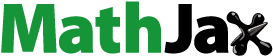
ABSTRACT
Permafrost plays an important role in numerous environmental processes at high latitudes and in high mountain areas. The identification of mountain permafrost, particularly in the discontinuous permafrost regions, is challenging due to limited data availability and the high spatial variability of controlling factors. This study focuses on mountain permafrost in a data-scarce environment of northern Mongolia, at the interface between the boreal forest and the dry steppe mid-latitudes. In this region, the ground temperature has been increasing continuously since 2011 and has a high spatial variability due to the distribution of incoming solar radiation, as well as seasonal snow and vegetation cover. We analyzed the effect of these controlling factors to understand the climate–permafrost relationship based on in situ observations. Furthermore, mean ground surface temperature (MGST) was calculated at 30-m resolution to predict permafrost distribution. The calculated MGST, with a root mean square error of ±1.4°C, shows permafrost occurrence on north-facing slopes and at higher elevations and absence on south-facing slopes. Borehole temperature data indicate a serious wildfire-induced permafrost degradation in the region; therefore, special attention should be paid to further investigations on ecosystem resilience and climate change mitigation in this region.
Introduction
Both simulations and field investigations at different locations in the Northern Hemisphere report the intensification of enhanced warming and its effects on high- and mid-latitude permafrost and frozen ground dynamics (Pepin et al. Citation2015; Biskaborn et al. Citation2019). Changes in frozen ground have important impacts on the surface energy balance, hydrological cycle, and carbon exchange between the atmosphere and ground surface (Zhang et al. Citation2005). Therefore, the response of permafrost to climate change is of major concern, but present knowledge is still limited, especially in remote areas where long-term environmental monitoring is scarce (Azócar, Brenning, and Bodin Citation2017). In Mongolia, the mean annual air temperature has increased by 2.07°C since 1940, with higher intensification over the mountainous regions (Ministry of Environment and Green Development Citation2014). Such an amplified warming has been found to increase the active layer thickness, eventually leading to permafrost degradation in northern Mongolia (Ishikawa et al. Citation2018).
Seasonal freezing and thawing total degree days of air and ground surface (FDDa/s and TDDa/s) are important factors that reflect changes in the cryosphere (Nelson Citation2003). These factors have been used to predict seasonally frozen ground and permafrost distribution at regional and global scales (Pepin et al. Citation2015; Obu et al. Citation2019). However, for smaller geographic scales, including northern Mongolia, the model outputs have been less accurate due to a lack of precise data sets, such as vegetation and snow cover and microclimatic conditions (Riseborough et al. Citation2008; Hasler et al. Citation2015). In mountainous terrain, air temperature, surface characteristics, and radiation balance are major factors in the variability of surface net energy balance (Williams and Smith Citation1989), as well as the extent of mountain permafrost, especially in semi-arid regions. Several studies have been conducted in the Khuvsgul Mountains of northern Mongolia regarding the distribution of mean ground surface temperature (MGST) to predict the permafrost distribution (Etzelmüller et al. Citation2006), active layer thickness (Heggem et al. Citation2006), and thawing n-factor (the ratio between air temperature [Ta] and surface temperature [Ts] during the thawing season) in different land covers (Anarmaa et al. Citation2007).
In regions with rugged topography, such as Sugnugur catchment in the Khentii Mountains, the distribution and dynamics of permafrost have not been well documented. Permafrost in this catchment plays an important role in the hydrological cycle and acts as an impermeable layer allowing lateral subsurface flow that sustains river discharge during the dry seasons (Kopp, Lange, and Menzel Citation2016). Therefore, it is important to assess the distribution of permafrost with a high spatial resolution. Negative MGST is a good indicator of permafrost presence over mountain terrains where the thermal properties of soil can differ significantly (Etzelmüller et al. Citation2006; Luo, Jin, and Bense Citation2019).
This study focuses on understanding the effects of snow and vegetation cover, potential solar radiation (PSR), and topography on the spatial variability of Ts and calculating MGST over the Sugnugur catchment to predict permafrost distribution with a 30-m resolution. The calculated MGST is validated against observed in situ Ts measurements and borehole data. The results contribute to a better understanding of the temporal and spatial variability of Ts and permafrost occurrence in this rugged mountainous terrain of northern Mongolia.
Study area
The study area is the Sugnugur catchment, which is situated in the discontinuous permafrost region of northern Mongolia at the southern boundary of Eurasian permafrost (). Thus, it includes the sensitive transition zone between the steppe and the boreal forest (Kopp, Lange, and Menzel Citation2016). This catchment generates an essential headwater stream of Kharaa River, which eventually drains into Lake Baikal through Selenga River (Menzel, Hofmann, and Ibisch Citation2011). Therefore, the seasonal frozen ground and permafrost in the catchment are of ecological and socioeconomic importance for the approximately 147,000 inhabitants living in the Kharaa River basin (Karthe et al. Citation2014).
Figure 1. Location of the study area, its topography, and the positions of temperature measurement transects across the Sugnugur Valley (indicated by T1–T4; see details in ). The inset shows the PPI (at 1-km resolution), which indicates the likelihood of permafrost existence at a global scale (Obu et al. Citation2019). The hydroclimatic station (green dot) is situated at 1,193 m.a.s.l. BH = the position of the two boreholes located on the north-facing slope near transect 3
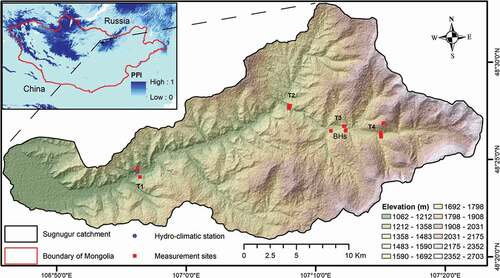
The elevation of the catchment ranges from 1,062 m.a.s.l. to 2,703 m.a.s.l. at the peak of the Khentii Mountains. Sugnugur catchment has rugged topography, with steep south-facing and relatively gentle north-facing slopes. Mean air temperature (MAT) for the last five years is slightly lower than −1°C and mean annual precipitation is about 350 mm (Kopp, Minderlein, and Menzel Citation2014). In the study area, north-facing slopes are covered by boreal forest with thick moss layers. South-facing slopes are sparsely vegetated, and the valley bottom is dominated by shrubs and short grasses. Since 2009, about one third of the forest cover has been affected by wildfires (Kopp, Lange, and Menzel Citation2016). Permafrost in northern Mongolia mostly exists on north-facing slopes and is absent on south-facing slopes (Etzelmüller et al. Citation2006; Ishikawa et al. Citation2008). Depending on the climatic conditions and surface characteristics, it can also sometimes be found in the valley bottoms. Distinct hydrological behaviors of runoff generation processes on burned (quick flow predominant) and unburned (preferential flow predominant) north-facing slopes are evident in this catchment (Lange et al. Citation2015). The soil in the shrub-dominated bottom of the valley has relatively high moisture contents throughout the summer months due to interflow from the north-facing slopes (Minderlein and Menzel Citation2014).
Data and methodology
Data
In situ measurements
Ongoing hydroclimatic observations have been monitored since 2010 at the bottom of the Sugnugur Valley on a permanent monitoring site (referred to as the hydroclimatic station site in this article; ). More than forty parameters are continuously measured with high temporal resolutions, including the individual radiation components, snow depth, Ts, and Ta (for a detailed list of sensors and parameters, see Minderlein and Menzel Citation2014). Before our monitoring program started, the study region was lacking any environmental data. We calculated the summer temperature lapse rate (LR) for summers 2011 and 2012 using daily mean Ta data from three temporary climatic stations distributed along the Sugnugur Valley and the permanent hydroclimatic station. Ta was also measured at four locations for 2016–2017 (including complete thawing Ta > 0°C and freezing Ta < 0°C seasons) using temperature measured from iButtons (Maxim integrated) with ±0.25°C accuracy (Way and Lewkowicz Citation2018). The iButtons were installed in radiation shields and placed 1.5 m above the surface and recorded temperature at 3-hour intervals. The measurement positions included the bottom of the valley and a mountain summit at 2,020 m.a.s.l. Temperature inversions, which frequently occur during winter, were also studied from the iButton measurements.
We also used iButtons to measure Ts at twelve locations at valley transects, in addition to the hydroclimatic station. Measurements were taken at a depth of 5 cm in diverse surface and topographic settings. Two iButtons were operated in parallel at each location in order to prevent any data loss. iButton measurements were evaluated using the Ts record with that of a Campbell 107 temperature probe installed at the same depth at the hydroclimatic station site. The iButtons provided slightly higher temperatures with regard to the standard probe, possibly as a result of a greenhouse effect created by the plastic shelters in which the iButtons were housed. The overall Pearson’s correlation coefficient between the two separate records was r = 0.997, allowing us to calibrate the iButton data with the Campbell 107 temperature probe using a linear regression.
In addition, two boreholes were dug manually in a severely burned forest (SBF, in December 2015) and in an unburned forest (UBF, in September 2016). The boreholes were each equipped with temperature and moisture sensors at depths ranging from 0 to 290 cm for the SBF site and from 0 to 120 cm for the UBF site. Deeper placement of sensors at the UBF site was not possible because we reached the permafrost table at a depth of 120 cm. Temperature data at 5 cm depth were used as an additional validation of MGST. The relative distance between the boreholes is roughly 600 m and both are located on the same north-facing slope. All temperature sensors were connected with HOBO-U12 data loggers; recordings were made at 4-hour intervals. Both iButton and borehole Ts measurement sites are described in .
Table 1. Descriptions of temperature measurement sites. South-facing slopes with greater inclination receive higher potential potential solar radiation (PSR)
Soil thermal conductivity and biomass measurements were also conducted during the thawing season in September 2017 at all iButton and borehole locations. Soil thermal conductivity was measured at depths of 10, 20, and 40 cm using a Decagon KD2 Pro thermal conductivity meter. Biomass samples were collected from 50 × 50 cm plots and weighed and then dried in an oven at 100°C for approximately 2 hours until the weight stabilized. The late winter accumulated snow depth (ASD) was manually measured during a winter field campaign (in the beginning of March 2017).
Remote sensing data
The spatial distribution of vegetation cover was obtained from the soil-adjusted vegetation index (SAVI; Abramov, Gruber, and Gilichinsky Citation2008; Boeckli et al. Citation2012), which was derived from six Landsat images (2012–2017). These images were taken in either July or August, the months assumed to represent peak vegetation cover (Pan et al. Citation2019). The snow cover area (SCA) over the Sugnugur catchment was derived from forty remote sensing images from Landsat 7 and 8 and Sentinel 2 satellites (Munkhjargal et al. Citation2019). A digital elevation model (DEM) with 30-m spatial resolution from the Advanced Spaceborne Thermal Emission and Reflection Radiometer (ASTER GDEM 2) was used to derive the spatial variability in Ta.
Methodology
This section describes the different processes in the framework of the applied methodology (). First, temperature regime and seasonal n-factors were calculated to understand the permafrost dynamics and the effect of snow and vegetation cover on Ts variability (Lunardini Citation1978; Smith and Riseborough Citation1996). We then calculated the spatial variability of MGST for 2016–2017, assuming that negative values can indicate the presence of permafrost. MAT for 2016–2017 was derived from the distribution of PSR and annual LR. Surface offset (SO), which is the difference between MAT and MGST, was calculated from in situ temperature measurements in various topographic settings with seasonal snow and vegetation cover and then added to the MAT to obtain MGST based on the derived SCA and SAVI. To reduce unreasonably high or low values, focal statistics were used for smoothing by averaging the values of the surrounding 3 × 3 pixels. The final MGST was validated using iButton surface temperature measurements at twelve locations and temperature data from the two boreholes near transect 3 () and were also compared to the recently published permafrost probability index (PPI) map by Obu et al. (Citation2019). The equations, parameters, and abbreviations are shown in .
Table 2. Parameters used in the article and their calculation
Temperature variability
Temperature regime
To analyze the effects of topography on Ts, we placed iButtons along transects from the south-facing to the north-facing slopes of the valley, including the valley bottom. In total, four transects (see ) were developed at different elevations. The surface characteristics and a description of each temperature measurement site are shown in . The iButtons were positioned at the same elevation on both slopes in each transect to reduce the effect of the adiabatic Ta gradient on Ts. Ta was observed at the bottom of the valley and on the mountain top, above the Ts measurements (). The summer (June–July–August) and winter (December–January–February) LRs were used to derive an annual LR, which was later used as an adjustment factor in the spatial distribution of MAT to 2016–2017. The frost number (FN), which indicates the likelihood of permafrost occurrence, was calculated for all Ta measurement locations (). The FN ranges from 0 to 1, with a threshold of >0.5 indicating the presence of permafrost at a regional or global scale (Heggem et al. Citation2006). However, it does not confirm the existence of permafrost, because this cannot be determined without knowing the effects of snow and vegetation covers and the thermal properties of the soil (e.g., Smith and Riseborough Citation2002). To understand the general temperature regime in this region, the TDDs for six seasons and FDDs for seven seasons were also calculated at the hydroclimatic station site.
Effect of surface cover
Seasonal snow and vegetation cover insulates the heat flow between the atmosphere and ground surface, resulting in higher during winter and cooler Ts during summer compared to Ta. Snow cover decouples the Ts from Ta due to its low thermal conductivity (Ishikawa Citation2003; Harris et al. Citation2009). Using the Ts and Ta measurements at the hydroclimatic station site, we evaluated the interannual variability of the thawing (nt) and freezing (nf) n-factors. Furthermore, the relation between biomass and TDDs was analyzed across the valley transects. The SO was also calculated at four locations where both Ts and Ta exist. The equations can be found in . The seasonally persistent SCA—that is, the snow that uninterruptedly covers the ground—was analyzed by determining the Normalized Difference Snow Index with a value of >0.4, indicating the presence of snow cover, as recommended by Hall et al. (Citation2002). Based on the SAVI, the study area was classified into vegetated and nonvegetated surfaces. To simplify the variability in SO over the study area, it was assumed to be different for the vegetated and persistent snow cover areas as well as the nonvegetated, mostly rock debris surfaces on steep slopes based on the calculated n-factors.
Regionalization of air temperature
In mountainous regions with strong topographic gradients, incoming solar radiation plays a significant role in the spatial distribution of both Ta and Ts. The relationship between PSR and either Ts or Ta has been studied in permafrost regions (Lacelle et al. Citation2016). Ta in heterogeneous terrain mainly depends on topography and other microclimate factors such as cloud cover, precipitation, and surface characteristics (Strachan and Daly Citation2017). Temperature and solar radiation follow similar patterns as they change with time, but variances in temperature lag behind variances in solar radiation. In this study, empirically derived MAT was considered as a good indicator of MGST because Ta usually follows the diurnal variation in Ts due to the reflected radiation from the ground surface (Chou, Sheng, and Zhu Citation2012). Therefore, we analyzed a five-year time series of incoming solar radiation and Ta data recorded at the hydroclimatic station and found that 30 days of lag is the best fit to remove the time lag between the two variables. Similar time lags were also found and used for modeling temperature in previous studies at both local and global scales (Douglass, Blackman, and Knox Citation2004; Huang et al. Citation2008). This suggests that if the study area is assumed to have homogenous atmospheric conditions, Ta can be predicted as a fraction of PSR after a series of adjustments.
Adjustment of Ta includes (1) aggregation of the observed daily values (from transects 2 and 3) to ten-day averages, (2) removal of the variation using discrete Fourier transform (FT), and (3) removal of the thirty-day time lag to improved the correlation with solar radiation. The FT uses two phases, which indicate the absolute values within the annual time series. This results in a smoothing of the Ta by sinusoidal oscillations around the mean value. To meet the time step of the adjusted Ta, PSR was also calculated for a whole year as a ten-day running average using the PSR model using ArcGIS spatial analysis tools (Fu and Rich Citation2002) and the DEM. The calculation is the sum of direct and diffuse radiation repeated for each feature location or every location on the topographic surface. Then, MAT for 2016–2017 was obtained from the calculated PSR using a linear regression analysis and adding the annual LR with the assumption that a clear sky was homogenous over the study area. The equation is provided in .
Results and discussion
Temperature regime
Data from the surface temperature iButtons indicate that the freezing season extends from the beginning of October to the end of March at all observed locations, with prolonged periods below 0°C at higher elevations (Munkhjargal et al. Citation2019). The surface freezing period on north-facing slopes starts a few days earlier and ends later compared to that on south-facing slopes due to extended snow cover, duration of phase change, surface cover, and less incoming radiation. FN values calculated from the observed Ta measurements ranged from 0.5 to 0.6, indicating the potential occurrence of permafrost in the study area (Etzelmüller, Berthling, and Sollid Citation1998). However, MGST is more sensitive to changes in n-factors, solar radiation, and soil moisture (Heggem et al. Citation2006; Juliussen and Humlum Citation2007).
The observed MGST on south-facing slopes was 3–4°C higher than that on north-facing slopes (). The temperature difference between the slopes is more pronounced during the thawing season, especially on hot days with clear skies. Observations of Ta and Ts at the four sites indicate that Ts is initially warmer than Ta at all sites, with notably less fluctuation in winter due to the insulating effect of snow. The difference in Ts between the opposite slopes is the lowest during winter. It increases again in late winter because Ts on the south-facing slope increases rapidly with Ta, whereas Ts on the north-facing slope gradually increases due to the extended snow cover and required latent heat energy to warm the soil during phase change. Therefore, the variability in MGST across the valley is high.
The observed MAT at the hydroclimatic station site was −1.06°C. The study period 2016–2017 was unusually warm with low FDDs and high TDDs due to a thicker snowpack in winter and a dry summer, respectively. Moreover, Ts observation revealed a continuous increase in both thawing and freezing seasons over the last seven years () as representative of the general temperature regime in the study area. Unusual wildfire events occurred in the region in summer 2017 as a result of the extreme dryness and increased temperatures. Thus, the continuously increasing Ts may have contributed to the deepening of the active layer, particularly in areas that were seriously affected by wildfires.
Figure 4. Calculated TDDs and FDDs at the hydroclimatic station. The study period covers the complete thawing and freezing seasons when the iButton measurements were conducted
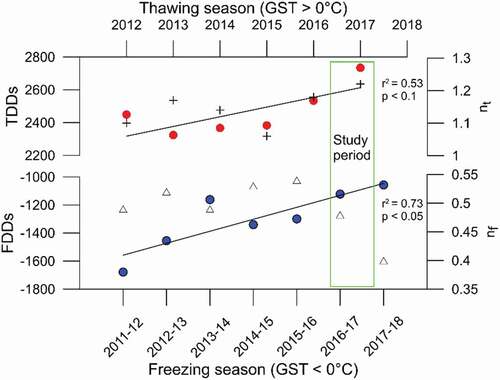
We analyzed the relationship between Ts and Ta at different elevations using linear regression analysis. The correlation coefficient (r2) between the two variables decreased as elevation increased (Figure S1, Supplementary material) and can be attributed to the extended snowpack at higher elevations and to slower temperature changes at the ground surface compared to in the air (Peng et al. Citation2015). Strong seasonal variability in Ta exists in the study area as well, as indicated by the mean summer LR of −0.011°C m−1 (found from the four climate stations) and winter inversion of 0.006°C m−1. Nevertheless, the annual LR was found to be −0.005°C m−1, or similar to the commonly accepted environmental LR of −0.006°C m−1 (Minder, Mote, and Lundquist Citation2010).
Effect of surface covers
Surface covers as insulating materials
To analyze the influence of the snow cover on Ts, the snow depth observed at the hydroclimatic station was examined in conjunction with other corresponding parameters (). The seasonally persistent snow cover lasts about 130–140 days from early November to late March, and its duration is substantially longer at higher elevations. The mean insulating effect of seasonal snow cover on Ts, or nf, was relatively consistent or roughly 0.5 for the last five winters at the hydroclimatic station site ().
Table 3. Mean and maximum snow depth (cm) at the hydroclimatic station site, together with ground surface temperature (Ts, °C), air temperature (Ta, °C), and incoming solar radiation (ISR, Wm−2) in winters since 2012
The development of a seasonal snow cover plays a significant role in the variability in Ts; a gradually weakening insulating effect with increasing snow depth and air temperature toward the end of the freezing season was evident (). During this time, Ts became less sensitive to variations in Ta due to the greater snow depth. The low Ts below the snowpack tended to remain for an extended period as a consequence of the low thermal conductivity of snow restricting the loss of heat from the ground.
Figure 5. Calculated n-factors at each transect during the study period. Values greater than 1.0 indicate lower Ts during the freezing season and higher Ts during the thawing season relative to Ta
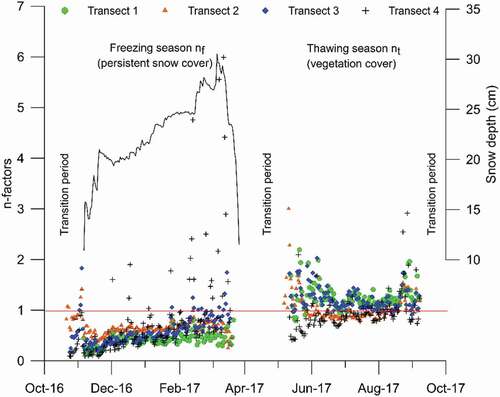
In general, snow depth appears to be independent of elevation based on the field measurements (Table S1, Supplementary material). The percentage of persistent snow cover area throughout winter was 94 percent in the Sugnugur catchment (Munkhjargal et al. Citation2019). We conclude that nf is homogenously 0.5 in the areas with persistent snow cover. Steep south-facing slopes were snow-free most of the time due to prevailing winds and relatively high incoming solar radiation, which resulted in snow redistribution and greater sublimation rates, respectively.
Forested north-facing slopes were the richest in biomass, followed by the valley bottom shrublands and sparsely vegetated south-facing slopes. Near transect 3, for instance, a biomass value of 0.07 kg/m2 on the south-facing slope, 0.825 kg/m2 on the bottom of the valley, and a value of 4.935 kg/m2 under dense forest were found. Notably, the amount of biomass in the SBF was 2.430 kg/m−2, or roughly half that of the UBF; this distinction demonstrates the effects of wildfires on biomass. Yoshikawa et al. (Citation2002) explained the implications of wildfire and reduction in vegetation cover on the ground thermal regime in Interior Alaska. The soil in the SBF showed the highest thermal conductivity due to the reduction in the moss layer and increased water content, which is similar to that found in Interior Alaska (Yoshikawa et al. Citation2002). The reduced vegetation cover resulted in a higher TDDs in the SBF compared to the adjacent UBF.
To evaluate the effect of vegetation cover on Ts, we correlated the sampled dry biomass measurements against the surface TDDs at all Ts measurement sites, except for high-elevation rock debris surfaces (Figure S2, Supplementary material). There was a significant negative relationship between the two variables, indicating that greater biomass protects the underlying surface from summer overheating. The lowest TDDs values were observed at the valley bottom site near transect 4, which is characterized by dense shrubs located near the Sugnugur riverbank. We speculate that this is probably due to increased soil moisture content, which reduces surface heating as a result of an interrelation between surface and subsurface flows from the north-facing slope and the shading effect of the dense shrubs.
N-factors and surface offset
The relationship between surface and air temperatures has not been previously described for the Khentii Mountains. The seasonal snow-induced freezing nf was relatively consistent, ranging between 0.5 and 0.6 at all sites, and thawing nt ranged from 0.8 at the top of the mountain to 1.2 in short grassland at lower elevations (). The nt values exceeding 1.0 indicate that Ts was warmer than Ta. Unlike at other sites, Ts at the rock debris surface was lower than Ta, probably due to high albedo resulting in less net radiation. However, other site characteristics such as canopy closure, dryness, and organic layer thickness can also influence this relationship (Karunaratne and Burn Citation2004). In winter, Ts at all locations was higher than Ta due to the snow insulation effect.
Table 4. Observed surface offset and n-factors at the measurement sites
The period with temperature measurements was not long enough to perform a comprehensive evaluation of the interannual variations in the n-factors. Nevertheless, we believe that seasonal n-factors at different elevations and slopes were identified for this region because the annual variations in the n-factors were relatively stable at the hydroclimatic station. Considering the similar nf and nt factors except for the rock debris surface, we conclude that SO is +4°C for vegetated and persistent snow cover area and +1.5°C for nonvegetated rock debris surface. These results are in line with Boeckli et al. (Citation2012), who assumed that the SO of the vegetated area is 2°C greater than that of nonvegetated rock debris surfaces in the European Alps, further suggesting that our results are reasonable.
The heat exchange between air and surface varied significantly in early spring and late autumn (the transition periods) when nf and nt converge. Therefore, the freezing season n-factors during the transition periods are important components of the overall seasonal nf. The calculated mid-season nf values were closest to the seasonal nf. Like nf, mid-season nt values were closest to the seasonal nt and during that time Ts and Ta were similar (). However, the seasonal nt may depend on the length of the thawing season. illustrates the ratios between daily Ts and Ta in the four transects. Note that calculations were only made for the bottom of the valley and on the mountain top, where both Ta and Ts measurements exist. There were some days with colder Ts than Ta during winter at transects 3 and 4 in the upper valley, which was probably due to the inversion effect.
Anarmaa et al. (Citation2007) studied nt under various vegetation covers in a valley of the Khuvsgul Mountains in northern Mongolia using a Ta measurement from a single location. This would mean that Ta is constant across the valley and that the only influence on Ts is the overlying vegetation. Such calculation may lead to relatively low nt on north-facing slopes and high nt on south-facing slopes. We suggest that Ta can vary at smaller scales depending on both exposure and elevation. Therefore, the variability in Ts across the Sugnugur Valley was considered as a result of the spatial distribution of snow and vegetation cover, incoming solar radiation, topography, and the length of the thawing season.
In addition, we checked the associations between the calculated parameters and MGST at all Ts measurement sites using Pearson’s correlation analysis (). The parameters include the measured snow and biomass, as well as 30-m gridded data including PSR, elevation, and mean land surface temperature (LST) derived from the Landsat images that were used to retrieve SAVI in this study. To avoid high collinearity, the parameters that require Ta measurement were not considered due to (1) the limited number of Ta measurements in the valley and (2) its strong relationship with Ts. The results show that the spatial distribution of MGST might be better explained by the variability in TDDs (r = 0.94, p < .01) rather than FDDs (r = 0.68, p < .05). This indicates that though nf is relatively consistent over the study area, nt plays a major role in the variability in MGST over the Sugnugur catchment. Both the PSR and biomass showed statistically significant correlations with MGST. Due to the high temperature differences across the valley transects during hot summer days, we also tested the relationship between the Landsat-derived LST and the corresponding parameters. These results showed significant negative correlations with biomass and elevation and a positive relationship with TDDs, indicating that the greater the biomass and the higher the elevation, the lower the Ts during the thawing season. The ASD did not show any significant relation with its associated parameters.
Table 5. Pearson’s correlation coefficient (r) matrix between some parameters;
Derivation of mean air temperature
The MAT for 2016–2017 was derived empirically from the PSR and annual LR. illustrates the ten-day running average curves of PSR and Ta. shows the linear regression analysis between the two variables and the obtained regression parameters that were used to calculate MAT over the study area. Following the thirty-day time lag adjustment, the r2 increased from 0.76 to 0.96. The result indicates that the distribution of MAT can be improved by considering the influence of incoming solar radiation in addition to the local adiabatic LR. Consequently, the derived MAT showed a significant difference between the north- and south-facing slopes at the same elevations. In a similar landscape, Dashtseren et al. (Citation2014) observed a much lower Ta in a forested north-facing slope in both summer (by 2–3°C) and winter (by 1–2°C) seasons, relative to the opposite south-facing slope. However, the linear relationship with PSR may lead to uncertainties on steep slopes with high inclinations where the incoming radiation is very high or low. Therefore, additional Ta measurements on different slopes, placed at short horizontal distances to minimize the effect of adiabatic air, may reduce the uncertainties.
Figure 6. (a) Distribution curves of ten-day average potential radiation and observed and adjusted air temperatures at transect 3. After time lag and FT adjustments, the variance between potential radiation and temperature was significantly reduced. (b) The linear regression on the right shows the correlation between the adjusted Ta and potential radiation at transects 2 and 3. The linear equation was later applied for the estimation of MAT for 2016–2017
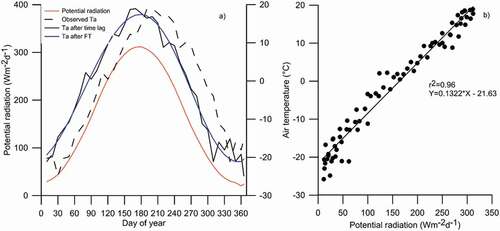
Determination of mean ground surface temperature
MGST was estimated for a one-year period from September 2016 to September 2017, including the complete freezing and thawing seasons. Although the study period was short, we believe that the spatial pattern of MGST and permafrost distribution has been successfully derived (). The comparison between the produced permafrost map and the recent MODIS-derived PPI using the temperature on top of permafrost model (Obu et al. Citation2019) shows overall agreement but with significant disagreement particularly on south-facing slopes. These discrepancies were expected due to the scale difference between the two maps (30 m and 1 km). Most of the Ts measurement sites at each transect, for instance, are within a single 1-km pixel. It is also worth mentioning that the modeled PPI is underestimated in this region because permafrost is covered by forest and moist organic layers, which favor the existence of permafrost (Obu et al. Citation2019).
Figure 7. Calculated MGST in the Sugnugur catchment for 2016–2017. The PPI lines indicate the permafrost probability index from Obu et al. (Citation2019). The inset shows the relationship between the observed MGST at individual sites and the estimated MGST. Red circles mark the outliers described in the text

The findings show good agreement with the Ts validation sites (inset in ), with the exception of a few outliers. In general, south-facing slopes indicate the absence of permafrost (MGST > 0°C), whereas north-facing slopes show the presence of permafrost (MGST < 0°C). The MGST for the study period ranges from +7.5 to −12°C with a root mean square error of ±1.4°C (r2 = 0.68). The two outliers with underestimations as much as 3°C represent the sites on strongly inclined north-facing slopes. In contrast, the outlier with an overestimation of 2°C comes from the valley bottom at transect 4, where a mixture of shrub and forest persists with shallow snow cover (only 12 cm). The areas in the valley bottom are mostly shaded by dense shrubs and show a relatively high soil moisture content (Kopp, Minderlein, and Menzel Citation2014). This may result in high evapotranspiration rates, reduced summer heating, and enhanced winter heat loss, thus favoring the existence of permafrost. We believe that such outliers appear due to the empirical linear relationship between PSR and MAT, as well as the shallow ASD and high moisture content.
Both the estimated MGST and PPI showed the possible existence of permafrost at the two borehole sites on the north-facing slope near transect 3. The calculated MGST in the SBF was 0.34°C (observed value 1.46°C) with a PPI of 0.41, and that in the UBF was −0.21°C (observed value 0.15°C) with a PPI of 0.44. Borehole temperature data between 2016 and 2018 in these two land covers indicate a significant increase in ground temperature in the SBF (Figure S3, Supplementary material). It is important to note that the combination of wildfire and an increased surface temperature in both thawing and freezing seasons over the last seven years may have triggered permafrost degradation in the study area. In addition to the borehole data, electrical resistivity surveys across the valley transects may help to further validate our results and draw a strong conclusion on permafrost distribution.
Conclusions
The distribution of permafrost in mountainous regions is a result of the heat balance between the ground and the air and can be predicted more precisely by determining the effects of vegetation and snow cover, microclimatic conditions, topography, and thermal conductivity of the soil. Based on in situ temperature measurements, we observed an MGST difference of 3–4°C on the north- and south-facing slopes within a short horizontal distance, seasonal Ta variability with elevational gradient including winter inversion, and generally increasing Ts in both freezing and thawing seasons in the study area. Moreover, the seasonal persistent snow cover insulates the Ts from Ta by roughly 50 percent as indicated by the average nt of 0.5. The sampled net biomass shows clear spatial variability across the valley transects and has a strong effect on the ground temperature regime and permafrost occurrence. However, short grass and sparse vegetation cover did not show any cooling effect (nt < 1) on Ts, probably due to the characteristics of the soil thermal conductivity and the summer overheating at the ground surface.
The overall SOs were roughly 4°C in the vegetated and persistent snow cover area and 1.5°C in the nonvegetated rock debris surfaces. We conclude that MAT can be approximated from PSR and LR after applying several adjustments and contribute to deriving the spatial variability of MGST. Finally, MGST was estimated for 2016–2017 with a 30-m resolution to predict permafrost distribution. The data limitation of the subsurface components did not allow us to derive the thermal offset (difference between MGST and temperature on top of the permafrost) for different soil types, a requirement for determining the temperature on top of the permafrost. The produced MGST indicated permafrost distribution on north-facing slopes and in higher elevations, whereas south-facing slopes were mostly permafrost-free. The result shows good agreement with the most recent PPI map by Obu et al. (Citation2019), except for south-facing slopes. In accordance with both of the maps, the borehole temperature data revealed a presence of permafrost in the UBF and degraded permafrost in the adjacent SBF.
Further investigations will focus on the stability of permafrost and the continuation of both Ta and Ts in situ measurements. Given that about one third of the forest in this headwater catchment is affected by wildfire, special attention should be paid to further investigations on ecosystem resilience and climate change mitigation in this region. Overall, we conclude that the main controlling factors of MGST and permafrost existence, such as topography, distribution of incoming solar radiation, and seasonal snow and vegetation cover, have been well investigated in this data-scarce mountainous permafrost region.
Author contributions
Munkhdavaa Munkhjargal analyzed the data, carried out the fieldwork, and wrote the article. Gansukh Yadamsuren contributed to the fieldwork and data analysis. Lucas Menzel provided climate data, supported the fieldwork, and supervised the study. Jambaljav Yamkhin carried out the snow measurements and provided crucial suggestions to improve the article.
Supplemental Material
Download Zip (597.1 KB)Disclosure statement
No potential conflict of interest was reported by the authors.
Supplementary material
Supplemental data for this article can be accessed here.
Additional information
Funding
References
- Abramov, A., S. Gruber, and D. Gilichinsky. 2008. Mountain permafrost on active volcanoes: Field data and statistical mapping, Klyuchevskaya Volcano Group, Kamchatka, Russia. Permafrost and Periglacial Processes 19:261–77. doi:https://doi.org/10.1002/ppp.622.
- Anarmaa, S., N. Sharkhuu, B. Etzelmüller, E. S. F. Heggem, F. E. Nelson, N. I. Shiklomanov, C. Goulden, and J. Brown. 2007. Permafrost monitoring in the Hovsgol mountain region, Mongolia. Journal of Geophysical Research: Earth Surface 112:F02S06. doi:https://doi.org/10.1029/2006JF000543.
- Azócar, G. F., A. Brenning, and X. Bodin. 2017. Permafrost distribution modelling in the semi-arid Chilean Andes. The Cryosphere 11:877–90. doi:https://doi.org/10.5194/tc-11-877-2017.
- Biskaborn, B. K., S. L. Smith, J. Noetzli, H. Matthes, G. Viera, D. A. Streletskiy, P. Schoeneich, V. E. Romanovsky, A. G. Lewkowicz, A. Abramov, et al. 2019. Permafrost is warming at a global scale. Nature Communications 10:264. doi:https://doi.org/10.1038/s41467-018-08240-4.
- Boeckli, L., A. Brenning, S. Gruber, and J. Noetzli. 2012. Permafrost distribution in the European Alps: Calculation and evaluation of an index map and summary statistics. The Cryosphere 6:807–20. doi:https://doi.org/10.5194/tc-6-807-2012.
- Chou, Y., Y. Sheng, and Y. Zhu. 2012. Cold regions science and technology study on the relationship between the shallow ground temperature of embankment and solar radiation in permafrost regions on Qinghai – Tibet Plateau. Cold Regions Science and Technology 78:122–30. doi:https://doi.org/10.1016/j.coldregions.2012.01.002.
- Dashtseren, A., M. Ishikawa, Y. Iijima, and Y. Jambaljav. 2014. Temperature regimes of the active layer and seasonally frozen ground under a forest-steppe mosaic, Mongolia. Permafrost and Periglacial Processes 25:295–306. doi:https://doi.org/10.1002/ppp.1824.
- Douglass, D. H., E. G. Blackman, and R. S. Knox. 2004. Temperature response of earth to the solar irradiance cycle. Physics Letters A 323 (3–4):315–22. doi:https://doi.org/10.1016/j.physleta.2004.01.066.
- Etzelmüller, B., I. Berthling, and J. L. Sollid. 1998. The distribution of permafrost in southern Norway: A GIS approach. Proceedings of the 7th International Conference on Permafrost 251–257, Centre d’etudes nordiques, University Laval, Quebec, Canada, June 23–27.
- Etzelmüller, B., E. S. F. Heggem, N. Sharkhuu, R. Frauenfelder, A. Kääb, and C. Goulden. 2006. Mountain permafrost distribution modelling using a multi-criteria approach in the Hövsgöl area, northern Mongolia. Permafrost and Periglacial Processes 17:91–104. doi:https://doi.org/10.1002/ppp.554.
- Fu, P., and P. Rich. 2002. A geometric solar radiation model with applications in agriculture and forestry. Computers and Electronics in Agriculture 37:25–35. doi:https://doi.org/10.1016/S0168-1699(02)00115-1.
- Hall, D. K., G. A. Riggs, V. V. Salomonson, N. E. Digirolamo, and K. J. Bayr. 2002. MODIS snow-cover products. Remote Sensing of Environment 83 (1–2):181–94. doi:https://doi.org/10.1016/S0034-4257(02)00095-0.
- Harris, C., L. U. Arenson, H. H. Christiansen, B. Etzelmüller, R. Frauenfelder, S. Gruber, W. Haeberli, C. Hauck, M. Hölze, O. Humlum, et al. 2009. Permafrost and climate in Europe: Monitoring and modelling thermal, geomorphological and geotechnical responses. Earth-Science Reviews 92 (3–4):117–71. doi:https://doi.org/10.1016/j.earscirev.2008.12.002.
- Hasler, A., M. Geertsema, V. Foord, S. Gruber, and J. Nötzli. 2015. The influence of surface characteristics, topography and continentality on mountain permafrost in British Columbia. The Cryosphere 9:1025–38. doi:https://doi.org/10.5194/tc-9-1025-2015.
- Heggem, E. S. F., B. Etzelmüller, S. Anarmaa, N. Sharkhuu, C. Goulden, and B. Nandintsetseg. 2006. Spatial distribution of ground surface temperatures and active layer depths in the Hövsgöl area, northern Mongolia. Permafrost and Periglacial Processes 17 (4):357–69. doi:https://doi.org/10.1002/ppp.568.
- Huang, S., P. M. Rich, R. L. Crabtree, C. Potter, and P. Fu. 2008. Modeling monthly near-surface air temperature from solar radiation and lapse rate: Application over complex terrain in Yellowstone National Park. Physical Geography 29 (2):158–78. doi:https://doi.org/10.2747/0272-3646.29.2.158.
- Ishikawa, M. 2003. Thermal regimes at the snow-ground interface and their implications for permafrost investigation. Geomorphology 52:105–20. doi:https://doi.org/10.1016/S0169-555X(02)00251-9.
- Ishikawa, M., Y. Iijima, Y. Zhang, T. Kadota, H. Yabuki, T. Ohata, B. Dorjgotov, and N. Sharkhuu. 2008. Comparable energy balance measurements on the permafrost and immediate adjacent permafrost-free slopes at the southern boundary of Eurasian permafrost, Mongolia. Proceedings of the 9th International Conference on Permafrost, University of Alaska, Fairbanks Alaska, USA, June 29–July 3.
- Ishikawa, M., J. Yamkin, A. Dashtseren, N. Sharkhuu, G. Davaa, Y. Ijima, N. Baatarbileg, and K. Yoshikawa. 2018. Thermal states, responsiveness and degradation of marginal permafrost in Mongolia. Permafrost and Periglacial Processes 29 (4):271–82. doi:https://doi.org/10.1002/ppp.1990.
- Juliussen, H., and O. Humlum. 2007. Towards a TTOP ground temperature model for mountainous terrain in central-eastern Norway. Permafrost and Periglacial Processes 18:161–84. doi:https://doi.org/10.1002/ppp.586.
- Karthe, D., N. S. Kasimov, S. R. Chalov, G. L. Shinkareva, M. Malsy, L. Menzel, P. Theuring, M. Hartwig, C. Schweitzer, J. Hofmann, et al. 2014. Integrating multiple-scale data for the assessment of water availability and quality in the Kharaa-Orkhon-Selenga River system. Geography, Environment, Sustainability 7 (3):65–86. doi:https://doi.org/10.24057/2071-9388-2014-7-3-40-49.
- Karunaratne, K. C., and C. R. Burn. 2004. Relations between air and surface temperature in discontinuous permafrost terrain near Mayo, Yukon Territory. Canadian Journal of Earth Sciences 41 (12):1437–51. doi:https://doi.org/10.1139/e04-082.
- Kopp, B. J., J. Lange, and L. Menzel. 2016. Effects of wildfire on runoff generating processes in northern Mongolia. Regional Environmental Change 17:1951–63. doi:https://doi.org/10.1007/s10113-016-0962-y.
- Kopp, B. J., S. Minderlein, and L. Menzel. 2014. Soil moisture dynamics in a mountainous headwater area in the discontinuous permafrost zone of northern Mongolia. Arctic, Antarctic, and Alpine Research 46 (2):459–70. doi:https://doi.org/10.1657/1938-4246-46.2.459.
- Lacelle, D., C. Lapalme, A. F. Davila, W. Pollard, M. Marinova, J. Heldmann, and C. McKay. 2016. Solar radiation and air and ground temperature relations in the cold and hyper-arid Quartermain Mountains, McMurdo Dry Valleys of Antarctica. Permafrost and Periglacial Processes 27 (2):163–76. doi:https://doi.org/10.1002/ppp.1859.
- Lange, J., B. J. Kopp, M. Bents, and L. Menzel. 2015. Tracing variability of run-off generation in mountainous permafrost of semi-arid north-eastern Mongolia. Hydrological Processes 29 (6):1046–55. doi:https://doi.org/10.1002/hyp.10218.
- Lunardini, V. J. 1978. Theory of N-factors and correlation of data. Proceedings of the 3rd International Conference on Permafrost, vol. 1, 40–46, Ottawa, Canada, July 10–13.
- Luo, D., H. Jin, and V. F. Bense. 2019. Ground surface temperature and the detection of permafrost in the rugged topography on NE Qinghai-Tibet Plateau. Geoderma 333:57–68. doi:https://doi.org/10.1016/j.geoderma.2018.07.011.
- Menzel, L., J. Hofmann, and R. Ibisch. 2011. Studies of water and mass fluxes to provide a basis for an Integrated Water Resources Management (IWRM) in the catchment of the River Kharaa in Mongolia. Hydrologie und Wasserbewirtschaftung. 55:88–103. Accessed April 25, 2018. http://www.ufz.de./.
- Minder, J. R., P. W. Mote, and J. D. Lundquist. 2010. Surface temperature lapse rates over complex terrain: Lessons from the cascade mountains. Journal of Geophysical Research Atmospheres 115 (14):1–13. doi:https://doi.org/10.1029/2009JD013493.
- Minderlein, S., and L. Menzel. 2014. Evapotranspiration and energy balance dynamics of a semi-arid mountainous steppe and shrubland site in northern Mongolia. Environmental Earth Science 73 (2):593–609. doi:https://doi.org/10.1007/s12665-014-3335-1.
- Ministry of Environment and Green Development. 2014. Mongolian second assessment report on climate change. Ministry of Environment and Green Development, Ulaanbaatar, Mongolia.
- Munkhjargal, M., S. Groos, G. P. Caleb, G. Yadamsuren, J. Yamkin, and L. Menzel. 2019. Multi-source based spatio-temporal distribution of snow in a semi-arid headwater catchment of northern Mongolia. Geosciences 9 (1):53. doi:https://doi.org/10.3390/geosciences9010053.
- Nelson, F. E. 2003. (Un) frozen in time. Science 299 (5613):1673–75.
- Obu, J., S. Westermann, A. Bartsch, N. Berdnikov, H. H. Christiansen, A. Dashtseren, R. Delaloy, B. Elberling, B. Etzelmüller, A. Kholodov, et al. 2019. Northern Hemisphere permafrost map based on TTOP modelling for 2000–2016 at 1 km2 scale. Earth-Science Reviews 193:299–316. doi:https://doi.org/10.1016/j.earscirev.2019.04.023.
- Pan, C. G., J. S. Kimball, M. Munkhjargal, N. P. Robinson, E. Tijdeman, L. Menzel, and P. B. Kirchner. 2019. Role of surface melt and icing events in livestock mortality across Mongolia’s semi arid landscape. Remote Sensing 11 (20):2392. doi:https://doi.org/10.3390/rs11202392.
- Peng, X., T. Zhang, B. Cao, Q. Wang, W. Shao, and H. Guo. 2015. Changes in freezing-thawing index and soil freeze depth over the Heihe River Basin, Western China. Arctic, Antarctic, and Alpine Research 48 (1):161–76. doi:https://doi.org/10.1657/AAAR00C-13-127.
- Pepin, N., R. S. Bradley, H. F. Diaz, M. Baraer, E. B. Caceres, N. Forsythe, H. Fowler, G. Greenwood, M. Z. Hashmi, X. D. Liu, et al. 2015. Elevation-dependent warming in mountain regions of the world. Nature Climate Change 5:424–30. doi:https://doi.org/10.1038/nclimate2563.
- Riseborough, D. W., N. Shiklomanov, B. Etzelmüller, S. Gruber, and S. Marchenko. 2008. Recent advances in permafrost modelling. Permafrost and Periglacial Processes 19:137–56. doi:https://doi.org/10.1002/ppp.615.
- Smith, M. W., and D. W. Riseborough. 1996. Permafrost monitoring and detection of climate change. Permafrost and Periglacial Processes 7:301–09.
- Smith, M. W., and D. W. Riseborough. 2002. Climate and the limits of permafrost: A zonal analysis. Permafrost and Periglacial Processes 13 (1):1–15. doi:https://doi.org/10.1002/ppp.410.
- Strachan, S., and C. Daly. 2017. Testing the daily PRISM air temperature model on semiarid mountain slopes. Journal of Geophysical Research: Atmospheres 122:5697–715. doi:https://doi.org/10.1002/2016JD025920.
- Way, R. G., and A. G. Lewkowicz. 2018. Environmental controls on ground temperature and permafrost in Labrador, northeast Canada. Permafrost and Periglacial Processes 29:73–85. doi:https://doi.org/10.1002/ppp.1972.
- Williams, P. J., and M. W. Smith. 1989. The frozen earth: Fundamentals of geocryology. Cambridge, UK: Cambridge University. Press.
- Yoshikawa, K., W. R. Bolton, V. E. Romanovsky, M. Fukuda, and L. D. Hinzman. 2002. Impacts of wildfire on the permafrost in the boreal forests of Interior Alaska. Journal of Geophysical Research: Atmospheres 107 (D1):FFR 4-1–FFR 4–14. doi:https://doi.org/10.1029/2001JD000438.
- Zhang, T., O. W. Frauenfeld, M. C. Serreze, A. Etringer, C. Oelke, J. McCreight, R. G. Barry, D. Gilichinsky, D. Yang, H. Ye, et al. 2005. Spatial and temporal variability in active layer thickness over the Russian Arctic drainage basin. Journal of Geophysical Research: Atmospheres 110:D16101. doi:https://doi.org/10.1029/2004JD005642.