ABSTRACT
Young children tend to rely on reactive cognitive control (e.g. strongly slow down after an error), even when task accuracy would benefit from proactive cognitive control (taking a slower task approach up front). We investigated if giving young primary school children opportunities to repeatedly experience tasks where success rates depend on balancing speed and accuracy by using proactive and reactive cognitive control promotes their selective use of proactive cognitive control strategies. Participants were children from the German-speaking part of Switzerland (N = 105; mean age 7.5 yrs). They were allocated to a “training with feedback,” “training without feedback,” or control condition. They completed a pre- and posttest (Hearts and Flower task), separated by six computerized training tasks over three weeks. We analyzed time-by-condition effects on reaction time, rate-correct, and post-error slowing. In line with our hypotheses, both training groups outperformed the control group on reaction time and rate-correct in the incongruent block. Contrary to expectation, feedback did not enhance the intervention effect and the training group without feedback additionally outperformed control participants on rate-correct in the mixed block and improved their post-error slowing. Thus, our short intervention triggered accelerated development of proactive control in a cognitive conflict task requiring accuracy-speed trade-offs. This shows that during the developmental period in which children are assumed ready for developing proactive cognitive control strategies, repeated experiences over a short time-period can lead to acute improvements. More research is needed to check for long-term benefits and generalizability beyond our sample and the tasks employed.
Introduction
In our daily lives, we use executive functions (EF), a set of higher-order cognitive skills, enabling us to regulate our thoughts, behaviors, and emotions to achieve our goals. EF entail first and foremost the inhibition of prepotent responses and resistance to interference, the ability to keep and operate on a circumscribed amount of information in working memory, and to switch attention and cognitive processing flexibly from one task rule to another. These processes are already important during childhood, and particularly evident in the classroom, where children must behave according to the class rules (e.g., raise their hand instead of calling out), ignore distractions to complete their tasks, and select the most productive strategies for completing tasks. Not unexpectedly, EF in children have widely been found to be predictive of school readiness (e.g., Blair & Raver, Citation2015; Korucu et al., Citation2020; Micalizzi et al., Citation2019), classroom behavior (e.g., Heemskerk & Roebers, Citation2023; McCoy et al., Citation2022; Nesbitt & Farrarn, Citation2022), and academic achievement (e.g., Cortés Pascual et al., Citation2019; Magalhães et al., Citation2020; Morgan et al., Citation2019; Robson et al., Citation2020; Spiegel et al., Citation2021) in western cultures.
Given the importance of EF for early (and later) cognitive and academic development, it is no surprise that an uncounted number of EF intervention studies have been realized; see Aksayli et al. (Citation2019), Diamond and Ling (Citation2020), and Pauli-Pott et al. (Citation2021), for reviews. Yet not all programs are successful at improving EF and where positive results are reported the gains are generally small (e.g., Aksayli et al., Citation2019; Diamond & Ling, Citation2020) and mostly do not generalize beyond the trained task (Kassai et al., Citation2019) or last beyond the intervention duration (Takacs & Kassai, Citation2019). In the present approach, we explore the possibility that monitoring processes and thereby especially an increased use of proactive cognitive control contribute to improvements in EF. Here, we operationalize cognitive control as strategic, goal-oriented micro-adjustments in approaching simple, multi-trial tasks with the aim to optimize performance (e.g., increasing accuracy by slowing down response times to avoid errors; shortening/speeding up response times and maintaining high accuracy when conflict is low) as a first step toward exploring this candidate factor for EF development. From this perspective, cognitive control is thought to be a meta-process inherent in EF: steering and controlling EF, contributing to further optimization and developmental progression of EF (Camerota et al., Citation2019). Consequently, and this is what we want to explore, if we give children opportunities to practice and optimize cognitive control, we may, in fact, trigger a developmental process contributing to EF development and orchestrating EF in any given task. Therefore, we will address the literature on cognitive control and its development in our age range next.
Cognitive control is thus considered here as an overarching process coordinating the dynamic interactions of the various specialized core components of EF (such as inhibition or switching; Botvinick et al., Citation2001; Braver et al., Citation2007). Dynamic adaptations in the engagement and coordination of EF processes are needed throughout any cognitive conflict task completion, but tasks differ in terms of how much cognitive control and what type of cognitive control are required and best suited to meet the demands of the task. For example, when an individual chooses a fast task approach, errors are likely to occur, and these are then typically associated with very short reaction times (Ambrosi et al., Citation2019; Botvinick et al., Citation2001; Roebers, Citation2022). Consequently, an individual might strategically slow down overall to prevent future errors or slow down strongly but only after an error occurs. In their theoretical view on cognitive control, Botvinick and Braver (Botvinick et al., Citation2001; Braver et al., Citation2007) differentiate between proactive and reactive cognitive control strategies.
When proactive cognitive control is engaged, we anticipate and prevent cognitive conflict before errors occur. By contrast, when mainly reactive cognitive control is engaged, conflicts are not prepared for but detected and resolved only when they occur (Kamijo et al., Citation2016). Engaging proactive cognitive control is related to flexible and precautional adaptations to the speed of responding depending on a task’s complexity, resulting in less impulsivity in reacting and the commission of fewer errors (Jentzsch & Leuthold, Citation2006).
However, proactive cognitive control requires anticipatory task- or task-block-specific adaptations, as well as trial-by-trial adjustments to optimize performance and is therefore considered as the more effortful and mature strategy to employ (Kamijo et al., Citation2016). And, despite proactive cognitive control being favorable from the perspective of task performance/accuracy (e.g. Botvinick et al., Citation2001; Braver, Citation2012; Jentzsch & Leuthold, Citation2006), young children tend to default to reactive cognitive control. For example, Chevalier et al. (Citation2020) used a cued task-switching task and demonstrated that young children defaulted to reactive cognitive control rather than proactive cognitive control like adults. And, Roebers (Citation2022) found that in their sample of six- to eight-year-old Swiss children, the older children demonstrated strategic slowing in the most demanding block of the Hearts and Flowers (HF) task requiring rule-switching, but not the younger children. At the same time, however, Roebers reported that even the 8-year-olds’ errors were associated with significantly shorter reaction times compared to correct responses and that all age groups exhibited significant post-error slowing (PES), indicative of reactive cognitive control. The latter results suggest that in this age window of 6 to 8 years, reactive cognitive control is still the predominant cognitive conflict task strategy.
Chevalier has put forward the idea that one reason for school children’s continuing improvement of EF lies in the development of proactive cognitive control. Precisely, he assumes a developmental shift between early and later primary school age (Chevalier & Blaye, Citation2016; Chevalier et al., Citation2015). Children in this age range are thought to become increasingly less reliant on external cues to monitor their errors (less reactive cognitive control) and learn to adjust their cognitive control modes flexibly and anticipatorily to varying task demands, increasingly engaging in proactive cognitive control (Chevalier, Citation2015; Chevalier & Blaye, Citation2016; Roebers, Citation2017, Citation2022).
Evidence supporting this notion comes from, for example, Ambrosi et al. (Citation2016), who found evidence of relatively coarse trial-by-trial adjustments in inhibitory control in five- and six-year-old French children in three conflict tasks (Flanker, Simon, and Stroop). Additionally, Brewer and Smith (Citation1989) used a serial four-choice reaction time (RT) task, where participants were instructed to respond as fast as possible without making mistakes and identified that proactive cognitive control was poor in five-year-olds, present but inconsistent in seven-year-olds, and becoming better and more consistent in nine-year-olds. Taking the fine-tuning of response times as their measure of cognitive control – i.e. finding one’s optimum response time for responding as fast as possible without making a mistake – Brewer and Smith (Citation1989) found that, like adults, older children made more subtle adjustments to their RT to prevent (recurring) errors (proactive cognitive control), whereas younger children greatly sped up their RT toward an error (impulsive errors) and then excessively increased their RT after committing an error (coarse/exaggerated PES; both evidence for reactive cognitive control). For a more elaborated review on cognitive control development in young children, see Doebel et al. (Citation2017).
The described developmental improvement toward increasingly proactive cognitive control between the ages of 6 and 8 years is thought to rely – first and foremost – on experience (Chevalier, Citation2015). Children in this age range need to be able to practice using proactive cognitive control to make it less effortful. Additionally, when they (repeatedly) experience the positive effects of using proactive cognitive control – such as higher accuracy or making fewer errors – this will lead to a greater likelihood that proactive cognitive control will be selected in future (Braver, Citation2012; Chevalier, Citation2015).
Development in core EF processes beyond the kindergarten age may thus be fueled by experiencing that finding the optimal response speed avoids many errors in easy, multi-trial tasks. However, young children are known to still have an overoptimistic view of their performance and overlook one in every two errors they make when asked to monitor their performance, for example, in a memory task (Destan & Roebers, Citation2015). Thus, young children may simply not recognize every error they commit and may thus not see the necessity of slowing down their speed of responding and use proactive cognitive control.
Therefore, feedback can help children to recognize more of their errors and to more efficiently engage effective cognitive control to monitor their performance and ensure they are on track to meet their goals (Hattie & Timperley, Citation2007). Van Loon and Roebers (Citation2020) found that kindergartners improved their error recognition after an intervention where they received feedback on their errors. Yet not every feedback is equally effective. Hattie and Timperley (Citation2007) specify that immediate (as opposed to delayed) feedback can be particularly helpful in accommodating faster skill acquisition. Moreover, they found that for learning new skills, corrective feedback was more effective than reward, praise, or punishment types of feedback (Hattie & Timperley, Citation2007). Therefore, one way to help children gain the most from their cognitive control experiences might be to give them objective, online feedback on the accuracy of their responses.
The present study
The aim of this study was the investigation of a possible causal effect of providing practice opportunities and immediate performance feedback, in terms of triggering proactive cognitive control development in the developmental window of early primary school. Thus, although we use an intervention design, this is not just another EF training study but rather a theory-driven attempt to prove the assumed principle that through experiences with or without feedback, children in the age range of 6 to 8 can learn to increasingly use proactive cognitive control for coordinating their EF. We selected children in first and second grade of primary schools in the German-speaking part of Switzerland. This is the age range where proactive cognitive control has been found to be developing, but is not yet reliably and spontaneously recruited (e.g., Chevalier et al., Citation2020).
We used the Hearts and Flower task (HF; Davidson et al., Citation2006; Diamond et al., Citation2007) to generate our measure of cognitive control. This task has been found to be reliable (α = .83, Rosas et al., Citation2019) and to correspond with measures of EF acquired from a range of other tests, including the Flanker, Stroop, and Go/No Go (Brocki & Tillman, Citation2014). The HF task consists of three blocks. First, in the congruent-only Hearts block, they must press a button on the same side as where the stimulus (a red heart) appears. Next, in the incongruent-only Flowers block triggering predominantly inhibition, they must press a button on the opposite side to where the stimulus (a red flower) is presented. Finally, a Mixed block with both congruent and incongruent stimuli is completed in which both rules have to be applied in an unpredictable order
(see ).
In this speeded-response task involving interference inhibition, participants must respond as quickly as possible without making mistakes, thus balancing their reaction time and accuracy – also known as the accuracy-speed trade-off (Smulders et al., Citation2016). Adapting to both requirements requires optimizing accuracy at the fastest possible speed; or, in other words, slowing down only as much as is necessary to avoid mistakes. This can be measured with the “rate-correct” value [R-C], a measure that integrates accuracy and RT (Woltz & Was, Citation2006) by reflecting the number of correct responses in a given time unit (e.g., a second). A better fine-tuning of the accuracy-speed trade-off, with smaller, anticipatory adjustments to the RT, requires proactive cognitive control and will result in a higher R-C.
Under proactive cognitive control, the motor action of pressing the response button is only initiated after the stimulus has been evaluated (Kang et al., Citation2022). This involves taking the time to collect all necessary information for stimulus evaluation, which takes longer when the task is complex or unpredictable (Chevalier et al., Citation2020; Jentzsch & Leuthold, Citation2006). Thus, in the HF task, we expect longer RTs in the more complex Mixed block than the simpler congruent and incongruent blocks. Additionally, effective employment of proactive cognitive control will avoid unnecessarily slow RT and increase accuracy, resulting in higher R-C values.
Between the pre- and posttest, participants completed six training sessions consisting of adaptations of two speeded-response tasks involving interference inhibition (HF and Simon task). Following evidence from previous training studies, we increased the level of difficulty in the training tasks to enhance the effectiveness and maintain the participants’ interest and motivation (Diamond, Citation2013; Diamond & Lee, Citation2011; Diamond & Ling, Citation2020; Dunning et al., Citation2013; Holmes et al., Citation2009). Additionally, as enjoyment of the training tasks is paramount, we embedded the training into a storyline to make the experience much more enjoyable for young children (Diamond & Ling, Citation2020). Finally, as varied practice has long been known to yield greater long-term benefits (Diamond & Ling, Citation2020), we used two different tasks to adapt and created six different storylines to increase variation, and further boost motivation and enjoyment.
In one training condition, we gave children error feedback on a trial-to-trial basis and encouraged them to slow down their responses if they made repeated errors. The participants in the other training condition completed the identical training without feedback. The provided feedback consisted of an auditory (beep) and visual (“oops” smiley on screen) indication that a mistake had been made, and, in the case of repeated errors or a too-slow response, additional auditory information how to proceed without further errors (see ). Thereby, the intervention provided participants with condensed practice opportunities for optimizing their accuracy-speed trade-off. The active control condition took part in a paired-associates memory task.
Figure 2. Feedback provided to participants in the training condition with feedback during training tasks.
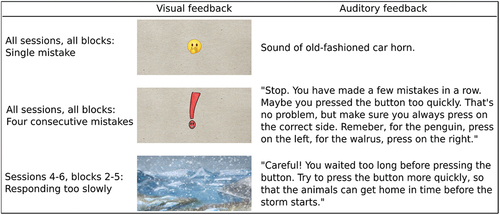
We hypothesized that children in both training conditions would improve more on their R-C on the HF task than those in the control condition, as intervention gains have been found to be more reliable on near-transfer tasks (Diamond & Ling, Citation2020; Kassai et al., Citation2019; Redick et al., Citation2013; Shipstead et al., Citation2012) and the control condition participants did not take part in tasks where success depended on an optimized accuracy-speed trade-off. Finally, we hypothesized that the addition of feedback would stimulate children to employ proactive cognitive control more often, leading to greater improvements in R-C and a greater reduction of impulsive errors (Jentzsch & Leuthold, Citation2006; Kang et al., Citation2022). Thus, we expected the training condition with feedback to outperform the training condition without feedback. To shed further light on how children might achieve a higher R-C, we will explore RT on error trials and PES in the pre- and posttest, as a function of condition.
Method
Sample and recruitment
Ethical clearance was provided by the ethics committee of the University of Bern. Data were collected during the final term of first grade (n = 51) and the first term of second grade (n = 114) in primary schools across the German-speaking part of Switzerland. Teachers were invited to participate in the study with their classes, and children with informed parental consent were allowed to take part. Data from 39 children could not be included in analyses, as they did not complete the Mixed block of the HF task at T1, 18 participants did not complete the Mixed block at T2, and data from another three participants were removed due to outliers. The sample was divided into three groups at the class level; two training conditions, one receiving trial-by-trial feedback on their errors (n = 42, 47.6% male, Mage = 90.4 m, SDage = 6.3), and one completing the exact same training without the feedback (n = 32, 50.0% male, Mage = 91.1 m, SDage = 5.9), and the active control condition (n = 31, 58.1% male, Mage = 88.5 m, SDage = 4.4). Analysis of variance (ANOVA) indicated no significant differences in age (F2,101 = 1.581, p = 0.211) or fluid intelligence (“Odd-Item-Out” task from the RIAS, (Hagmann von Arx & Grob, Citation2014; Reynolds & Kamphaus, Citation2003)) (F2,101 = 0.210, p = 0.811) between the three groups, and chi-square testing indicated no differences in sex (chi2(2) = 0.817, p = 0.665). Fisher’s exact test indicated differences in the highest level of parental education between the groups (p = 0.016), favoring the training group with feedback. A full overview of the sample characteristics can be found in .
Table 1. Sample descriptives by condition.
Procedure
All tasks were completed on touchscreen tablets (Samsung Galaxy Tab A7 and S4, screen size 10.5” and 10.4,” respectively), with instructions and feedback provided via headphones. Pre- and post-testing were done in a group setting in the classroom. At pre- and posttest, participants completed the Hearts and Flowers task (HF) (Davidson et al., Citation2006). Additionally, we collected a measure of fluid intelligence to assess individual differences. Pre- and post-testing lasted approximately 60 minutes. The intervention lasted three weeks, with two training sessions per week. For training sessions, research assistants took half the students of the participating class at a time to a separate room within the school. These sessions lasted approximately 15 minutes.
Materials and measures
Pre- and post-test
Hearts and flowers
The HF task consisted of three blocks (congruent − 12 trials, incongruent − 24 trials, and mixed − 36 trials), each preceded by eight practice trials, of which five needed to be correct to proceed to the main task. If participants did not succeed in the practice trials, the practice trials were repeated, and the task automatically terminated if they got them wrong twice. Children responded to the stimuli via external response buttons placed to the left and right of the screen. In the congruent block, participants needed to press the button on the same side as where the stimulus (a red heart) appeared. In the incongruent block, the opposite button to where the stimulus (a red flower) appeared needed to be pressed. In the Mixed block, if a heart was presented, the button on the same side needed to be pressed, and if a flower was presented (20% of trials), the button on the opposite side needed to be pressed (see ). Accuracy and response time (RT) were recorded for each trial. Every trial was preceded by a screen with a central fixation cross for 500 ms. Stimulus presentation time was 600 ms.
Fluid intelligence
We used the “Odd-Item-Out” task from the RIAS (Hagmann von Arx & Grob, Citation2014; Reynolds & Kamphaus, Citation2003) as an estimation of fluid intelligence. In each presented matrix, participants had to identify the “odd item out” from a set of related stimuli. Four practice matrices were completed at the start, with feedback provided. In the main task, when three consecutive matrices were solved incorrectly, the task automatically ended. For correct answers within 30 seconds participants received two points, for correct answers within 50 seconds they received one point. No points were scored in the practice trials. Possible scores were 0 to 102 points.
Training sessions
Training conditions
Training sessions for the children in both training conditions (with and without feedback) consisted of an adaptation of either the Simon task (Simon, Citation1990) (sessions 1, 3, and 5) or the HF task (Davidson et al., Citation2006; Diamond et al., Citation2007) (sessions 2, 4, and 6), both of which are interference inhibition (conflict) tasks with speeded responses. The only difference between the training tasks for the two conditions was that one group received error and speed feedback, whereas the other group did not (see “Feedback” below).
The adapted versions used for training consisted of five instead of three blocks (two additional Mixed blocks) to allow for increasing difficulty (increased speed, varying stimulus presentation height, added distractors – more details are provided below). To enhance variation and boost motivation, we used two different tasks, and each session was presented as a game, based on a story about two animals, who needed help to get home before a storm or natural danger. We further changed the theme for each session (starfish fleeing a squid, desert animals fleeing a sandstorm, crabs fleeing a flood wave, birds fleeing a tropical rainstorm, arctic animals fleeing a snowstorm, and woodland animals fleeing a thunderstorm) and added background music to the tasks, which also changed with each session.
Each stimulus consisted of one of the two animals and appeared either on the left or the right of the screen. In the Simon task adaptations, one animal always lived on the left of the screen and the other on the right (see for an example). In the HF task adaptations, one animal always lived on the same side as where the stimulus was presented, and the other lived on the opposite side of stimulus presentation. Using the same external response buttons as in the HF task, the children needed to press on the correct side to send each animal home before, for example, they got caught in a storm. Accuracy and RT were recorded for each trial. Stimulus presentation time decreased over the sessions to increase difficulty (see ). Between trials, a fixation cross was presented in the center of the screen for 500 ms.
Table 2. Progression of difficulty in the training sessions.
Each task consisted of five blocks (congruent, incongruent, and three mixed blocks with 25% incongruent trials, randomized to occur every 3rd, 4th, or 5th trial), which progressively increased in difficulty. Blocks 1–3 were preceded by four practice trials, which were repeated until 100% accuracy was achieved before progressing to the main trials of the block. As the rules for blocks 4 and 5 were identical to block 3, no additional practice trials were completed. In both training conditions, difficulty in blocks 4 and 5 was increased by decreasing the stimulus presentation time, increasing the tempo of the background music, adding occasional (25%) distractor images during the inter-stimulus interval (ISI), and varying the presentation height of the stimuli as well as the presentation location of the inter-stimulus distractor. Distractors were randomized to appear in every 3rd, 4th, or 5th ISI, with a different randomization than the incongruent stimuli. In addition to within-task increases in difficulty, the difficulty in both conditions also increased from week to week, so that the starting tempo in the following week would equal the final tempo of the previous week. An overview of all adaptations to increase the difficulty level can be found in .
Feedback
The tasks completed by the two groups were identical, but those in the condition with feedback received feedback on their accuracy (notification on incorrect trials) and speed (slow responses, final three sessions only), as illustrated in . The training group without feedback received neither accuracy nor speed feedback.
Feedback for incorrect trials consisted of the sound of an old-fashioned car-horn accompanied by the appearance of a smiley with their hand covering their mouth in an ‘oops’ gesture. The background music stopped and only restarted when a correct trial was completed. If four consecutive errors were made, an exclamation mark appeared on the screen, and the child was reminded via audio that they needed to take enough time to answer correctly, including a reminder of the response rules in the current block.
In the second half of the training sessions (sessions 4–6), feedback on response speed was added. During blocks 2–5 of these sessions, participants needed to respond within 2000–3000 ms, dependent on the block and session (see ). Otherwise, a warning appeared that the animal had not gotten home quickly enough before the storm. These trials were recorded as incorrect.
Control condition
The training sessions for the active control group consisted of a paired associates memory task, where participants helped to feed animals. They were presented with 12 animals and needed to remember their corresponding food. The type of animals changed each session (forest, sea, birds, rodents, savannah, and insects). Each animal-food pairing was displayed for 5 seconds. Next, the children played a one-minute filler task; a mouse appeared in a random location on the tablet’s touch screen and the child needed to catch the mouse by tapping on it. They then moved on to the recognition phase, where each animal was shown along with four food options. The participants were asked to choose which food was the correct one for this animal. They received visual and auditory feedback on each trial (correct or incorrect), but there was no requirement to respond quickly. Thus, no accuracy-speed trade-off was involved in this task.
Statistical analyses
From the HF task data, trials with RT <200 ms or >2000 ms were removed (1256 trials, 3.5%). Next, the proportion of correct trials, mean RT on correct and incorrect trials (separately), and R-C (number of correct answers per second) were calculated per block. Participants with an accuracy below chance (<.5) on any block were removed from the data set (n = 3). R-C was calculated by dividing the total number of correct answers of each block by the total time in seconds taken to complete the block. The R-C variable takes into account meaningful variance in both the RT and the accuracy of answers, thus it reflects the response speed adjusted for errors committed (Woltz & Was, Citation2006).
Analysis was carried out in RStudio (version 4.2.0, RStudio Team, Citation2022). We report Spearman correlations to account for the non-normal distribution in some of the variables. RT and R-C in the Flowers and Mixed blocks were used as outcome variables. To assess the effect of feedback on the outcome variables, we performed mixed ANOVAs (analysis of variance) with condition as the between factor and measurement point (pre or post) as the within factor, using the package “nlme” (Pinheiro et al., Citation2022). Follow-up pairwise comparisons of rates of change were carried out using the “emmeans” package (Lenth, Citation2023). Significance was set at .05.
Results
Descriptive results
HF task’s mean accuracy, RT, and R-C for each group at pretest are included in . In the Mixed block, there were significant differences between conditions in RT and R-C. We carried out ANOVAs to assess the influence of pretest performance on posttest performance as an interaction of pretest performance and condition allocation, and found no significant interaction for RT or R-C (p = 0.516 and 0.382, respectively). Thus, these pretest differences did not affect the rate of improvement in any of the groups.
As can be seen in at pretest, RT did not change noticeably from the Flowers to the Mixed block, whereas accuracy dropped. At posttest, it appears the training groups adjusted their RT to the increased difficulty in the Mixed block by slowing down compared to the Flowers block. Their accuracy dropped less dramatically from the Flowers to the Mixed than at pretest. This change from pre- to posttest was not observed in the control group.
Table 3. Hearts and Flowers data at pre- and posttest, by condition.
HF task’s accuracy, RT, and R-C were highly correlated across blocks. R-C correlated only weakly with accuracy and strongly with RT. RT and accuracy did not correlate with each other, except in the Hearts block. RIAS test score correlated weakly with accuracy in the Flowers and Mixed blocks, but not with RT or RC. Parental education level did not correlate with any measures from the HF task or RIAS (p = 0.145–0.970). The full correlation matrix can be found in .and Figure S.2) indicating that
Table 4. Correlation matrix of Hearts and Flowers variables.
Intervention effects
Reaction time
In both the Flowers and the Mixed block, we found main effects of time on RT within the block, with all groups getting faster from pre- to posttest (see ). Additionally, we found an interaction effect of time and condition in the Flowers, but not the Mixed block.
Figure 4. Plots of the reaction time in the Flowers and Mixed blocks across conditions and measurement points.
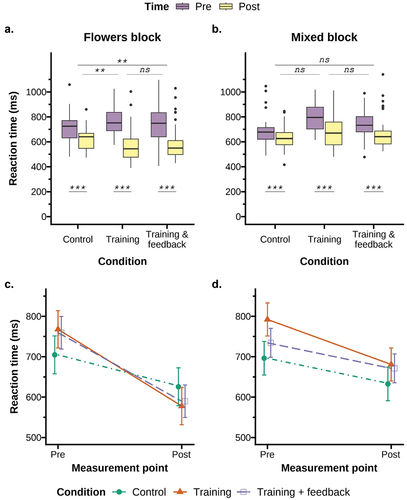
In the Flowers block, the interaction of time and condition was significant (F 2,102 = 7.290, p = 0.001; see , panels A and C, and Figure S.1). Both training groups decreased their RT more than the control group did (training with feedback vs. control: estimate = 90.7, 95% CI [20.9, 160.4], p = 0.007; training without feedback vs. control: estimate = 111.0, 95% CI [36.8, 185.3], p = 0.002). The two training groups did not differ from each other in their reduction of RT (p = 0.764). In the Mixed block, the time-by-condition interaction for RT was not significant and all groups decreased their RT by equivalent margins (p = 0.090; see , panels B and D, and Figure S.1).
In our analysis of proactive cognitive control, that is, slowing down at the task-block level, we looked at RT across the Flowers and Mixed blocks. We noticed that, at pretest, there was no difference in RT between the Flowers and Mixed blocks in any of the three groups (t(306) <1.44, p’s > 0.152; , panel A, and Figure S.2) indicating that children did not generally slow down when moving on to the more demanding block. However, at posttest, RT differed between the Flowers and Mixed blocks in both training conditions: participants responded more slowly in the Mixed block than in the Flowers block (training without feedback: estimate = −103.0, 95% CI [−142.3, −63.7], p < 0.001; training with feedback: estimate = −81.3, 95% CI [−115.6, −47.0], p < 0.001). This difference was not observed in the control condition (p = 0.734; see , panel B, and Figure S.2). Moreover, the training groups did not differ from each other in their rate of slowing down between the Flowers and Mixed block (p = 0.471). To assess if this use of proactive cognitive control (slowing down in the Mixed block relative to the Flowers block) was productive, we analyzed it in combination with accuracy: the R-C variable.
Rate-correct
For R-C, the time-by-condition effect in the Flowers block was significant (F (2,102) = 8.159, p < 0.001). Post-hoc tests revealed the same pattern of differences as for RT; all groups improved their R-C, but the control group differed from both training groups (training without feedback vs. control: estimate = −0.30, 95% CI [−0.49, −0.12], p < 0.001; training with feedback vs. control: estimate = −0.20, 95% CI [−0.37, −0.03], p < 0.017), with participants in the training conditions more strongly improving their R-C compared to those in the control condition. No differences in R-C improvement were found between the two training conditions (p = 0.331; see , panel A and C, and Figure S.3).
Figure 6. Plots of the rate-correct in the Flowers and Mixed blocks across conditions and measurement points.
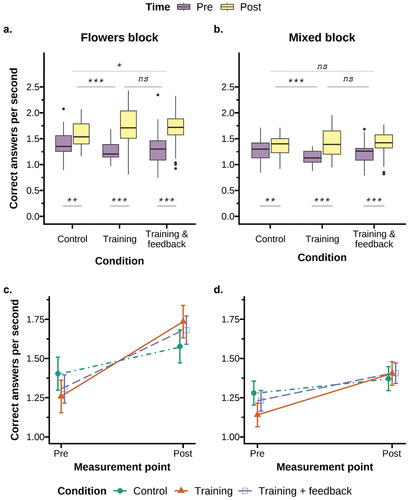
We also found a significant interaction effect for R-C in the Mixed block (F (2,102) = 6.840, p = 0.002). The improvements were once more significant in all groups (p’s < 0.007). In this block, only the training group without feedback differed significantly from the control group (estimate = −0.17, 95% CI [−0.28, −0.06], p = 0.001). The training groups again did not significantly differ from each other (p = 0.111; see , panel B and D, and Figure S.3). This may indicate that the training group with feedback slowed down too much to avoid errors, whereas the training group without feedback could optimize their accuracy-speed trade-off.
Impulsive errors
We found a significant difference in RT on correct and incorrect trials in the Flowers block at pretest in all conditions (control: estimate = −162.5, 95% CI [−228.5, −96.4], p < 0.001; training without feedback: estimate = −98.1, 95% CI [−163.5, −32.8], p = 0.003; training with feedback: estimate = −97.6, 95% CI [−155.4, −39.9], p = 0.001), with shorter RT on incorrect than correct trials (see , panel A). This indicates that the errors committed at pretest were – at least in part – impulsive in nature. At posttest, we found impulsive errors only in the control condition (estimate = −116.0, 95% CI [−182.6, −49.4], p < 0.001; see , panel B), whereas both training groups no longer responded significantly faster on incorrect trials compared to correct trials (p’s > 0.257).
Figure 7. Line graphs of the difference in reaction time between correct and incorrect trials of the Flowers block at pre- and posttest.
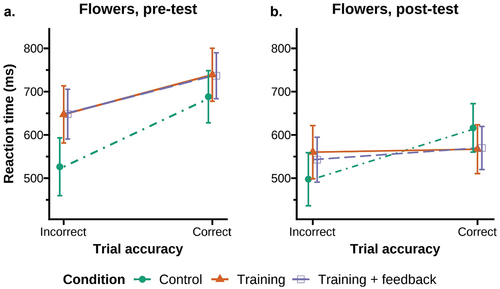
We were unable to run meaningful analyses on correct vs. incorrect RT in the Mixed block. In this block, there is the additional trial congruency to consider, as errors may occur on congruent or incongruent trials. Our sample did not commit sufficient errors to be able to differentiate between trial types and analyze RT accordingly and reliably (Schroder et al., Citation2020).
Post-error slowing
To further investigate the trial-by-trial adjustment in responding, we analyzed PES. Due to the low number of errors committed, we again could not disentangle the congruency effect in the Mixed block and run reliable and meaningful analyses for this block. We therefore present the results for the Flowers block only. These findings must still be interpreted with caution.
In the Flowers block at pretest, RT was longer on post-error than post-correct trials, indicating substantial PES in all three groups (control: estimate = −168.8, 95% CI [−252.0, −85.3], p < 0.001; training without feedback: estimate = −120.3, 95% CI [−204.0, −36.4], p = 0.005; training with feedback: estimate = −130.4, 95% CI [−203.0, −58.0], p < 0.001; see , solid lines). At posttest, PES persisted in the control group (estimate = −100.2, 95% CI [−185.0, −15.3], p = 0.021) and the training group with feedback (estimate = −123.6, 95% CI [−195.0, −52.7], p < 0.001), but not in the training group without feedback (p = 0.386). This indicates that the training group without feedback was able to make more proactive cognitive control adjustments at posttest than the other two groups.
Figure 8. Line graphs of the difference in reaction time between post-correct and post-error trials of the Flowers block at pre- and posttest.
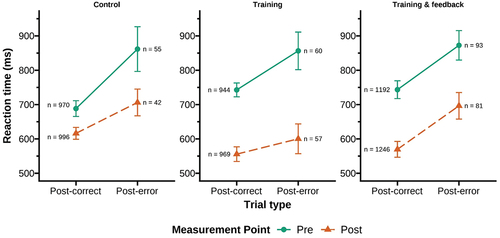
Discussion
We carried out a short intervention with first and second graders, exploring whether we can get children in this age range to use proactive cognitive control more often. We used the Flowers and Mixed blocks of the Hearts and Flowers task (HF) (Davidson et al., Citation2006; Diamond et al., Citation2007) at pre- and posttest, and different yet similar conflict tasks during the six intervention sessions. Our hypotheses were partly confirmed. R-C in the Flowers block improved in both training conditions, but no difference was found between the training conditions. And, in the more difficult Mixed block, only the training group without feedback improved from pre- to posttest. On impulsive errors, the training groups outperformed the control group, with no difference between the training conditions with and without feedback. With regard to PES, the training group without feedback developed smoother adjustments, that is, showed less exaggerated PES at posttest compared to the other two groups.
The tasks used for training in the training conditions, like the HF task used at pre- and posttest, were speeded-response interference inhibition tasks, calling for reactive as well as proactive cognitive control. The control condition’s training was a paired-associates memory task without timed responses. Participants in this group did still improve their R-C and decreased their RT from pre- to posttest, which could be attributed to a task-familiarity effect with the HF task. The increased improvements in the training conditions, then, can be interpreted as the intervention effect, above and beyond this familiarity effect. Thus, completing just six 10-minute training tasks has been sufficient to stimulate six- to eight-year-old Swiss children to increasingly select proactive cognitive control. Moreover, they were found to be efficient in their use of cognitive control, in that they responded faster at posttest than at pretest, but no less accurately.
Specifically, we saw that after participation in the intervention, participants in the training conditions learned to recognize the increased cognitive demand of the Mixed block. That is, they approached this task block generally more slowly to prevent them from making too many errors, as demonstrated by their longer RT in the Mixed compared to the Flowers block at posttest, which did not occur at pretest. Thus, the experience of participating in the training appears to spark – at least in the short term – the selection of surprisingly efficient proactive cognitive control in these children, whereas those in the control condition did not adjust their strategy in the HF task’s Mixed block at posttest. This finding is in line with a recent study by Kubota et al. (Citation2023), who found that EF training with and without added metacognitive training led to greater proactive cognitive control engagement in Scottish and German school children. By anticipating the increased difficulty and selecting proactive cognitive control, participants in both training conditions prevented more errors at posttest than they did at pretest.
This finding underlines that children in this age range are in principle able to adapt their strategy so that they better balance speed and accuracy. Although they may not spontaneously do so, repeated experiences with this type of task appears to lead them to demonstrate improved cognitive control skills. This finding must be viewed in the wider context of interventions to stimulate cognitive control in young children and can be viewed as an additional, rather than a conflicting, method to aid children’s development. For example, Doebel et al. (Citation2018) found that the use of language and labels helps children to employ more proactive cognitive control.
Early primary school children, unlike older children or adults, tend to keep their RT consistent when task difficulty increases – at the cost of reducing their accuracy (e.g. Davidson et al., Citation2006). This is indeed what we observed in all three groups at pretest: a drop in accuracy in the Mixed block compared to the Flowers block, whilst RT remained constant. However, at posttest, the training groups slowed down their RT in the Mixed block compared to the Flowers block and their accuracy remained more stable. This shows that participants in the training conditions used proactive cognitive control to make adjustments to their speed-accuracy trade-off. By doing so, the training group without feedback optimized their trade-off sufficiently to improve their R-C by a greater margin than participants in the control condition.
We hypothesized that the training group with feedback would outperform the training group without feedback, but no differences between the two training conditions were found for impulsive errors, PES, or R-C in the Flowers block, and the opposite occurred in the Mixed block, where the training group without feedback outperformed the group with feedback. This could be due to a variety of factors.
Firstly, the absence of a difference between the training conditions on several of our outcomes may be related to participants’ error recognition being sufficient to benefit from practice on the training tasks, even without feedback. This aligns with the idea that children in the sample’s age group are developmentally ready to begin making more frequent use of proactive cognitive control strategies, and that, most of all, they need to be provided opportunities to practice this (Braver, Citation2012; Chevalier, Citation2015). As we identified PES at pretest in all groups, this would indicate that participants were indeed aware they were making errors, as they slowed down as a result of committing these errors. Thus, providing this age group with specific opportunities to experience success as a result of selecting proactive cognitive control strategies may have been sufficient for both training conditions to trigger a development the children were ready for, as demonstrated by their improved performance on the Flowers R-C and impulsivity, and the block-level slowing down from the Flowers to the Mixed block at posttest.
Alternatively, the automated feedback delivered by the digital devices may not have provided additional motivation for the children to adjust their strategy selection. Although Wisniewski et al. (Citation2020) concluded that the feedback mode (e.g., oral, written, computer) does not lead to differences in its effects, Elliott et al. (Citation2020) highlight the role of the relationship between the teacher and the student in the effectiveness of feedback. Thus, the automated feedback integrated into the training tasks may not have had additional value beyond the opportunity to practice proactive cognitive control. Future studies should investigate if feedback provided in a more personal manner may yield greater results.
Another possibility is that participants performed within their error tolerance – which is plausible, considering their high accuracy rate. According to Metcalfe (Citation2017), in contexts where the occurrence of mistakes is fairly low, participants may perceive the commission of a few errors as non-problematic. This may affect the trade-off between speed and accuracy, whereby the child does not feel compelled to take action to reduce their (low) error rate and thus they are less likely to slow down their response time. And, as discussed above, through participating in the training, both groups did make adjustments to maximize their accuracy. Then, in the easier Flowers block, where error rates were particularly low, making participants aware of their infrequent errors may not have triggered additional changes to the speed-accuracy trade-off in the training condition with feedback compared to training condition without feedback, so that no difference on R-C scores between the two conditions was found. This could also explain why, in the more complex Mixed block, the group without feedback outperformed the group with feedback. As error rates were higher, the error tolerance may have been exceeded and the training group with feedback may have taken the feedback to “make sure you do not respond too quickly and make mistakes” to heart. This could lead to overcompensation; dropping their speed more than necessary to increase their accuracy and leading to a smaller increase in R-C than in the training condition without feedback.
Finally, the additional improvements in proactive cognitive control engagement in the condition without feedback may be explained by the feedback presenting an interruption to the independent selection of proactive cognitive control. As children in this age range are thought to become increasingly less reliant on external cues (Chevalier & Blaye, Citation2016; Chevalier et al., Citation2015), providing external cues in the form of feedback may have led these children to remain more reliant on this external feedback, rather than needing to internally monitor themselves. This is in line with the study by Hadley et al. (Citation2020) finding that 5-year-olds engaged the most proactive cognitive control when they were made to self-monitor their accuracy. And, as specified by Hattie and Timperley (Citation2007), direct feedback, such as employed in the present intervention, most effectively promotes the learning of new skills, but may interrupt automation and fluency. This also points to the possibility that participants in the condition with feedback remained reliant on the external cues provided by this feedback. Then, in a situation without feedback (i.e., the posttest HF task), those children who practiced without feedback may have been more able to independently engage proactive control. The training tasks without feedback may thus have been more effective in reducing PES in the Flowers block and improving R-C on the more challenging Mixed block, as participants were accustomed to self-monitoring their own performance rather than receiving external feedback.
Conclusion
In this study, we have demonstrated that a brief digital intervention, presented during a developmental period in which children are ready, can trigger acute accelerated employment of proactive cognitive control in cognitive tasks involving accuracy-speed trade-off. The game-based nature of the training tasks and their relatively short duration of around ten to fifteen minutes make the tasks enjoyable for the children and easy to implement for teachers. The lack of broader transfer tasks and of a follow-up are certainly limitations of the present study. But this study was realized as “a proof of principle” of the idea that during this developmental period, children shift from predominately reactive to more efficient proactive cognitive control. With that in mind, the present approach offers an explanation of why some interventions in this domain are found ineffective. In a next step, it will be crucial to find out whether children maintain the use of proactive rather than reactive cognitive control. In any case, training cognitive control strategies as meta-processes orchestrating and controlling EF, and as a developmental factor for academic performance early in a child’s school career, seems to be a useful tool for positively affecting their educational trajectory.
AFL_Fig_S1.jpg
Download JPEG Image (693.7 KB)AFL_Fig_S2.jpg
Download JPEG Image (553.9 KB)AFL_Fig_S3.jpg
Download JPEG Image (786.1 KB)Disclosure statement
No potential conflict of interest was reported by the author(s).
Supplementary material
Supplemental data for this article can be accessed online at https://doi.org/10.1080/15248372.2024.2363190
References
- Aksayli, N. D., Sala, G., & Gobet, F. (2019). The cognitive and academic benefits of cogmed: A meta-analysis. Educational Research Review, 27, 229–243. https://doi.org/10.1016/j.edurev.2019.04.003
- Ambrosi, S., Lemaire, P., & Blaye, A. (2016). Do young children modulate their cognitive control? Experimental Psychology, 63(2), 117–126. https://doi.org/10.1027/1618-3169/a000320
- Ambrosi, S., Servant, M., Blaye, A., & Burle, B. (2019). Conflict processing in kindergarten children: New evidence from distribution analyses reveals the dynamics of incorrect response activation and suppression. Journal of Experimental Child Psychology, 177, 36–52. https://doi.org/10.1016/j.jecp.2018.06.006
- Blair, C., & Raver, C. C. (2015). School readiness and self-regulation: A developmental psychobiological approach. Annual Review of Psychology, 66(1), 711–731. https://doi.org/10.1146/annurev-psych-010814-015221
- Botvinick, M. M., Braver, T. S., Barch, D. M., Carter, C. S., & Cohen, J. D. (2001). Conflict monitoring and cognitive control. Psychological Review, 108(3), 624–652. https://doi.org/10.1037/0033-295X.108.3.624
- Braver, T. S. (2012). The variable nature of cognitive control: A dual mechanisms framework. Trends in Cognitive Sciences, 16(2), 106–113. https://doi.org/10.1016/j.tics.2011.12.010
- Braver, T. S., Gray, J. R., & Burgess, G. C. (2007). Explaining the many varieties of working memory variation: Dual mechanisms of cognitive control. In A. R. A. Conway, C. Jarrold, M. J. Kane, A. Miyake, & J. N. Towse (Eds.), Variation in working memory (pp. 75–106). Oxford University Press.
- Brewer, N., & Smith, G. A. (1989). Developmental changes in processing speed: Influence of speed-accuracy regulation. Journal of Experimental Psychology: General, 118(3), 298–310. https://doi.org/10.1037/0096-3445.118.3.298
- Brocki, K. C., & Tillman, C. (2014). Mental set shifting in childhood: The role of working memory and inhibitory control. Infant and Child Development, 23(6), 588–604. https://doi.org/10.1002/icd.1871
- Camerota, M., Willoughby, M. T., & Blair, C. B. (2019). Speed and accuracy on the hearts and flowers task interact to predict child outcomes. Psychological Assessment, 31(8), 995–1005. https://doi.org/10.1037/pas0000725
- Chevalier, N. (2015). The development of executive function: Toward more optimal coordination of control with age. Child Development Perspectives, 9(4), 239–244. https://doi.org/10.1111/cdep.12138
- Chevalier, N., & Blaye, A. (2016). Metacognitive monitoring of executive control engagement during childhood. Child Development, 87(4), 1264–1276. https://doi.org/10.1111/cdev.12537
- Chevalier, N., Martis, S. B., Curran, T., & Munakata, Y. (2015). Metacognitive processes in executive control development: The case of reactive and proactive cognitive control. Cognitive Neuroscience, 27(6), 1125–1136. https://doi.org/10.1162/jocn_a_00782
- Chevalier, N., Meaney, J. A., Traut, H. J., & Munakata, Y. (2020). Adaptiveness in proactive control engagement in children and adults. Developmental Cognitive Neuroscience, 46(Article 100870), 100870. https://doi.org/10.1016/j.dcn.2020.100870
- Cortés Pascual, A., Moyano Muñoz, N., & Quílez Robres, A. (2019). The relationship between executive functions and academic performance in primary education: Review and meta-analysis. Frontiers in Psychology, 10(Article 1582). https://doi.org/10.3389/fpsyg.2019.01582
- Davidson, M. C., Amso, D., Anderson, L. C., & Diamond, A. (2006). Development of cognitive control and executive functions from 4 to 13 years: Evidence from manipulations of memory, inhibition, and task switching. Neuropsychologia, 44(11), 2037–2078. https://doi.org/10.1016/j.neuropsychologia.2006.02.006
- Destan, N., & Roebers, C. M. (2015). What are the metacognitive costs of young children’s overconfidence? Metacognition and Learning, 10(3), 347–374. https://doi.org/10.1007/s11409-014-9133-z
- Diamond, A. (2013). Executive functions. Annual Review of Psychology, 64(1), 135–168. https://doi.org/10.1146/annurev-psych-113011-143750
- Diamond, A., Barnett, W. S., Thomas, J., & Munro, S. (2007). Preschool program improves cognitive control. Science, 318(5855), 1387–1388. https://doi.org/10.1126/science.1151148
- Diamond, A., & Lee, K. (2011). Interventions shown to aid executive function development in children 4 to 12 years old. Science, 333(6045), 959–964. https://doi.org/10.1126/science.1204529
- Diamond, A., & Ling, D. (2020). Review of the evidence on, and fundamental questions about, efforts to improve executive functions, including working memory. In J. M. Novick, M. F. Bunting, M. R. Dougherty, & R. W. Engle (Eds.), Cognitive and working memory training: Perspectives from psychology, neuroscience, and human development (pp. 143–431). Oxford University Press. https://doi.org/10.1093/oso/9780199974467.003.0008
- Doebel, S., Barker, J. E., Chevalier, N., Michaelson, L. E., Fisher, A. V., & Munakata, Y. (2017). Getting ready to use control: Advances in the measurement of young children’s use of proactive control. Public Library of Science ONE, 12(4), e0175072. https://doi.org/10.1371/journal.pone.0175072
- Doebel, S., Dickerson, J. P., Hoover, J. D., & Munakata, Y. (2018). Using language to get ready: Familiar labels help children engage proactive control. Journal of Experimental Child Psychology, 166, 147–159. https://doi.org/10.1016/j.jecp.2017.08.006
- Dunning, D. L., Holmes, J., & Gathercole, S. E. (2013). Does working memory training lead to generalized improvements in children with low working memory? A randomized controlled trial. Developmental Science, 16(6), 915–925. https://doi.org/10.1111/desc.12068
- Elliott, V., Randhawa, A., Ingram, J., Nelson-Addy, L., Griffin, C., & Baird, J. (2020). Feedback: Practice review. Education Endowment Foundation.
- Hadley, L. V., Acluche, F., & Chevalier, N. (2020). Encouraging performance monitoring promotes proactive control in children. Developmental Science, 23(1), e12861. https://doi.org/10.1111/desc.12861
- Hagmann von Arx, P., & Grob, A. (2014). RIAS. Reynolds intellectual assessment scales and screening. Deutschsprachige adaptation der Reynolds Intellectual Assessment Scales (RIAS) & des Reynolds Intellectual Screening Test (RIST) von Cecil R. Reynolds und Randy W. Kamphaus. Huber.
- Hattie, J., & Timperley, H. (2007). The power of feedback. Review of Educational Research, 77(1), 81–112. https://doi.org/10.3102/003465430298487
- Heemskerk, C. H. H. M., & Roebers, C. M. (2023). Executive functions and classroom behaviour in second graders. Frontiers in Education, 8, 1141586. https://doi.org/10.3389/feduc.2023.1141586
- Holmes, J., Gathercole, S. E., & Dunning, D. L. (2009). Adaptive training leads to sustained enhancement of poor working memory in children. Developmental Science, 12(4), F9–15. https://doi.org/10.1111/j.1467-7687.2009.00848.x
- Jentzsch, I., & Leuthold, H. (2006). Short article: Control over speeded actions: A common processing locus for micro- and macro-trade-offs? Quarterly Journal of Experimental Psychology, 59(8), 1329–1337. https://doi.org/10.1080/17470210600674394
- Kamijo, K., Bae, S., Masaki, H., & DiRusso, F. (2016). The association of childhood fitness to proactive and reactive action monitoring. PLoS, 11(3), e0150691. https://doi.org/10.1371/journal.pone.0150691
- Kang, W., Hernández, S. P., Rahman, M. S., Voigt, K., & Malvaso, A. (2022). Inhibitory control development: A network neuroscience perspective. Frontiers in Psychology, 13(Article 651547). https://doi.org/10.3389/fpsyg.2022.651547
- Kassai, R., Futo, J., Demetrovics, Z., & Takacs, Z. K. (2019). A meta-analysis of the experimental evidence on the near- and far-transfer effects among children’s executive function skills. Psychological Bulletin, 145(2), 165–188. https://doi.org/10.1037/bul0000180. PMID: 30652908.
- Korucu, I., Litkowski, E., & Schmitt, S. A. (2020). Examining associations between the home literacy environment, executive function, and school readiness. Early Education and Development, 31(3), 455–473. https://doi.org/10.1080/10409289.2020.1716287
- Kubota, M., Hadley, L. V., Schaeffner, S., Könen, T., Meaney, J. A., Morey, C. C., Auyeung, B., Moriguchi, Y., Karbach, J., & Chevalier, N. (2023). The effect of metacognitive executive function training on children’s executive function, proactive control, and academic skills. Developmental Psychology, 59(11), 2002–2020. https://doi.org/10.1037/dev0001626
- Lenth, R. (2023). emmeans: Estimated marginal means, aka least-squares means. ( Computer Program; Version 1.8.9). https://CRAN.R-project.org/package=emmeans
- Magalhães, S., Carneiro, L., Limpo, T., & Filipe, M. (2020). Executive functions predict literacy and mathematics achievements: The unique contribution of cognitive flexibility in grades 2, 4, and 6. Child Neuropsychology, 26(7), 934–952. https://doi.org/10.1080/09297049.2020.1740188
- McCoy, D. C., Koepp, A. E., Jones, S. M., Bodrova, E., Leong, D. J., & Deaver, A. H. (2022). An observational approach for exploring variability in young children’s regulation-related skills within classroom contexts. Developmental science, 25, e13250. https://doi.org/10.1111/desc.13250 https://doi.org/10.1111/desc.13250
- Metcalfe, J. (2017). Learning from errors. Annual Review of Psychology, 68(1), 465–489. https://doi.org/10.1146/annurev-psych-010416-044022
- Micalizzi, L., Brick, L. A., Flom, M., Ganiban, J. M., & Saudino, K. J. (2019). Effects of socioeconomic status and executive function on school readiness across levels of household chaos. Early Childhood Research Quarterly, 47(2), 331–340. https://doi.org/10.1016/j.ecresq.2019.01.007
- Morgan, P. L., Farkas, G., Wang, Y., Hillemeier, M. M., Oh, Y., & Maczuga, S. (2019). Executive function deficits in kindergarten predict repeated academic difficulties across elementary school. Early Childhood Research Quarterly, 46, 20–32. https://doi.org/10.1016/j.ecresq.2018.06.009
- Nesbitt, K. T. & Farran, D. C.(2022). Executive function skills and classroom behaviors of U.S. Prekindergartners with special needs [original research]. Frontiers in Education, 7, 447. https://doi.org/10.3389/feduc.2022.944224
- Pauli-Pott, U., Mann, C., & Becker, K. (2021). Do cognitive interventions for preschoolers improve executive functions and reduce ADHD and externalizing symptoms? A meta-analysis of randomized controlled trials. European Child & Adolescent Psychiatry, 30(10), 1503–1521. https://doi.org/10.1007/s00787-020-01627-z
- Pinheiro, J., Bates, D., & Team, R. C. (2022). Nlme: Linear and nonlinear mixed effects models. (Computer Program; Version 3.1-157). https://CRAN.R-project.org/package
- Redick, T. S., Shipstead, Z., Harrison, T. L., Hicks, K. L., Fried, D. E., Hambrick, D. Z., Kane, M. J., & Engle, R. W. (2013). No evidence of intelligence improvement after working memory training: A randomized, placebo-controlled study. Journal of Experimental Psychology: General, 142(2), 359–379. https://doi.org/10.1037/a0029082
- Reynolds, C. R., & Kamphaus, R. W. (2003). Rias. Reynolds Intellectual Assessment Scales.
- Robson, M. S., Allen, D. A., & Howard, S. J. (2020). Self-regulation in childhood as a predictor of future outcomes: A meta-analytic review. Psychological Bulletin, 146(4), 324–354. https://doi.org/10.1037/bul0000227
- Roebers, C. M. (2017). Executive function and metacognition: Towards a unifying framework of cognitive self-regulation. Developmental Review, 45, 31–51. https://doi.org/10.1016/j.dr.2017.04.001
- Roebers, C. M. (2022). Six-to eight-year-olds’ performance in the heart and flower task: Emerging proactive cognitive control. Frontiers in Psychology, 13(Article 923615). https://doi.org/10.3389/fpsyg.2022.923615
- Rosas, R., Espinoza, V., Porflitt, F., & Ceric, F. (2019). Executive functions can be improved in preschoolers through systematic playing in educational settings: Evidence from a longitudinal study. Frontiers in Psychology, 10(Article 02024). https://doi.org/10.3389/fpsyg.2019.02024
- RStudio Team. (2022). RStudio: Integrated development environment for R (Computer program). http://www.rstudio.com/
- Schroder, H. S., Nickels, S., Cardenas, E., Breiger, M., Perlo, S., & Pizzagalli, D. A. (2020). Optimizing assessments of post‐error slowing: A neurobehavioral investigation of a flanker task. Psychophysiology, 57(2), e13473. https://doi.org/10.1111/psyp.13473
- Shipstead, Z., Redick, T. S., & Engle, R. W. (2012). Is working memory training effective? Psychological Bulletin, 138(4), 628–654. https://doi.org/10.1037/a0027473
- Simon, J. R. (1990). The effects of an irrelevant directional cue on human information processing. Advances in Psychology, 65, 31–86. https://doi.org/10.1016/S0166-4115(08)61218-2
- Smulders, S. F. A., Soetens, E., & van der Molen, M. W. (2016). What happens when children encounter an error? Brain and Cognition, 104, 34–47. https://doi.org/10.1016/j.bandc.2016.02.004
- Spiegel, J. A., Goodrich, J. M., Morris, B. M., Osborne, C. M., & Lonigan, C. J. (2021). Relations between executive functions and academic outcomes in elementary school children: A meta-analysis. Psychological Bulletin, 147(4), 329–351. https://doi.org/10.1037/bul0000322
- Takacs, Z. K., & Kassai, R. (2019). The efficacy of different interventions to foster children’s executive function skills: A series of meta-analyses. Psychological Bulletin, 145(7), 653–697. https://doi.org/10.1037/bul0000195
- Van Loon, M. H., & Roebers, C. M. (2020). Using feedback to improve monitoring judgment accuracy in kindergarten children. Early Childhood Research Quarterly, 53(4), 301–313. https://doi.org/10.1016/j.ecresq.2020.05.007
- Wisniewski, B., Zierer, K., & Hattie, J. (2020). The power of feedback revisited: A meta-analysis of educational feedback research. Frontiers in Psychology, 10(Article 3087). https://doi.org/10.3389/fpsyg.2019.03087
- Woltz, D. J., & Was, C. A. (2006). Availability of related long-term memory during and after attention focus in working memory. Memory & Cognition, 34(3), 668–684. https://doi.org/10.3758/BF03193587