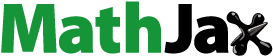
ABSTRACT
Implicit gender bias may affect hiring and promotion decisions, implying inefficiencies in the outcome of selection processes. We focus on the dynamics of gender bias when selecting candidates for a committee or position, and obtain the long-run female share as well as the conditions for a glass ceiling effect in a hierarchical structure. Candidate selection is modeled as a Markov process, where the outcome of the dynamic selection process is affected by bias asymmetries and incumbency advantages. Moreover, we formalize and characterize different types of glass ceiling, analyze how the gap between the groups evolves in the corporate hierarchy and show that the dynamics of promotion may indeed generate glass ceilings.
1. Introduction
The metaphor for a glass ceiling was initially used to represent the blocked promotional opportunities for women in the corporate hierarchy (Hymowitz and Schellhardt, The Wall Street Journal 1986).Footnote1 A glass ceiling inequality is a gender or racial difference, not explained by other job-relevant characteristics of the employee, which increases as one moves up the hierarchy (Cotter, Hermsen, Ovadia, & Vanneman, Citation2001). In the empirical literature, the glass ceiling has been identified with higher wage differences at higher levels (e.g., Arulampalam, Booth, & Bryan, Citation2007; Gardeazabal & Ugidos, Citation2005; Morgan, Citation1998), a probability of promotion that becomes smaller at higher levels (e.g., Elliot & Smith, Citation2004; McDowell, Singell, & Ziliak, Citation1999; Zeng, Citation2011; Olson & Becker, Citation1983; Maume, Citation1999; Gjerde, Citation2002), a lower ratio of probabilities of women to men being promoted (Baxter & Wright, Citation2000), or simply a lower proportion of women at the top levels (e.g., Bain & Cummings, Citation2000). Purcell, MacArthur, and Samblanet (Citation2010) contain a good summary of the empirical evidence on the glass ceiling effect in a large variety of occupational settings. They acknowledge the fact that some studies are not comparable due to “the ambiguity over what the glass ceiling really means and how it should be measured”. Clearly, the results cannot be expected to be the same when using the female share in top positions, the probability of promotion, or the ratio of probabilities of women to men being promoted. In this paper we clarify the relationship between the different glass ceiling definitions used in empirical work, the kind of inequality they are reflecting and provide conditions to obtain each of them.
The theoretical explanations for the presence of discrimination can be extended to explain why the consequences of discrimination are more pronounced at the top levels of corporate or political hierarchies. The main challenge is to explain how differences in wage or promotion, not based on differences in productivity, can be maintained in the labor market. Becker (Citation1957) introduced the idea of a taste for discrimination that would lead employers to forego profits in order to maintain the differences. Market-based explanations also include statistical discrimination: when abilities are not observable, employers may use the correlation between race or gender and productivity as a signal (Arrow, Citation1972, Citation1973; Phelps, Citation1972). Milgrom and Oster (Citation1987) model the abilities of disadvantaged workers as less visible, more difficult to evaluate, and therefore employers pay and promote members of this group less. This effect interacts with the human capital investment decision of disadvantaged workers, who find this investment less profitable so that their average productivity is lower (Coate & Loury, Citation1993a; Lundberg & Startz, Citation1983). As an extension of this theory, one possible interpretation for glass ceiling effects is that the disadvantaged group’ s abilities are even less visible or more difficult to evaluate (relative to the other group) at higher levels than at lower levels, which would increase the discrimination gap, under the assumption that the problem of observability of minority workers productivity becomes relatively more severe at higher levels.
Other explanations for discrimination are based on homophilic preferences in coworkers and clients. Assuming that productivity is influenced by a good work relationship among coworkers and clients and that the initial proportion of males is higher, homophilic preferences would perpetuate discrimination against women. Furthermore, if at top levels productivity is more influenced by male clients or coworkers preferences or mentorship than at low levels, this could produce a glass ceiling effect.
The notion of implicit bias has been considered an important element of discrimination in hiring (Espinosa & Gardeazabal, Citation2020; Kleinberg & Raghavan, Citation2019). In this case, there is no need to explain how discrimination can be maintained in the labor market, since it is unintentional and unobserved. If the use of unconscious gender stereotypes is more relevant at the top levels of the corporate hierarchy, then this would also contribute to the explanation of glass ceilings.
This paper introduces a new element that contributes to the explanation of glass ceiling effects and it is not based on an increasing gender bias or any differences getting more severe at the top levels of the hierarchy. We show that the dynamics of promotion may indeed reinforce an existing gender difference and lead to a glass ceiling effect even with a constant perception bias.
We look at three different indexes of gender gap: (i) the percentage of women at each hierarchical level, (ii) the odds of reaching a given level for men and women, and (iii) the odds ratio of female and male promotion at each level. Using these indexes we formalize glass ceiling effects.
It is worth noting that glass ceiling effects in our model do not come from differences in abilities or preferences, which are assumed to be identical for men and women, but from the dynamics of a selection process that reinforces initial differences even with a constant perception bias. Although the assumption of a constant bias might not be realistic, it allows us to study the net effect of the bias in the long run. The sequential candidate selection for a given level of a hierarchical structure is modeled as a Markov process. When all candidates have the same abilities or abilities are not observable, the selection process is conceived as a random draw from the population of participating individuals. We first analyze the short and long run effects of a constant gender-specific bias at different hierarchical levels, which could be asymmetric among the decision makers. Under our assumptions, the female ratio is driven by a discrete Markov process, and we set the conditions to obtain the limit of the process using the properties of the associated Markov chain.
Our main contribution is to show that glass ceiling effects (discrimination becoming more severe at higher levels) may appear without the need for a larger bias at higher levels. Even with a constant bias at all levels of the hierarchy, the dynamics of a selection process with apparently gender-neutral features (e.g., previous job experience) reinforces the initial differences and produces a glass ceiling effect. A better understanding of why a constant gender bias has more pronounced effects at the top levels of corporate hierarchies may be helpful to design policies that mitigate the effects and reduce gender disparities. One implication of our model is that when incumbency matters, programs that enhance diversity or affirmative action may be necessary.
The paper is organized as follows. Section 2 sets up the main assumptions of the model and the mathematical framework. Section 3 analyzes the time dynamics and the effect of incumbency advantages (inertia) and bias asymmetries. Section 4 introduces a hierarchical structure and presents the glass ceiling effect results. Section 5 discusses further research and concludes.
2. Framework and main assumptions
In this section, we model selection processes that choose from among a population of candidates. Throughout the paper, we refer to the selection of members of a committee, but more generally our model can be applied to promotion decisions. We deal with the dynamics driving the proportion of women (or minority groups) on committees, which are represented by a random variable . The index
accounts for time, whereas the index
accounts for the position of the committee in a hierarchical structure. We assume there is a large number of candidates and hierarchical structures in the economy so that we can deal with proportions. To simplify the notation, we may sometimes drop index
when the analysis is focused on time for a given hierarchical level, or we may drop index
when we study the hierarchy.
2.1. Selection process over time. Promotion to level

We first study the promotion process to a given hierarchical level over time. Concerning the candidates, let
,
denote the number of women and men in the population, which are assumed to be constant over time. Among them,
,
are the number of those who run as candidates for a position at period
. Let
be the proportion of women in the pool of candidates at period
. We assume there is no difference between the behavior or abilities of men and women, or that any differences in abilities are not observable to the decision makers.Footnote2
At each period , committees are renewed. Let us denote by
the gender composition of the committee at
,
, where
is the number of women and
the number of men, and
is the size of the committee, assumed for simplicity to be constant over time. The female ratio at period
is thus
, and the male ratio is
.
We represent the selection process as a dynamic model: . This conditional expectation indicates that the expected proportion of women in period
is related to the past proportion
and some set of parameters
. This formulation represents a link between past and future decisions and introduces dynamic considerations. We describe two possible scenarios that could fit within this general dynamic model.
First, consider situations where actual members of committees have the power to influence the choice of future members. This may be the case, for instance, of the selection process for a job or decisions regarding tenure in academic contexts.Footnote3 More generally, it reflects the idea that the decision on promotions up the academic, political or corporate ladder are taken by people who have climbed that ladder before.Footnote4 As will be seen, if the decision makers are heterogeneous concerning their perceptions of candidates (asymmetric bias), the conditional expectation will be different from the unconditional and will depend on the past composition of the committee.
Second, the decision makers may use the proportion of women on past committees as a signal of female ability for that particular committee or perhaps as a signal of their willingness to run for office. That is, the decision process takes into account not only the proportion of female candidates but also the proportion of women on past committees (inertia).
Our goal is to analyze the dynamics of the random variable , the effect of the initial conditions and the long-run female share.
This framework is characterized by the following assumptions:
Assumption A1. The number of selected female members follows a binomial distribution , where
is the size of the committee, assumed to be constant over time, and
is the probability of a woman being selected.
This assumption is motivated by the fact that, when the abilities of the candidates are not observable or they are equal, the selection process can be conceived as a random draw from the population of participating male and female candidates (), so that the number of female members will follow a binomial distribution.Footnote5 With no gender bias, the probability of success coincides with the female proportion in the pool of candidates
, but gender bias will decrease the probability of success so that
.
Assumption A2. for all
.
That is, for each level of the hierarchy, the proportion of female candidates remains constant over time (although it will differ across levels).
We analyze the dynamics of a sequence of the proportion of women, which evolves over time. For each level , the proportion of women given by the steady state for that level is denoted
.
2.2. The selection process up the corporate ladder. Glass ceiling effects
To study promotion decisions at different levels of the hierarchy, we consider that for each hierarchical level , the female proportion
is given by the steady state, that is,
.
Our ultimate goal is to check whether female candidates have fewer opportunities to reach the higher levels. One possibility is to see whether the ratio of women is decreasing along the corporate ladder. However, we may be more interested in the individual probabilities of promotion for participating female candidates. In this case, a test for a glass ceiling effect should look at whether the female odds ratio (female vs. male) for the initial (at the beginning of a career) individual probability of reaching level is a sequence that decreases with
. A third possibility is to check whether the odds ratio at
is lower than at
.
The number of women (men) that are candidates to reach level in the steady state is denoted by
. We need to describe the dynamics of the female proportion
and the limit of
when
in order to analyze the conditions for a glass ceiling effect.
We use and
to denote the unconditional individual probabilities of reaching level
, for female and male candidates, respectively;
and
are the individual probabilities of reaching level
, for female and male candidates who have already reached level
.
We can now state the formal definitions of a glass ceiling effect:
Definition 1. There is a type 1 glass ceiling effect () when the sequence of unconditional expected female proportions
is strictly decreasing with level
.
Definition 2. There is a type 2 glass ceiling effect () when the odds ratio
is a sequence strictly decreasing with
.
Definition 3. There is a type 3 glass ceiling effect () when the odds ratio
is a sequence strictly decreasing with
.
As we will see, these definitions are not equivalent although they are related.
3. Time dynamics
This section presents and discusses the evolution over time of the gender composition of a given level in the hierarchy, that is when only one step in the promotion process is considered. We present the results in two different scenarios: asymmetries in the implicit bias and inertia in the decision processes. Since the dynamics is stochastic, we present the average results.Footnote6
3.1. The effect of asymmetric bias
We assume that there is a gender bias in the selection process and that the bias of each decision maker has the same weight in the final decision. We allow for an asymmetric perception of candidates by male and female decision makers.Footnote7 As a consequence, the final decision will have the average bias of the decision makers,Footnote8 that is, if a fraction of the decision makers at
have a bias
and a fraction
has a bias
, the average bias of the decision at
will be
.Footnote9 We assume that the parameters that measure bias do not change with the level of the committee or through time.
Given a hierarchical level , we analyze the promotion decisions over time. In this setup and under the assumptions above, the probability of selecting a woman for a position at each period
will be determined by:
where . Thus, the average bias affects the probability of selecting a woman (see Assumption
). In particular, the probability of success
diminishes in proportion to the average bias of the decision makers. The unbiased perception, (
), implies that the probability of selecting a woman coincides with the female ratio in the candidate population. When
, and
, only male decision makers suffer from gender bias; in the case
, both groups select with the same biased perspective (symmetric bias); last, when
, both groups have a biased perception, but the bias of male decision makers is greater than that of females. In this setup, we can state:
Theorem 1. Under Assumptions A1 and A2, if is given by (1) then
is a random variable with unconditional mean:
where denotes the initial female ratio.
When ,
converges in distribution to a unique limit
. with mean equal to
Proof. See the appendix.
Remark 1. When (symmetric bias),
.
Corollary 1. is a decreasing sequence whenever
, strictly decreasing if
.
Proof. See the appendix.
As expected, the female ratio in the steady state increases with and
(i.e., it decreases with the gender bias). Less obvious is the fact that bias asymmetry affects the long-run shares. As stated in the next result, in the steady state asymmetric biases have a stronger effect on gender inequality.
Proposition 1. Let be the weighted average bias in the population, where
and
are the female and male proportions, respectively. Then, an increase in the asymmetry between female and male bias,
and
, keeping the average bias of the decision makers constant, leads to lower female rates in the long run. The long run female share,
, reaches its maximum value when
.
Proof. See the appendix.
3.2. The effect of incumbency advantage (inertia)
Political scientists have focused on the incumbency advantage as an explanation for the underrepresentation of women in politics; this entry barrier inhibits electoral opportunities for previously excluded groups such as women or minorities (Fox & Lawless, Citation2005, Citation2011; Karnig & Welch, Citation1979; Studlar & Welch, Citation1990). A similar argument is given in Welch (Citation1977): Women tend not to work in the fields of law and business, fields from which most political candidates emerge, so that women may lack the qualifications to pursue elective office.
In this section, we use this motivation to introduce an incumbency advantage so that the decision makers at any given period are influenced by the female proportion in the past committees.
To that end, we consider that the probability of success (see Assumption A1) is affected not only by the proportion of women in the pool of candidates but also by the perception of decision makers, who take into account the female share observed in the past on that committee. In other words, when inertia is important, fewer women on previous committees will be associated with fewer women being considered for present committees. For this case, we model the probability of a woman being selected as a linear combination between the observed ratio of experienced women, denoted by , and the female ratio in the pool of candidates
, that is,
, where
, and similarly for men:
This linear combination indicates how the decision makers weigh the two factors: the proportion of women in the pool of eligible candidates, , and the inertia, measured as the past proportion of women on the committee,
. For high values of
, it will be very difficult to select a female candidate for committees or in fields where women have been historically absent. If hardly any women are seen on those committees, it would seem “natural” to keep this proportion low with the argument that the proportion of experienced women is also low (although the proportion of women in the pool of eligible candidates may be larger). Thus, parameter
accounts for the importance of previous experience in the job and inertia. The higher the parameter
is, the stronger the inertia.
Under no bias, the proportion of women perceived as possible candidates is , and therefore, this would be the resulting theoretical proportion of women on the new committee,
.
However, when there is a gender bias, , the probability of success is diminished in proportion to the bias:
Remark 2. Inertia and bias are taking into account different effects. Inertia models the idea that changes may take place at a very low pace, even if bias were decreasing; this may be due to several factors: the importance of previous experience in the job, incumbency advantages, the absence of information on eligible female candidates, partial renewal of existing committees, etc. Inertia makes the perceived set of eligible female candidates smaller. On the other hand, the bias models the fact that, considering the perceived set of suitable female candidates, decision makers select them with a lower probability of success than their proportion in the pool of eligible candidates.
Theorem 2. Under Assumptions A1 and A2, if is given by (4) then
is a convergent Markov process with a unique limit
, with mean
Proof. See the appendix.
When there is no bias (), as long as inertia is not extreme (
), we have that
so that the expected female share in the long run turns out to be equal to the female proportion in the pool of candidates. This implies that inertia alone will not lead to a gender gap in the long run. For a finite number of periods, however, the outcome may depend on the initial proportion, so that if
, the larger the inertia (the higher
), the longer it will take to get closer to the limit
.
When , we have that
, so the expected female share
(see (5)) is strictly lower than the proportion of women in the population
. Moreover, the term
is decreasing with
. When there is no incumbency advantage (
),
, the expected female share is constant over time, and it corresponds to the symmetric bias case (see Remark 1). However, for
,
: the inertia or incumbency advantage reinforces the effect of the bias, and the impact is higher, the higher the weight of previous experience (inertia) is. We summarize our results in the following proposition:
Proposition 2. Under Assumptions A1 and A2, if is given by (4), then the more important the incumbency advantage (the higher
), the lower the expected female share will be in the limit,
.
presents the limit expected female shares for different parameters values ( in all cases), to show the interaction of bias and inertia. Note that, even under a small bias (
close to one), if the inertia parameter is high, the effect may be rather large. In other words, we could have nearly unbiased committees, but when there is incumbency advantage, discrimination will persist even in the long run. Moreover, the effect is noticeable even when the inertia is moderate.
Remark 3. The introduction of incumbency advantage or inertia is symmetric, equal for men and women, but when it interacts with gender bias, it reinforces its effect resulting in a lower female share. Our results indicate that the interaction between bias and incumbency advantage tends to exacerbate the consequences of a bias, and this is independent of the initial value . On the contrary, in the absence of bias, any unbalanced initial composition would be corrected in the long run, no matter how large
is, as long as it is lower than
. The time needed to reach the proportion
will depend on the inertia parameter, but in the limit the female share is the same as their proportion in the population.
Remark 4. Note that if and
there are two absorbent states
, since once the event
occurs,
will always be zero for any period
, and the same would be the case for
if
. When
,
is still an absorbent case since
. However,
which leads to a positive probability,
, of male selection.
Remark 5. In the asymmetric case, ,
becomes non-linear:
Table 1. The interaction of inertia and bias. Limit expected female shares
and the closed-form solution for is not straightforward. It can be obtained from the limit probability distribution
, being
the unique solution of
.
The next section introduces a hierarchical structure to study glass ceiling effects.
4. The corporate ladder. Glass ceiling effects
Assume the corporate ladder has steps (
). The selection process to reach any level
takes place as described previously (see
):
where includes, among other parameters, the female proportion in the pool of candidates for promotion to level
,
, and the size of the committee at level
,
, assumed non-increasing in
. Let
be the proportion of women hired at each hierarchical level
. For simplicity, we consider that these proportions are given by the expected values in the long run:
Assumption A3. (Long run) At each level , the female proportion
is:
.
To make the problem tractable, we further assume that professional careers are such that only people at hierarchical level may be considered for promotion at level
and that the individual probabilities of being hired are independent.
Assumption A4. (No jumps) To be a candidate for a position at level , it is necessary and sufficient to have reached level
. Thus,
.
This assumption implies in particular that the female proportion in the pool of candidates for promotion at level ,
, depends on
.
Assumption A5. (Independence) The female (male) individual probabilities of being promoted from level to level
are equal and independent.
Under these assumptions, the individual probabilities from level to level
will be the ratio between the number of female candidates selected for level
,
, and the number of female candidates,
, and can be written as:
The following result relates the three definitions of glass ceiling (,
and
).
Theorem 3. Under Assumptions ,
and
There is GC1 if and only if there is GC2.
If there is GC1, then there is GC3 if and only if the sequence
is decreasing with
, where
denotes the odds ratio
.
Proof. See the appendix.
From Assumptions A3 and A4, since , the female proportion at a given level
,
, can be written as a function of the previous period proportion as:
where can be interpreted as the link function between consecutive levels.
This notation will be used to characterize the evolution of the female proportion and the presence of glass ceiling effects in terms of the link function .
From Theorem 3, we can rewrite ,
and
in terms of
:
Proposition 3. Under Assumptions A3, A4 and A5,
(i) There is GC1 and GC2 if and only if
(ii) If , there is GC3 if and only if
Proof. See the appendix.
Remark 6. If , there is no
and no
. In that case we have
if and only if
Proposition 3 characterizes the dynamic selection processes that would result in the different types of glass ceilings. The next subsection shows that a constant bias is sufficient to generate and
, but not the stricter version of glass ceiling,
. We then show that asymmetric bias and inertia, modeled as in Section 3, reinforce
and
. However, if the bias and inertia parameters are constant across levels, the model will not yield
. Finally, we provide some family of dynamic selection processes that generate
.
4.1. Selection processes and glass ceiling effects
From Theorem 1, under asymmetric bias, the expected proportion of women at level in the steady state is EquationEquation (3)
(3)
(3) :
If the parameters (,
) are constant across levels, the link function
can be written as
where the subindex denotes that bias is asymmetric. Considering the dynamics under inertia, from
we have:
If the bias and inertia parameters (,
) are constant across levels, the link function
can be written as:
where the subindex denotes that there is inertia. The next result characterizes the glass ceiling effects in the two scenarios, (8) and (9).
Theorem 4. Under Assumptions A1 to A5, if the dynamics is driven by the link function or
, there is GC1 and GC2 but there is no GC3.
Proof. See the appendix.
Remark 7. Note that when the bias is symmetric and there is no inertia (), the link functions (8) and (9) become:
To illustrate how the link functions that generate the sequences of are related to the three types of glass ceilings, we show the results for
:
Proposition 4 (Symmetric Constant Bias). Under Assumptions A3 to A5, for the link function :
The female share at each level
,
is decreasing in
(GC1).
The odds ratio for the unconditional probabilities of reaching level
is a decreasing sequence that tends to zero (GC2):
For the conditional probabilities, given that level
has been reached, the odds ratio is an increasing sequence that tends to
as
becomes large (no GC3):
Proof. The results are straightforward from Theorem 4.
From this proposition, we find type 1 and 2 glass ceilings in the baseline model, but not of type 3. The odds ratio for the conditional probability is increasing with , reflecting the fact that the lower the gender bias, the better the odds ratio for female candidates are. The odds ratio increases with
, so that there is no
. If we fix the selection rate
(the lower the number of positions at
for each position at
, the more competitive the process is) a female individual probability is constant with
and equal to
. However, for male candidates, the individual probability gets worse at the upper levels (
decreases with
); the reason is that the lower proportion of women at the top levels (due to the bias) makes the terms of the competition tougher for men. A constant female individual probability and a decreasing male probability result in an increasing odds ratio for female candidates. Note also that as
gets large, the individual male probability tends to
, while the individual female probability is
, so that the female odds ratio tends to
.
For the asymmetric bias, from (8):
so is a decreasing sequence (
and
) which tends to zero. For the odds ratio
we have that
which is increasing in (no
) and tends to
. With inertia, from (9) we have
Thus,
so is a decreasing sequence (
and
) which tends to zero. For the odds ratio
, we have that
which tends to .
A natural question arises: Which are the selection processes that yield ? The conditions on
are given in Proposition 3 (ii). Consider simple polynomials of the form
, with
and
. Then, Condition (7) can be written as
which requires for the expression to hold for any
. In other words, nonlinear dynamics are necessary for the condition on
to hold. To illustrate this, we present two examples of nonlinear dynamics.
Example 1. To illustrate the selection processes that would generate nonlinear dynamics, consider the case of a symmetric bias, which is not constant, but an increasing function of the female proportion : As the female presence in a given field increases, the lower the bias is (or if the female rate at the upper levels decreases, then the bias of the selection process increases). Then, from
:
Assume is a linear function
, with
, then
(
). On the other hand, in this case Condition (7):
holds for any as long as
.
Example 2. The nonlinear dynamics could also be driven by a reduction in the participation rate along the corporate ladder. Consider the case where the female share is a linear function of the female rate among the candidates, , but there are participation costs that make the participation rate decrease as
decreases:
Assume for instance that the participation rate as a function of is of the form
. Then, the dynamics are the same as in the previous example.
5. Conclusions and further research
In a recent OECD report, Thévenon, Ali, Adema, and Salvi Del Pero (Citation2012) estimate the effect of the gender gap on economic growth for OECD countries: a potential gain of 12% of the size of the total economy by 2030, if complete convergence occurs.Footnote10 This magnitude is by no means negligible, and it would call for policy measures. It is important to understand how selection processes in the labor market interact with gender bias in order to implement the correct policies. We propose a framework to study the theoretical underpinnings of the glass ceiling effect.
Assuming there is gender bias, we show how the dynamics of the underlying stochastic processes lead to different female shares in the long run and how features such as inertia or bias asymmetries reinforce the glass ceiling effects. Some of these features are present in many selection processes, and they may not be considered discriminatory or responsible for the glass ceiling effect by themselves. For example, the requirement of previous job experience may be symmetric for men and women and, nevertheless, it exacerbates inequality when combined with gender bias; in the extreme case of incumbency advantage, the stationary proportion of women converges to zero.
Our analysis could be extended in several directions. First, perception bias may not remain constant; for example, if members of the committee update their beliefs after observing group or individual performance, the bias could decrease over time. Second, female and male participation in the labor market may respond to the discrimination bias, affecting the composition of the pool of suitable candidates. Last, job-seekers may be heterogeneous in abilities with high ability individuals being more productive; this last scenario introduces efficiency considerations in the analysis of the glass ceiling effect. These extensions are left open for future research.
This paper focuses on demand-side explanations for the low female representation in corporate leadership. There are also supply-side explanations based on women’s preferences, productivity or expectations (Bertrand, Goldin, & Katz, Citation2010; Hernan-Gonzalez & Kujal, Citation2015; Espinosa & Kovarik, Citation2015;; Sassler, Glass, Levitte, & Michelmore, Citation2017, among others), or willingness to participate in competition for promotion (Gneezy, Leonard, & List, Citation2009; Gneezy, Niederle, & Rustichini, Citation2003; Gneezy & Rustichini, Citation2004; Günther, Ekinci, Schwieren, & Strobel, Citation2010; Niederle & Vesterlund, Citation2007). However, whether this lower participation rate in promotion contests comes from preferences or it is partly the best response to biased selection processes is an open question. Our model provides a setup to analyze these and other issues concerning the dynamics of group discrimination.
Acknowledgments
This study was funded by the Ministry of Science and Innovation (PID2019-108718GB-I00) and the Basque Government (IT 1336-19).
Disclosure statement
No potential conflict of interest was reported by the authors.
Additional information
Funding
Notes on contributors
María Paz Espinosa
María Paz Espinosa (MA and Ph D in Economics, Harvard University) is Professor of Economics at the University of the Basque Country.
Eva Ferreira
Eva Ferreira (MS in Probability and Statistics, Courant Institute of Mathematical Science - New York University; Ph D in Economics, UPV/EHU) is Professor of Statistics at the University of the Basque Country.
Notes
1 “The glass ceiling effect is an unseen, yet unbreachable barrier that keeps minorities and women from rising to the upper rungs of the corporate ladder, regardless of their qualifications or achievements.” (Federal Glass Ceiling Commission, Citation1995). See also Jackson and O’Callaghan (Citation2009).
2 See Espinosa and Ferreira (Citation2017) for a selection process where abilities may be heterogeneous.
3 Matsa and Miller (Citation2011) find a positive association between the female share of the board of directors in the previous year and the female share among current top executives. See also Powell and Butterfield (Citation2002).
4 Even for political positions involving an election process, the candidates need to be nominated by the parties.
5 The binomial can be replaced by the hypergeometric distribution and the results hold.
6 The existence of a limit distribution and its statistical properties are studied in Ferreira and Stute (Citation2016) and Espinosa, Ferreira, and Stute (Citation2016).
7 Bagues and Esteve-Volart (Citation2010) provide evidence that the gender composition of recruiting committees matters for the female chances of success, for positions in the four main Corps of the Spanish Judiciary from 1987 to 2007.
8 This assumption does not fit, for example, decisions taken by majority; in this case the majority could impose their bias.
9 It could also be the case that influence on the decision is related to gender, but we do not model this gender asymmetry here.
10 See Lundberg and Startz (Citation1983) on discrimination and allocative efficiency.
References
- Arrow, K. J. (1972). Models of job discrimination. In A. H. Pasacl (Ed.), Racial discrimination in economic life. Lexington: Lexington Books.
- Arrow, K. J. (1973). The theory of discrimination. In O. Ashenfelter & A. Rees (Eds.), Discrimination in labor markets (pp. 3–33). Princeton, NJ: Princeton University Press.
- Arulampalam, W., Booth, A. L., & Bryan, M. L. (2007). Is there a glass ceiling over Europe? Exploring the gender pay gap across the wages distribution. Industrial and Labor Relations Review, 60(2), 163–186.
- Bagues, M. F., and B. Esteve-Volart (2010). Can Gender Parity Break theGlass Ceiling? Evidence from a Repeated Randomized Experiment. The Review of Economic Studies, 77, 1301-1328.
- Bain, O., & Cummings, W. (2000). Academe’s glass ceiling: Societal, professional-organizational, and institutional barriers to the career advancement of academic women. Comparative Education Review, 44(4), 493–514.
- Baxter, J., & Wright, E. O. (2000). The glass ceiling hypothesis: A comparative study of the United States, Sweden and Australia. Gender & Society, 14, 275–294.
- Becker, G. S. (1957). The economics of discrimination. Chicago: University of Chicago Press.
- Bertrand, M., Goldin, C., & Katz, L. (2010). Dynamics of the gender gap for young professionals in the financial and corporate sectors. American Economic Journal. Applied Economics, 2(3), 228–255.
- Coate, S., & Loury, G. C. (1993a). Will affirmative action policies eliminate negative stereotypes?. American Economic Review, 83(5), 1220–1240.
- Cotter, D. A., Hermsen, J. M., Ovadia, S., & Vanneman, R. (2001). The glass ceiling effect. Social Forces, 80(2), 655–681.
- Elliot, J. R., & Smith, R. A. (2004). Race, gender and workplace power. American Sociological Review, 69, 365–386.
- Espinosa, M. P., Ferreira, E., & Stute, W. (2016). Discrimination, binomials and glass ceiling effects. In Ricardo Cao, Gonzalez Manteiga, Wenceslao, and Romo, Juan, (Eds.). Nonparametric statistics (pp. 175). Switzerland: Springer Proceedings in Mathematics & Statistics.
- Espinosa, M. P., & Ferreira, E. (2017). Hierarchical organizations and glass ceiling effects. In From statistics to mathematical finance (pp. 429–440). Springer International Publishing AG: Springer.
- Espinosa, M. P., & Gardeazabal, J. (2020). The gender-bias effect of test scoring and framing: A concern for personnel selection and college admission. The B.E. Journal of Economic Analysis & Policy, 20(3), 20190316.
- Espinosa, M. P., & Kovarik, J. (2015). Prosocial behavior and gender. Frontiers in Behavioral Neuroscience, 9, 88.
- Federal Glass Ceiling Commission. (1995). Good for business: Making full use of the nation’s human resources. Washington, DC: U.S. Department of Labor.
- Ferreira, E., & Stute, W. (2016). Dynamic binomials with an application to gender bias analysis. Journal of Applied Probability, 53, 82–90.
- Fox, R. L., & Lawless, J. L. (2005). To run or not to run for office: Explaining nascent political ambition. American Journal of Political Science, 49, 642–659.
- Fox, R. L., & Lawless, J. L. (2011). Gendered perceptions and political Candidacies: A central barrier to women’s equality in electoral politics. American Journal of Political Science, 55(1), 59–73.
- Gardeazabal, J., & Ugidos, A. (2005). Gender wage discrimination at quantiles. Journal of Population Economics, 18(1), 165–179.
- Gjerde, K. A. P. (2002). The existence of gender-specific promotion standards in the U.S. Managerial and Decision Economics, 23(8), 447–459.
- Gneezy, U., Leonard, K., & List, J. A. (2009). Gender differences in competition: Evidence from a matrilineal and a patriarchal society. Econometrica, 77(5), 1637–1664.
- Gneezy, U., Niederle, M., & Rustichini, A. (2003). Performance in competitive environments: Gender differences. Quarterly Journal of Economics, 118, 1049–1074.
- Gneezy, U., & Rustichini, A. (2004). Gender and competition at a young age. American Economic Review Papers and Proceedings, 94, 377–381.
- Günther, C., Ekinci, N. A., Schwieren, C., & Strobel, M. (2010). Women can’t jump? An experiment on competitive attitudes and stereotype threat. Journal of Economic Behavior & Organization, 75(3), 395–401.
- Hernan-Gonzalez, R., & Kujal, P. (2015). Gender differences in cooperation and competition. In P. Branas-Garza, and A. Cabrales (Eds.), Experimental economics I: Economic decisions (pp. 154–168). London, UK: Palgrave Macmillan Ltd.
- Jackson, J. F., & O’Callaghan, E. M. (2009). What do we know about glass ceiling effects? A taxonomy and critical review to inform higher education research. Research in Higher Education, 50, 460–482.
- Karnig, A. K., & Welch, S. (1979). Sex and ethnic differences in municipal representation. Social Science Quarterly, 60(3), 465–481.
- Kleinberg, J., & Raghavan, M. (2019). Selection problems in the presence of implicit bias. Ithaca, NY: Cornell University mimeo.
- Lundberg, S. J., & Startz, R. (1983). Private discrimination and social intervention in Competitive labor markets. American Economic Review, 73(3), 340–347.
- Matsa, D. A., & Miller, A. R. (2011). Chipping away at the glass ceiling: Gender spillovers in corporate leadership. American Economic Review: Papers and Proceedings, 101(3), 635–639.
- Maume, D. J. (1999). Occupational segregation and the career mobility of white men and women. Social Forces, 77, 1433–1459.
- McDowell, J. M., Singell, L. D., & Ziliak, J. P. (1999). Cracks in the glass ceiling: Gender and promotion in the economics profession. The American Economic Review, 89(2), 392–396.
- Milgrom, P., & Oster, S. (1987). Job discrimination, market forces, and the invisibility hipothesis. Quarterly Journal of Economics, 102, 453–476.
- Morgan, L. A. (1998). Glass-ceiling effect or cohort effect? A longitudinal study of the gender earnings gap for engineers, 1982 to 1989. American Sociological Review, 63(4), 479–493.
- Niederle, M., & Vesterlund, L. (2007). Do women shy away from competition? Do men compete too much?. Quarterly Journal of Economics, 122, 1067–1101.
- Olson, C. A., & Becker, B. E. (1983). Sex discrimination in the promotion process. Industrial & Labor Relations Review, 36(4), 624–641.
- Phelps, E. (1972). The statistical theory of racism and sexism. American Economic Review, 62(4), 659–661.
- Powell, G. N., & Butterfield, A. (2002). Exploring the influence of decision makers’ race and gender on actual promotions to top management. Personnel Psychology, 55, 397–428.
- Purcell, D., MacArthur, K. R., & Samblanet, S. (2010). Gender and the glass ceiling at work. Sociology Compass, 4(9), 705–717.
- Sassler, S., Glass, J., Levitte, Y., & Michelmore, K. M. (2017). The missing women in STEM? Assessing gender differentials in the factors associated with transition to first jobs. Social Science Research, 63, 192–208.
- Studlar, D. T., & Welch, S. (1990). Multi-member districts and the representation of women: Evidence from Britain and the United States. Journal of Politics, 52(2), 391–412.
- Thévenon, O., Ali, N., Adema, W., & Salvi Del Pero, A. (2012). Effects of reducing gender gaps in education and labour force participation on economic growth in the OECD OECD Social, Employment and Migration Working Papers, No. 138, OECD Publishing, doi: 10.1787/5k8xb722w928-en.
- Welch, S. (1977). Women as political animals? A test of some explanations for male-female political participation differences. American Journal of Political Science, 21, 711–730.
- Zeng, Z. (2011). The myth of the glass ceiling: Evidence from a stock-flow analysis of authority attainment. Social Science Research, 40, 312–325.
Appendix
Proof of Theorem 1
Under Assumptions and
, from (1) the ratio of selected female members
can be represented as a discrete Markov process, where the conditional probabilities are determined by
For no degenerate cases (), the transition matrix
is homogeneous and regular, as all probabilities are strictly positive. Therefore, the chain is ergodic and there is a unique limit of
, which means, using standard Markov chain results, that
in distribution, where
has probability distribution
, being
the unique solution of
. To obtain the explicit expression for
write (1) as
. Then,
,
. Using this recursive relation and the geometric sum properties, the result for
follows.
To find the limit , it is sufficient to take the limit
, or use the fact that the stationary mean is the fixed point of
. ■
Proof of Corollary 1
Note that from . Set the function
. Since this function is zero at
and
,
takes on negative values when
, and positive values otherwise.
Consider , then
, since
.
Finally, using an induction argument, we have that if then
for all
, and
otherwise. ■
Proof of Proposition 1
Consider a fixed average gender bias , where
. From (3) we can write the long run expected female proportion as
Substituting , we get
As a function of ,
is an increasing function, and the maximum value is reached at
, i.e.,
■
Proof of Theorem 2
It is sufficient to check that the transition matrix with elements:
is homogeneous and regular. It is homogeneous since , where
does not depend on
. Note that
Regularity comes from the fact that all elements in are positive whenever
. ■
Proof of Theorem 3
(i) Assume there is and rewrite the odds ratio for the unconditional probabilities (
) as:
implies
. Thus,
. This inequality holds if and only if
(from (6))
, that is
is a decreasing sequence (
). Finally, this holds whenever
is decreasing; i.e., when there is glass ceiling of Type 1 (
).
(ii) From (6)
where denotes the odds ratio
, and there is
if and only if:
for all . ■
Proof of Proposition 3
(i) It is straightforward since
(ii) To check whether there is , we have to check that the ratio
is decreasing in
:
Rewriting as a function of
and
:
Since (there is
),
will be decreasing with level
if and only if
is an increasing function of
; that is, when
decreases (up the corporate ladder)
also decreases. Taking the first derivative in the expression for
:
Thus,
Therefore, there is if and only if
■
Proof of Theorem 4
1) From (8)
since , which holds since
.
For , we check Condition (7):
On the other hand,
Then, we have that Condition (7) is equivalent to
2) From (9)
since . Therefore, there is
and
.
For , we check Condition (7):
which holds since
■