt–Shin – KPSS (Kwiatkowski, Phillips, Schmidt, & Shin, Citation1992). The tests show that the DESV_P series is nonstationary in level. It is important to say that even if the series were stationary, there could be an underestimation of (the series would be sub differentiated). One characteristic of a fractionally integrated process is that the first difference of the series may result in an overdifferencing of the series. It is noteworthy that the autocorrelation function for the DESV_P series in first difference showed positive and negative signs, indicating that series may have been over differentiated (Morettin, Citation2008).
In turn, to test the presence of a fractionally integrated process, i.e., the presence of long-memory in DESV_P, the method of GPH (Geweke & Porter-Hudak, Citation1983) was adopted. The results of the semiparametric estimation of for different bandwidths,
, with
equal to the number of observations and
, are presented in . The results show that regardless of the choice of
, the estimates indicate a statistically significant behavior of long-memory in DESV_P series, namely: (i) stationary for
,
; and, nonstationary for the other bandwidths, with
(mean reversion).
Table 1. Unit root test for the variable in level.
Table 2. Estimated GPH for different bandwidths.
As described by Reisen et al. (Citation2001), the semiparametric estimators may exhibit bias in the estimator when the autoregressive
or moving average
parameters are nonzero. The best ARFIMA
models estimated by semiparametric and parametric methods are shown in and , respectively. For semiparametric estimators, when a model with autoregressive and moving average components was estimated, firstly the DESV_P series was filtered with
(
(see Reisen, Citation1994) and the filtered series,
, was adopted in the estimation of the ARFIMA
model’s short-memory parameters. To choose the best estimated models, the Akaike Information Criteria was used, with
. The Box-Pierce and Ljung-Box tests revealed that residuals of the estimated models were uncorrelated.
Table 3. Estimated semiparametric models.
Table 4. Estimated parametric modelsa.
The results of , and show that the values ranged between 0.4291 and 0.90. In all cases, the
value was below 1, that is, below the unit root case. In short, the univariate results suggest that the DESV_P series has long-memory, but with mean reversion. It means that if we based our analysis only on the typical unit root tests () we would overdifference DESV_P.
4.2. B. ARFIMAX estimates
To complement the ARFIMA analysis of the previous section, three parametric ARFIMAX models were estimated, considering three periods of time: (i) Model 1: period from May/2004 to Dec/2015; (ii) Model 2: period from May/2004 to Dec/2009; and, (iii) Model 3: period from Jan/2010 to Dec/2015. The ARFIMAX model is an extension of the ARFIMA model, using other time series as explanatory variables. Here, the explanatory variables used were: COMM, DESV_EXP_P, DIV, E and Y.
presents the estimated parametric models. As previously described, in this case, the short memory (AR(1)) and long-memory () parameters are estimated simultaneously. Thus, in the ARFIMAX estimates, the variable DESV_P was adopted in level. Furthermore, in order to avoid spurious estimates regarding the identification of the integration order (over or under-differentiation) of the explanatory variables, the GPH (Geweke & Porter-Hudak, Citation1983) method was applied, as a way for correctly estimating the value of each time series. The results of the semiparametric estimates for
are shown in and the applied bandwidth was given by
(see Reisen, Citation1994), thereby filtering and extracting the short-memory time series by means of
, given
(
) and
representing each explanatory variable.
Table 5. Estimates of parametric ARFIMAX models.
Table 6. Estimated GPH () for the explanatory time series.
Based on the ARFIMAX estimates we can address the main factors determining the behavior of the inflation deviation in Brazil over the adopted sample. From the explanatory variables perspective, commodity prices movements, nominal exchange changes and economic activity were the main factors determining the magnitude of the inflation deviation, especially if we account for the entire time sample (Model 1). The higher the level of such explanatory variables the higher the level of inflation deviation in the whole period. However, there exists a break on these coefficients when we divide the sub-samples. From May/2004 to Dec/2009, only economic activity affects inflation deviation with statistical significance (Model 2); on the other hand, from Jan/2010 to Dec/2015, expected inflation deviation (DESV_EXP_P) assumes the main driving role on inflation deviation.
Despite such idiosyncrasy of each sub-sample, it is possible to infer that the inflation deviation has a considerable inertia degree, even after removing the long-memory process. In other words, even when we apply all the time series with short-memory (by filtering the long-memory), DESV_P still presents an autoregressive (AR(1)) component that varies from 0.80 to 0.93, depending on the time sample, and with statistical significance at 1% in all cases. As we mentioned earlier, there are two basic visions regarding inflationary inertia. We follow that according to which such inertia can be analyzed even when there is not unit root, contrasting with the criterion in Reisen et al. (Citation2003).
Although throughout the entire time sample (Model 1) the inertia degree of inflation deviations was at 0.80, such a parameter was not constant over time or when we controlled for sub-samples. In Model 2, from May/2004 to Dec/2009, the inertia degree (AR(1)) stayed at 0.88, while it climbed up to 0.93 when we regarded the period from Jan/2010 to Dec/2015 (Model 3). This evidence corroborates our preliminary intuition on the issue mentioned in Section 2.2. It is important to point out that this latter sample is mainly associated with the macroeconomic policy performed by the president Dilma Rousseff (since 2011), which was ruled by goals in divergence from the Taylor’s principle (Barbosa et al., Citation2016; Moreira, Citation2015).
With regard to the estimates for the long-memory parameter in each ARFIMAX model, we observe that the estimated persistence degree is consistent with a stationary process with average reversion and long-memory, as the estimates ranged from 0.39 to 0.49. Again, from Jan/2010 to Dec/2015 (Model 3), the estimated
was higher (0.43) than from May/2004 to Dec/2009 (Model 2) (0.39), thereby confirming our results of a deterioration in the inflation deviation process over these last years in Brazil.
5. Concluding remarks
This work applied the fractional integration approach to study inflation deviation inertia in Brazil over the period from May/2004 to Dec/2015. We addressed the possible determinants of such a deviation by means of appropriately dealing with the long-memory behavior in data. From this perspective, this work contrasts with the most part of the recent literature in two aspects: (a) instead of studying the inflation inertia per se, we assessed the inflation deviation inertia, which is the main issue under a monetary regime based on inflation targeting; (b) instead of using conventional unit root tests to identify the integration order of the time series, as mostly found in the related literature, we applied the fractional integration approach, so that we avoided problems of spurious estimates in our ARFIMAX analysis.
Among the main results, we can focus on the considerable inflation deviation inertia in Brazil over the entire sample. Based on the univariate analysis, on the one hand, we confirmed the hypothesis of a long-memory generator process in Brazil’s inflation deviation, corroborating the previous findings in Reisen et al. (Citation2003) and Figueiredo and Marques (Citation2009). That is, studying such a variable by means of conventional unit root tests, such as ADF, PP and KPSS tests, and traditional differentiation mechanism leads to over or under-differencing time series and spurious estimates in multivariate models; on the other hand, even by excluding the long-memory component of the time series, based on our ARFIMAX estimates, DESV_P still presents an expressive and statistically significant autoregressive (or short-memory) (AR(1)) component.
Furthermore, we identified a process of deterioration of Brazil’s inflation targeting regime associated with the sample from Jan/2010 to Dec/2015, compared to the previous period (from May/2004 to Dec/2009). The ARFIMAX estimates showed an increase of estimated AR(1) over the former, besides a higher long-memory parameter for inflation deviation in such a period (Model 3). For economic policy purposes, a way for mitigating inflation deviation inertia is by controlling the indexation of administered or public prices and general wages, as well as by increasing the monetary and fiscal policies’ credibility. Finally, we also identified that, among our explanatory variables for the ARFIMAX estimation, commodity prices, nominal exchange rates and economic activity played the main role in affecting inflation deviation in Brazil when we consider the entire sample.
Finally, our work contributes to the international literature on inflation targeting regimes as it converges to previous evidence and intuition such as in Baxa et al. (Citation2015), Mishkin and Schimidt-Hebel (Citation2007), Benati (Citation2008) and Moreira (Citation2016). That is, although the adoption of an inflation target can be accompanied by improvements in inflation dynamics over time, the true factors determining or at least conditioning such an outcome could be beyond the choice of a specific nominal anchor. Constraints relating to monetary and fiscal policies’ credibility are an important condition (Benati, Citation2008; Moreira, Citation2016). In our study, the evidence for a deterioration of the inflation deviation dynamics since 2010, in Brazil, can be associated with a social loss in terms of an increase of the public debt to GDP ratio and the public’s general perception of less efficiency in the conduction of the economic policy during President Dilma Rousseff’s mandate.
Disclosure statement
No potential conflict of interest was reported by the authors.
Additional information
Notes on contributors
Ricardo Ramalhete Moreira
Ricardo Ramalhete Moreira is Associate Professor at the Federal University of Espírito Santo (Brazil). He has a Ph.D. in Economics and his main research is based on monetary and fiscal issues.
Edson Zambon Monte
Edson Zambon Monte is Adjunct Professor at the Federal University of Espírito Santo (Brazil). He has a Ph.D. in Environmental Engineering and his main research is based on econometric methods.
André Abdala
André Abdala has a Master in Science degree in Economics (Federal University of Espírito Santo).
Notes
1 According to the reported literature on inflation inertia (e.g., Reisen et al., Citation2003), the existence of unit root (I(1)) in the inflation rate would be a sufficient condition for the emergence of hyperinflation processes over time. An I(2) time series is not a necessary condition for such a phenomenon. If an inflation rate presents unit root in level values, but is stationary in first difference (I(1)) with a positive and statistically significant constant, the inflation rate will grow indefinitely over time and around a constant mean growth rate. We did not focus on cases of hyperinflation in this work, but only on cases in which inflation deviation does not present stationarity (short-memory), that is, in cases of slow average reversion (i.e., long-memory) under a context of inflation targeting regime, like in Brazil in recent years.
2 In this model, the dependent time series presents a fractional behavior in both average and variance. Arfima stands for Auto-Regressive Fractionally Integrated Moving Average; Figarch stands for Fractionally Integrated Generalized Auto-Regressive Conditionally Heteroskedastic.
3 The intermediate memory of an ARFIMA model is defined when the memory parameter is in [−0.5; 0).
4 The periodogram function describes how the total variability of the series is partitioned over the various components relating to each of the Fourier frequencies.
References
- Baillie, R. T., Chung, C.-F., & Tieslau, M. A. (1996). Analysing inflation by the fractionally integrated Arfima–Garch model. Journal of Applied Econometrics, 11, 23–40.
- Ball, L. (1999a). Efficient rules for monetary policy. International Finance, 2, 63–83.
- Ball, L. (1999b). Policy rules for open economies. In J. B. Taylor (Ed.), Monetary policy rules (pp. 127–144). Chicago, IL: University of Chicago Press.
- Ball, L. M., & Sheridan, N. (2004). Does inflation targeting matter? In B. S. Bernanke & M. Woodford (Eds.), The inflation-targeting debate (pp. 249–282). Chicago, IL: Chicago University Press.
- Barbosa, F. D. H., Camelo, F. D., & Joao, I. C. (2016). A Taxa de Juros Natural e a Regra de Taylor no Brasil: 2003-2015. Revista Brasileira De Economia, 70, 399–417.
- Barbosa, F. D. H., & McNelis, P. D. (1989). Indexation and inflationary inertia: Brazil 1964–1985. World Bank Economic Review, 3, 339–357.
- Barro, R. J., & Gordon, D. B. (1983). Rules, discretion and reputation in a model of monetary policy. Journal of Monetary Economics, 12, 101–121.
- Baxa, J., Plašil, M., & Vašíček, B. (2015). Changes in inflation dynamics under inflation targeting? Evidence from Central European countries. Economic Modelling, 44, 116–130.
- Benati, L. (2008). Investigating inflation persistence across monetary regimes. The Quarterly Journal of Economics,123, 1005–1060.
- Bernanke, B. S., & Mishkin, F. S. (1997). Inflation targeting: A new framework for monetary policy? Journal of Economic Perspectives, 11, 97–116.
- Brockwell, P. J., & Davis, R. A. (2002). Introduction to time series and forecasting (2nd ed.). New York: Springer.
- Cerisola, M., & Gelos, R. G. (2009). What drives inflation expectations in Brazil? An empirical analysis. Applied Economics, 41, 1215–1227.
- Clarida, R., Galí, J., & Gertler, M. (1999). The science of monetary policy: A new Keynesian perspective. Journal of Economic Literature, 37, 1661–1707.
- Cysne, R. P. (1985). A Relação Phillips no Brasil: 1964-66×1980-1984. Revista Brasileira De Economia, 39, 401–422.
- de Oliveira Veloso, G., Feistel, P. R., de Oliveira Hoeckel, P. H., Casagrande, D. L., & Dos Santos, C. A. P. (2013). A curva de phillips: Uma análise da economia brasileira de 2002 a 2012. Revista Economia & Tecnologia (RET), 9, 05–19.
- Dickey, D. A., & Fuller, W. A. (1981). Likelihood ratio statistics for autoregressive time series with a unit root. Econometrica : Journal of the Econometric Society, 49, 1057–1073.
- Diebold, F. X., & Rudebush, G. D. (1991). On the power of Dickey–Fuller tests against fractional alternatives. Economics Letters, 35, 155–160.
- Durevall, D. (1998). The dynamics of chronic inflation in Brazil, 1968-1985. Journal of Business and Economic Statistics, 16, 423–432.
- Figueiredo, E. A. D., & Marques, A. M. (2009). Inflação inercial como um processo de longa memória: Análise a partir de um modelo Arfima-Figarch. Estudos Econômicos, 39, 437–458.
- Galí, J., & Gertler, M. (1999). Inflation dynamics: A structural econometric analysis. Journal of Monetary Economics, 44, 195–222.
- Geweke, J., & Porter-Hudak, S. (1983). The estimation and application of long memory time series models. Journal of Time Series Analysis, 4, 221–238.
- Gonçalves, C., Eduardo, S., & Carvalho, A. (2009). Inflation targeting matters: Evidence from OECD economies’ sacrifice ratios. Journal of Money, Credit and Banking, 41, 233–243.
- Gonçalves, C., Eduardo, S., & Salles, J. M. (2008). Inflation targeting in emerging economies: What do the data say? Journal of Development Economics, 85, 312–318.
- Gottschalk, R. (2003). Testing for breaks in inertia: An alternative approach. Applied Economics Letters, 10, 161–163.
- Hassler, U., & Wolters, J. (1994). On the power of Dickey-Fuller tests against fractional alternatives. Economic Letters, 45, 1–5.
- Kwiatkowski, D., Phillips, P. C. B., Schmidt, P., & Shin, Y. (1992). Testing the null hypothesis of stationarity against the alternative of unit root. Journal of Econometrics, 54, 159–178.
- Lee, H. S., & Amsler, C. (1997). Consistency of the KPSS unit root test against fractionally integrated alternative. Economics Letters, 55, 151–160.
- Machado, V. D. G., & Portugal, M. S. (2014). Measuring inflation persistence in Brazil using a multivariate model. Revista Brasileira De Economia, 68, 225–241.
- Melnick, R., & Strohsal, T. (2017). Disinflation in steps and the Phillips curve: Israel 1986–2015. Journal of Macroeconomics, 53, 145–161.
- Mishkin, F. S., & Schmidt-Hebbel, K., 2007. Does inflation targeting make a difference? (Technical report). National Bureau of Economic Research.
- Molinares, F. F., Reisen, V. A., & Cribari-Neto, F. (2009). Robust estimation in long-memory processes under additive outliers. Journal of Statistical Planning and Inference, 139, 2511–2525.
- Moreira, R. R. (2015). Reviewing Taylor rules for Brazil: Was there a turning-point? Journal of Economics and Political Economy, 2, 276–289.
- Moreira, R. R. (2016). Measuring the monetary policy’s structural credibility by the expected inflation determinants: A Kalman filter approach for Brazil. Brazilian Review of Econometrics, 36, 162–183.
- Morettin, P. A. (2008). Econometria financeira: Um curso em séries temporais financeiras. São Paulo: Editora Blucher.
- Novaes, A. D. (1993). Revisiting the inertial inflation hypothesis for Brazil. Journal of Development Economics, 42, 89–110.
- Phillips, P. C. B., & Perron, P. (1988). Testing for unit roots in time series regression. Biometrika, 75, 335–346.
- Ponzoni, G. A., & Zilli, J. B. (2015). Unemployment and inflation: An estimated Phillips Curve for Brazil (2002-2014). Journal of Finance and Economics, 3, 77–85.
- Reisen, V., Abraham, B., & Lopes, S. (2001). Estimation of parameters in ARFIMA processes: A simulation study. Communications in Statistics Simulation and Computation, 30, 787–803.
- Reisen, V. A. (1994). Estimation of the fractional difference parameter in the ARIMA(p, d, q) model using the smoothed periodogram. Journal of Time Series Analysis, 15, 335–350.
- Reisen, V. A., Cribari-Neto, F., & Jensen, M. E. (2003). Long memory inflationary dynamics: The case of Brazil. Studies in Nonlinear Dynamics & Econometrics, 7, 1–16.
- Rudebusch, G. D. (2006). Monetary policy inertia: Fact or fiction? International Journal of Central Banking, 2, 85–135.
- Svensson, L. E. O. (1997). Inflation forecast targeting: Implementing and monitoring inflation targets. European Economic Review, 41, 1111–1146.
- Taylor, J. B. (1993). Discretion versus policy rules in practice. Carnegie Rochester Conference Series on Public Policy, 39, 195–214.
- Wei, W. W. S. (2006). Time series analysis: Univariate and multivariate methods (2nd ed.). Nova York: Pearson Education.
- Woodford, M. (2001). Fiscal requirements for price stability. Journal of Money, Credit and Banking, 33, 669–728.
- Wu, T. Y. (2004). Does inflation targeting reduce inflation? An analysis for the OECD industrial countries. Brasília: Brazilian Central Bank, 2004 (Working Paper Series n. 83).