ABSTRACT
A widespread concern in society is that adolescents experience an increased inability to concentrate and sustain attention because they are continuously distracted by social media. The current experience sampling method (ESM) study examined whether adolescents who use more social media than their peers experience more distraction (between-person association), whether social media use (SMU) and distraction co-fluctuate within adolescents (momentary within-person associations), and to what extent this within-person association differs from person to person (person-specific associations). With a sample of 383 adolescents (Mage = 14.11), who together completed 35,099 ESM surveys (73% compliance), we found both a positive between-person association (β = .31) and a positive momentary within-person association (β = .12) of SMU with distraction. The momentary within-person association differed from adolescent to adolescent: While SMU and distraction were positively associated among 82.5% of all adolescents, they were not associated among 15.7% of the adolescents, and negatively associated among 1.8% of the adolescents. Additional analyses on the direction of the effect showed that the within-person effect of SMU on subsequent levels of distraction was somewhat stronger (β = .05) than the effect of distraction on subsequent levels of SMU (β = .03).
Developing the ability to voluntarily focus attention and control potential distractions (i.e., attentional control; Chin et al., Citation2020; Diamond, Citation2013; Tavares & Freire, Citation2016) is an important task for adolescents (Higgins & Turnure, Citation1984; Luna, Citation2009; Luna, Garver, Urban, Lazar, & Sweeney, Citation2004). Yet, concerns have been raised that adolescents experience more and more difficulty in developing this ability because of the distractions posed by social media. This is hardly surprising given that adolescents are avid users of social media and receive notifications and messages throughout the entire day from various social media apps, such as WhatsApp, Instagram, and Snapchat (Anderson & Jiang, Citation2018; van Driel, Pouwels, Beyens, Keijsers, & Valkenburg, Citation2019). However, whether and to what extent adolescents’ social media use (SMU) goes hand in hand with greater distractions still largely remains unclear.
Up until now, about a dozen studies have tapped into the association between SMU and distraction, mostly among (young) adults. One part of these studies has investigated to what extent social media are distracting. These studies have shown that even the mere presence of a smartphone, the device adolescents typically use for social media, can lead to distraction (e.g., Johannes, Veling, Verwijmeren, & Buijzen, Citation2019; Kushlev, Proulx, & Dunn, Citation2016; Stothart, Mitchum, & Yehnert, Citation2015; Thornton, Faires, Robbins, & Rollins, Citation2014). A second part of these studies has shown that these social media or smartphone distractions are related to various outcomes, such as academic achievement (e.g., Fox, Rosen, & Crawford, Citation2009; McCoy, Citation2016), task performance (Brooks, Citation2015), well-being (Johannes et al., Citation2020), and productivity (Mark, Iqbal, & Czerwinski, Citation2017). Finally, a third part of these studies has revealed that (social) media use is related to distraction and various indicators of failure in attentional control, such as concentration problems (Aalbers et al., Citation2019; Levine, Waite, & Bowman, Citation2007; Xie, Rost, Wang, Wang, & Monk, Citation2021).
While providing important insights into the topic of SMU and distraction, the current literature leaves two important gaps. A first gap is that knowledge about the association between SMU and distraction among adolescents is scarce, especially about the momentary associations. Whereas the few prior studies predominantly focused on the associations between SMU and trait-like operationalizations of distraction, it has been shown that trait-like levels of attention and distraction can also fluctuate on a momentary basis, for example, as a consequence of tiredness (Riley, Esterman, Fortenbaugh, & DeGutis, Citation2017) or disturbing environmental factors (Vasilev, Kirkby, & Angele, Citation2018). Thus, the question of whether adolescents’ SMU and distraction co-fluctuate on a momentary basis remains unanswered. Therefore, the first aim of this study is to examine both the between-person and the momentary within-person associations of adolescents’ SMU with distraction.
A second related gap in the literature is that studies have overlooked the idea that the association between SMU and distraction may differ from person to person. Media effects theories and models such as the Differential Susceptibility to Media Effects Model (DSMM; Valkenburg & Peter, Citation2013) assume that adolescents differ in how they select and respond to social media. Following this line of thought, the association between SMU and distraction may be stronger for certain adolescents than for others, for instance, because they may vary in their overall usage of social media or in their trait-like ability to sustain attention. To truly understand how SMU and distraction are related within each individual adolescent, scholars have recently called for a person-specific (or idiographic) approach in research on SMU (e.g., Beyens, Pouwels, van Driel, Keijsers, & Valkenburg, Citation2020b; Odgers & Jensen, Citation2020; Valkenburg, Beyens, Pouwels, van Driel, & Keijsers, Citation2021a). Therefore, our second aim is to investigate the association between SMU and distraction for every single adolescent and to examine to what extent these person-specific associations differ from adolescent to adolescent.
To address these two gaps in the literature, the current study investigated the between-person, the within-person, and the person-specific associations between SMU and distraction among adolescents. To that end, we conducted an experience sampling study among a sample of 383 adolescents (35,099 observations in total). We focused on adolescents because, on the one hand, their underdeveloped abilities for attentional control make them more vulnerable to experience distractions compared to other age groups, such as young adults (Cohen Kadosh, Heathcote, & Lau, Citation2014; Stawarczyk, Majerus, Catale, & D’Argembeau, Citation2014). On the other hand, adolescents are avid users of social media, and social media platforms form the perfect stage to fulfill developmental tasks, such as forming an identity, bonding with peers, and becoming autonomous from parents (Borca, Bina, Keller, Gilbert, & Begotti, Citation2015; Meeus, Eggermont, & Beullens, Citation2018).
The Between-Person Association of Social Media Use with Distraction
Young adults who spend more time on social media than others experience more distraction (Aalbers et al., Citation2019; Levine et al., Citation2007; Xie et al., Citation2021). An important explanation for this finding is the fact that some individuals may be more alert to social media notifications than others. This alertness, called “online vigilance” (Johannes et al., Citation2019; Reinecke et al., Citation2018), may develop automatic tendencies to check social media regularly (Bayer, Campbell, & Ling, Citation2016). These automatic checking behaviors, or “connection habits” (Bayer et al., Citation2016), could make it difficult to focus, shift, and sustain attention voluntarily. Hence, adolescents who display higher levels of constant alertness may have more difficulties sustaining attention and stay focused than their peers, and may use social media more than their peers.
The first contribution of this study is to shed light on these individual differences between adolescents. Building on the approach of Aalbers et al. (Citation2019), we investigated the between-person association of SMU with distraction by aggregating across all within-person momentary measures of SMU and distraction (i.e., 126 momentary assessments at maximum) toward a trait-like measure (Fleeson, Citation2001). Such momentary assessments generally reduce recall bias, and, as a result, yield more ecologically valid data than one-time, global measurements (Van Gog, Kirschner, Kester, & Paas, Citation2012; van Roekel, Keijsers, & Chung, Citation2019). Altogether, in line with prior studies among young adults and assumptions from online vigilance, we expected to find a positive between-person association of SMU with distraction.
H1: SMU is positively associated with distraction at the between-person level, such that adolescents who spend more time using social media (compared to other adolescents) experience more distraction (compared to other adolescents).
The Momentary Within-Person Association of Social Media Use with Distraction
Indications exist that adolescents’ preoccupation with social media may fluctuate from moment to moment (Johannes et al., Citation2020). In fact, online vigilance is typically described as a “state of alertness” (Johannes et al., Citation2019, p. 215). For instance, adolescents may sometimes be more occupied with receiving messages from friends on WhatsApp or receiving likes on an Instagram post than at other moments. At moments when adolescents experience a heightened state of alertness, they may experience more difficulties to focus their attention and a stronger tendency to check their social media, and thus spend more time using social media.
Only one study among young adults has tapped into the momentary co-fluctuations between SMU and attentional control (Aalbers et al., Citation2019). Aalbers et al. adopted a dynamic network approach to examine to what extent SMU and concentration problems are associated contemporaneously. They found that college students experienced more concentration problems during hours when they spent more time on social media, compared to hours when they spent less time on social media. As concentration problems and distraction are both indicators of attentional control failure, we hypothesized a positive association between adolescents’ momentary SMU and momentary distraction at the within-person level.
H2: Momentary SMU is positively associated with momentary distraction at the within-person level, such that an increase in an adolescent’s time spent using social media (relative to the adolescent’s mean) is accompanied by an increase in this adolescent’s distraction (relative to the adolescent’s mean).
Heterogeneity in the Association between SMU and Distraction
Models of media uses and effects suggest that the way adolescents select and respond to media differs substantially between individuals (Valkenburg & Peter, Citation2013). Likewise, the association between SMU and distraction may not be the same for each adolescent. Recently, scholars have called for research that examines heterogeneity at the person level and provides clarity on how media selection and effects differ from adolescent to adolescent (Lerner, Lerner, & Chase, Citation2019; Russell & Gajos, Citation2020). Instead of estimating an average effect size that applies to the overall sample (i.e., nomothetic approach) or subgroups (i.e., group-differential approach; e.g., based on active vs. passive use; Alloway & Alloway, Citation2012), a person-specific (or idiographic) approach estimates unique effect sizes for every individual (Lerner et al., Citation2019). This approach allows drawing conclusions based on the dispersion of associations that are unique for every person in the sample.
Recent studies have introduced this idiographic approach to social media research. For instance, Beyens et al. (Citation2020b) showed that adolescents varied greatly in the extent to which passive SMU affected their well-being. The effect sizes found in this study ranged between −.24 and +.68. Similarly, other studies showed that there was substantial heterogeneity in the person-specific associations between SMU and a wide range of indicators of well-being and psychosocial functioning, such as depressive symptoms, self-esteem, friendship closeness, envy, inspiration, and enjoyment (Pouwels, Valkenburg, Beyens, van Driel, & Keijsers, Citation2021; Rodriguez, Aalbers, & McNally, Citation2021; Valkenburg et al., Citation2021a; Valkenburg, Beyens, Pouwels, van Driel, & Keijsers, Citation2021b). These studies underline that investigating person-specific susceptibilities is essential to obtain a deeper understanding of the scope of various effects that might manifest in a given population.
Up to now, studies have shown individual differences in both SMU and distraction: Adolescents vary in the extent to which they use social media, how they use it, and for what purposes (Rideout & Fox, Citation2018), and they differ in their attentional control strategies and their level of distraction (Irons & Leber, Citation2020). However, what remains unknown is whether the within-person association between SMU and distraction also varies from adolescent to adolescent. The third contribution of this study is to shed light on how the association between SMU and distraction differs from person to person. Since this study is the first to examine the unique associations between SMU and distraction per adolescent, we had no clear expectations as to how heterogeneity would manifest itself. Thus, as a first step, we investigated the following research question:
RQ1: To what extent does the association between SMU and distraction differ from adolescent to adolescent?
Method
The current preregistered study (see https://osf.io/cszk7/) is part of a larger project that investigates the psychosocial consequences of SMU among adolescents. The current study uses data from the first three-week ESM wave of the project, which was conducted in November and December 2019. While other studies in the larger project used data from this first ESM wave to investigate SMU in relation to different aspects of adolescents’ psychosocial functioning (e.g., well-being, self-esteem), this study was the first to investigate SMU in relation to distraction (for a full overview, see https://osf.io/spuza/).
Participants
A priori power analyses conducted before setting up the larger project (see https://osf.io/tk8pw/) suggested that a sample size of 300 participants with at least 63 assessments (i.e., 50% of all assessments) would be sufficient to be able to detect small effect sizes and variance around these effects with a minimum of 80% power and a significance level of 5%. To account for potential technical failures and participant drop-out, we aimed for a sample size of 400 participants.
In total, 745 7th and 8th graders at a secondary school in the south of the Netherlands were invited to participate in the research project and 387 gave consent (52% consent rate) and signed up for the first ESM wave of this project. Following our preregistered plan, we excluded four participants from the analyses because they did not use social media, which resulted in a final sample of 383 subjects. On average, adolescents were 14.11 years old (SD = 0.69), 56% were girls, and 96% were born in the Netherlands and self-identified as being Dutch. The educational level of the adolescents varied between the prevocational secondary education track (42%), the intermediate general secondary education track (31%), and the academic preparatory education track (27%).
Procedure
The procedure of this project was approved by the Ethics Review Board of the Faculty of Social and Behavioral Sciences at the University of Amsterdam. Several weeks ahead of data collection, researchers visited the school to provide adolescents and parents with detailed information about the goals of the research project. The students were enrolled after they and their parents gave written consent for participation. At the end of November 2019, researchers visited the school to provide participants with procedural instructions and to conduct a baseline survey. After completion of the baseline survey, participants installed the Ethica app on their mobile phones, which was required to collect the ESM data. An initial ESM survey was prompted from the app to check whether the installation was successful and to collect data on adolescents’ SMU required for subsequent ESM triggers (i.e., to personalize their trigger schedule based on the school schedule and to tailor the SMU questions in the ESM surveys). Participants were free to ask questions to the three researchers who were present. The three-week ESM study started four days after this baseline session.
ESM assessments
In total, each participant received 126 ESM surveys distributed over six time points per day, for 21 consecutive days. This total number was based on the recommendation to have at least 50 to 100 assessments per participant to allow a meaningful interpretation of person-specific effects (Molenaar & Campbell, Citation2009; Voelkle, Oud, von Oertzen, & Lindenberger, Citation2012). The assessments were semi-randomly distributed throughout the day, that is, at random times within fixed intervals to account for class schedules and sleeping in on Saturdays and Sundays (the notification scheme is available at https://osf.io/9dnjm/). Participants had 30 min to complete the survey before it expired. For the first and last surveys of each day, the expiration time was extended to 60 min and 120 min, respectively, to account for travel time and evening activities. After five to ten minutes, an automatic reminder popped up if participants had not yet completed the survey.
Incentives
Participants received a small gadget for completing the baseline survey and €0.30 for every completed ESM survey. In addition, each day we ran a lottery in which four winners were drawn from a pool of participants who had completed all surveys on the day before. The lottery winners all received a bonus of €25. An interactive website for real-time monitoring (built with Shiny R; Chang, Cheng, Allaire, Xie, & McPherson, Citation2020) allowed participants to inspect FAQs, the page where lottery winners were announced, and their personal dashboard including an overview of their total earnings and compliance.
Compliance
In theory, a total of 48,258 surveys could be completed in this study, based on 383 participants who each received 126 assessments. Due to unforeseen technical errors, the Ethica app failed to send out 449 surveys (1%). As such, a total of 47,809 surveys was sent successfully (for a compliance overview of the full project, see https://osf.io/se98b/). With 35,099 completed surveys, the overall compliance in this study was 73%, which is a good compliance rate compared to other ESM studies among adolescents (van Roekel et al., Citation2019). On average, participants completed 91.6 surveys each (SD = 23.5, range = 11–125), which gave sufficient power to conduct person-specific effects analyses (Molenaar & Campbell, Citation2009; Voelkle et al., Citation2012). The group of lowly committed participants (i.e., those who completed less than a quarter of all surveys) was relatively small (4.2%) compared to the group of highly committed participants (i.e., those who completed more than three-quarters of all surveys; 53.8%).
Measures
Each ESM survey consisted of 23 items, including questions about SMU, distraction, and other topics not included in the current study. The morning and evening surveys each contained one additional question.
Social media use
Social media use was measured with eight items over three different social media platforms: Instagram, WhatsApp, and Snapchat. Participants only received questions about a specific platform if they used it more than once a week, as indicated in the initial survey that was distributed directly after the baseline measurement. The total number of ESM items was equally balanced across individuals by providing alternative questions to those participants who did not use Instagram, WhatsApp, or Snapchat. Instagram use was measured with three items (“How much time over the past hour have you spent viewing posts/ stories of others on Instagram?” “ … reading direct messages on Instagram?” and “ … sending direct messages on Instagram?”), WhatsApp use with two items (“ … reading messages on WhatsApp?” and “ … sending messages on WhatsApp?”), and Snapchat use with three items (“ … viewing stories of others on Snapchat?” “ … viewing snaps of others on Snapchat?” and “ … sending snaps on Snapchat?”). Participants could choose a value between 0 and 60 min, with 1-min intervals. Sum scores were established by summing the items per platform and were recoded as 60 min in case they exceeded 60 min (3.9% of all completed assessments). The platform scores were summed to create the total SMU measure, and values were again recoded as 60 min upon exceedance (10.5% of all completed assessments). Finally, we divided the SMU score by 10 to facilitate the interpretation and comparability with the distraction measure (i.e., scale from 0 to 6), so that an increase of 1 unit reflects an increase of 10 min of SMU. To investigate the between-person association of SMU and distraction (H1), the mean score of all momentary SMU assessments over the three weeks was used for each participant (see Fleeson, Citation2001).
Distraction
To assess distraction, participants responded to the question “To what extent were you distracted by something over the past hour?” on a 7-point scale ranging from 0 (not at all) to 6 (completely), with 3 (a little) as the midpoint. This item was based on the momentary attentional control measure of Chin et al. (Citation2020). To investigate the between-person association between SMU and distraction (H1), the mean score of all momentary distraction assessments over the three weeks was used for each participant (see Fleeson, Citation2001).
Statistical Analyses
As preregistered, we examined the between-person, within-person, and person-specific associations between SMU and distraction using multilevel modeling to account for the nested structure of the data. We conducted the analyses in Mplus 8.4 because, unlike other statistical software, it provides standardized parameter estimates for the fixed effects models (Muthén & Muthén, Citation2017, p. 799). An improvement was made to our original preregistered plan: Instead of using Maximum likelihood estimation with robust standard errors (MLR), we used Bayesian Markov Chain Monte Carlo (MCMC) estimation, for two reasons. First, in addition to standardizing the fixed effects, Bayesian estimation also standardizes the parameters for every single person (Schuurman, Ferrer, de Boer-Sonnenschein, & Hamaker, Citation2016). More specifically, for each individual, Mplus standardizes the coefficients at the within-person level, thereby providing the person-specific within-person standardized parameters. We refer to this as person-specific associations and interpret the estimates as person-specific effect sizes. Second, the Bayes estimator uses latent person means and latent person-mean centering for disentangling between- and within-person associations, which is preferred over observed means and observed person-mean centering (McNeish & Hamaker, Citation2020). Since we did not specify priors, the parameter estimation fully relied on the data. The results of the Bayesian estimation method are therefore practically similar to those obtained when using the preregistered MLR estimation method (Van de Schoot et al., Citation2014; see supplement A on https://osf.io/tzn34/ for the model results obtained with MLR estimation).
We estimated four multilevel models with distraction as outcome variable. The null model (or intercept-only model, Model 0) was estimated with three levels, namely observations (level 1) nested within persons (level 2), who were nested within classes (level 3), to determine the amount of variance at each level. Since the variance in distraction at the class level was only 5%, we continued the analyses by estimating two-level rather than three-level models. We used the two-level intercept model to compute the Intra-Class Correlations (ICC) via the statsBy() function from the psych package in R (R Core Team, Citation2020; Revelle, Citation2020).
The first model (Model 1) served as our reference model and included two fixed control variables to detrend the data: the notification number of the day and a dummy variable indicating whether it was a weekday (0) or a weekend day (1). Detrending was required because we were interested in the within-person associations of SMU with distraction irrespective of the type of day or time of the day (Wang & Maxwell, Citation2015). In the second model (Model 2), SMU was added as a fixed effect both at the between-person level (i.e., latent person means) and the within-person level (i.e., latent person-mean centered scores) to investigate the between-person (H1) and within-person associations (H2) of SMU with distraction, respectively. Finally, random slopes for SMU were added to the third model (Model 3) to examine the heterogeneity in person-specific associations of SMU with distraction (RQ1).
We ran the models with a minimum of 5,000 iterations. The models successfully converged when the Potential Scale Reduction (PSR) values approached 1. We then doubled the number of iterations and ran each model again to exclude the potential of a premature stoppage problem, as recommended by Schultzberg and Muthén (Citation2018). While our original preregistered plan was to compare models based on the Akaike information criterion (AIC) and Bayesian information criterion (BIC), the Bayesian estimation approach does not provide such indices. Therefore, we compared the explained variance of each model, both at the within- and between-person level.
For between-person associations, we interpreted .10, .20, and .30 as small, medium, and large effects, respectively, thereby following Gignac and Szodorai (Citation2016). For the within-person and person-specific associations, we interpreted effect sizes as small as .05 as meaningful, as recommended by Adachi and Willoughby (Citation2015). We evaluated the person-specific effect sizes obtained from Model 3 to interpret the amount of heterogeneity and to generate a corresponding distribution plot.
Availability Statement
The codebook (https://osf.io/y85mp/), the preregistration of the design and sampling plan (https://osf.io/327cx), the preregistration of the analysis plan (https://osf.io/xszta), and the syntaxes used to prepare, analyze, and visualize the data (https://osf.io/5ts3r/) are publicly available on the Open Science Framework (OSF). The anonymized data set that was used for the current study is published on Figshare (Siebers, Beyens, Pouwels, & Valkenburg, Citation2021).
Results
Descriptive Statistics and Correlations
As shows, on average, participants reported little distraction (M = 1.42, SD = 1.72, range = 0–6) and spent 16.97 min on social media (SD = 20.90) in the preceding hour (see supplement B on https://osf.io/g29vb/ for the distributions of both variables). The results demonstrated a strong between-person correlation between SMU and distraction (r = .33, p < .001), meaning that adolescents who spent on average more time using social media than their peers across the three weeks also reported more distraction than their peers. Similarly, there was a small within-person correlation between momentary SMU and distraction (r = .12, p < .001), meaning that, on average, adolescents experienced more distraction during hours in which they spent more time on social media. The ICCs of SMU and distraction were .47 and .38, respectively. This indicates that the larger part of the variance in SMU (53%) and distraction (62%) could be attributed to within-person fluctuations (and error) and that the remaining part of the variance in SMU and distraction (i.e., 47% and 38%, respectively) could be explained by differences between adolescents.
Table 1. Descriptive statistics and within-person, between-person, and intra-class correlations of social media use and distraction
Confirmatory Analyses
All models that we used to test our hypotheses converged after 5,000 iterations. As shown in , the reference model (Model 1) indicated that distraction was higher on weekdays compared to weekend days (β = −.07, p < .001) and increased throughout the day (β = .04, p < .001). Together, the control variables explained 0.2% of the within-person variance in distraction.
Table 2. Bayesian multilevel model estimates of the within-person association, between-person association, and random effects of social media use and distraction
Our first hypothesis (H1) predicted a positive between-person association of SMU with distraction. Consistent with this hypothesis, the fixed effect of Model 2 pointed at a strong positive between-person association (β = .31, p < .001), meaning that adolescents who had spent more time on social media, on average, also experienced more distraction, on average, than their peers. Our second hypothesis (H2) predicted a positive within-person association of momentary SMU with distraction. Supporting this hypothesis, the fixed effect indicated a small positive within-person association (β = .12, p < .001), implying that, on average, adolescents experienced more distraction during hours when they used more social media. Specifically, after controlling for beep time and weekday, SMU explained 1.4% of the within-person variance in distraction and 9.7% of the between-person variance.
Heterogeneity Analysis
Our research question (RQ1) asked to what extent the within-person association of SMU with distraction differs from adolescent to adolescent. As shown in , the explained within-person variance of distraction increased from 1.6% (Model 2) to 4% (Model 3) by estimating a random effect of SMU. The variance around the slope of SMU (σ2 = 0.017, p < .001) indicated the presence of heterogeneity in the within-person association of momentary SMU with distraction (see ). illustrates how the person-specific effect sizes are distributed across the sample, with effect sizes ranging from β = −.15 to β = +.46. For most adolescents (82.5%) the association between SMU and distraction was positive (i.e., β ≥ .05). The association was non-existent (i.e., −.05 < β < .05) for 15.7% of the adolescents. Finally, for only a few adolescents (1.8%) there was a negative association (i.e., β ≤ −.05). shows two time series of the co-fluctuation between SMU and distraction, one for a participant with a positive momentary association and one for a participant with a negative momentary association.
Figure 1. Time series for the association between social media use and distraction. The x-axis displays the days of the study (ranging from 1 to 21) and covers 126 assessments.The anonymized participant’s ID and the model-based person-specific effect sizes (#β’s) are presented above the graphs. The top time series belong to an adolescent for whom a negative association was found, and the bottom time series belong to an adolescent for whom a positive association was found.
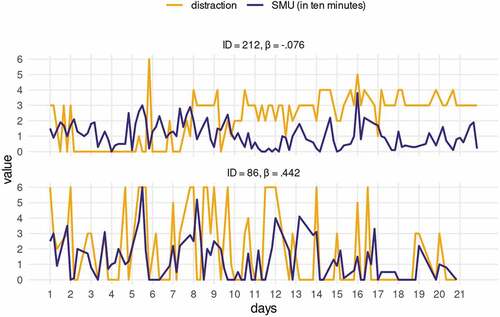
Exploratory Analyses
In addition to our preregistered analyses, we conducted two types of exploratory analyses. First, we investigated whether the heterogeneity in the within-person association between momentary SMU and distraction (Beta) could be explained by adolescents’ average levels of SMU and distraction. As presented in (Model 3), there was an interaction between average SMU and momentary SMU, as indicated by a negative effect of average SMU on Beta (β = −.16, p = .020). This interaction suggests that the positive momentary association between SMU and distraction was stronger among adolescents who spent, on average, relatively less time using social media than adolescents who spent relatively much time using social media. The cross-level interaction between the average distraction and Beta was nonsignificant (β = .08, p = .143), indicating that the association between SMU and distraction did not depend on adolescents’ average level of distraction.
In the second exploratory analysis, we ran a lag-1 multilevel Vector Autoregressive (multilevel VAR(1)) model using Dynamic Structural Equation Modeling (DSEM) in Mplus (Asparouhov, Hamaker, & Muthén, Citation2018) to examine how SMU and distraction would affect each other over time (i.e., two-hour lag). Since the model did not converge after 5,000 iterations (PSR = 1.388), we increased the number of iterations and simplified the model by excluding the correlations of the random effects with average levels of SMU and distraction. The model converged well after 10,800 iterations (PSR = 1.088). Although the effect was small, we found that SMU predicted distraction two hours later, β = .05, p < .001, 95% CI [0.03, 0.07]. The reversed effect, that is, the effect of distraction on SMU two hours later was also significant, yet almost non-existent, β = .03, p = .001, 95% CI [0.01, 0.05].
Sensitivity Analyses
We conducted sensitivity analyses to examine the robustness of the results. To that end, we conducted three sets of analyses, by excluding participants based on one of three criteria and comparing the output with our main analyses, in which all participants were included. First, as preregistered, we excluded one participant because the participant’s mean score on SMU (i.e., 59.2 min) was larger than two standard deviations above the sample mean of SMU. Second, following the procedures of van Roekel et al. (Citation2019) and following our preregistration, we excluded four participants because they provided inappropriate responses (i.e., gross comments or jokes) to the open question (i.e., the final question of the day: “What was the most pleasant experience that you had today?”). Third, we excluded twelve participants because their sum score of at least one social media platform repeatedly exceeded the maximum usage time of 60 min (i.e., in at least one-third of all assessments). The results of the sensitivity analyses showed that the main findings were robust against outliers, potentially untrustworthy response patterns, and overreporting of SMU (see Table C1, Table C2, and Table C3 on https://osf.io/5cpdw/).
Discussion
The current three-week ESM study (35,099 assessments in total) investigated the between-person, the within-person, and the person-specific associations between adolescents’ SMU and distraction. Although adolescents reported little distraction on average, our results showed that distraction fluctuated substantially within adolescents. Interestingly, we found that these fluctuations in distraction co-occurred with fluctuations in adolescents’ SMU (β = .12), implying that adolescents experienced more distraction at moments when they spent more time on social media, irrespective of the time or type of day. This finding is in line with Aalbers et al. (Citation2019), who showed that college students experienced more concentration problems at moments when they used more social media. This association of SMU and distraction at the momentary level was also found at the trait-like level (β = .31), implying that adolescents who spent, on average, more time on social media across the three weeks than their peers reported more distraction, on average, than their peers. These results corroborate previous findings among college students (Aalbers et al., Citation2019; Levine et al., Citation2007; Xie et al., Citation2021).
An explanation as to why adolescents perceived more distraction when they spent more time using social media could be that they experienced an increased state of online vigilance and preoccupation with social media (Johannes et al., Citation2019). More specifically, at moments when adolescents felt more preoccupied with social media, they may have experienced more difficulties focusing their attention and a stronger tendency to check social media regularly. Countless affordances of social media platforms (e.g., keeping in touch with friends, expressing oneself, learning about social norms) have made these platforms more ubiquitous than ever for adolescents (Anderson & Jiang, Citation2018). In addition, social media have become more proximal to our body, since “the devices with which we communicate have moved from the desktop (on our desks) to the laptop (in our bags) to the smartphone (in our pockets), and are now appearing on our wrists” (Valkenburg & Piotrowski, Citation2017, p. 261). This ubiquity and proximity of social media apps, and the idea of permanent connectedness may have blurred the lines between attention to the task at hand and attention to social media interferences.
While, overall, our results showed that adolescents felt more distracted when they spent more time on social media, the wide range in person-specific effect sizes (β = −.15 to β = +.46) indicates that there is substantial heterogeneity across adolescents. More specifically, the vast majority of all adolescents (82.5%) experienced more distraction when they spent more time using social media, a smaller group of adolescents (15.7%) experienced no differences in distraction, and only a few adolescents (1.8%) even experienced less distraction. This evidence for heterogeneity aligns with recent results yielding heterogeneity in the effects of SMU on well-being (Beyens, Pouwels, van Driel, Keijsers, & Valkenburg, Citation2020a; Rodriguez et al., Citation2021; Valkenburg et al., Citation2021b), friendship closeness (Pouwels et al., Citation2021), and self-esteem (Valkenburg et al., Citation2021a). However, while the majority of participants in these studies experienced no effect, most participants in the current study experienced increased distraction when spending more time on social media. This predominance of positive person-specific associations was also found in recent work investigating the effects of social media on procrastination (Aalbers, vanden Abeele, Hendrickson, De Marez, & Keijsers, Citation2021). As such, it seems that different degrees of heterogeneity exist in the effects of SMU on different outcomes: Whereas most adolescents hardly experience changes in well-being, self-esteem, or friendship closeness when their SMU increases, most adolescents do experience more distraction and procrastination when their SMU increases.
Cross-level interactions showed that individual differences in adolescents’ average level of SMU, but not in their average level of distraction, could explain part of this heterogeneity. More specifically, the positive momentary association between SMU and distraction was less pronounced for adolescents who, on average, spent more time using social media than adolescents who spent less time using social media. This finding could indicate that heavier social media users are more accustomed to switching attention to social media notifications and alerts (Karpinski, Kirschner, Ozer, Mellott, & Ochwo, Citation2013), and less prone to feel distracted when they spend more time using social media. At the same time, it is also possible that heavier users are less aware that they are distracted or less inclined to admit that they feel distracted.
To further investigate what factors explain the heterogeneity in the association of SMU and distraction, Bolger, Zee, Rossignac-Milon, and Hassin (Citation2019) proposed to focus on the degree of stability of the heterogeneity, that is, whether person-specific associations of SMU with distraction remain consistent over time spans such as weeks, months, or even years. Such knowledge can provide guidance for introducing relevant moderators. More specifically, if the heterogeneity of the person-specific associations between SMU and distraction is stable, the heterogeneity may be explained by factors that are also relatively stable over time. In this case, trait-like characteristics, such as gender (Throuvala et al., Citation2021), trait levels of fear of missing out (Franchina, Vanden Abeele, van Rooij, Lo Coco, & De Marez, Citation2018), parental control (Fardouly, Magson, Johnco, Oar, & Rapee, Citation2018), or proactive self-control strategies (Brevers & Turel, Citation2019) are likely to moderate the effects of social media use on distraction. Conversely, if the heterogeneity appears to be unstable across time, it is more likely that the heterogeneity in person-specific associations is explained by situational factors, such as mindfulness (Chin et al., Citation2020), exhaustion (Reinecke & Hofmann, Citation2016), sleep quality (Baumeister, Wright, & Carreon, Citation2019; Roca et al., Citation2012), or other factors that may fluctuate across different weeks, months, or years.
By investigating the reciprocal effects between SMU and distraction, we found that the effect of SMU on distraction is somewhat stronger than the reversed effect, although the difference in strength was very small. This suggests that SMU leads to reduced attentional control, making it more difficult for adolescents to sustain attention and suppress distractions. One reasonable explanation for this directional effect is that SMU evokes “task-irrelevant thoughts” that persist beyond the actual time spent on social media (Stothart et al., Citation2015). For instance, after sending a message on WhatsApp or updating a story on Instagram, adolescents might think about replies, comments, or likes to such extent that they fail to focus their attention on subsequent tasks.
Avenues for Future Research
To move the field forward, we propose four directions for future research. First, future studies should further investigate the longitudinal association of SMU and distraction across different time intervals. This may help to better understand the direction and timing of the effect, and it may provide insight into whether an adolescent is likely to end up in a reinforcing spiral over time (Slater, Citation2007; Valkenburg & Peter, Citation2013). While the time lag between two measurements in the current study was two hours, the effects likely develop at a shorter rate (e.g., within minutes or seconds) since the momentary associations were much stronger than the lagged causal effects. In addition, it may be interesting to examine how such short-term effects are related to the development of attentional control in the long run, given that adolescents’ long-term developments may unfold from short-term effects (e.g., Smith & Thelen, Citation2003). When knowledge about the direction, timing, and the long-term development of the effect is combined with the idiographic approach, interventions can be created that address the fundamental cause for those adolescents who need it.
A second avenue for future research is to unravel why SMU is associated with distraction. For instance, it could be that adolescents experienced more distractions because they were distracted by social media, for instance, because of disturbing factors from their smartphones (e.g., notifications, beeps, and sounds) or because of internal thoughts related to social connectedness (Johannes et al., Citation2019; Stothart et al., Citation2015). In addition, it could be that, due to the constant availability of social media, adolescents may have developed a processing style characterized by scattered attention, or so-called “breadth biased” attentional control (Baumgartner & Sumter, Citation2017; Baumgartner, van der Schuur, Lemmens, & te Poel, Citation2018; Ophir, Nass, & Wagner, Citation2009; Ralph, Thomson, Cheyne, & Smilek, Citation2014; van der Schuur, Baumgartner, Sumter, & Valkenburg, Citation2015). Therefore, adolescents with a more scattered processing style may have more difficulties in filtering out irrelevant environmental stimuli. This may explain why heavier social media users experience more distractions and difficulties focusing attention than their peers.
A third avenue for future research is to examine how fragmented use is associated with distraction. Since the experience of “being distracted” denotes a switch in attention from the task at hand toward irrelevant stimuli, the frequency of using social media might be more indicative of attentional control failures than the time spent on social media. In other words, the co-fluctuations that we found in the current study might have been even more pronounced when participants were asked to report how often they checked social media apps over the past hour. For instance, checking social media apps 20 times for only 30 s might be more intrusive and distracting than spending ten consecutive minutes on social media. Research is needed to understand the differential effects of the time spent on social media versus the fragmented use of social media on distraction.
A final avenue for future research is to investigate the consequences of social media-induced distractions. For instance, maintaining control over one’s online connectivity has been recognized as an important condition for achieving digital well-being, that is, a healthy balance between connectivity and disconnectivity (Vanden Abeele, Citation2020). As such, being distracted by social media may undermine this balance. In addition, social media-induced distractions may affect adolescents’ well-being more generally, as a recent study showed that having more thoughts about social media messages was associated with decreased affective well-being (Johannes et al., Citation2020). Moreover, studies have repeatedly shown that social media-induced distractions have a negative impact on goal attainment, such as academic achievement (Fox et al., Citation2009; Marker, Gnambs, & Appel, Citation2018). Future research is needed that investigates how and to what extent social media-induced distractions affect digital well-being, general well-being, and goal attainment.
Conclusion
Digital technology is growing rapidly these days, making social media omnipresent in daily lives. This poses a serious challenge for adolescents as they are in a crucial period for developing attentional control abilities, and at the same time, have social media at their disposal that may distract them numerous times a day. We found that moments of increased SMU were accompanied by increases in distraction and that this applied to the vast majority of all adolescents. Therefore, this study served as an initial step toward understanding the association between SMU and distraction in daily life, and future studies are encouraged to paint a more refined picture of the dynamics over time.
Open Scholarship
This article has earned the Center for Open Science badges for Open Data, Open Materials and Preregistered. The data are openly accessible at https://doi.org/10.21942/uva.14199134.v1; materials are openly accessible at https://osf.io/cszk7/; registration of study design and sampling plan of the larger project are openly accessible at https://osf.io/jfzrw; registration of the hypotheses and analysis plan are openly accessible at https://osf.io/xszta.
Acknowledgments
We would like to thank Irene van Driel and Tim Verbeij for their contribution to the data collection of this study.
Disclosure Statement
No potential conflict of interest was reported by the author(s).
Data availability statement
The data described in this article are openly available in the Open Science Framework at https://doi.org/10.21942/uva.14199134.v1.
Additional information
Funding
References
- Aalbers, G., McNally, R. J., Heeren, A., de Wit, S., & Fried, E. I. (2019). Social media and depression symptoms: A network perspective. Journal of Experimental Psychology, 148(8), 1454–1462. doi:10.1037/xge0000528
- Aalbers, G., vanden Abeele, M. M., Hendrickson, A. T., de Marez, L., & Keijsers, L. (2021). Caught in the moment: Are there person-specific associations between momentary procrastination and passively measured smartphone use? Mobile Media & Communication, 2050157921993896. doi:10.1177/2050157921993896
- Adachi, P., & Willoughby, T. (2015). Interpreting effect sizes when controlling for stability effects in longitudinal autoregressive models: Implications for psychological science. European Journal of Developmental Psychology, 12(1), 116–128. doi:10.1080/17405629.2014.963549
- Alloway, T. P., & Alloway, R. G. (2012). The impact of engagement with social networking sites (SNSs) on cognitive skills. Computers in Human Behavior, 28(5), 1748–1754. doi:10.1016/j.chb.2012.04.015
- Anderson, M., & Jiang, J. (2018). Teens social media & technology 2018. Retrieved from https://www.pewresearch.org/internet/2018/05/31/teens-social-media-technology-2018/
- Asparouhov, T., Hamaker, E. L., & Muthén, B. (2018). Dynamic structural equation models. Structural Equation Modeling: A Multidisciplinary Journal, 25(3), 359–388. doi:10.1080/10705511.2017.1406803
- Baumeister, R. F., Wright, B. R., & Carreon, D. (2019). Self-control “in the wild”: Experience sampling study of trait and state self-regulation. Self and Identity, 18(5), 494–528. doi:10.1080/15298868.2018.1478324
- Baumgartner, S. E., & Sumter, S. R. (2017). Dealing with media distractions: An observational study of computer-based multitasking among children and adults in the Netherlands. Journal of Children and Media, 11(3), 295–313. doi:10.1080/17482798.2017.1304971
- Baumgartner, S. E., van der Schuur, W. A., Lemmens, J. S., & te Poel, F. (2018). The relationship between media multitasking and attention problems in adolescents: Results of two longitudinal studies. Human Communication Research, 44(1), 3–30. doi:10.1111/hcre.12111
- Bayer, J. B., Campbell, S. W., & Ling, R. (2016). Connection cues: Activating the norms and habits of social connectedness. Communication Theory, 26(2), 128–149. doi:10.1111/comt.12090
- Beyens, I., Pouwels, J. L., van Driel, I. I., Keijsers, L., & Valkenburg, P. M. (2020a). The effect of social media on well-being differs from adolescent to adolescent. Scientific Reports, 10, 10763. doi:10.1038/s41598-020-67727-7
- Beyens, I., Pouwels, J. L., van Driel, I. I., Keijsers, L., & Valkenburg, P. M. (2020b). The effect of social media on well-being differs from adolescent to adolescent. Scientific Reports, 10(1), 1–11. doi:10.1038/s41598-020-67727-7
- Bolger, N., Zee, K., Rossignac-Milon, M., & Hassin, R. (2019, April 1). Causal processes in psychology are heterogeneous. Journal of Experimental Psychology: General, 148, 601–618. doi:10.1037/xge0000558
- Borca, G., Bina, M., Keller, P. S., Gilbert, L. R., & Begotti, T. (2015). Internet use and developmental tasks: Adolescents’ point of view. Computers in Human Behavior, 52, 49–58. doi:10.1016/j.chb.2015.05.029
- Brevers, D., & Turel, O. (2019). Strategies for self-controlling social media use: Classification and role in preventing social media addiction symptoms. Journal of Behavioral Addictions, 8(3), 554–563. doi:10.1556/2006.8.2019.49
- Brooks, S. (2015). Does personal social media usage affect efficiency and well-being? Computers in Human Behavior, 46, 26–37. doi:10.1016/j.chb.2014.12.053
- Chang, W., Cheng, J., Allaire, J., Xie, Y., & McPherson, J. (2020). shiny: Web application framework for R (R package version 1.4.0.2). Retrieved from https://CRAN.R-project.org/package=shiny
- Chin, B., Lindsay, E. K., Greco, C. M., Brown, K. W., Smyth, J. M., Wright, A. G., & Creswell, J. D. (2020). Mindfulness interventions improve momentary and trait measures of attentional control: Evidence from a randomized controlled trial. Journal of Experimental Psychology: General. doi:10.1037/xge0000969
- Cohen Kadosh, K., Heathcote, L. C., & Lau, J. Y. (2014). Age-related changes in attentional control across adolescence: How does this impact emotion regulation capacities? Frontiers in Psychology, 5, 111. doi:10.3389/fpsyg.2014.00111
- Diamond, A. (2013). Executive functions. Annual Review of Psychology, 64, 135–168. doi:10.1146/annurev-psych-113011-143750
- Fardouly, J., Magson, N. R., Johnco, C. J., Oar, E. L., & Rapee, R. M. (2018, July 1). Parental control of the time preadolescents spend on social media: Links with preadolescents’ social media appearance comparisons and mental health. Journal of Youth and Adolescence, 47(7), 1456–1468. doi:10.1007/s10964-018-0870-1
- Fleeson, W. (2001). Toward a structure-and process-integrated view of personality: Traits as density distributions of states. Journal of Personality and Social Psychology, 80(6), 1011. doi:10.1037/0022-3514.80.6.1011
- Fox, A. B., Rosen, J., & Crawford, M. (2009). Distractions, distractions: Does instant messaging affect college students’ performance on a concurrent reading comprehension task? CyberPsychology & Behavior, 12(1), 51–53. doi:10.1089/cpb.2008.0107
- Franchina, V., Vanden Abeele, M., van Rooij, A. J., Lo Coco, G., & De Marez, L. (2018). Fear of missing out as a predictor of problematic social media use and phubbing behavior among Flemish adolescents. International Journal of Environmental Research and Public Health, 15(10), 1–18. doi:10.3390/ijerph15102319
- Gignac, G. E., & Szodorai, E. T. (2016, November 1). Effect size guidelines for individual differences researchers. Personality and Individual Differences, 102, 74–78. doi:10.1016/j.paid.2016.06.069
- Higgins, A. T., & Turnure, J. E. (1984). Distractibility and concentration of attention in children’s development. Child Development, 55, 1799–1810. doi:10.2307/1129927
- Irons, J. L., & Leber, A. B. (2020). Developing an individual profile of attentional control strategy. Current Directions in Psychological Science, 29(4), 364–371. doi:10.1177/096372142092401
- Johannes, N., Meier, A., Reinecke, L., Ehlert, S., Setiawan, D. N., Walasek, N., … Veling, H. (2020). The relationship between online vigilance and affective well-being in everyday life: Combining smartphone logging with experience sampling. Media Psychology, 1–25. doi:10.1080/15213269.2020.1768122
- Johannes, N., Veling, H., Verwijmeren, T., & Buijzen, M. (2019). Hard to resist? The effect of smartphone visibility and notifications on response inhibition. Journal of Media Psychology: Theories, Methods, and Applications, 31(4), 214. doi:10.1027/1864-1105/a000248
- Karpinski, A. C., Kirschner, P. A., Ozer, I., Mellott, J. A., & Ochwo, P. (2013). An exploration of social networking site use, multitasking, and academic performance among United States and European university students. Computers in Human Behavior, 29(3), 1182–1192. doi:10.1016/j.chb.2012.10.011
- Kushlev, K., Proulx, J., & Dunn, E. W. (2016). “Silence your phones” smartphone notifications increase inattention and hyperactivity symptoms. In Proceedings of the 2016 CHI conference on human factors in computing systems, San Jose California USA.
- Lerner, R. M., Lerner, J. V., & Chase, P. A. (2019). Toward enhancing the role of idiographic‐based analyses in describing, explaining, and optimizing the study of human development: The sample case of adolescent ⟷ family relationships. Journal of Family Theory & Review, 11(4), 495–509. doi:10.1111/jftr.12347
- Levine, L. E., Waite, B. M., & Bowman, L. L. (2007). Electronic media use, reading, and academic distractibility in college youth. CyberPsychology & Behavior, 10(4), 560–566. doi:10.1089/cpb.2007.9990
- Luna, B. (2009). Developmental changes in cognitive control through adolescence. Advances in Child Development and Behavior, 37, 233–278.
- Luna, B., Garver, K. E., Urban, T. A., Lazar, N. A., & Sweeney, J. A. (2004). Maturation of cognitive processes from late childhood to adulthood. Child Development, 75(5), 1357–1372. doi:10.1111/j.1467-8624.2004.00745.x
- Mark, G., Iqbal, S., & Czerwinski, M. (2017). How blocking distractions affects workplace focus and productivity. In Proceedings of the 2017 ACM International Joint Conference on Pervasive and Ubiquitous Computing and Proceedings of the 2017 ACM International Symposium on Wearable Computers, Maui Hawaii.
- Marker, C., Gnambs, T., & Appel, M. (2018). Active on Facebook and failing at school? Meta-analytic findings on the relationship between online social networking activities and academic achievement. Educational Psychology Review, 30, 651–677. doi:10.1007/s10648-017-9430-6
- McCoy, B. R. (2016). Digital distractions in the classroom phase II: Student classroom use of digital devices for non-class related purposes. Journal of Media Education, 7(1), 5 - 32.
- McNeish, D., & Hamaker, E. L. (2020). A primer on two-level dynamic structural equation models for intensive longitudinal data in Mplus. Psychological Methods, 25(5), 610–635. doi:10.1037/met0000250
- Meeus, A., Eggermont, S., & Beullens, K. (2018). Constantly connected: The role of parental mediation styles and self-regulation in pre- and early adolescents’ problematic mobile device use. Human Communication Research. doi:10.1093/hcr/hqy015
- Molenaar, P. C. M., & Campbell, C. G. (2009). The new person-specific paradigm in psychology. Current Directions in Psychological Science, 18(2), 112–117. doi:10.1111/j.1467-8721.2009.01619.x
- Muthén, B. (2010). Bayesian analysis in Mplus: A brief introduction (Unpublished manuscript). Retrieved from http://www.statmodel.com/download/IntroBayesVersion%201.pdf.
- Muthén, L. K., & Muthén, B. O. (1998-2017). Mplus User’s Guide. Eighth Edition. Los Angeles, CA: Muthén & Muthén.
- Odgers, C. L., & Jensen, M. R. (2020, January 17). Annual research review: Adolescent mental health in the digital age: Facts, fears, and future directions. Journal of Child Psychology and Psychiatry, 61, 336–348. doi:10.1111/jcpp.13190
- Ophir, E., Nass, C., & Wagner, A. D. (2009). Cognitive control in media multitaskers. Proceedings of the National Academy of Sciences, 106(37), 15583–15587. doi:10.1073/pnas.0903620106
- Pouwels, J. L., Valkenburg, P. M., Beyens, I., van Driel, I. I., & Keijsers, L. (2021). Social media use and friendship closeness in adolescents’ daily lives: An experience sampling study. Developmental Psychology, 57(2), 309–323. doi:10.1037/dev0001148
- R Core Team. (2020). R: A language and environment for statistical computing. R Foundation for Statistical Computing. Retrieved from https://www.R-project.org/
- Ralph, B. C., Thomson, D. R., Cheyne, J. A., & Smilek, D. (2014). Media multitasking and failures of attention in everyday life. Psychological Research, 78(5), 661–669. doi:10.1007/s00426-013-0523-7
- Reinecke, L., & Hofmann, W. (2016). Slacking off or winding down? An experience sampling study on the drivers and consequences of media use for recovery versus procrastination. Human Communication Research, 42(3), 441–461. doi:10.1111/hcre.12082
- Reinecke, L., Klimmt, C., Meier, A., Reich, S., Hefner, D., Knop-Huelss, K., … Vorderer, P. (2018). Permanently online and permanently connected: Development and validation of the Online Vigilance Scale. PloS One, 13(10), e0205384. doi:10.1371/journal.pone.0205384
- Revelle, W. (2020). psych: Procedures for psychological, psychometric, and personality research (R package version 2.0.12). Northwestern University. Retrieved from https://CRAN.R-project.org/package=psych
- Rideout, V., & Fox, S. (2018). Digital health practices, social media use, and mental well-being among teens and young adults in the US. Retrieved from https://www.commonsensemedia.org/
- Riley, E., Esterman, M., Fortenbaugh, F. C., & DeGutis, J. (2017). Time-of-day variation in sustained attentional control. Chronobiology International, 34(7), 993–1001. doi:10.1080/07420528.2017.1308951
- Roca, J., Fuentes, L. J., Marotta, A., López-Ramón, M.-F., Castro, C., Lupiáñez, J., & Martella, D. (2012). The effects of sleep deprivation on the attentional functions and vigilance. Acta psychologica, 140(2), 164–176. doi:10.1016/j.actpsy.2012.03.007
- Rodriguez, M., Aalbers, G., & McNally, R. J. (2021). Idiographic network models of social media use and depression symptoms. PsyArXiv. doi:10.31234/osf.io/t4hc3
- Russell, M. A., & Gajos, J. M. (2020). Annual research review: Ecological momentary assessment studies in child psychology and psychiatry. Journal of Child Psychology and Psychiatry, 61(3), 376–394. doi:10.1111/jcpp.13204
- Schultzberg, M., & Muthén, B. (2018). Number of subjects and time points needed for multilevel time-series analysis: A simulation study of dynamic structural equation modeling. Structural Equation Modeling: A Multidisciplinary Journal, 25(4), 495–515. doi:10.1080/10705511.2017.1392862
- Schuurman, N. K., Ferrer, E., de Boer-Sonnenschein, M., & Hamaker, E. L. (2016). How to compare cross-lagged associations in a multilevel autoregressive model. Psychological Methods, 21(2), 206. doi:10.1037/met0000062
- Siebers, T., Beyens, I., Pouwels, J. L., & Valkenburg, P. M. (2021). Data set belonging to Siebers et al. (2021). Social media and distraction: An experience sampling study among adolescents [Data set]. University of Amsterdam/Amsterdam University of Applied Sciences. doi:10.21942/uva.14199134.v1
- Slater, M. D. (2007). Reinforcing spirals: The mutual influence of media selectivity and media effects and their impact on individual behavior and social identity. Communication Theory, 17(3), 281–303. doi:10.1111/j.1468-2885.2007.00296.x
- Smith, L. B., & Thelen, E. (2003). Development as a dynamic system. Trends in Cognitive Sciences, 7(8), 343–348. doi:10.1016/s1364-6613(03)00156-6
- Stawarczyk, D., Majerus, S., Catale, C., & D’Argembeau, A. (2014). Relationships between mind-wandering and attentional control abilities in young adults and adolescents. Acta psychologica, 148, 25–36. doi:10.1016/j.actpsy.2014.01.007
- Stothart, C., Mitchum, A., & Yehnert, C. (2015). The attentional cost of receiving a cell phone notification. Journal of Experimental Psychology: Human Perception and Performance, 41(4), 893–897. doi:10.1037/xhp0000100.supp
- Tavares, D., & Freire, T. (2016). Flow experience, attentional control, and emotion regulation: Contributions for a positive development in adolescents. Psicologia: Revista da Associacao Portuguesa Psicologia, 30(2), 77–94. doi:10.17575/rpsicol.v30i2.1119
- Thornton, B., Faires, A., Robbins, M., & Rollins, E. (2014). The mere presence of a cell phone may be distracting: Implications for attention and task performance. Social Psychology, 45(6), 479. doi:10.1027/1864-9335/a000216
- Throuvala, M. A., Pontes, H. M., Tsaousis, I., Griffiths, M. D., Rennoldson, M., & Kuss, D. J. (2021). Exploring the dimensions of smartphone distraction: Development, validation, measurement invariance, and latent mean differences of the Smartphone Distraction Scale (SDS). Frontiers in Psychiatry, 12, 199. doi:10.3389/fpsyt.2021.642634
- Valkenburg, P. M., Beyens, I., Pouwels, J. L., van Driel, I. I., & Keijsers, L. (2021a). Social media and adolescents’ self-esteem: Heading for a person-specific media effects paradigm. Journal of Communication, 71(1), 56–78. doi:10.1093/joc/jqaa/039
- Valkenburg, P. M., Beyens, I., Pouwels, J. L., van Driel, I. I., & Keijsers, L. (2021b). Social media browsing and adolescent well-being: Challenging the Passive Social Media Use Hypothesis. PsyArXiv. doi:10.31234/osf.io/gzu3y
- Valkenburg, P. M., & Peter, J. (2013). The differential susceptibility to media effects model. Journal of Communication, 63(2), 221–243. doi:10.1111/jcom.12024
- Valkenburg, P. M., & Piotrowski, J. T. (2017). Plugged in: How media attract and affect youth. New Haven, NJ, Yale University Press.
- Van de Schoot, R., Kaplan, D., Denissen, J., Asendorpf, J. B., Neyer, F. J., & Van Aken, M. A. (2014). A gentle introduction to Bayesian analysis: Applications to developmental research. Child Development, 85(3), 842–860. doi:10.1111/cdev.12169
- van der Schuur, W., Baumgartner, S. E., Sumter, S. R., & Valkenburg, P. M. (2015). The consequences of media multitasking for youth: A review. Computers in Human Behavior, 53, 204–215. doi:10.1016/j.chb.2015.06.035
- van Driel, I. I., Pouwels, J. L., Beyens, I., Keijsers, L., & Valkenburg, P. M. (2019). Posting, scrolling, chatting, and snapping: Youth (14-15) and social media in 2019. Center for Research on Children, Adolescents, and the Media (CcaM), Universiteit van Amsterdam.
- Van Gog, T., Kirschner, F., Kester, L., & Paas, F. (2012). Timing and frequency of mental effort measurement: Evidence in favour of repeated measures. Applied Cognitive Psychology, 26(6), 833–839. doi:10.1002/acp.2883
- van Roekel, E., Keijsers, L., & Chung, J. M. (2019). A review of current ambulatory assessment studies in adolescent samples and practical recommendations. Journal of Research on Adolescence, 29(3), 560–577. doi:10.1111/jora.12471
- Vanden Abeele, M. M. P. (2020). Digital wellbeing as a dynamic construct. Communication Theory. doi:10.1093/ct/qtaa024
- Vasilev, M. R., Kirkby, J. A., & Angele, B. (2018). Auditory distraction during reading: A Bayesian meta-analysis of a continuing controversy. Perspectives on Psychological Science, 13(5), 567–597. doi:10.1177/1745691617747398
- Voelkle, M. C., Oud, J. H. L., von Oertzen, T., & Lindenberger, U. (2012). Maximum likelihood dynamic factor modeling for arbitrary N and T using SEM. Structural Equation Modeling: A Multidisciplinary Journal, 19(3), 329–350. doi:10.1080/10705511.2012.687656
- Wang, L. P., & Maxwell, S. E. (2015, March). On disaggregating between-person and within-person effects with longitudinal data using multilevel models. Psychological Methods, 20(1), 63–83. doi:10.1037/met0000030
- Xie, J.-Q., Rost, D. H., Wang, F.-X., Wang, J.-L., & Monk, R. L. (2021). The association between excessive social media use and distraction: An eye movement tracking study. Information & Management, 58(2), 103415. doi:10.1016/j.im.2020.103415