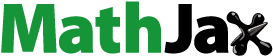
ABSTRACT
Logistics is an essential service for manufacturing because it supports the coordination and integration of global production activities, ultimately reducing costs and improving efficiency. However, it is not clear that the complex structural relationship between logistics and manufacturing under the Global Value chains (GVCs). Consequently, employing the input-output model, we develop the logistics input complexity (LIC) to capture the complex structural characteristics of logistics services embodied in manufacturing sectors and investigate its role in manufacturing comparative advantage. We find that the logistics input complexity can boost manufacturing competitiveness. Furthermore, we also confirm that the domestic and foreign groups of LIC have at least a partially complementary effect on the export competitiveness of manufacturing. Finally, we identify heterogeneity in the domestic and foreign groups’ five subdivision logistics modes of LIC. It suggests that logistics service providers must precisely match the related manufacturing production chains to cultivate a comparative advantage in manufacturing.
1. Introduction
Comparative advantage in manufacturing is pivotal to a nation’s economic growth and its quest for a competitive edge in the global market. As highlighted by Cassini (Citation2023), it is particularly instrumental in developing a country’s production capacity and establishing a robust National Innovation System (NIS). In the face of economic globalization, understanding the dynamics that drive this advantage is essential for nations aiming to achieve and sustain economic prosperity through their manufacturing prowess.
While mainstream research has extensively analyzed the formation of comparative advantage across countries and industries (Chor, Citation2010), the pivotal role of logistics in bolstering manufacturing competitiveness has been largely neglected. Within the framework of Global Value Chains (GVCs), logistics not only serves as a critical infrastructure but also actively enhances manufacturing’s global standing through coordinated factor inputs and expanded market access, as posited by Fawcett and Closs (Citation1993). By streamlining the movement of production factors and facilitating product distribution, logistics significantly reduces manufacturing costs and enhances market competitiveness. This underscores the indispensable nature of logistics in the modern manufacturing landscape, where it is no longer just a support function but a key driver of efficiency and cost reduction.
Industry-level databases have enabled research on the service-manufacturing relationship within Global Value Chains (GVCs) (Bamieh et al., Citation2020; Falk & Peng, Citation2013; Hoekman & Shepherd, Citation2015; Liu et al., Citation2020), yet the impact of logistics on the manufacturing industry’s competitiveness has been understudied. While S. Park (Citation2020) and Yang et al. (Citation2022) introduced the concept of logistics input intensity, highlighting logistics as a significant input for manufacturing, their work and others have not fully explored the structural intricacies of logistics within GVCs. The complex organization of global production processes, as described by Baldwin and Venables (Citation2013), which includes both sequential (“snakes”) and modular (“spiders”) structures, demands sophisticated logistics and transportation expertise. This is essential for achieving the geographical and functional integration necessary to navigate the spatial and functional fragmentation of Global Production Networks (GPNs), as noted by Hesse and Rodrigue (Citation2006) and Rodrigue (Citation2006). We argue that the complex structure of logistics services is a critical, yet often overlooked, factor contributing to the comparative advantage of manufacturing industries.
Consequently, this paper attempts to bridge the gap in the previous literature about S. Park (Citation2020) and Yang et al. (Citation2022) by investigating the intricate structural relationship between logistics and manufacturing in the global value chains. Specifically, we first develop the logistics input complexity (LIC) to measure the complex structural level for logistics services embodied in the manufacturing sectors along GVCs. This indicator is defined as the value-added propagation length of logistics sectors in all countries for each manufacturing sector, which is derived from the calculation of the value-added propagation length from a group of value-added creators(industries) to a specific final product proposed by Meng et al. (Citation2020). We then easily explore the role of LIC in the export competitiveness of manufacturing. Furthermore, we also profoundly investigate the effect of the domestic and foreign groups and the five logistics services modes of LIC on comparative advantage in manufacturing by dividing the LIC into the two groups of domestic and foreign or the five subdivision logistics services modes.
The contributions of this study are several as follows. First, combining the theory of GVCs and the input-output model, we develop an indicator – LIC – to measure the complex structural characteristics of logistics services embodied in the manufacturing sectors under GVCs. Second, we identify and confirm the effect of the LIC on comparative advantage in manufacturing using a multidimensional fixed-effect model. Third, after dividing the LIC into the two groups of domestic and foreign or the five subdivision logistics services modes, we deeply investigate the effect of the domestic and foreign groups and the five logistics services modes of LIC on manufacturing’s comparative advantage.
The structure of this paper is outlined as follows: Section 2 offers a comprehensive literature review that establishes the theoretical foundation and context for our study. Section 3 details the measurement of variables and the sources of data utilized in our analysis. Section 4 elaborates on the econometric model and the empirical strategy employed to test our hypotheses. Section 5 showcases the empirical results derived from our analysis. Section 6 provides an in-depth discussion of the results, exploring their implications and relevance to the field. Finally, Section 7 presents the conclusions drawn from the study, along with recommendations for future research.
2. Literature review
2.1. The sources of comparative advantage in manufacturing
Comparative advantage, a central concept in international trade theory, has been an important research topic for many years. The conventional theory and empirical have explored various determinants contributing to a country’s comparative advantage, including productivity, factor endowments, and institutions (Chor, Citation2010).
Productivity differences have long been recognised as a fundamental source of comparative advantage, pivotal in shaping international trade patterns. Based on the Ricardian framework, Eaton and Kortum (Citation2002) introduced a parameterisation that accurately captures the distribution of productivity levels and addresses multi-country contexts. Consequently, several scholars have quantitatively validated the Ricardian model’s predictions (Choi & Park, Citation2018; Costinot & Donaldson, Citation2012; Costinot & Komunjer, Citation2007; Costinot & Vogel, Citation2015; Costinot et al., Citation2012; Deardorff, Citation2005; Levchenko & Zhang, Citation2014). Moreover, other researchers recently have delved into the determinants of productivity disparities among countries, such as country size and technology (Ara, Citation2020), innovation and production (Somale, Citation2021), technological R&D efficiency and firm-level technology investments (Sampson, Citation2023), knowledge diffusion (Bahar et al., Citation2014; Cai et al., Citation2022), capital heterogeneity (Ishise, Citation2016). These studies have provided a multifaceted view of factors contributing to a nation’s comparative advantage in the global marketplace.
Factor endowments theory, initially proposed by the Heckscher-Ohlin model and expanded into the HOV model by Vanek (Citation1968), has faced challenges like Leontief’s paradox (Leontief, Citation1953), catalysing a wave of empirical studies aimed at testing the theory’s validity. For instance, Debaere (Citation2003) and Romalis (Citation2004) found evidence that supports the factor endowments theory. Trefler (Citation1993, Citation1995) suggested incorporating technology and country-specific productivity differences to refine the HOV model. Many scholars have dug deeper into the sources of technological differences from different perspectives based on the HOV model (Burstein & Vogel, Citation2017; Morrow & Trefler, Citation2017, Citation2022; Navas, Citation2018; Nishioka, Citation2012). Others have refined the factor endowment theory by incorporating the analysis of intermediate traded goods or value-added exports (Brondino, Citation2023; Ito et al., Citation2017; Koch & Fessler, Citation2020; Nishioka, Citation2013), as well as by examining digital tasks (Stöllinger & Guarascio, Citation2023) and knowledge capital (Chen & Shao, Citation2020).
Finally, some studies emphasise the role of institutions and other factors in shaping comparative advantage, including financial development (Beck, Citation2003; Gong & Zhou, Citation2014; Gur & Avşar, Citation2016; Ju & Wei, Citation2011; Keuschnigg & Kogler, Citation2022; Manova, Citation2008), contract enforcement (Costinot, Citation2009; Essaji & Fujiwara, Citation2012; Feenstra et al., Citation2013; Ferguson & Formai, Citation2013; Levchenko, Citation2007; Ma et al., Citation2010; Nunn, Citation2007; S. M. Park, Citation2023), labor market flexibility (Cuñat & Melitz, Citation2012), and demographic structure (Cai & Stoyanov, Citation2016), alongside other factors like large firms (Gaubert & Itskhoki, Citation2021), multinational production (Alviarez, Citation2019), and economic complexity (Ghasemkhani et al., Citation2022a, Citation2022b; Krishna & Levchenko, Citation2013; Y. Wang & Turkina, Citation2020).
However, recent work by Hausmann et al. (Citation2022) has significantly advanced our understanding of the dynamics of comparative advantage. Building upon the foundational research of Hausmann and Klinger (Citation2006, Citation2007), Hidalgo et al. (Citation2007), and Bahar et al. (Citation2014), their study innovatively combines theoretical modelling with empirical analysis to demonstrates how the relatedness of inter-industry and inter-location can effectively predict shifts in comparative advantage. They challenge the assumption of a common national productivity parameter in the Ricardian models of trade (Costinot et al., Citation2012; Eaton & Kortum, Citation2002), integrating the factor requirements of industries with the factor endowments of locations to provide a comprehensive estimation of comparative advantage.
Our study draws on the insight of Hausmann et al. (Citation2022) and focuses on the complex structural relationship of the logistics industry embedded within the manufacturing sector along the Global Value Chains (GVCs), which may be one of the key factors shaping the comparative advantage in manufacturing.
2.2. The role of logistics in export competitiveness of manufacturing
As the Logistics Performance Index (LPI) proposed by Arvis et al. (Citation2007) is a multidimensional and comprehensive quantitative tool for logistics performance at the national level, many scholars empirically study the relationship between logistics performance and global economics or trade. They have confirmed that high-level logistics performance can significantly boost a country’s trade volume from different perspectives (Bottasso et al., Citation2018; Bugarčić et al., Citation2020; Çelebi, Citation2019; Gani, Citation2017; Kabak et al., Citation2018; Korinek & Sourdin, Citation2011; Mendes dos Reis et al., Citation2020; Saslavsky & Shepherd, Citation2014; Töngür et al., Citation2020).
However, few studies pay attention to the role of logistics in the comparative advantage of manufacturing at the industry level. Most researchers have examined the relationship between services and manufacturing as the emergence of servitisation. They developed the service intensity or service input intensity (SII) to measure the degree of service input for manufacturing (Falk & Peng, Citation2013; Hirsch, Citation1989) and considered it as a key moderating variable to analyse the effect of services on manufacturing (Bamieh et al., Citation2020; Liu et al., Citation2020). To our knowledge, S. Park (Citation2020) was the first to empirically confirm that logistics and transport infrastructure quality is a source of comparative advantage in logistics-intensive industries. As the follower, Yang et al. (Citation2022) argued that national-level logistics performance could promote comparative advantage in manufacturing sectors with a higher usage intensity of logistics, especially in developing countries. In sum, they both proposed the logistics input intensity, originated from the idea that logistics is a particular input factor of manufacturing industries, and introduced it into the model to investigate the effect of logistics on comparative advantage in manufacturing. However, the above studies merely reflect the quantitative proportion characteristic of value-added contribution from logistics services to manufacturing’s comparative advantage, ignoring the complex structural characteristics of logistics services embodied in manufacturing under the global value chains, which may be another critical dimension of the contribution of logistics services to manufacturing comparative advantage.
2.3. The logistics input complexity for manufacturing based on GVCs perspective
Global Value Chains (GVCs) have transformed global production into intricate networks where countries specialise in specific production stages, leading to complex coordination challenges that require advanced logistics and transportation systems for integration (Hesse & Rodrigue, Citation2006; Hummels et al., Citation2001; Rodrigue, Citation2006). Logistics, or supply chain management (SCM), is a critical mechanism supporting global manufacturing strategies of factor input and market access (Fawcett & Closs, Citation1993).
Researchers have utilised input-output databases to analyse GVCs along two dimensions: degree (value added) and position (length). A robust literature exists on measuring the degree of value added in GVCs (Ceglowski, Citation2017; Johnson & Noguera, Citation2012; Kee & Tang, Citation2016; Koopman et al., Citation2014; Los et al., Citation2015; Meng et al., Citation2017; Pahl & Timmer, Citation2020; Stehrer, Citation2012; Timmer et al., Citation2014; Z. Wang et al., Citation2013, Citation2017a; Zhang et al., Citation2018), with Johnson (Citation2018) providing a comprehensive summary and outlook.
However, these measures do not fully capture the complexity and geometry of GVCs, which stem from the intricacies of production networks. Studies have thus focused on the position of linkages within GVCs, using metrics like average propagation length (APL) to gauge the sequencing of global production chains (Dietzenbacher & Romero, Citation2007; Dietzenbacher et al., Citation2005; Fally, Citation2012). Other measures assess the “upstreamness” of industries (Antràs & Chor, Citation2018; Antràs et al., Citation2012; Miller & Temurshoev, Citation2017) and employed a combination of firm-level data, manufacturing survey data, and industry-based measures of upstreamness to exhibit the positioning of firms within global production networks and how this evolves with productivity and performance throughout the firm’s life-cycle (Chor et al., Citation2021).
Nevertheless, these measures start from a sector’s gross output rather than primary inputs (or value-added) such as labour and capital because the gross outputs are endogenous variables; primary inputs and final demand are exogenous variables in the standard Leontief model (Z. Wang et al., Citation2017a).Footnote1 Consequently, Z. Wang et al. (Citation2017a) define the average length of production as the average number of times the value added created by the production factors in the sequential production process is computed as total output (induced by value-added). With the deepening of research, Meng et al. (Citation2020) introduce the average value added propagation length (focusing on the “value-added” propagation process in GVCs), offering a unified framework for measuring the distance between producers and consumers in GPNs.
To capture the complexity of logistics service embodied in manufacturing, we prefer to employ the group-wise value-added propagation length from the particular group of final products to the specific group of industries proposed by Meng et al. (Citation2020).
3. Measure of variables and data sources
3.1. Measure of critical variables
3.1.1. Revealed comparative advantage (RCA) based on VAX
With the increasing segmentation of global production worldwide, each economy specialises in particular production tasks in complex global production networks (GPNs) rather than final products in traditional trade (Hummels et al., Citation2001). This significant change in international trade patterns has resulted in the misleading of the traditional statistical framework under GVCs, neglecting that the competitiveness of an economy in international trade has shifted from gross exports based on export products to value added in particular production tasks (Johnson & Noguera, Citation2012). Specifically, the value-added embodied in trade has increasingly accorded for more intermediate goods or services than final goods (Bohn et al., Citation2018; Markusen, Citation1989).
Consequently, given the above situation, a new statistical framework is essential for measuring the comparative advantage since most economic activities tend to trade within the value chain (Miroudot & Cadestin, Citation2017). For the dependent variable – manufacturing comparative advantage, this paper uses the revealed comparative advantage(RCA) based on value-added export proposed by (Koopman et al., Citation2014), and the specific calculation formula is as follows:
3.1.2. Logistics input complexity (LIC)
This section defines the logistics input complexity for final manufacturing sectors. Based on the calculation of the value-added propagation length from a group of value-added creators(industries) to a specific final product proposed by Meng et al. (Citation2020), we first develop the logistics input complexity (LIC) for all countries, which denote as the value-added propagation length of logistics sectors in all countries for each manufacturing sector. The specific formula is as follows:
To deeply investigate the effect of LIC on manufacturing RCA, we divide the LIC into the domestic and foreign groups and estimate separately the domestic and foreign LIC in country to each manufacturing sector as follows:
Where and
denote the specific country
and the logistics sectors, respectively. The
in formula (2) measures the aggregate of logistics input complexity in all countries to each manufacturing sector, while the
and
in formulas (3) and (4) measure the domestic and foreign groups of logistics input complexity in country
to each manufacturing sector in country
. The
denotes a
Footnote2 direct value-added coefficient vector of logistics sectors in all countries (with values for other sectors being 0). While the
and
denote the
domestic and foreign direct value-added coefficient vector of logistics sectors in country.Footnote3 A is the
input coefficient matrix (the share of intermediate input in output). B refers to the
Leontief inverse matrix.
refers to the
diagonal matrix of the final demand of manufacturing sectors in all countries.
denotes a
vector of the value-added contribution of logistics sectors in all countries to each manufacturing sector. While
and
denote the
vector of the domestic and foreign value-added contribution of logistics sectors in the country
to each manufacturing sector in the country
. The numerator on the right of the formula (2) represents the total output of the logistics sectors in all countries to each manufacturing sector. In comparison, the denominator represents the value-added contribution of the logistics sectors in all countries to each manufacturing sector.
3.2. Data sources and control variables
We construct our sample from two sources: the World Input-Output Database (2016 version) (WIOD) and World Development Indicators (WDI), including panel data at the country-sector-year level, with 10,095 observations covering 43 countries, 18 manufacturing sectors (WIOD sectors 5–22), and five logistics sectors (WIOD sectors 31–35) for 2000–2014.Footnote4
We incorporate a series of control variables based on Trade or Comparative advantage Theory from previous literature to account for some factors affecting manufacturing comparative advantage. Firstly, we chose the three variables: (1) GDP per capita (GDP/capita), (2) Gross Output (GO), and (3) Employment size (Emp), representing the development level of the country and the scales of industry separately (Gouma et al., Citation2018; Liu et al., Citation2020); Secondly, we prefer the two variables: (4) Sector productivity (Prod) and (5) Sector capital-labour ratio (KLR), representing separately the Ricardian productivity and Heckscher-Ohlin’s factor endowments (Chor, Citation2010; Gouma et al., Citation2018; Liu et al., Citation2020); Finally, we also take (6) GVC participation level (Wang et al., Citation2017b) into account for our control variable because our dependent variable is the value-added comparative advantage. Specifically, their definitions are presented in below.
Table 1. The definition and source of control variables.
4. Econometric model and empirical strategy
We first construct a measure of logistics input complexity based on the method proposed by Meng et al. (Citation2020) by calculating the value-added propagation length from logistics to manufacturing sectors. We then employ revealed comparative advantage(RCA) based on valued-added exports(VAX) proposed by Koopman et al. (Citation2014) as the dependent variable and build the econometric models to confirm the effect of logistics input complexity on comparative advantage in manufacturing. Finally, we deeply investigate the effect of the domestic and foreign groups and the five logistics services modes of LIC on manufacturing RCA by dividing the LIC into the two groups of domestic and foreign or the five subdivision logistics services modes.
To estimate the effect of the aggregate of LIC in all countries on manufacturing RCA, the benchmark specification of the econometric model is as follows:
To deeply investigate the above effect, we divided the LIC into domestic and foreign groups; the specifications of the econometric models are as follows:
where ,
, and
represent the country, sector, and year, respectively; the dependent variable
denotes the revealed comparative advantage based on value-added export of country
in sector
in
year; the
and
measure the aggregate of LIC in all countries, the domestic and foreign LIC of country
in sector
in
year, respectively.
is the control variable vector;
stands for fixed effect (including three dimensions of country, sector, and year);
is the error term. According to the above empirical strategy, we focus on the coefficients of
in formula (5) and
and
in formulas (6) and (7), respectively.
,
and
are all expected to be positive.
The main problem of our econometric models is the endogeneity caused by omitted variables and reverse causality. The former issue is that some common shocks may exist in unobserved invariant-time industry characteristics and varying-time macro fluctuation factors affecting manufacturing RCA and LIC. The latter issue is that there may be the probability that the manufacturing industries in a particular country with higher RCA might have a complex production chain which attracts complex logistics services.
On the one hand, to address the omitted variables problems, we add some fixed effects, including the country, sector and year, in our models to control the unobserved characteristics at the country and industry level and macro fluctuation factors at the year level. On the other hand, to deal with the reverse causality problems, we employ the lagged period of the LIC as an instrumental variable and adopt the two-stage least squares (2SLS) method to revise the estimation of the benchmark regression.
To strengthen our benchmark regression’s robustness, we also add some robustness specifications, including alternative variable and Logit regression models, sample adjustments by excluding developed countries, and Panel quantile regression.
5. Empirical results
5.1. Benchmark regression
presents results for the effect of logistics input complexity on comparative advantage in manufacturing. The dependent variable is the manufacturing RCA based on forward-linkage VAX. The LIC is the ratio of the total output of the logistics sectors embodied in the final demand of the manufacturing sectors to the value-added contribution of the logistics sectors to each manufacturing sector. There may be the issue of unobserved omitted variables even if we add some control variables into our models. As a result, we have set a string of fixed effects for all columns to address the endogeneity problem caused by the above issue. Specifically, as follows: column (1) sets the fixed effect of the sector to control the influence of invariant-time sector characteristics, column (2) and (4) set the fixed effects of the sector and year to control the influence of invariant-time sector characteristics and varying-time macro fluctuation factors on the dependent variables. Columns (3) and (5) set the fixed effects of the interaction between sector and year to control the comprehensive characteristics of the invariant-time sector and varying-time macro fluctuation factors on the dependent variables. The standard errors are robust to heteroscedasticity and clustered by sector and year in columns (1), (2), (3), and sector*year in columns (4) and (5) to solve the potential sequence and correlation in the error terms over time for a specific sector.
Table 2. The effect of the LIC on manufacturing RCA (benchmark regressions).
Generally, we can see the LIC are always significantly positive at the 10% level in column (1)-(3) and at the 1% level in column (4)-(5). It indicates that LIC always positively affects RCA in manufacturing. The effect is even strengthener at the sector*year robust standard errors cluster than the sector and year cluster.
The results in also show that the significant control variables have the expected signs. Specifically, apart from log(GO) and GDP/capita, employment size, Sector productivity, Sector capital-labour ratio (KLR), and GVC participation level increase a country’s comparative advantage in manufacturing value-added exports. Their effects are primarily significant at the 1% level.
5.2. Robustness tests
5.2.1. Instrumental variables method for the endogeneity problem
Although the endogeneity problem due to omitted variables has been mitigated previously through various fixed effects models, there may also be endogeneity problems due to reverse causation in our econometric models. Manufacturing industries in certain countries with more export competitiveness may affect logistics input complexity inversely. To mitigate this type of endogeneity problem, we use the lagged period of the LIC as an instrumental variable and adopt the two-stage least squares (2SLS) method. presents the results of the 2SLS regression for the instrumental variable (the lagged period of LIC). The results show the two critical points for the instrumental variable method: Firstly, our instrumental variable is reasonable. The F value is greater than 10, and the statistical test values of K-P LM and C-D LM are greater than the 10% threshold for the Stock-Yogo instrumental variable test. In addition, the regression coefficient of the lagged period of the LIC for the LIC is positively significant at the 1% level at the first stage of regression. Secondly, the instrumental variable regression results are consistent with the benchmark regression results because the regression coefficient of the LIC for the RCA_manu is positively significant at the 10% level at the second regression stage.
Table 3. Robustness check (1) (Instrumental variables 2SLS).
5.2.2. Alternative variable and Logit regression models
Following some approaches in the literature on the evolution of comparative advantage, we also transform the dependent variable – manufacturing RCA – to the 0–1 binary variable and adopt the logit regression models to examine whether the LIC affect the structural change of manufacturing RCA. The fixed effects and control variables are consistent with the benchmark regression. The results from show that the LIC regression coefficients for the RCA_bi Footnote5 are all positively significant at the 1% level in all columns (different fixed effects). It indicates that LIC also significantly affects the structural change of manufacturing RCA and supports our robustness of benchmark regression.
Table 4. Robustness check (2) (an alternative measure of RCA and Logit regression models).
5.2.3. Sample change – dropping the sample of developed countries
Our sample includes both developing and developed countries. Previous research on the “smile curve” in GVCs (Aggarwal, Citation2017; Meng et al., Citation2020) has shown that the industrial structure in international trade varies between the two groups. Developed countries primarily emphasise exports in service sectors, which rely heavily on innovation (such as R&D, design, brand building, after-sales service, and marketing). These countries have progressed beyond the initial stages of industrial development and are typically positioned at the forefront and tail end of GVCs, specialising in high-value-added processes. On the other hand, developing countries tend to focus on exports in manufacturing sectors, leveraging their labour or resource advantages within the global product system of GVCs. They are usually situated in the middle of GVCs and specialise in low-value-added processes.
Consequently, to eliminate the influence of service sectors and maintain the focus on manufacturing sectors, we have excluded the sample of developed countries for our robustness analysis. presents the robustness analysis outcomes for excluding developed countries from the sample, using the benchmark regression as a reference. The results demonstrate that the regression coefficients for LIC for RCA_manu are all significantly positive at the 1% level across all columns and larger than those obtained in the benchmark regression. It suggests that the impact of LIC on manufacturing RCA is amplified when only developing countries are included in the sample, which is consistent with our benchmark regression findings.
Table 5. Robustness check (3) (sample change – dropping the sample of developed countries).
5.2.4. Panel quantile regression
In this specification, we further enhance the robustness of our findings by employing panel quantile regression analysis, which allows us to investigate the impact of the independent variables on different segments of the conditional distribution of the dependent variable while accounting for the panel structure of the data, capturing both cross-sectional and time-series dimensions. This approach complements our primary regression models by providing insights into how the relationship between the variables varies across different quantiles of the outcome variable. Specifically, we focus on the 25th, 50th (median), and 75th quantiles to capture the effects on the distribution’s lower, middle, and upper parts.
The panel quantile regression results, presented in , reveal that the coefficients for our key independent variable, Logistics Input Complexity (LIC), remain positive and significant across all quantiles, indicating a consistent positive association with the Revealed Comparative Advantage (RCA) in manufacturing. Notably, the magnitude of the coefficients suggests that the impact of LIC on RCA is more pronounced in the middle and upper quantiles, which may imply that the benefits of complex logistics services are more markedly realised among higher-performing manufacturing sectors. This finding underscores the importance of logistics in enhancing the competitiveness of manufacturing industries, particularly for those that are more advanced and competitive in the global market.
Table 6. Robustness check (4) (Panel quantile regression).
The consistency of the panel quantile regression results with our benchmark regression analysis provides additional evidence of the robustness of our findings. It suggests that the positive relationship between LIC and RCA is not confined to a specific segment of the manufacturing sector but is pervasive across various levels of competitiveness. This comprehensive analysis, which accounts for potential heterogeneity in the data, further validates our conclusions regarding the strategic role of logistics in fostering manufacturing competitiveness.
5.3. The domestic and foreign of LIC
As the LIC contain the aggregation output accumulated of logistic services in all countries, we can divide the LIC into domestic and foreign groups to profoundly investigate the effect of the two groups of LIC on manufacturing RCA. Consequently, the domestic and foreign LIC contain the unobserved national characteristics that may affect the manufacturing RCA. In this section, we add the country-fixed effect based on the sector and year of fixed effects to control the national characteristics embodied in the domestic and foreign LIC. presents the results for the regression of the domestic and foreign LIC for the manufacturing RCA. The results show that the coefficients of the domestic and foreign LIC are all significantly positive at the 5% level. Specifically, we can also see that the coefficients of domestic LIC are more significant than those of foreign LIC. The differences between domestic and foreign groups indicate that the comparative advantage of manufacturing industries in various countries can benefit from domestic and foreign LIC but mainly depend on the domestic LIC.
Table 7. The effect of the domestic and foreign LIC on manufacturing RCA.
Furthermore, we investigate the interaction effect of domestic and foreign logistics input complexity on comparative advantage in manufacturing. From the results in , the coefficients of the interaction term of the domestic and foreign LIC are always significantly positive at above 5%. These results suggest that the effect of domestic logistics input complexity on comparative advantage in manufacturing will increase with the improvements in foreign logistics input complexity. It means that at least a partially complementary effect exists for foreign and domestic logistics input complexity on the manufacturing RCA.
Table 8. The interaction effect of domestic and foreign LIC.
5.4. Different logistics service modes for the LIC
In this section, we deeply investigate the effect of LIC under different logistics service modes on comparative advantage in manufacturing. As the index of logistics sectors in the input-output tables (WIOTs) of WIOD is 31 to 35, we can divide the LIC into five different subdivision modes, including land transport, water transport, air transport, warehousing, and postal and courier. Based on the analyses for the effect of the domestic and foreign LIC on manufacturing RCA in the 5.3 section, we separately estimate the impact of the five subdivision modes of LIC in the domestic and foreign groups, whose results are presented in and , respectively. We controlled for the influence of invariant-time country and sector characteristics and varying-time macro fluctuation factors on the dependent variables through fixed effects for country, sector, and year in both groups of five logistic services modes regressions.
Table 9. The effect of LIC for five domestic logistics modes on manufacturing RCA.
Table 10. The effect of LIC for five foreign logistics modes on manufacturing RCA.
The results in show that the coefficients of the five different subdivision modes of LIC (including domestic and foreign groups) in all columns except those of column (2) in are significantly positive at above 5%. It suggests that the effects of the four logistics service modes in both groups, except for domestic water transport, are considerable. The results give us some surprise, but we believe that it is reasonable after profoundly thinking about the characteristics of the domestic water transport in the production chain, which is different from other logistics services modes in two groups. Specifically, domestic water transport serves bulk cargoes, mainly in the production chain of simple manufacturing products, compared to other logistics service modes. Hence, the effect of the input complexity of the domestic water transport for the production chain of manufacturing exports is not considerable. In addition, we find the coefficients of the domestic group more significant than the foreign group, indicating that each country’s manufacturing export competitiveness mainly depends on the domestic logistics input complexity.
6. Discussion
Our study advances the understanding of logistics’ role in manufacturing competitiveness within Global Value Chains (GVCs) by introducing the Logistics Input Complexity (LIC), which captures the intricate structural characteristics of logistics services within manufacturing sectors. This novel metric extends the work of S. Park (Citation2020) and Yang et al. (Citation2022), who previously introduced the concept of Logistics Input Intensity (LII). While LII focuses on the quantitative aspect of logistics inputs, our LIC measure delves deeper into the qualitative complexity of these inputs, providing a more nuanced understanding of their role in manufacturing competitiveness.
Our findings complement the work of Antràs et al. (Citation2012) and Fally (Citation2012), who have emphasised the importance of production staging and the length of production chains in global manufacturing. Our research adds to this by quantifying the complexity of logistics inputs and demonstrating its impact on manufacturing RCA, offering new insights into the strategic importance of logistics in the global production network.
The novelty of our work lies in developing the LIC measure and applying an input-output model to analyse the role of logistics services in manufacturing competitiveness within the GVC framework. Our findings challenge the traditional view of logistics as a simple input factor and position it as a complex, multifaceted service that significantly influences manufacturing’s ability to compete in the global market.
Our research on logistics input complexity (LIC) and its impact on manufacturing comparative advantage has implications for firm-level decision-making and policy formulation. Firms can optimise their supply chain strategies by aligning with the complexity of logistics services, making informed investment decisions in the context of GVCs, and innovating in services development to leverage logistics as a comparative advantage. Policymakers can inform infrastructure development, shape trade policies, and reform regulatory environments to enhance the efficiency and competitiveness of logistics services, thereby supporting the manufacturing sector.
7. Conclusions
Our study affirms the pivotal role of Logistics Input Complexity (LIC) as a determinant of comparative advantage in the manufacturing sector. By examining the intricate structural characteristics of logistics services within manufacturing, we have revealed how these complexities can significantly bolster a country’s competitive stance in the global market.
Drawing from our empirical analysis, we present the following conclusions:
(1) The Complexity of Logistics Services: Policymakers are encouraged to recognize the complexity of logistics services as a key differentiator in manufacturing competitiveness. Our findings indicate that sophisticated logistics services can enhance the comparative advantage of the manufacturing sectors.
(2) Complementarity Between Domestic and Foreign Logistics Services: We discovered a complementary effect between domestic and foreign components of LIC on manufacturing competitiveness. This suggests that a cohesive strategy leveraging both domestic and international logistics capabilities can lead to a synergistic enhancement of a country’s manufacturing advantage.
(3) Heterogeneity in Logistics Service Modes: The subdivision of LIC into various modes revealed that, except for domestic water transport, all other logistics modes significantly contribute to comparative advantage. This highlights the need for tailored approaches that acknowledge the distinct functional attributes of different logistics service modes.
Based on these conclusions, we propose the following policy recommendations:
(1) Integration of Logistics and Manufacturing: Policymakers should foster deeper integration between logistics and manufacturing sectors to develop production service chains that can elevate overall competitiveness.
(2) Synergy in Domestic and Foreign Logistics Services: Policies should be designed to create synergy between domestic and foreign logistics services, aiming to enhance the global competitiveness of the manufacturing sector.
(3) Precision Matching of Logistics Modes: It is crucial to identify the functional characteristics of different logistics service modes and match them accurately with various manufacturing production chains to optimize resource allocation and production efficiency.
However, the study’s limitations, particularly the lack of firm-level data, prevent a detailed analysis of logistics’ functional tasks across the manufacturing supply chain. Future research should consider adopting a logistics service chain perspective and integrating complex network analysis to measure and characterise the complexity of logistics services within manufacturing sectors. This approach would offer a more nuanced understanding of logistics’ role in the “smiling curve” of value chains, identifying key stages where logistics complexity contributes most to manufacturing competitiveness and providing targeted insights for logistics service providers to optimise operations and enhance the comparative advantage of manufacturing industries.
Credit authorship contribution statement
Lei Yang: Conceptualization, Data curation, Methodology, Investigation, Formal analysis, Software, Writing – original draft, Writing – review & editing, Funding acquisition.
Qianli Dong: Supervision, Funding acquisition, Project acquisition and administration.
Ziqiang Tong: Data curation, Formal analysis, Writing – review & editing.
Jiani Wu: Writing – review & editing, Funding acquisition.
Hua Yao: Writing – review & editing.
0624_Appendix_AB_cleann.docx
Download MS Word (18.3 KB)Acknowledgments
The authors would like to thank Professor Liangliang Zhang for the valuable feedback on earlier versions of the paper.
Disclosure statement
No potential conflict of interest was reported by the author(s).
Data availability statement
Our datasets from the WIOD and WDI can be accessed via the following websites (http://www.wiod.org/) and (https://databank.worldbank.org/data/source/world-development-indicators), respectively.
Supplementary Material
Supplemental data for this article can be accessed online at https://doi.org/10.1080/15140326.2024.2376958
Additional information
Funding
Notes on contributors
Lei Yang
Lei Yang is an associate professor in the College of Transportation Engineering at Chang’an University, China. His research interests include applied econometrics, logistics economics, sustainable development, and international trade. His works appear in journals including The Journal of International Trade & Economic Development, Environment, Development and Sustainability, Journal of Simulation, PLoS ONE, etc.
Qianli Dong
Qianli Dong is a professor in the School of Economics and Management at Chang’an University, China. His research interests include supply chain, green logistics, the relationship between logistics and manufacturing, and sustainable development. His works appear in journals including The Journal of International Trade & Economic Development, Sustainability, Environmental Science and Pollution Research, Environmental Technology & Innovation, etc.
Ziqiang Tong
Ziqiang Tong is a lecturer in the School of Economics and Management at Shanxi University, China. His research interests include applied econometrics, Digital transformation, financial performance, and sustainable development. His works appear in journals including Environment, Development and Sustainability, The Journal of International Trade & Economic Development, Journal of Simulation, etc.
Jiani Wu
Jiani Wu is an associate professor in the School of Economics and Management at Chang’an University, China. Her research interests include sustainable logistics, supply chain management, and applied econometrics. Her works appear in journals including Journal of Manufacturing Technology Management, Journal of Clearner Production, Logistics Research, The Journal of International Trade & Economic Development, Environmental Technology & Innovation, etc.
Hua Yao
Hua Yao is a PhD in the School of Transportation at Southeast University, China. Her research interests include macroeconomics, applied econometrics, logistics network, and sustainable development.
Notes
1 Consequently, the critical contribution of the gross trade accounting framework proposed by Koopman et al. (Citation2014) and Z. Wang et al. (Citation2013) is converting the gross output into final demand.
2 G and N are the numbers of countries and sectors in the sample, respectively.
3 the former with values for other sectors except for logistics sectors in country and all sectors in other countries being 0, while the latter with values for all sectors in country
and other sectors except logistics sectors in other countries being 0.
4 A detailed list of countries and codes covered in this paper is presented in Appendix A. A detailed list of the manufacturing and logistics sectors covered in this paper is presented in Appendix B.
5 it is a 0–1 binary variable transformed from the dependent variable manufacturing RCA.
References
- Aggarwal, S. (2017). Smile curve and its linkages with global value chains. Journal of Economics Bibliography, 4(3), Article 3. https://doi.org/10.1453/jeb.v4i3.1434
- Alviarez, V. (2019). Multinational production and comparative advantage. Journal of International Economics, 119, 1–26. https://doi.org/10.1016/j.jinteco.2019.03.004
- Antràs, P., & Chor, D. (2018). On the measurement of upstreamness and downstreamness in global value chains. NBER WORKING PAPER, No. 24185.
- Antràs, P., Chor, D., Fally, T., & Hillberry, R. (2012). Measuring the upstreamness of production and trade flows. The American Economic Review, 102(3), 412–416. https://doi.org/10.1257/aer.102.3.412
- Ara, T. (2020). Country size, technology, and Ricardian comparative advantage. Review of International Economics, 28(2), 497–536. https://doi.org/10.1111/roie.12461
- Arvis, J.-F., Mustra, M. A., Panzer, J., Ojala, L., & Naula, T. (2007). Connecting to compete: Trade logistics in the global economy. World Bank.
- Bahar, D., Hausmann, R., & Hidalgo, C. A. (2014). Neighbors and the evolution of the comparative advantage of nations: Evidence of international knowledge diffusion? Journal of International Economics, 92(1), 111–123. https://doi.org/10.1016/j.jinteco.2013.11.001
- Baldwin, R., & Venables, A. J. (2013). Spiders and snakes: Offshoring and agglomeration in the global economy. Journal of International Economics, 90(2), 245–254. https://doi.org/10.1016/j.jinteco.2013.02.005
- Bamieh, O., Fiorini, M., Hoekman, B., & Jakubik, A. (2020). Services input intensity and US manufacturing employment responses to the China shock. Review of Industrial Organization, 57(2), 333–349. https://doi.org/10.1007/s11151-020-09770-2
- Beck, T. (2003). Financial dependence and international trade. Review of International Economics, 11(2), 296–316. https://doi.org/10.1111/1467-9396.00384
- Bohn, T., Brakman, S., & Dietzenbacher, E. (2018). The role of services in globalisation. World Economy, 41(10), 2732–2749. https://doi.org/10.1111/twec.12640
- Bottasso, A., Conti, M., de Sa Porto, P. C., Ferrari, C., & Tei, A. (2018). Port infrastructures and trade: Empirical evidence from Brazil. Transportation Research Part A: Policy and Practice, 107, 126–139. https://doi.org/10.1016/j.tra.2017.11.013
- Brondino, G. (2023). Fragmentation of production, comparative advantage, and the Heckscher-Ohlin theory. Review of Political Economy, 35(3), 803–822. https://doi.org/10.1080/09538259.2021.1977540
- Bugarčić, F. Ž., Skvarciany, V., & Stanišić, N. (2020). Logistics performance index in international trade: Case of Central and Eastern European and Western Balkans countries. Business: Theory and Practice, 21(2), 452–459. https://doi.org/10.3846/btp.2020.12802
- Burstein, A., & Vogel, J. (2017). International trade, technology, and the skill premium. Journal of Political Economy, 125(5), 1356–1412. https://doi.org/10.1086/693373
- Cai, J., Li, N., & Santacreu, A. M. (2022). Knowledge diffusion, trade, and innovation across Countries and sectors. American Economic Journal Macroeconomics, 14(1), 104–145. https://doi.org/10.1257/mac.20200084
- Cai, J., & Stoyanov, A. (2016). Population aging and comparative advantage. Journal of International Economics, 102, 1–21. https://doi.org/10.1016/j.jinteco.2016.04.006
- Cassini, L. (2023). Path-dependent productive specialization: Should prematurely deindustrialized countries shift to a KIBS export-led strategy? Structural Change and Economic Dynamics, 65, 199–209. https://doi.org/10.1016/j.strueco.2023.02.016
- Ceglowski, J. (2017). Assessing export competitiveness through the lens of value added. World Economy, 40(2), 275–296. https://doi.org/10.1111/twec.12362
- Çelebi, D. (2019). The role of logistics performance in promoting trade. Maritime Economics & Logistics, 21(3), 307–323. https://doi.org/10.1057/s41278-017-0094-4
- Chen, X., & Shao, Y. (2020). Product life-cycle, knowledge capital, and comparative advantage. Review of International Economics, 28(1), 252–278. https://doi.org/10.1111/roie.12449
- Choi, N., & Park, S. (2018). Ricardian comparative advantage and value added in exports. Korea and the World Economy, 19(1), 23–50. https://doi.org/10.46665/kwe.2018.04.19.1.23
- Chor, D. (2010). Unpacking sources of comparative advantage: A quantitative approach. Journal of International Economics, 82(2), 152–167. https://doi.org/10.1016/j.jinteco.2010.07.004
- Chor, D., Manova, K., & Yu, Z. (2021). Growing like China: Firm performance and global production line position. Journal of International Economics, 130, 103445. https://doi.org/10.1016/j.jinteco.2021.103445
- Costinot, A. (2009). On the origins of comparative advantage. Journal of International Economics, 77(2), 255–264. https://doi.org/10.1016/j.jinteco.2009.01.007
- Costinot, A., & Donaldson, D. (2012). Ricardo’s theory of comparative advantage: Old idea, new evidence. The American Economic Review, 102(3), 453–458. https://doi.org/10.1257/aer.102.3.453
- Costinot, A., Donaldson, D., & Komunjer, I. (2012). What goods do Countries trade? A quantitative exploration of Ricardo’s ideas. The Review of Economic Studies, 79(2), 581–608. https://doi.org/10.1093/restud/rdr033
- Costinot, A., & Komunjer, I. (2007). What goods do Countries trade? New Ricardian predictions (w13691; p. w13691). National Bureau of Economic Research. https://doi.org/10.3386/w13691
- Costinot, A., & Vogel, J. (2015). Beyond Ricardo: Assignment models in international trade. Annual Review of Economics, 7(1), 31–62. https://doi.org/10.1146/annurev-economics-080213-041435
- Cuñat, A., & Melitz, M. J. (2012). Volatility, labor market flexibility, and the pattern of comparative advantage. Journal of the European Economic Association, 10(2), 225–254. https://doi.org/10.1111/j.1542-4774.2011.01038.x
- Deardorff, A. V. (2005). Ricardian comparative advantage with intermediate inputs. The North American Journal of Economics & Finance, 16(1), 11–34. https://doi.org/10.1016/j.najef.2004.11.003
- Debaere, P. (2003). Relative factor abundance and trade. Journal of Political Economy, 111(3), 589–610. https://doi.org/10.1086/374179
- Dietzenbacher, E., Luna, I. R., & Bosma, N. S. (2005). Using average propagation lengths to identify production chains in the Andalusian economy. Estudios de Economia Aplicada, 23, 405–422. https://research.rug.nl/en/publications/using-average-propagation-lengths-to-identify-production-chains-i
- Dietzenbacher, E., & Romero, I. (2007). Production chains in an interregional framework: Identification by means of average propagation lengths. International Regional Science Review, 30(4), 362–383. https://doi.org/10.1177/0160017607305366
- Eaton, J., & Kortum, S. (2002). Technology, geography, and trade. Econometrica, 70(5), 1741–1779. https://doi.org/10.1111/1468-0262.00352
- Essaji, A., & Fujiwara, K. (2012). Contracting institutions and product quality. Journal of Comparative Economics, 40(2), 269–278. https://doi.org/10.1016/j.jce.2011.07.005
- Falk, M., & Peng, F. (2013). The increasing service intensity of European manufacturing. The Service Industries Journal, 33(15–16), 1686–1706. https://doi.org/10.1080/02642069.2011.639872
- Fally, T. (2012). Production staging: Measurement and facts. Boulder, Colorado: University of Colorado Boulder. https://citeseerx.ist.psu.edu/document?repid=rep1&type=pdf&doi=8ff103e6b2573a063bdfcac61ef73550b79467c7
- Fawcett, S. E., & Closs, D. J. (1993). Coordinated global manufacturing, the logistics/manufacturing interaction, and firm performance. Journal of Business Logistics, 14(1), 1.
- Feenstra, R. C., Hong, C., Ma, H., & Spencer, B. J. (2013). Contractual versus non-contractual trade: The role of institutions in China. Journal of Economic Behavior and Organization, 94, 281–294. https://doi.org/10.1016/j.jebo.2013.08.009
- Ferguson, S., & Formai, S. (2013). Institution-driven comparative advantage and organizational choice. Journal of International Economics, 90(1), 193–200. https://doi.org/10.1016/j.jinteco.2012.11.007
- Gani, A. (2017). The logistics performance effect in international trade. The Asian Journal of Shipping and Logistics, 33(4), 279–288. https://doi.org/10.1016/j.ajsl.2017.12.012
- Gaubert, C., & Itskhoki, O. (2021). Granular comparative advantage. Journal of Political Economy, 129(3), 871–939. https://doi.org/10.1086/712444
- Ghasemkhani, A., Ghavidel Doostkouei, S., Gholamabri, A., & Mousavi, M. H. (2022a). Economic complexity, inefficiency, and comparative advantage. Journal of Econometric Modelling. https://doi.org/10.22075/jem.2022.24184.1629
- Ghasemkhani, A., Ghavidel Doostkouei, S., Gholamabri, A., & Mousavi, M. H. (2022b). The origins of comparative advantage: Economic complexity and industrial inefficiency. Applied Economics, 55(55), 6551–6566. https://doi.org/10.1080/00036846.2022.2159011
- Gong, B., & Zhou, H. (2014). Financial development, the choice of technology, and comparative advantage. The Journal of International Trade & Economic Development, 23(8), 1238–1261. https://doi.org/10.1080/09638199.2013.868023
- Gouma, R., Chen, W., Woltjer, P., & Timmer, M. (2018). WIOD socio-economic accounts 2016. University of Groningen.
- Gur, N., & Avşar, V. (2016). Financial system, R&D intensity and comparative advantage. The Journal of International Trade & Economic Development, 25(2), 213–239. https://doi.org/10.1080/09638199.2015.1045928
- Hausmann, R., & Klinger, B. (2006). Structural transformation and patterns of comparative advantage in the product space. CID Working Paper, No. 128. https://doi.org/10.2139/ssrn.939646
- Hausmann, R., & Klinger, B. (2007). The structure of the product space and the evolution of comparative advantage. Cid Working Papers,No. 146.
- Hausmann, R., Stock, D. P., & Yıldırım, M. A. (2022). Implied comparative advantage. Research Policy, 51(8), 104143. https://doi.org/10.1016/j.respol.2020.104143
- Hesse, M., & Rodrigue, J.-P. (2006). Global production networks and the role of logistics and transportation. Growth and Change, 37(4), 499–509. https://doi.org/10.1111/j.1468-2257.2006.00337.x
- Hidalgo, C. A., Klinger, B., Barabasi, A.-L., & Hausmann, R. (2007). The product space conditions the development of nations. Science, 317(5837), 482–487. https://doi.org/10.1126/science.1144581
- Hirsch, S. (1989). Services and service intensity in international trade. Review of World Economics, 125(1), 45–60. https://doi.org/10.1007/BF02707518
- Hoekman, B., & Shepherd, B. (2015). Services productivity, trade policy, and manufacturing exports. SSRN Electronic Journal, 26. https://doi.org/10.2139/ssrn.2553931
- Hummels, D., Ishii, J., & Yi, K.-M. (2001). The nature and growth of vertical specialization in world trade. Journal of International Economics, 54(1), 75–96. https://doi.org/10.1016/s0022-1996(00)00093-3
- Ishise, H. (2016). Capital heterogeneity as a source of comparative advantage: Putty-clay technology in a ricardian model. Journal of International Economics, 99, 223–236. https://doi.org/10.1016/j.jinteco.2015.11.004
- Ito, T., Rotunno, L., & Vézina, P. (2017). Heckscher–Ohlin: Evidence from virtual trade in value added. Review of International Economics, 25(3), 427–446. https://doi.org/10.1111/roie.12230
- Johnson, R. C. (2018). Measuring global value chains. Annual Review of Economics, 10(1), 207–236. https://doi.org/10.1146/annurev-economics-080217-053600
- Johnson, R. C., & Noguera, G. (2012). Accounting for intermediates: Production sharing and trade in value added. Journal of International Economics, 86(2), 224–236. https://doi.org/10.1016/j.jinteco.2011.10.003
- Ju, J., & Wei, S.-J. (2011). When is quality of financial system a source of comparative advantage? Journal of International Economics, 84(2), 178–187. https://doi.org/10.1016/j.jinteco.2011.03.004
- Kabak, Ö., Ülengin, F., & Önsel Ekici, Ş. (2018). Connecting logistics performance to export: A scenario-based approach. Research in Transportation Economics, 70, 69–82. https://doi.org/10.1016/j.retrec.2018.05.007
- Kee, H. L., & Tang, H. (2016). Domestic value added in exports: Theory and firm evidence from China. The American Economic Review, 106(6), 1402–1436. https://doi.org/10.1257/aer.20131687
- Keuschnigg, C., & Kogler, M. (2022). Trade and credit reallocation: How banks help shape comparative advantage. Review of International Economics, 30(1), 282–305. https://doi.org/10.1111/roie.12564
- Koch, P., & Fessler, C. (2020). A test for Heckscher-Ohlin using value-added exports. arXiv: General Economics. https://www.semanticscholar.org/paper/14818cc8258f34e505fac6895344c41e5b291edb
- Koopman, R., Wang, Z., & Wei, S.-J. (2014). Tracing value-added and double counting in gross exports. The American Economic Review, 104(2), 459–494. https://doi.org/10.1257/aer.104.2.459
- Korinek, J., & Sourdin, P. (2011). To what extent are high-quality logistics services trade facilitating? OECD Publishing.
- Krishna, P., & Levchenko, A. A. (2013). Comparative advantage, complexity, and volatility. Journal of Economic Behavior and Organization, 94, 314–329. https://doi.org/10.1016/j.jebo.2012.11.004
- Leontief, W. (1953). Domestic production and foreign trade; the American capital position re-examined. Proceedings of the American Philosophical Society, 97(4), 332–349.
- Levchenko, A. A. (2007). Institutional quality and international trade. The Review of Economic Studies, 74(3), 791–819. https://doi.org/10.1111/j.1467-937X.2007.00435.x
- Levchenko, A. A., & Zhang, J. (2014). Ricardian productivity differences and the gains from trade. European Economic Review, 65, 45–65. https://doi.org/10.1016/j.euroecorev.2013.10.006
- Liu, X., Mattoo, A., Wang, Z., & Wei, S. J. (2020). Services development and comparative advantage in manufacturing. Journal of Development Economics, 144, 102438. https://doi.org/10.1016/j.jdeveco.2019.102438
- Los, B., Timmer, M. P., & de Vries, G. J. (2015). How global are global value chains? A new approach to measure international fragmentation. Journal of Regional Science, 55(1), 66–92. https://doi.org/10.1111/jors.12121
- Ma, Y., Qu, B., & Zhang, Y. (2010). Judicial quality, contract intensity and trade: Firm-level evidence from developing and transition countries. Journal of Comparative Economics, 38(2), 146–159. https://doi.org/10.1016/j.jce.2009.09.002
- Manova, K. (2008). Credit constraints, heterogeneous firms, and International trade (NBER working papers 14531). National Bureau of Economic Research, Inc. https://ideas.repec.org/p/nbr/nberwo/14531.html
- Markusen, J. R. (1989). Trade in producer services and in other specialized intermediate inputs. The American Economic Review, 79(1), 85–95.
- Mendes dos Reis, J. G., Sanches Amorim, P., Sarsfield Pereira Cabral, J. A., & Toloi, R. C. (2020). The impact of logistics performance on Argentina, Brazil, and the US soybean exports from 2012 to 2018: A gravity model approach. Agriculture, 10(8), 338. https://doi.org/10.3390/agriculture10080338
- Meng, B., Fang, Y., Guo, J., & Zhang, Y. (2017). Measuring China’s domestic production networks through trade in value-added perspectives. Economic Systems Research, 29(1), 48–65. https://doi.org/10.1080/09535314.2017.1282435
- Meng, B., Ye, M., & Wei, S. (2020). Measuring smile curves in global value chains. Oxford Bulletin of Economics and Statistics, 82(5), 988–1016. https://doi.org/10.1111/obes.12364
- Miller, R. E., & Temurshoev, U. (2017). Output upstreamness and input downstreamness of industries/countries in World production. International Regional Science Review, 40(5), 443–475. https://doi.org/10.1177/0160017615608095
- Miroudot, S., & Cadestin, C. (2017). Services in global value chains: Trade patterns and gains from specialisation (OECD trade policy papers 208). OECD Publishing. https://www.oecd-ilibrary.org/trade/services-in-global-value-chains-trade-patterns-and-gains-from-specialisation_06420077-en
- Morrow, P. M., & Trefler, D. (2017). Endowments, skill-biased technology, and factor prices: A unified approach to trade (w24078; p. w24078). National Bureau of Economic Research. https://doi.org/10.3386/w24078
- Morrow, P. M., & Trefler, D. (2022). How do endowments determine trade? Quantifying the output mix, factor price, and skill-biased technology channels. Journal of International Economics, 137, 103620. https://doi.org/10.1016/j.jinteco.2022.103620
- Navas, A. (2018). Technology and the dynamics of comparative advantage. Review of International Economics, 26(1), 140–164. https://doi.org/10.1111/roie.12309
- Nishioka, S. (2012). International differences in production techniques: Implications for the factor content of trade. Journal of International Economics, 87(1), 98–104. https://doi.org/10.1016/j.jinteco.2011.11.010
- Nishioka, S. (2013). R&D, trade in intermediate inputs, and the comparative advantage of advanced countries. Journal of the Japanese and International Economies, 30, 96–110. https://doi.org/10.1016/j.jjie.2013.10.004
- Nunn, N. (2007). Relationship-specificity, incomplete contracts, and the pattern of trade. Quarterly Journal of Economics, 122(2), 569–600. https://doi.org/10.1162/qjec.122.2.569
- Pahl, S., & Timmer, M. P. (2020). Do global value chains enhance economic upgrading? A long view. The Journal of Development Studies, 56(9), 1683–1705. https://doi.org/10.1080/00220388.2019.1702159
- Park, S. (2020). Quality of transport infrastructure and logistics as source of comparative advantage. Transport Policy, 99, 54–62. https://doi.org/10.1016/j.tranpol.2020.07.016
- Park, S. M. (2023). Domestic formal and informal institutions: Their substitutability and comparative advantage. Review of World Economics, 159(4), 853–886. https://doi.org/10.1007/s10290-022-00483-0
- Rodrigue, J.-P. (2006). Transportation and the geographical and functional integration of global production networks. Growth and Change, 37(4), 510–525. https://doi.org/10.1111/j.1468-2257.2006.00338.x
- Romalis, J. (2004). Factor proportions and the structure of commodity trade. The American Economic Review, 94(1), 67–97. https://doi.org/10.1257/000282804322970715
- Sampson, T. (2023). Technology gaps, trade, and income. The American Economic Review, 113(2), 472–513. https://doi.org/10.1257/aer.20201940
- Saslavsky, D., & Shepherd, B. (2014). Facilitating international production networks: The role of trade logistics. The Journal of International Trade & Economic Development, 23(7), 979–999. https://doi.org/10.1080/09638199.2013.811534
- Somale, M. (2021). Comparative advantage in innovation and production. American Economic Journal Macroeconomics, 13(3), 357–396. https://doi.org/10.1257/mac.20180295
- Stehrer, R. (2012). Trade in value added and the valued added in trade. (Working Paper 81). wiiw Working Paper. https://www.econstor.eu/handle/10419/203946
- Stöllinger, R., & Guarascio, D. (2023). Comparative advantages in the digital era–A Heckscher-Ohlin-Vanek approach. International Economics, 175, 63–89. https://doi.org/10.1016/j.inteco.2023.05.002
- Timmer, M. P., Erumban, A. A., Los, B., Stehrer, R., & de Vries, G. J. (2014). Slicing up global value chains. Journal of Economic Perspectives, 28(2), 99–118. https://doi.org/10.1257/jep.28.2.99
- Töngür, Ü., Türkcan, K., & Ekmen-Özçelik, S. (2020). Logistics performance and export variety: Evidence from Turkey. Central Bank Review, 20(3), 143–154. https://doi.org/10.1016/j.cbrev.2020.04.002
- Trefler, D. (1993). International factor price differences: Leontief was right! Journal of Political Economy, 101(6), 961–987. https://doi.org/10.1086/261911
- Trefler, D. (1995). The case of the missing trade and other mysteries. The American Economic Review, 85(5), 1029–1046.
- Vanek, J. (1968). The factor proportions theory: The N—factor case. Kyklos, 21(4), 749–756. https://doi.org/10.1111/j.1467-6435.1968.tb00141.x
- Wang, Y., & Turkina, E. (2020). Economic complexity, product space network and Quebec’s global competitiveness. Canadian Journal of Administrative Sciences/Revue Canadienne des Sciences de L’administration, 37(3), 334–349. https://doi.org/10.1002/cjas.1555
- Wang, Z., Wei, S.-J., Yu, X., & Zhu, K. (2017a). Characterizing global value chains: Production length and upstreamness (w23261; p. w23261). National Bureau of Economic Research. https://doi.org/10.3386/w23261
- Wang, Z., Wei, S.-J., Yu, X., & Zhu, K. (2017b). Measures of participation in global value chains and global business cycles (w23222; p. w23222). National Bureau of Economic Research. https://doi.org/10.3386/w23222
- Wang, Z., Wei, S. J., & Zhu, K. (2013). Quantifying international production sharing at the bilateral and sector levels (w19677). National Bureau of Economic Research. https://doi.org/10.3386/w19677
- Yang, L., Dong, Q., Tong, Z., Wang, Q., & Wu, J. (2022). Logistics input intensity, trade facilitation and comparative advantage. The Journal of International Trade & Economic Development, 31(5), 725–741. https://doi.org/10.1080/09638199.2021.2011380
- Zhang, H., Meng, B., & Ma, S. (2018). Determinants of China’s bilateral trade balance in global value chains. The Journal of International Trade & Economic Development, 27(5), 463–485. https://doi.org/10.1080/09638199.2017.1391322