Abstract
Psychiatry is rapidly adopting digital phenotyping and artificial intelligence/machine learning tools to study mental illness based on tracking participants’ locations, online activity, phone and text message usage, heart rate, sleep, physical activity, and more. Existing ethical frameworks for return of individual research results (IRRs) are inadequate to guide researchers for when, if, and how to return this unprecedented number of potentially sensitive results about each participant’s real-world behavior. To address this gap, we convened an interdisciplinary expert working group, supported by a National Institute of Mental Health grant. Building on established guidelines and the emerging norm of returning results in participant-centered research, we present a novel framework specific to the ethical, legal, and social implications of returning IRRs in digital phenotyping research. Our framework offers researchers, clinicians, and Institutional Review Boards (IRBs) urgently needed guidance, and the principles developed here in the context of psychiatry will be readily adaptable to other therapeutic areas.
INTRODUCTION
A University research team is developing an app to conduct a digital phenotyping study of mental illness in young adults. The goal of this study is to better understand the factors that increase or decrease the likelihood of symptoms of severe mental illness, paving the way for more effective interventions. Using smart watches and smart phones, multiple streams of data will be collected and then analyzed by artificial intelligence/machine learning: GPS location, phone/screen/Internet usage, phone logs, text logs, and content of social media posts, web search queries, and text messages; sleep, 24/7 continuous measurement of movement and physical activity, and heart rate. Virtual video interviews with the research team will deploy multiple validated clinical instruments. In addition to knowing when symptoms of mental illness are abating or rising, the research team also anticipates that they will likely be able to know other information, for example when participants are involved in activities such as using illicit drugs, having sex, buying liquor, driving while drunk, visiting pornography websites, and having suicidal ideations. The digital phenotyping research team has the technology to facilitate return of individualized results to each participant, on a monthly, weekly, daily, or even more frequent interval. But the research team is unsure about how to formulate its protocol for return of Individual Research Results (IRRs), especially since the research may have concurrent validity (i.e., the relationship between past observed behavior and mental states), but will not yet have predictive validity (i.e., the ability to predict future behavior and mental states). The researchers need to know: When, in what format, and with what additional guidance should IRRs be returned to participants in this digital phenotyping study of mental illness, if at all?
The question posed in this opening vignette is urgent as psychiatric research and practice are rapidly embracing digital phenotyping methods (Gratzer et al. Citation2021; Torous et al. Citation2019; Liang, Zheng, and Zeng Citation2019; Onnela and Rauch Citation2016). Data gathered from smart phones, smart watches, other wearables, implantables, social media, and virtual-reality (VR) activity, combined with additional individualized data such as brain scans (Ressler and Williams Citation2021; Camacho et al. Citation2021) and clinical assessments (Baker et al. Citation2018; Torous et al. Citation2016) may lead to transformative clinical applications (Huckvale, Venkatesh, and Christensen Citation2019; Onnela Citation2021). Digital phenotyping in psychiatry utilizes large multimodal datasets to assess and even predict mental conditions. Digital phenotyping also offers researchers opportunities to intervene in real time to modify participant behavior and may necessitate high-stakes decision-making about returning results on a short timeframe, thus presenting novel issues in returning IRRs that are critical to address.
Digital phenotyping has been defined by Torous et al. (Citation2016) as the “moment-by-moment quantification of the individual-level human phenotype in-situ using data from smartphones and other personal digital devices” and more broadly by Martinez-Martin et al. (Citation2018) as “approaches in which personal data gathered from mobile devices and sensors … [are] analyzed to provide health information.” In this article we use the term “digital phenotyping” broadly to refer to research that might combine some or all of (1) smart phone and social media data; (2) data from wearables and implantables, (3) data from ambient sensors such as smart home sensors; (4) brain data, including magnetic resonance imaging (MRI), electroencephalography (EEG), functional near-infrared spectroscopy (fNIRS) and other modalities; and (5) clinical assessments. The term “digital phenotyping” has become a term of art in multiple fields, and as this list shows, includes non-digital assessments such as biological measures and clinical assessments as potential components of digital phenotyping. We include clinical assessments because current research is already utilizing data from wearables alongside, and integrated with, more traditional clinical assessments (Torrado et al. Citation2022). As Melcher, Hays, and Torous (Citation2020) have pointed out in the context of college mental health, “Data from smartphones paired with clinical assessment data can reveal what behaviors or combination of behaviors are correlated with mental health problems …” [emphasis added]. The specific clinical assessments to be included in a digital phenotype will vary across research contexts. Our broad definition of “digital phenotyping” can be read to overlap with alternative terms such as deep phenotyping, computational phenotyping, deep biobehavioral-typing, and deep geno/phenotyping (Baker Citation2019; Shen et al. Citation2022). We also use the term phenotyping in this article interchangeably with “phenotypic assays” and “phenotype”.
Digital phenotyping offers an opportunity to collect and analyze multimodal individualized data streams of real-world behavior (Jain et al. Citation2015; Baker et al. Citation2018; Huckvale, Venkatesh, and Christensen Citation2019) and virtual-reality behavior (Freeman et al. Citation2017). A digital phenotyping study in psychiatry might include many different types of data streams, some potentially collected continuously and in real time (Box 1). For instance, so long as a participant’s GPS-enabled smart phone is near them, that participant’s location data is available to the research team. In addition, there is a range of passive and active data collection involved in phenotyping research. Once an app, for instance, is installed and preferences on data sharing are set, participants’ GPS data is sent to the research team passively. By contrast, that same participant might be actively responding to daily questions about mood.
Advocates of digital phenotyping for psychiatric research suggest that digital collection of real-world data might offer the possibility of increased participant pools, lower costs, and continuous and perhaps more objective data on psychiatric symptoms (Smith Citation2018; Miller et al. Citation2022). Although digital phenotyping approaches are being explored in many areas of medicine including oncology (Blom et al. Citation2021), cardiology (Nasir and Khera Citation2020), and surgery (Jayakumar et al. Citation2020), we focus here on the use of digital phenotyping in mental and behavioral health (Davidson Citation2022; Hsin et al. Citation2018), with considerations that can be applied to other fields.
Disorders in psychiatry are often defined as patterns of behavior, but reliable data on real-world behavior have been elusive using traditional methods (Insel Citation2017), and therefore digital phenotyping offers distinct advantages. Widespread collection of real-world behavioral data, however, raises ethical and legal issues because psychiatric data are often sensitive and potentially stigmatizing; psychiatric disease severity may directly impact decision making capacity and self-awareness (e.g., during a manic episode or severe psychosis); and digital phenotyping data, such as data suggesting illicit drug use or suicidal ideations, may have legal implications including criminal prosecution, involuntary hospitalization, or third-party interventions such as “wellness checks” by police or emergency services.
In this article, we address a challenging and unresolved issue in digital phenotyping: the return of specific individual research results (IRRs). Return of individual and aggregate research results is a well-known issue (The Multi-Regional Clinical Trials (MRCT) Center Citation2017, Citation2023; Burke, Evans, and Jarvik Citation2014; Lévesque, Joly, and Simard Citation2011). Multiple scholars have argued that there is an ethical duty to allow participants to receive results in certain types of research (Knoppers et al. Citation2006; Faucett and Davis Citation2016), and multiple empirical studies clearly show that when asked, participants overwhelmingly express a desire to have individual research results returned (Facio et al. Citation2013; Yu et al. Citation2013). The advent of digital health data, along with more calls for open science, has increased the urgency and interest in addressing this return of results issue (Wong, Hernandez, and Califf Citation2018; Botkin et al. Citation2018). But although a growing body of research on the ethical, legal, and social implications (ELSI) of digital phenotyping has emerged (Martinez-Martin et al. Citation2018; Khodyakov et al. Citation2019; Torous et al. Citation2016; Nebeker, Bartlett Ellis, and Torous Citation2020; Marsch Citation2018), the literature has yet to provide guidance for how digital phenotyping results should be returned in real-world settings.
To address this gap, we convened an interdisciplinary, multi-institutional, expert working group, supported by the National Institute of Mental Health (NIMH). The working group began its work with consideration of existing ethical and legal guidance. In genetics and genomics, considerable progress has been made in establishing principles and guidelines for when and how to share individual and group research results, as well as incidental findings, with participants (MRCT Center 2017, 2023; Clayton and McGuire Citation2012; Holm et al. Citation2014; Wolf et al. Citation2008; Jarvik et al. Citation2014). These established guidelines and the emerging norm of returning results in participant-centered research meaningfully inform return of results in digital phenotyping research but are insufficient on their own.
Informed by existing recommendations on the return of research results generally, with particular focus on return of results in genetic research (MRCT Center 2017, 2023; Jarvik et al. Citation2014; Botkin et al. Citation2018; Burke, Evans, and Jarvik Citation2014; Clayton and McGuire Citation2012; Holm et al. Citation2014; Knoppers et al. Citation2006; Wolf Citation2013; Lázaro-Muñoz, Torgerson, and Pereira Citation2021), we developed an ethical framework for return of IRRs in digital phenotyping research in psychiatry. We do not claim here that return of IRRs in digital phenotyping is categorically unique. Indeed, as we discuss below, many challenges associated with return of IRRs in genetics/genomics are present in return of IRRs in digital phenotyping research in psychiatry, including uncertainty about the validity of IRRs, effective communication of complex results to participants in meaningful ways, and the impact of return of IRRs on third parties such as family members. But we do argue that several features of return of IRRs in digital phenotyping research in psychiatry distinguish it qualitatively and quantitatively from return of IRRs in genetics, including: the many streams of IRRs that could be returned, at many intervals (weekly, daily, hourly); the ability of digital phenotyping IRRs to change behavior in both intentional/foreseen and unintentional/unforeseen ways (as compared to return of genetics IRRs, which does not change the genotype); and the wider population of people that may be affected by return of IRRs.
We begin by proposing a defined, common terminology to guide researcher decision-making and institutional review board (IRB) review of return of IRR policies. With key definitions in place, we then discuss several aspects of digital phenotyping research that have implications for decisions about return of IRRs. We present a novel Framework for Returning IRRs in Digital Phenotyping Research. The framework is grounded in the core bioethics principles of balancing benefit vs. risk, respect for persons, and promoting justice in returning data. We recommend how the framework can be used to foster dialogue around the Who, What, Where, When, and How of returning results in digital phenotyping research.
TERMINOLOGY
We recommend using a defined, common terminology to inform researcher decision-making and institutional review board (IRB) review of return of IRR policies. There is significant variation in the terms used to describe both research methods (e.g., digital phenotyping, computational phenotyping, computational psychiatry) and the content to return (e.g., results, data, raw data, interpreted data, information of value). Establishing consistent, defined, and shared terminology is important for effective communication and decision-making within and between research teams, IRBs, clinicians, and participants.
In we present definitions for key language relevant to return of IRRs in digital phenotyping in psychiatry. In laying out these terms, we aim to articulate meaningful differences within concepts that merit special consideration when returning results.
Table 1. Definition of terminology related to returning IRRs in digital phenotyping research, presented in alphabetical order.
Our proposed terminology makes clear that the term “data” is not synonymous with “result.” Data is a term that, without qualification, can lead to unnecessary confusion. We suggest differentiating “data” from “raw data,” “processed data,” and “interpreted data,” and then various sub-categories of “results” (). While categorizing data may sometimes be difficult, there are meaningful differences that will advance clarity in determining the details of a return of IRRs protocol and the language and methods to be deployed in communicating with participants throughout the research process.
The key distinction between raw and processed data is that the latter have undergone cleaning and processing. Processing of data may be conducted by multiple parties, including the research team, automation with AI/ML, a third party, and/or the research participant themselves. Examples of initial processing include using raw GPS location data to count the number of times someone left the house and using accelerometer data to track how many steps walked in a day.
The distinction between processed data and interpreted data is that, through analysis and interpretation, the processed data is given meaning. For example, a summary of the number of hours slept per day would be processed data, but it would become interpretated data (and hence a “result”) when that average sleep number is compared to the individual’s previous sleep patterns, compared to a national average, or used (in conjunction with other interpreted data) to make a diagnosis.
Some processed data are analyzed by automated pipelines or algorithms without a human reviewing the data first and may thus create different moral obligations than manually processed data. To illustrate, researchers are gaining the capability to apply artificial intelligence techniques to large amounts of personal sensor data in order to detect mood, affect, and the presence of mental disorders (Mohr, Zhang, and Schueller Citation2017). Supervised learning, unsupervised learning, and deep learning techniques are expanding in this space, but as Mohr, Zhang, and Schueller (Citation2017) note, “the availability of easy-to-use tools for machine learning is expanding faster than the expertise, resulting in a growing number of publications using questionable methods.” For return of IRRs, the implication is that more caution may be warranted in returning data and results that were processed solely by a machine learning tool without a human in the loop.
A “result” is interpreted data at the individual or group level. We define an “Individual Research Result” as any “result” (i.e., “interpreted data”) or “finding” (primary, incidental, secondary, exploratory) about the individual research participant collected or generated by the research team as part of the research project. In some medical contexts, an interpretation might be synonymous with a diagnosis, e.g., processed data from an MRI indicates a subdural hematoma. But a result is not always a diagnosis, e.g., while a sudden change in sleep pattern may increase concern for mood or medication changes, it is not a diagnosis. As a nonclinical example, basic research utilizing personality assessments may reach a conclusion that someone is high in extraversion or openness or emotional stability, relative to the population. As these examples illustrate, interpretating data typically involves correlation with other collected information outside the dataset (e.g., medical literature, pattern recognition) and/or implicit or explicit comparisons to population norms.
CONSIDERATIONS IN RETURNING IRRS IN DIGITAL PHENOTYPING IN PSYCHIATRY
Despite the growth of digital phenotyping research, and recognition that return of results should be addressed (Khodyakov et al. Citation2019; Wilkins et al. Citation2019), there has been no published guidance about return of IRRs in digital phenotyping research. In formulating such guidance, we start with the recognition that established guidelines for return of IRRs generally in biomedical research, and the emerging norm of returning results in participant-centered research, can meaningfully inform decision making by Institutional Review Boards, researchers, and participants. We ground our discussion here in the vignette presented at the outset, and utilize the terminology as defined in .
We note three aspects of digital phenotyping research that have implications for decisions about return of IRRs: (1) a very large number and various types of results could be returned, with much of the data collected passively and many results having the potential to affect participant behavior and health, or reveal socially stigmatized, politically sensitive, or legally relevant behavior; (2) great uncertainty about the actionability, analytical validity, clinical validity, personal utility of many of the results, including potential bias in the AI/ML systems being used to model the data and facilitate interpretation; and (3) significant risks to third parties from unintentional secondary collection of data from those third parties through the digital phenotyping tools.
Digital Phenotyping Tools Passively Collecting Large Amounts of Sensitive Data
Digital phenotyping research utilizes large amounts of data from multiple sources (Box 1). Moreover, much of these data will be collected passively. For example, as distinct from psychiatric research that requires participants to consciously engage with a researcher or research tool (e.g., participants are speaking to a researcher in an interview or completing a survey), in some digital phenotyping research, a single consent to have their electronic devices monitored could give researchers access to continuously gathered data such as: every website visited, image viewed, call made, text sent, physical location visited, and step taken. While the informed consent process would be a safeguard to ensure that participants agree to ongoing data collection, research suggests that after a period of adjustment, participants in digital phenotyping studies may revert to their normal behavioral patterns even though they are now being monitored (Mittelstadt Citation2017; Essén Citation2008; van Hoof et al. Citation2011).
It is not only the amount of data collected, but the types of data (and hence the types of results) involved that make return of IRRs challenging. Casting a net so wide that it includes every text and email someone sends, and every physical location and every website someone visits, makes it more likely that those conducting phenotyping research may collect information that can create a revealing snapshot of certain behaviors and choices that may be socially stigmatized, politically sensitive, or have legal implications.
For example, the research team described in the opening vignette might learn if a person in recovery from addiction has purchased liquor, if symptoms of a mental disorder are manifesting, if a married participant is having sex in someone else’s home, or if child pornography images are viewed. At the same time, the research team will learn potentially more innocuous details about their participants such as how many steps are taken each day, how participants are sleeping, and where participants like to hang out on the weekend. The research team might also be able to infer, in real time, rare medical events such as if the participant falls and is not able to move. A policy for the return of IRRs must thus be flexible to accommodate this broad range of IRRs that could be returned.
The policy for return of IRRs will also need to be cognizant of how the return of raw, processed, or interpreted data may affect participants. For instance, there is a deep literature on mental health stigma (Parcesepe and Cabassa Citation2013; Sickel, Seacat, and Nabors Citation2014), its serious consequences (Sharac et al. Citation2010), and efforts to reduce it (Yanos et al. Citation2015). To be clear, we do not describe here a research agenda for empirically assessing how return of IRRs affects participant behavior, e.g., how return of IRRs could serve as a psychiatric intervention or change behavior, though we view such a future research agenda to be valuable. We focus here instead on the current situation in which researchers need to develop polices on IRR despite the paucity of research on the impact that IRRs will have on those who receive them.
In the context of digital phenotyping research, a return of results approach should consider the dynamic relationship between public and personal stigma and self-stigma, and the results being returned. In the context of mental disorders, “personal” or “public” stigma is recognized as the attitudes that others have toward those thought to have a mental illness, while self-stigma is the individual’s internalized perception of public stigma, both actual (that is, an accurate internal perception of the public stigma) and perceived (Vogel et al. Citation2013). These two types of stigma are interrelated (Latalova, Kamaradova, and Prasko Citation2014). Relevant to return of IRRs, the potential for exacerbating the impact of public stigma exists if the returned data becomes known to others and contributes to negative attitudes about the individual receiving the results. For instance, imagine that a smart phone app sends an alert when a participant’s data suggests that the participant may wish to see a mental health provider. If others, such as classmates or coworkers who regularly spend time with the participant, see or hear the alert, it could lead them to stigmatize the participant, and thus for the participant to be impacted by public stigma in this environment. Even if others don’t actually stigmatize the participant, if a participant anticipates that they will or might, it could lead to increased self-stigma.
In addition, some of the results could expose an individual to criminal liability and other serious repercussions such as loss of employment. Digital phenotyping data can find, document, and preserve direct evidence of statutory violations, for instance being outside one’s house after a city-imposed curfew, excessive speeding, and being a registered sex offender frequenting school playgrounds.
To illustrate, consider a situation where the research team infers that one of their participants is spending significant time at a bar drinking alcohol, and that on days when he drinks, he is much more likely to yell vulgarities at or threaten his family (measured through in-home sensors). The researchers could return results to this participant via smart phone on a daily, weekly, monthly basis, or even could program their system to send results in real-time. There are also many choices for how to communicate the result. For example, the participant could receive a notification indicating not only the purchase of alcohol but also the amount of time at the bar, as well as messages such as, “You are in the top 1% of all adults for number of hours spent drinking in a bar,” or “It looks like you’re going to the bar a lot. And on days when you go the bar, your behavior at home changes too. Please remember that consuming too much alcohol can lead to serious health problems.” The research team could also communicate with the participant about resources that might be of interest. And of course, the research team could do nothing during the course of the study, but 2 years later, after the study has been published, send a full report discussing the (now 2 years old) behavior. The bottom line is that with so much sensitive data being collected, and so many ways to return results, the return of IRRs policy should address how it is anticipated participants may respond—and what, if any, responses the research team wishes to promote. Given the absence of research on the efficacy of these various methods, we do not promote a particular method of returning IRRs as the preferred one a priori. As discussed below, we do recommend that an initial method be chosen prior to conducting the study, and then the return of IRRs approach should be reviewed at least annually. This balance of structure and flexibility is appropriate given how little is known about what effect return of IRRs will have on behavior and well-being.
Uncertainty About Analytical Validity, Clinical Validity, Actionability, Clinical Utility, and Personal Utility
Because digital phenotyping research is utilizing new combinations of data streams and novel analytical techniques on those data (e.g., automatic speech recognition in psychotherapy, Miner et al. Citation2020), there is likely to be much uncertainty about the analytical validity, clinical validity, actionability, and personal utility of the results. While genetic data now have benchmarks for reliability of the raw data itself (or the calls at least), there are no benchmarks for most digital phenotyping data streams, nor for the techniques that are assembling them. For instance, consider the opening vignette, where a research team may be able to infer when a participant has used, or is at risk of using, illicit drugs. This inference might arise from the participant’s geographic location (e.g., first visiting an ATM and remaining there for the time it takes to withdraw cash, then visiting a location known for drug sales, sudden change in blood pressure and heart rate associated with heroin use, and text message activity consistent with a drug sale).
In order to make such an inference, the research team would need to ensure analytical validity of the relevant measures: the GPS device is accurately measuring the participant’s GPS location, the heart rate and blood pressure measures are not fluctuating due to equipment malfunction or another cause, such as exercise; and that the measure of text message activity is reliably tracking actual text message usage. It is not hard to imagine challenges with analytical validity, e.g., the blood pressure measurement device gets jostled when the participant pedals a bicycle, so there is a higher blood pressure reading even though the participant’s blood pressure has not actually changed.
Even with analytical validity, the results will not have clinical validity unless they can accurately and reliably detect or predict a relapse of heroin use. It could be that a certain combination of heart rate, movement patterns, and text messaging activity may be indicative of a health concern. Or it could be indicative of nothing. Many digital phenotyping studies aim to establish correlation and predictive validity; returning IRRs in real-time may be premature.
Further complicating return of results are concerns regarding bias and fairness in models of behavioral interpretation and prediction. For example, racial bias has been identified in some widely-used health care algorithms (Obermeyer et al. Citation2019; Buolamwini and Gebru Citation2018; Goodman, Goel, and Cullen Citation2018), and concerns regarding bias and fairness are relevant to the data and analyses used for digital phenotyping (Martinez-Martin, Greely, and Cho Citation2021; Mulvenna et al. Citation2021). When datasets are not representative of the populations in which they will be used, which is common due to lack of diversity and representativeness in research enrollment, then the resulting tools may not be as useful, or could even be harmful, for the groups not represented in the research.
Digital phenotyping can utilize data streams that rely on linguistic interpretation (e.g., electronic health records, social media posts) or categorization of specific activity (e.g., video, movement), both of which may be more subject to misinterpretation or mislabeling, or simply missing data, for minoritized populations (Gianfrancesco et al. Citation2018; Haque, Milstein, and Fei-Fei Citation2020; Martinez-Martin, Greely, and Cho Citation2021). To date, little data is available on the representativeness of digital phenotyping samples, and this raises the question of what data should not be returned until and unless sufficiently representative samples are used, and when a sample is sufficiently representative across which demographics. To improve representativeness, the design and implementation of digital phenotyping data collection tools will need to take into account differences in how devices may be used in different communities. These differences can impact equitable data collection and analysis across diverse groups, such as race, gender, ethnicity, religion, socioeconomic or disability status.
In addition, the research team will still need to evaluate actionability. For instance, if the research team can accurately and reliably predict when a participant is at risk of using heroin, then the result would be actionable because there are certainly well-established treatment regimes for substance-use disorders, and knowledge of risk factors for relapse could guide participant decision-making, e.g., a participant might seek treatment for addressing the substance-use disorder or choose to act differently in order to mitigate risk of relapse.
But even if the results are not “clinically actionable,” they may be actionable if the result has personal utility for the participant. For instance, assume that the research team has established the analytical validity of its sleep measurements, GPS tracker, and measure of text message activity. Further assume that the research team has correlated location data and text messaging with a change in sleep patterns—when the participant visits a certain location and text volumes increase after 7 pm, they go to sleep later and wake up later—but have not yet been able to reliably connect these measures to risk of drug use. This result, showing a relationship between sleep patterns and text/GPS, might have personal utility for the participant because it improves the participant’s self-understanding of their daily rhythms and might also inform their future decision-making about how to optimize their sleep habits.
Guidance for return of IRRs in digital phenotyping will have to acknowledge this uncertainty concerning analytical validity, clinical validity, actionability, clinical utility, and personal utility, but it can consider existing guidance from related fields where uncertainty has been addressed. For instance, the American College of Medical Genetics and Genomics (ACMG) developed (Richards et al. Citation2008) and refined (Richards et al. Citation2015) guidance for the interpretation of sequence variants, acknowledging both the continuum of clinical significance from benign to pathogenic as well as the rapid evolution of understanding. This approach has been used by the ACMG to recommend whether and which genetic variants should be returned to participants and patients (ACMG Board of Directors Citation2012; Miller et al. Citation2021; Green et al. Citation2013).
As in other new fields, clinical validity and clinical utility are likely to increase over time. This suggests that research teams, in consultation with their IRBs, may need to consider updating return of IRRs protocol over the course of the study. For example, midway through a research study the research team may feel more confident that a certain pattern in the data is meaningful for predicting a health outcome. Accuracy and reliability of results will also vary by the level of analysis. For example, although historical weather patterns cannot tell us the exact day of a snowstorm, they can reliably tell us that, on average, winter will be colder than summer. Similarly, digital phenotyping data might be able to identify group-averaged patterns of heightened risk for relapse, even if those models are not predictive of a particular individual’s relapsing behavior.
A cross-cutting issue related to validity is the possibility that results were generated by an AI/ML system that was trained on data that were not sufficiently representative of the relevant characteristics of the participants in the present study. It is well recognized that non-representative training data may introduce biases into medical AI (Food and Drug Administration Center for Devices & Radiological Health Citation2021; Vokinger, Feuerriegel, and Kesselheim Citation2021). For return of IRRs, the question is whether it is a prerequisite for returning IRRs generated by AI is that the AI system must be trained on data that is inclusive of the populations being studied in the present research study. While such a bright line approach might prevent potentially biased results from being returned, it also could lead to inequities in return of IRRs because such a policy would prevent return of IRRs in studies with more diverse populations but allow return of IRRs in research with more homogenous populations. To balance these concerns, we believe the best approach is to proceed with caution. In some instances, for example of high potential benefit to a participant, it may be ethical to return IRRs even if the results were generated by an AI/ML system with unknown representativeness or lack of representativeness in its training data. In this setting, however, the limits of predictability (or validity) and potential for bias should be explained to participants as part of the returned result.
We think this approach of cautious application while explaining limitations has a relevant precedent in sub-specialty medical care. In sub-specialty medical care, it is sometimes felt to be appropriate to cautiously apply information gained from one population (e.g., a general adult population) to guide the care of another target population (e.g., a geriatric population, or an adolescent population, or a population with rare health conditions) when the relevant information on the target population does not exist (as is often encountered in geriatric psychiatry or child psychiatry, for example).
Risk to Third Parties
Digital phenotyping research has the ability to collect highly sensitive information, not only from research participants but also from individuals who come into contact with the research participant. For instance, a study using Bluetooth proximity data might identify many others whose GPS could be correlated to a place of illegal drug use. Or a research participant might be included as a recipient on an email in which the sender seems to be making a threat and has attached a photo of the sender with a stockpile of guns. In these hypothetical situations, the research team would be confronted with the challenge of what, if anything, to do with regard to third parties—those who may not have a connection to the research—implicated by the data being collected and possibly returned.
These effects on third parties are not unique to digital phenotyping research (as, for instance, genomics research reveals information about the subjects’ parents and children, who may not have consented), and existing discourse from these related fields can be of use in developing policy for digital phenotyping researchers (Gordon and Koenig Citation2022). Effects on third parties should be incorporated into the risk/benefit calculus described in the next section.
FRAMEWORK FOR RETURNING IRRS IN DIGITAL PHENOTYPING RESEARCH
Recognizing the three features just described—many different data streams being collected, with potentially great uncertainty about validity/actionability/utility, and unanticipated impact on third parties—we propose a framework that is grounded in the core bioethics principles of balancing benefit/value vs. risk of returning the results/data, respect for persons (both participants and other third parties affected by return of the results), and promoting justice in returning data (The National Commission for the Protection of Human Subjects of and Biomedical and Behavioral Research Citation1979). Further, we adopt a practical ethics approach that allows for flexibility in application, emphasizing procedural justice, justification for the ethical choices made, and transparency in the decision-making process.
We recommend that decisions about the return of results be made on a study-by-study basis. A study-by-study approach is consistent with similar analyses (MRCT Center 2017; Botkin et al. Citation2018), and it requires documentation of choices made. In advance of study initiation, one first step is to identify whether the research will be subject to certain laws that can affect policies for returning results. Depending on the goals of the research and the types of data analysis to be performed, some studies may generate interpreted data/results or individual-specific findings that resemble diagnoses or predictions about the participants’ physical or mental health. Returning such IRRs to participants could potentially trigger a need to obtain an Investigational Device Exemption from the U.S. Food and Drug Administration (or equivalent requirements for research performed in other nations) (Congress House of Representatives Citation2020a). Another concern is that some research is governed by privacy laws of the European Union, the United States, and some U.S. states that require individuals, upon request, to be granted access to certain types of data and results (“Sec. 13.04 MN Statutes - Rights of Subjects of Data” Citation2022, Subd. 3; U.S. Department of Health & Human Services Citation2020; The European Parliament and the Council of the European Union Citation2016; Social Security Administration Citation1974; “Assembly Bill No. 375 - Chapter 55 - Privacy: Personal Information: Businesses” Citation2018). These mandated access rights are conceptually distinct from return of results—they exist so people can assess the level of privacy risk they face from personal data stored by others—but they sometimes seem in conflict with ethical policies that IRRs should not be returned. When such laws apply to a research project, researchers are required by law to grant access to certain types of data upon request, and researchers, IRBs, or others cannot block or override such access (The Multi-Regional Clinical Trials (MRCT) Center Citation2023). Thus, a first step in developing a protocol on IRRs is to contact the responsible personnel at one’s institution to assess which laws apply to the research. At most institutions, this would mean contacting the general counsel’s office, the chief privacy officer (for HIPAA and state privacy requirements), the human research protection program/IRB, and/or the regulatory affairs office (for FDA-related questions).
In the absence of legal or regulatory directives requiring individual access to data, returning IRRs appears to be consistent with participant expectations, especially when IRRs are clinically and/or personally actionable, and with emerging best practices and regulations (Botkin et al. Citation2018; Secretary’s Advisory Committee on Human Research Protections Citation2015). In collaboration with the IRB regarding local norms and context, research teams should discuss and decide whether, and how, each stream of individual data, as well as key analyses of those streams, will be returned (or not) to research participants, and then communicate, with justification, that decision to the IRB. The IRB-approved decisions on return of results should then be clearly communicated to participants as part of obtaining informed consent (Presidential Commission for the Study of Bioethical Issues Citation2013).
To facilitate development of study-by-study decisions about returning IRRs in psychiatric digital phenotyping research, we propose a 3 × 3 benefit-harm framework to contrast low, high, and unknown benefits of returning data versus low, high, and unknown risk of harm to participants ().
Figure 1. Framework for developing individual-specific return of results protocols in digital phenotyping research in psychiatry.
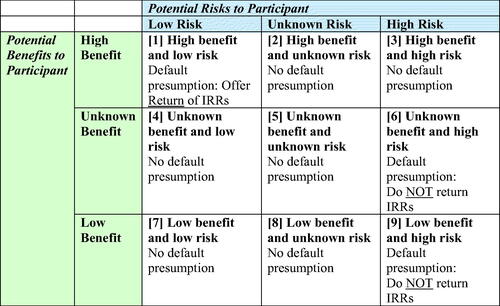
The balance between potential benefits and risks varies by the type of data stream being collected. While both benefits and risk are continuous variables, along a spectrum, our framework operationalizes the concept into a 3 × 3 matrix of “high,” “low,” and “unknown” potential benefit/risk. To place a data stream into a cell requires two initial steps: (1) assessing potential benefit as high, low, or unknown; and (2) assessing potential risk as high, low, or unknown. We use the term “benefit” to capture the many dimensions of “utility” and “value” captured in .
We use the term “unknown” to describe a wide array of situations in which there is insufficient information (e.g., on accuracy, reliability, validity, utility, safety, etc.) to adequately assess benefit or risk. We recommend presumptive default rules for each of the cells in this matrix. We also show how this framing can be used to consider issues such as equity, e.g., by determining if returning IRRs will systematically harm/benefit certain groups of participants more than others; resource capacity, e.g., if the research team has the requisite resources to implement any promised return; and parentalism, e.g., if the research team underestimates the capabilities of the participants in understanding and acting on certain results.
For research conducted at sites governed by privacy laws that grant individual rights of access to certain types of data (e.g., the HIPAA Privacy Rule and GDPR), it may not be possible to refuse to return IRRs, if the individual requests access. The HIPAA Privacy Rule provides a narrow exception allowing researchers to suspend individuals’ access to data and results from clinical research studies temporarily while the research is in progress (Office of Federal Register Citation2023, (a)(2)(iii)). To take advantage of this exception, researchers would need to obtain participants’ consent to the suspension of their access rights at the very outset of the study, as part of participants’ consent to the research. Moreover, access must be reinstated as soon as the research is completed, so this exception defers access only temporarily (Office of Federal Register Citation2023, (a)(2)(iii)). Unless these preparatory steps are taken at the start of the study, this exception does not apply, and individuals have a right to request access to their data and IRRs held in designated record sets at HIPAA-covered research sites.
When confronted with an individual access request, HIPAA-regulated researchers “may not impose unreasonable measures on an individual requesting access that serve as a barrier to or unreasonably delay the individual from obtaining access” (U.S. Department of Health & Human Services Citation2020). It would violate the law for researchers, IRBs, or counselors to try to discourage participants from exercising their lawful access rights. Researchers and IRBs can, however, help protect participants and address ethical concerns by preparing “point of data delivery (PODD)” disclosures, which are standard warnings and disclosures that will accompany data and IRRs at the time researchers respond to HIPAA access requests (Tayeh et al. Citation2022). These PODD disclosures could include, for example, disclosing any data-quality concerns and uncertainties about the analytical validity, clinical validity, and clinical utility of the data; warnings against misuse of the data—for example, a warning not to base medical decisions on the data without consulting their health care professional; disclosing that the information is being provided strictly to comply with privacy law and is not intended for medical decision-making; and warning recipients to protect their own privacy by making responsible decisions about any further sharing of the data they receive (for example, by checking privacy policies before contributing the data to a citizen science research project) (Tayeh et al. Citation2022).
Spurring Dialogue on Return of Results: Who, What, Where, When, How?
The framework encourages dialogue around the following: Who will receive results from the research team (e.g., how might the participant’s culture and race, ethnicity, age, sex, gender, culture, disability, and socioeconomic class affect decisions about returning results); What will be returned (e.g., raw data, processed data, interpreted data); Where the data is being sent (e.g., in what location and with whom will the participant be when receiving the results and what local/state/country regulations are implicated by that geographic location), When (and how frequently) the data will be returned (e.g., once at the end of the research project; at intervals such as daily, monthly, hourly; in real time); and How the data will be returned (e.g., on the participant’s smart phone app, in an electronic health record, verbally through a phone call or meeting with researchers or clinicians). Implied in the framework is the overarching question of “Whether” to return data at all, since applying the framework, and considering the who/what/where/when/how, may result in a decision not to return a data stream.
At the core of the framework lies a challenge that can only be resolved on a study-by-study, and data-by-data basis: determining the return-worthiness of the data. That is: by what metrics and criteria should “benefit” and “risk” be evaluated? We encourage approaches that include research participants in the evaluation of potential benefits and potential risks (Wilkins et al. Citation2019). Participants and researchers may vary in their preferences related to return of results (Lázaro-Muñoz, Torgerson, and Pereira Citation2021) and, whenever possible, participants should be given options for which data to receive, and even when to receive it (e.g., notify me when I’ve had over 3 h of screen time in a single day.)
Research teams will need to ensure that they have the resources to deliver on promised return of results policies. We envision that resource availability determinations would be independent of the initial risk/benefit calculus. That is, the research team should identify the IRRs they wish to return, estimate the resources required to return these IRRs, and build that into the study design and if applicable, request for funding. If researchers do not have adequate resources to conduct all of the return of IRRs they aspire to, the protocol should reflect current ability to return IRRs. Revision of the IRR protocol can expand scope if additional resources are secured. Resource availability may mitigate the risk or augment the benefit of returning a particular result (e.g., if return was done by a specialized provider, or came with access to particular health or personal resources), but this should be thought of as separate from the initial risk/benefit calculus of the IRR in question.
Research teams will also need to remain vigilant in revising initial risk/benefit calculations as the research progresses, and especially as research findings lead to improvements in validity and utility of results. Revision of risk/benefit analysis is also vital for dealing with unanticipated incidental findings (IFs). While anticipated IFs can be factored into the initial protocol, by definition the protocol cannot account for unanticipated IFs. We recommend that the return of IRRs approach be reviewed and revised at least annually.
Who? There is significant heterogeneity in participants both across and within digital phenotyping studies. Thus, we encourage an approach to return of results that is sensitive to the backgrounds, values, priorities, cultures, and lived experience of those participants. Factors relevant to return of results might include race, ethnicity, gender, culture, disability, and socioeconomic class. For instance, in the context of genetic counseling, significant concerns have been raised about ELSI issues in implementing genetic counseling services and returning genomic research results in diverse communities (Raymond et al. Citation2021; Young et al. Citation2021) and low-resource countries (Holzer et al. Citation2021; Zhong et al. Citation2021).
Research on genetic counseling and in the context of returning results in genomic studies has found a need for culturally-informed counseling (Burnett-Hartman et al. Citation2020; Young et al. Citation2021; Fehniger et al. Citation2013). At present there is no analogous field of “digital phenotyping counseling” and little guidance on which aspects of digital phenotyping results may be most culturally sensitive. But research in genomics return of results would imply that a one-size-fits-all approach to returning results in digital phenotyping research would overlook important variations in value/culture in the populations to whom results are being returned. In digital phenotyping research, researchers might need to consider whether certain data streams have the potential to generate stigma in particular cultures.
What? Deciding what to return involves related decisions about which types and combinations of data to return—raw data, processed data, and interpreted data. If research participants are simply given raw data, it may not be user-friendly and equity concerns may arise if processing that data requires specific expertise or an out-of-pocket expense to pay a third-party data analyst service. For example, in genomics research the “BAM file” (a specialized file format containing sequence info) could be returned as raw data to participants. However, this file type requires special knowledge to learn how to open, let alone analyze oneself. One may need graduate training in genetics or to hire someone with that training to access and interpret the data. Similar phenomena could be encountered depending on the format of the data returned in digital phenotyping. Of course, users may have the requisite abilities to manage such data and may desire it even if they don’t. For example, one review of the literature on this issue found that participants frequently want access to raw data such as BAM files, even though researchers worry about the usability of it (Wright et al. Citation2017). Researchers in digital phenotyping will need to balance concerns regarding how such data might be reasonably returned with considerations of benefits such as “autonomy, empowerment, health prevention, reciprocity, and improved trust” (Wright et al. Citation2017).
Interpreted and processed data, including data patterns and predictive data, might lead to recommendations to seek medical care. The granularity of the data, as well as the comparisons that are presented (e.g., compared to others in the study, compared to a normed sample), will likely affect both utility and risks. Of particular note is that a large number of data streams combined with powerful analytic methods means that researchers will have many ways of analyzing, presenting, and thus returning data and results. Our framework does not presume that data processing that is conducted by a human is prima-facie preferable from a risk/benefit perspective than that done by an AI/ML system if the result and IRR is otherwise the same. That is, in some cases data processed by AI/ML models may provide greater analytic or clinical validity than data that does not employ such methods. In other cases, use of AI/ML may be riskier or less beneficial because the models may be biased.
A prerequisite to successful digital phenotyping is the consistent and reliable collection of data in real time (Nebeker, Bartlett Ellis, and Torous Citation2020; Torous et al. Citation2019), and increasingly “the main intellectual challenge in smartphone-based digital phenotyping has arguably moved from data collection to data analysis” (Onnela Citation2021, 50). Advances in data analysis will provide an even more complex menu of options for returning IRRs. While the traditional distinctions between “raw data,” “processed data,” and “interpreted data” are still useful, researchers will be faced with decisions about which combinations of raw, processed, and interpreted data streams to return.
Participants may also be able to take their raw data to other vendors and have it processed and interpreted, as is possible with genetics data, which allows them to seek second opinions and have the data re-analyzed as technology improves. For example, a polygenic risk calculator may not have existed for a certain disease at the initial time of the participant’s sequencing, but if it emerges later a participant could run their raw data through the new pipeline.
When? Much existing guidance on returning IRRs conceptualizes the timing of return at either the end of a study, or perhaps at a few critical moments while the study is underway. By contrast, many of the data collected in digital phenotyping research can be more easily returned in real time, at researcher-specified intervals or at a moment triggered by an algorithm. For instance, participants could receive daily reports on their sleep data, hourly data on their step counts, or minute by minute data on heart rate and screen usage (Reeves, Robinson, and Ram Citation2020; Reeves et al. Citation2021). Thus, return of results in digital health research could be a continuous process and not a singular event. Thresholds, informed by participant preference, could also be incorporated. For instance, a user could choose to receive heart rate data only when it went below 50 or above 140 beats per minute.
When a threshold is reached and a result is returned immediately, there may not be time in that moment for contemplation or consultation. In such instance, the threshold value or calculation needs to be pre-defined, and effectively communicated to all involved, potentially including participants, those they live with (e.g., since in certain cases and certain alerts, law enforcement might be involved), outpatient clinical team members, study doctors, and the IRB or other institutional bodies.
It should also be noted that for those data that do require confirmatory testing or analysis, a lag between knowledge of a result and returning that result (i.e., only after sufficient further analysis is conducted) can produce anxiety in both participant and researcher. Just because more alerts can be sent, however, does not mean they should. “Alarm fatigue” is a challenge for clinicians (Sendelbach and Funk Citation2013), and participants who receive too many alerts may learn to ignore them.
As the possibility of real-time interventions and the ability to automate return of data increase, timing may be particularly challenging. For instance, if there is a small window for an IRR intervention (e.g., send the warning message now and not 2 h from now), with little time for extensive consultation, then thresholds for return and associated protocols need to be pre-defined and outlined in detail to all stakeholders, e.g., patient participants, outpatient clinical team members, study doctors, and the IRB or other institutional bodies.
Where and How? A unique aspect of return of IRRs in digital phenotyping research is that the smart phone or other device platform for data collection is also a potential platform for return of IRRs. Researchers might also send results to an electronic health record, send a secure email, or mail a hard copy letter. There are differing privacy concerns with each modality. Many health systems have made their electronic health records immediately accessible to patients via electronic gateways, so researchers could conceivably leverage that existing infrastructure to facilitate timely IRRs. Yet the return of data to smart phones must account for the possibility that the participant could conceivably be in any state or country, potentially implicating that jurisdiction’s law, concerns around remote research more generally (Gelinas et al. Citation2021), and data policies of the phone manufacturer and service carrier or internet service provider. In addition, the smart phone may not be in the possession of the participant, as when parents give their phone to a child to watch a video or when a group of friends are making a group video call. In such situations, even an alert (such as “New results from the hospital research study”) could be information that the participant does not want others to see. Researchers will thus need to consider privacy settings and methods of communicating that ensure the participant is the one receiving the information.
Utility and risk are also related to how the results are returned. For instance, will participants simply receive a text message alert with a link to a lengthy report, or will they receive a phone call from an expert who can explain the results (including, for instance, whether the results have clinical validity or not) and answer questions from the participant? Will any of the IRRs be returned automatically, with only a computer and AI/ML algorithm analyzing them? In which instances will a clinical consult be utilized? Visualization is also important and dovetails with the question of what is being returned. For instance, a smart phone alert that reads “IMPORTANT!” is likely to be understood differently than a lengthy email that conveys the same underlying information. The language used in returning results could also have an effect, positive or negative, on participant behavior.
Balancing Risks and Benefits
Dialogue concerning the Who, What, Where, When, How of returning results will help researchers determine into what risk/benefit cell a particular result fits. For example, when a result is returned will affect its benefits and risks. To illustrate, consider an alert that—if returned before a participant relapses into self-harm behavior—could potentially prevent the self-harm by helping the participant seek out support. The potential benefit of such an alert is very high, but only if returned in time to prevent the harmful event. To take another example, if results are returned in a language that is not well understood by the participant (or in a form that requires substantial additional analysis or interpretation), its benefit will be reduced—and risk might increase because the likelihood of misunderstanding rises.
These determinations should include assessment of risks and benefits to third parties, as discussed above. Also relevant to these determinations is the availability of the data stream to participants outside of the research protocol. For example, many apps allow users to generate daily sleep quality reports, so there may be low relative benefit from return of sleep quality data from the research team. We anticipate that where the greatest benefits will accrue is from the integration of multiple streams, typically not available via commercial apps, that generate additional insights (and perhaps hold greater risk) for participants.
The default presumption is to offer the return of data for data streams in the high benefit and low risk category (Cell 1). Of course, some type of benefit has to be sufficiently documented before cell 1 is relevant. An example would be returning step count data and exercise activity data. With regard to benefit, there is evidence from the consumer market that individuals often like to track this data. This suggests that participants would derive value from having these data, and with regard to risk, individuals learning of their step counts might be deemed low risk. Returning nighttime inactivity data (as a proxy for sleep) is already done in the consumer market and could be another example of high benefit and low risk; if, however, the study involved people with prominent anxiety about insomnia or bipolar disorder (where sleep patterns are clinically significant), then this same data might become high benefit and unknown risk. By contrast, for data streams in the low benefit and high risk category (Cell 9) the default presumption is not to return IRRs. An example would be providing the participant with a message that they should seek out a clinician because they may be at risk of developing a neurodegenerative disease based on an analytic method that has not been validated. Here, the benefit could be low because the data is more noise than signal. The risk could be high because the participant might experience stress and might even pursue medical treatment that would not be warranted. For the same reason, the presumption is not to return data in the unknown benefit and high risk category (Cell 6).
For the remaining six cells in the table, including all those with unknown risk, we do not offer a default presumption. Where the cell notes that there is no default presumption, the research team should weigh the benefits and risks and determine (for each data stream, as well as combinations of data streams) whether data will be returned. For example, the high benefit and high risk category (Cell 3) might include results (such as statistical markers) that suggest the likely onset of a psychotic break. The data could contribute to prevention of the psychotic break or earlier intervention for support or treatment. On the other hand, returning the data could lead to stigma and distress—including unnecessary distress if the prediction turns out incorrect—so it is also high risk. There is also no default presumption for data in the low benefit and low risk category (Cell 7). An example would be raw data such as the amount of data transferred on a cell phone in a month that has few privacy concerns, but also limited benefit. Finally, an example of an unknown benefit, unknown risk could be the detection of periods of pauses in respirations at night in a study with a wearable device when there is uncertainty about whether this is a technical artifact of the device or true apneic events that could signal the incidental finding of sleep apnea.
As these examples make clear, there are both opportunities and challenges of returning IRRs. For instance, returning participant data may be part of developing more “rigorous, inclusive, and representative” behavioral research in psychiatry (Germine et al. Citation2021). A further plausible, yet unknown, benefit or risk of returning IRRs is that the participant’s behavior and/or mental state may change. For instance, if researchers are collecting sleep data, perhaps returning daily sleep report data will allow participants to develop better sleep habits. Such outcomes are unknown at present.
Additional benefits may include improved data transparency if returning data—especially data that are collected passively—leads to a better understanding of what data are being collected and how they are being used, and better retention in the study (if participants feel more engaged). To the extent that returning data is part of a broader effort to further participatory research in science, it can also be a recruitment tool as “participation in research is incentivized by the desire to contribute to science, insight into the research question being studied, as well as return of study data and individual research results” (Germine et al. Citation2021, 5). Relatedly, an additional benefit of returning data may be enhanced trust between the research team and participants. Trust is particularly important because digital phenotyping relies on technological intermediaries that may be suspect due to privacy concerns (Brown Citation2020). Returning data might also increase comprehension of the study since research participants will have a better understanding of what data, including sensitive data, have been collected.
A drawback of returning IRRs is the potential to produce negative effects on behavior and mental states. As discussed above, there is a lack of knowledge about when processed data have been sufficiently validated to justify return, and how returning results will affect participant behavior. One related concern is the lack of “digital phenotyping counselors,” in contrast to genetic counselors who are well established, with dedicated journals, graduate programs, and a professional society. Genetic counselors play a vital role in the return of genetics results. But there are simply no parallel counselors to help participants understand their digital phenotyping results. Privacy concerns also emerge with regard to how the data is shared and with whom. As with return of results in other biomedical research, there may also be confusion of research participation with clinical care, uncertainty about whether digital phenotyping results are entered into the medical record, and participant apprehension, as returned results may raise more questions and distress than they resolve.
How to navigate these many challenges will necessarily require consideration of practical constraints, such as the research team’s resources and the logistics of returning results. Our recommendations for digital phenotyping research in psychiatry are generally consistent with previous guidance on return of results, including the recommendation that whenever participants are offered the opportunity to receive data, it should be the participants’ choice as to whether to do so (The Multi-Regional Clinical Trials (MRCT) Center Citation2023, Citation2017; Botkin et al. Citation2018).
Applying this framework will be context-specific and many important issues deserve further attention. These include: informed consent; legal issues such as who controls access to data, and the applicability of HIPAA and CLIA for various data streams; ethical issues with AI digital phenotyping research, such as whether AI results are biased and thus inaccurate for some population subgroups, and/or whether findings from AI systems are transparent and understandable to researchers, care providers, and the people whose data were processed; post-study obligations to individual participants and to the community; appropriate data storage and sharing in ways that protect privacy; ethical obligations, if any, to re-analyze data periodically; policies on participants’ ability to opt-out of receiving IRRs, opt-out from secondary uses for their data, or revoke consent after a study has begun and have their data removed from the study; return of aggregate group results; incidental findings in the context of these new types of data streams; the potential sharing of results with others beyond the participant (such as clinicians and law enforcement); sharing of data with other researchers; returning results in international research contexts; and obligations to submit programs to regulators, such as the U.S. Food and Drug Administration (FDA), for review prior to commercialization.
Nor did our analysis explore in depth the issue of the conditions under which reporting of results to a third party or to the participants themselves may be mandated or prohibited by law, ethics, or institutional policy. For instance, if a research team is collecting screenomics data (Reeves et al. Citation2021) and a participant’s screen shows engagement with a child pornography website, the researchers may be under obligation to report.
Partnerships with private firms and third parties for data collection and analysis further complicate the analysis. For instance, rather than generate their own datasets a research team may be granted access to digital phenotyping data already collected from a company’s users. Such arrangements would create a large lag between the moment of data collection and the moment of data analysis, with implications for real-time versus retrospective return of results.
Updating Return of Results Policies as Research Emerges
An important goal for digital phenotyping research is to study return of results as an intervention. A promise of digital phenotyping research is that it can positively change the trajectory of behavior, from encouraging someone to get more exercise to reducing self-harm and antisocial behavior. For example, multiple research teams are attempting to use in-app interventions to improve college student mental health (Melcher, Hays, and Torous Citation2020), and to prevent relapse in schizophrenia (Rodriguez-Villa et al. Citation2021). In such studies, in order to assess the effect of returning results, study design may require that different groups of participants receive results at different times, in different ways, or not at all. An ethical framework should distinguish between return of results offered during the study as a study intervention and return of results that are not part of a study intervention.
The approach we propose allows for significant flexibility for researchers, clinicians, IRB members, sponsors, and participants in using these new research findings to update whether, what, and how data should be returned in digital health research in psychiatry. More specific guidance and applied decision-making tools for specific types of digital phenotyping research will require further research.
At present, however, the analytical validity, clinical validity, actionability, clinical utility, and personal utility for many digital data streams are unknown, and there is minimal empirical evidence to know whether and how return of IRRs (or the withholding of IRRs) will affect the behavior and mental states of those who receive the information. Digital phenotyping research designs presently include many exploratory and hypothesis-generating studies, and the data streams are expanding (e.g., incorporating data from smart shoe sensors, car sensors, and in-home appliance sensors). Return of results guidance must thus be flexible to account for the continuous expansion of data and data sources.
For some research data streams—such as MRI brain scans (Shoemaker et al. Citation2016)—there is existing guidance related to returning IRRs. But even these more established guidelines may require revision as collected data may not be reviewed by humans in a way that makes it compatible with mandated reporting guidance or timely return of results. Some digital phenotyping data may be reviewed soon after they are collected, but other data may be reviewed months or years later. Participants should be informed about the anticipated types of timing of data analysis during the consent process.
We clarify that it is beyond our scope in this article to provide detailed analysis of the return of results to third parties, for instance which results will be returned for medical, clinical, or legal reasons. Future work can consider whether and when researchers should share or offer to share data with a third party designated by the participant (e.g., spouse, therapist, psychiatrist), or an unaffiliated third party (e.g., emergency services or employers). Also beyond the scope of this article is a more extensive legal analysis of pertinent regulations, federal and state laws, and institutional policies related to mandatory reporting, duty to warn, and the like. But we note that researchers should be aware of participants’ federal right of access to the contents of their “designated record set” (DRS) held by any HIPAA-covered entity (Wolf and Evans 2018). In addition, participants should be informed of mandated reporting that might be implicated by the research study. For instance, in 11 states, faculty and staff at higher education institutions are required to report instances of child abuse and neglect under certain conditions, and healthcare professionals are mandatory reporters of child abuse in 47 states (Congress House of Representatives Citation2020b, (b)(2)(B)(i)).
Applying Framework to Illustrative Hypothetical Case
We opened this article with an illustrative hypothetical to motivate our analysis, and we return to that hypothetical case here to apply our proposed framework. The hypothetical research team aims to understand the factors that increase or decrease the likelihood of symptoms of severe mental illness in youth, paving the way for more effective interventions. Our framework makes clear that it is of paramount importance that the research team initially develop its return of IRRs approach before the research begins. The approach should then be revisited, and if needed revised, as the research progresses.
In initial development of the return of IRRs plan, our framework suggests that the research team should think carefully about who their participants will be, and to avoid parentalism by not underestimating the capabilities of those participants in understanding and acting on certain results. This could be accomplished by talking with potential participants and patient advocates during the research design phase. This is consistent with our recommendation that researchers include participants in the evaluation of potential benefits and potential risks. The team should also assess its resources to implement any promised return of IRRs and discuss with current and potential funders.
While a more detailed vignette would be required to formulate a specific plan, we can consider the outline of what such a plan should entail. Who: the participants in this study are potentially vulnerable and marginalized youth—young people with mental illness. If the youth are minors, parents and legal guardians will also need to be consulted. It is well recognized that access to mental health services is scarce, and thus the research team should factor that in its return protocol, for instance clarifying that these are research not clinical results and sharing resources for further care along with results. What: the data being collected is broad and deep, allowing for sensitive inferences about participants use of illicit drugs, sexual activity, liquor purchases, driving while drunk, visiting pornography websites, and having suicidal ideation. Returning data streams that could allow for such inferences falls into the High Risk category, and this data should only be returned if there is high benefit to participants. More details would be needed to determine high benefit but conceivably a return of IRRs that mitigated the risk of drug use relapse, severe psychiatric decompensation (e.g., hyper-sexuality in a severe manic episode), or self-harm behavior could qualify. While the high-risk data should only be returned where the benefit is high, other data streams may be low-risk. For example, daily measures of sleep may be of low or unknown risk and may provide benefit if it helps the participant improve sleep quality or seek closer follow-up with established providers (e.g., if the person already knows that insomnia is one of their early indicators of a possible manic or hypomanic episode). It is worth emphasizing, as we do above, that the researchers here will be faced with decisions about which combinations of raw, processed, and interpreted data streams to return.
When: The hypothetical assumes that the digital phenotyping technology being used in this research project has adequate resources to facilitate return of individualized results to each participant, on a monthly, weekly, daily, or even more frequent interval. Intervals for return of IRRs should be aligned with estimated benefit and risk. A sleep quality report, for instance, might be returned daily. An example of real-time feedback might be positive reinforcement related to activity data, e.g., an alert sent immediately after the wearable device detects exercise. Real-time return of IRRs may also be warranted in the high-benefit/high-risk situations described above, e.g., to avoid relapse or self-harm.
Where and How: Although the data is being largely collected by technology and could be returned with messages in apps and on devices, the research team should think carefully about where it will need humans to return IRRs. In this research, where participants are youth with mental illness, the research team should consider engaging professionals with expertise in working with youth to facilitate return of IRRs. If the research study is geographically dispersed, then these conversations with participants may need to occur via video or telephone, rather than in-person.
Providing clarity on where to start with an individual study: Even without more details other than the vignette description, our framework demonstrates its utility in outlining core aspects of a return of IRRs plan for an individual digital phenotyping study. With these core aspects in place, the return of IRRs plan can be further developed as the details of the research design are considered and refined.
CONCLUSION
This article provides a framework to address a critical unmet need: guidance for returning results in rapidly expanding research in psychiatry using digital phenotyping tools.
The framework can serve as a practical foundation on which to explore the many unanswered questions surrounding returning IRRs in digital phenotyping research in psychiatry. Future dialogue and research should include a diverse and expanded group of stakeholders; indeed, stakeholder input into the question of returning results is of heightened importance when research is being conducted in vulnerable and marginalized populations. Relatedly, returning IRRs requires time and resources, and research teams must be able to deliver on what they promise participants with respect to returning data. Empirical research, especially research with those most likely to be affected by return of IRR policies, is needed on the question of what participants want in terms of return of results and how returning particular types of results will affect individual and group behavior.
In the absence of such empirical data and given the likelihood of significant variation in analytical validity, clinical validity, actionability, and personal utility across emerging digital phenotyping tools, we recommend that research teams balance the interests discussed in this article. There will be instances in which the return of IRRs poses little risk and could generate significant personal benefit for participants. In such cases, participants should be given the option to receive IRRs. Where the benefits and risks are unknown, the judgment is more difficult. But as digital phenotyping tools improve, we anticipate more research in which return of IRRs could provide benefits to participants. Thus, it is imperative that all digital phenotyping research teams begin preparing now to address the Who, What, Where, When, and How of returning IRRs in their research programs.
JTB has received consulting fees and equity options from Mindstrong Health, Inc., and consulting fees from Healios Limited, Inc. MLB receives royalties from Oxford University Press from the sale of his monograph, “The Neuroethics of Biomarkers”. CAB is a Board Member of Dream Impact Trust. LTG is President and Director of a nonprofit organization (The Many Brains Project) that supports return of research results for cognitive testing. MM is an Advisory Board Member, Psychedelic Medicine Coalition; Member, Psychedelic Bar Association; and Board of Advisors, Plant Medicine Healing Alliance. JPO is a co-founder of a recently established commercial entity that will operate in digital phenotyping. SLR is employed by Mass General Brigham/McLean Hospital; paid as secretary of Society of Biological Psychiatry, and for Board service to Mindpath Health/Community Psychiatry and National Association of Behavioral Healthcare; served as volunteer member of the Board for Anxiety & Depression Association of America, and The National Network of Depression Centers; received royalties from Oxford University Press, American Psychiatric Publishing Inc, and Springer Publishing; received research funding from NIMH. CS serves as a community member for an Advarra IRB. IV is a consultant for Otsuka; contracts with Mary Ann Morse Senior Living; and receives royalties from Oxford University Press.
Box 1 Examples of data streams for digital phenotyping research in psychiatry.
Note. The data streams listed in Box 1 are illustrative, not exhaustive. The category headings illustrate some of the primary mechanisms by which data may be collected, but these headings are not mutually exclusive, e.g., we list GPS under smart phones, but GPS can also be collected from wearables and implantables.
Additional information
Funding
REFERENCES
- ACMG Board of Directors. 2012. Points to consider in the clinical application of genomic sequencing. Genetics in Medicine 14 (8):759–61. doi:10.1038/gim.2012.74.
- ACMG Board of Directors. 2015. Clinical utility of genetic and genomic services: A position statement of the American College of Medical Genetics and Genomics. Genetics in Medicine: Official Journal of the American College of Medical Genetics 17 (6):505–7. doi:10.1038/gim.2015.41.
- “Assembly Bill No. 375 - Chapter 55 - Privacy: Personal Information: Businesses.” 2018. California Legislative Information. Accessed June 28, 2018. https://leginfo.legislature.ca.gov/faces/billTextClient.xhtml?bill_id=201720180AB375.
- Baker, J. T. 2019. The digital future of psychiatry. Psychiatric Annals 49 (5):193–4. doi:10.3928/00485713-20190416-02.
- Baker, J. T., L.T. Germine, K. J. Ressler, S. L. Rauch, and W. A. Carlezon. 2018. Digital devices and continuous telemetry: Opportunities for aligning psychiatry and neuroscience. Neuropsychopharmacology 43 (13):2499–503. doi:10.1038/s41386-018-0172-z.
- Blom, J. M. C., C. Colliva, C. Benatti, F. Tascedda, and L. Pani. 2021. Digital phenotyping and dynamic monitoring of adolescents treated for cancer to guide intervention: Embracing a new era. Frontiers in Oncology 11:673581. doi:10.3389/fonc.2021.673581.
- Botkin, J. R., Michelle Mancher, Emily R. Busta, and Autumn S. Downey, eds. 2018. Returning individual research results to participants: Guidance for a new research paradigm. Washington, DC: National Academies Press. doi:10.17226/25094.
- Brown, E. 2020. A healthy mistrust: Curbing biometric data misuse in the workplace. Stanford Technology Law Review 23 (2):252–98.
- Buolamwini, J., and T. Gebru. 2018. Gender shades: Intersectional accuracy disparities in commercial gender classification. In Proceedings of the 1st Conference on Fairness, Accountability and Transparency, 77–91. New York: PMLR. https://proceedings.mlr.press/v81/buolamwini18a.html.
- Burke, W., B. J. Evans, and G. P. Jarvik. 2014. Return of results: Ethical and legal distintions between research and clinical care. American Journal of Medical Genetics. Part C, Seminars in Medical Genetics 166 (1):105–11. doi:10.1002/ajmg.c.31393.
- Burnett-Hartman, A. N., E. Blum-Barnett, N. M. Carroll, S. D. Madrid, C. Jonas, K. Janes, M. Alvarado, R. Bedoy, V. Paolino, N. Aziz, et al. 2020. Return of research-related genetic test results and genetic discrimination concerns: Facilitators and barriers of genetic research participation in diverse groups. Public Health Genomics 23 (1–2):59–68. doi:10.1159/000507056.
- Camacho, E., R. O. Brady, P. Lizano, M. Keshavan, and J. Torous. 2021. Advancing translational research through the interface of digital phenotyping and neuroimaging: A narrative review. Biomarkers in Neuropsychiatry 4 (June):100032. doi:10.1016/j.bionps.2021.100032.
- Center for Disease Control. 2010. “ACCE model process for evaluating genetic tests.” https://www.cdc.gov/genomics/gtesting/acce/index.htm.
- Clayton, E. W., and A. L. McGuire. 2012. The legal risks of returning results of genomics research. Genetics in Medicine 14 (4):473–7. doi:10.1038/gim.2012.10.
- Congress House of Representatives. 2020a. “21 U.S.C. 812 - schedules of controlled substances.” Government. Govinfo.Gov. U.S. Government Publishing Office. Accessed December 31, 2020. https://www.govinfo.gov/app/details/USCODE-2020-title21/https%3A%2F%2Fwww.govinfo.gov%2Fapp%2Fdetails%2FUSCODE-2020-title21%2FUSCODE-2020-title21-chap13-subchapI-partB-sec812.
- Congress House of Representatives. 2020b. “42 U.S.C. 5106a - grants to states for child abuse or neglect prevention and treatment programs.” Government. Govinfo.Gov. U.S. Government Publishing Office. Accessed December 31, 2020. https://www.govinfo.gov/app/details/USCODE-2020-title42/https%3A%2F%2Fwww.govinfo.gov%2Fapp%2Fdetails%2FUSCODE-2020-title42%2FUSCODE-2020-title42-chap67-subchapI-sec5106a.
- Davidson, B. I. 2022. The crossroads of digital phenotyping. General Hospital Psychiatry 74 (January):126–32. doi:10.1016/j.genhosppsych.2020.11.009.
- Essén, A. 2008. The two facets of electronic care surveillance: An exploration of the views of older people who live with monitoring devices. Social Science & Medicine (1982) 67 (1):128–36. doi:10.1016/j.socscimed.2008.03.005.
- Facio, F. M., H. Eidem, T. Fisher, S. Brooks, A. Linn, K. A. Kaphingst, L. G. Biesecker, and B. B. Biesecker. 2013. Intentions to receive individual results from whole-genome sequencing among participants in the ClinSeq study. European Journal of Human Genetics 21 (3):261–5. doi:10.1038/ejhg.2012.179.
- Faucett, W. A., and F. D. Davis. 2016. How geisinger made the case for an institutional duty to return genomic results to biobank participants. Applied & Translational Genomics 8 (February):33–5. doi:10.1016/j.atg.2016.01.003.
- Fehniger, J., F. Lin, M. S. Beattie, G. Joseph, and C. Kaplan. 2013. Family communication of BRCA1/2 results and family uptake of BRCA1/2 testing in a diverse population of BRCA1/2 Carriers. Journal of Genetic Counseling 22 (5):603–12. doi:10.1007/s10897-013-9592-4.
- Food and Drug Administration Center for Devices and Radiological Health. 2017. “Software as a Medical Device (SAMD): Clinical Evaluation – Guidance for Industry and Food and Drug Administration Staff.” FDA. Washington, DC. https://www.fda.gov/files/medical%20devices/published/Software-as-a-Medical-Device-%28SAMD%29--Clinical-Evaluation---Guidance-for-Industry-and-Food-and-Drug-Administration-Staff.pdf.
- Food and Drug Administration Center for Devices & Radiological Health. 2021. “Artificial Intelligence/Machine Learning (AI/ML)-Based Software as a Medical Device (SaMD) Action Plan.” Washington, DC: FDA. https://www.fda.gov/media/145022/download.
- Freeman, D., S. Reeve, A. Robinson, A. Ehlers, D. Clark, B. Spanlang, and M. Slater. 2017. Virtual reality in the assessment, understanding, and treatment of mental health disorders. Psychological Medicine 47 (14):2393–400. doi:10.1017/S003329171700040X.
- Gelinas, L., W. Morrell, S. A. White, and B. E. Bierer. 2021. Navigating the ethics of remote research data collection. Clinical Trials (London, England) 18 (5):606–14. doi:10.1177/17407745211027245.
- Germine, L., R. W. Strong, S. Singh, and M. J. Sliwinski. 2021. Toward dynamic phenotypes and the scalable measurement of human behavior. Neuropsychopharmacology 46 (1):209–16. doi:10.1038/s41386-020-0757-1.
- Gianfrancesco, M. A., S. Tamang, J. Yazdany, and G. Schmajuk. 2018. Potential biases in machine learning algorithms using electronic health record data. JAMA Internal Medicine 178 (11):1544–7. doi:10.1001/jamainternmed.2018.3763.
- Goodman, S. N., S. Goel, and M. R. Cullen. 2018. Machine learning, health disparities, and causal reasoning. Annals of Internal Medicine 169 (12):883–4. doi:10.7326/M18-3297.
- Gordon, D. R., and B. A. Koenig. 2022. ‘If relatives inherited the gene, they should inherit the data.’ Bringing the family into the room where bioethics happens. New Genetics and Society 41 (1):23–46. doi:10.1080/14636778.2021.2007065.
- Gratzer, D., J. Torous, R. W. Lam, S. B. Patten, S. Kutcher, S. Chan, D. Vigo, K. Pajer, and L. N. Yatham. 2021. Our digital moment: Innovations and opportunities in digital mental health care. The Canadian Journal of Psychiatry 66 (1):5–8. doi:10.1177/0706743720937833.
- Green, R. C., J. S. Berg, W. W. Grody, S. S. Kalia, B. R. Korf, C. L. Martin, A. L. McGuire, R. L. Nussbaum, J. M. O’Daniel, K. E. Ormond, et al. 2013. ACMG recommendations for reporting of incidental findings in clinical exome and genome sequencing. Genetics in Medicine 15 (7):565–74. doi:10.1038/gim.2013.73.
- Haque, A., A. Milstein, and L. Fei-Fei. 2020. Illuminating the dark spaces of healthcare with ambient intelligence. Nature 585 (7824):193–202. doi:10.1038/s41586-020-2669-y.
- Holm, I. A., S. K. Savage, R. C. Green, E. Juengst, A. McGuire, S. Kornetsky, S. J. Brewster, S. Joffe, and P. Taylor. 2014. Guidelines for return of research results from pediatric genomic studies: Deliberations of the Boston Children’s Hospital gene partnership informed cohort oversight board. Genetics in Medicine 16 (7):547–52. doi:10.1038/gim.2013.190.
- Holzer, K., K. A. Culhane-Pera, R. J. Straka, Y. F. Wen, M. Lo, K. Lee, T. Xiong, K. Peng, J. Bishop, B. Thyagarajan, et al. 2021. Hmong participants’ reactions to return of individual and community pharmacogenetic research results: ‘A positive light for our community’. Journal of Community Genetics 12 (1):53–65. doi:10.1007/s12687-020-00475-3.
- van Hoof, J., H. S. Kort, P. G. Rutten, and M. S. Duijnstee. 2011. Ageing-in-place with the use of ambient intelligence technology: Perspectives of older users. International Journal of Medical Informatics 80 (5):310–31. doi:10.1016/j.ijmedinf.2011.02.010.
- Hsin, H.,. M. Fromer, B. Peterson, C. Walter, M. Fleck, A. Campbell, P. Varghese, and R. Califf. 2018. Transforming psychiatry into data-driven medicine with digital measurement tools. NPJ Digital Medicine 1 (1):1–4. doi:10.1038/s41746-018-0046-0.
- Huckvale, K., S. Venkatesh, and H. Christensen. 2019. Toward clinical digital phenotyping: A timely opportunity to consider purpose, quality, and safety. NPJ Digital Medicine 2 (1):88. doi:10.1038/s41746-019-0166-1.
- Insel, T. R. 2017. Digital phenotyping: Technology for a new science of behavior. JAMA 318 (13):1215–6. doi:10.1001/jama.2017.11295.
- Jain, S. H., B. W. Powers, J. B. Hawkins, and J. S. Brownstein. 2015. The digital phenotype. Nature Biotechnology 33 (5):462–3. doi:10.1038/nbt.3223.
- Jarvik, G. P., L. M. Amendola, J. S. Berg, K. Brothers, E. W. Clayton, W. Chung, B. J. Evans, J. P. Evans, S. M. Fullerton, C. J. Gallego, et al. 2014. Return of genomic results to research participants: The floor, the ceiling, and the choices in between. American Journal of Human Genetics 94 (6):818–26. doi:10.1016/j.ajhg.2014.04.009.
- Jayakumar, P., E. Lin, V. Galea, A. J. Mathew, N. Panda, I. Vetter, and A. B. Haynes. 2020. Digital phenotyping and patient-generated health data for outcome measurement in surgical care: A scoping review. Journal of Personalized Medicine 10 (4):282. doi:10.3390/jpm10040282.
- Khodyakov, D., A. Mendoza-Graf, S. Berry, C. Nebeker, and E. Bromley. 2019. Return of value in the new era of biomedical research—one size will not fit all. The American Journal of Bioethics Empirical Bioethics 10 (4):265–75. doi:10.1080/23294515.2019.1666175.
- Knoppers, B. M., Y. Joly, J. Simard, and F. Durocher. 2006. The emergence of an ethical duty to disclose genetic research results: International perspectives. European Journal of Human Genetics: EJHG 14 (11):1170–8. doi:10.1038/sj.ejhg.5201690.
- Latalova, K., D. Kamaradova, and J. Prasko. 2014. Perspectives on perceived stigma and self-stigma in adult male patients with depression. Neuropsychiatric Disease and Treatment 10:1399–405. doi:10.2147/NDT.S54081.
- Lázaro-Muñoz, G., L. Torgerson, and S. Pereira. 2021. Return of results in a global survey of psychiatric genetics researchers: Practices, attitudes, and knowledge. Genetics in Medicine 23 (2):298–305. doi:10.1038/s41436-020-00986-x.
- Lévesque, E., Y. Joly, and J. Simard. 2011. Return of research results: General principles and international perspectives. The Journal of Law, Medicine & Ethics: A Journal of the American Society of Law, Medicine & Ethics 39 (4):583–92. doi:10.1111/j.1748-720X.2011.00625.x.
- Liang, Y., X. Zheng, and D. D. Zeng. 2019. A survey on big data-driven digital phenotyping of mental health. Information Fusion 52 (December):290–307. doi:10.1016/j.inffus.2019.04.001.
- Marsch, L. A. 2018. Opportunities and needs in digital phenotyping. Neuropsychopharmacology 43 (8):1637–8. doi:10.1038/s41386-018-0051-7.
- Martinez-Martin, N., H. T. Greely, and M. K. Cho. 2021. Ethical development of digital phenotyping tools for mental health applications: Delphi study. JMIR mHealth and uHealth 9 (7):e27343. doi:10.2196/27343.
- Martinez-Martin, N., T. R. Insel, P. Dagum, H. T. Greely, and M. K. Cho. 2018. Data mining for health: Staking out the ethical territory of digital phenotyping. NPJ Digital Medicine 1 (1):68. doi:10.1038/s41746-018-0075-8.
- Melcher, J., R. Hays, and J. Torous. 2020. Digital phenotyping for mental health of college students: A clinical review. Evidence-Based Mental Health 23 (4):161–6. doi:10.1136/ebmental-2020-300180.
- Miller, D. T., K. Lee, A. S. Gordon, L. M. Amendola, K. Adelman, S. J. Bale, W. K. Chung, M. H. Gollob, S. M. Harrison, G. E. Herman, et al. 2021. Recommendations for reporting of secondary findings in clinical exome and genome sequencing, 2021 update: A Policy Statement of the American College of Medical Genetics and Genomics (ACMG). Genetics in Medicine 23 (8):1391–8. doi:10.1038/s41436-021-01171-4.
- Miller, M. L., I. M. Raugh, G. P. Strauss, and P. D. Harvey. 2022. Remote digital phenotyping in serious mental illness: Focus on negative symptoms, mood symptoms, and self-awareness. Biomarkers in Neuropsychiatry 6 (June):100047. doi:10.1016/j.bionps.2022.100047.
- Miner, A. S., A. Haque, J. A. Fries, S. L. Fleming, D. E. Wilfley, G. Terence Wilson, A. Milstein, D. Jurafsky, B. A. Arnow, W. Stewart Agras, et al. 2020. Assessing the accuracy of automatic speech recognition for psychotherapy. NPJ Digital Medicine 3 (1):1–8. doi:10.1038/s41746-020-0285-8.
- Mittelstadt, B. 2017. Ethics of the health-related internet of things: A narrative review. Ethics and Information Technology 19 (3):157–75. doi:10.1007/s10676-017-9426-4.
- Mohr, D., K. Shilton, and M. Hotopf. 2020. Digital phenotyping, behavioral sensing, or personal sensing: names and transparency in the digital age. NPJ Digital Medicine 3 (1): 45. doi:10.1038/s41746-020-0251-5.
- Mohr, D. C., M. Zhang, and S. M. Schueller. 2017. Personal sensing: Understanding mental health using ubiquitous sensors and machine learning. Annual Review of Clinical Psychology 13 (1):23–47. doi:10.1146/annurev-clinpsy-032816-044949.
- Mulvenna, M. D., R. Bond, J. Delaney, F. M. Dawoodbhoy, J. Boger, C. Potts, and R. Turkington. 2021. Ethical issues in democratizing digital phenotypes and machine learning in the next generation of digital health technologies. Philosophy & Technology 34 (4):1945–60. doi:10.1007/s13347-021-00445-8.
- Nasir, K., and R. Khera. 2020. Digital phenotyping of myocardial dysfunction with 12-lead ECG: Tiptoeing into the future with machine learning. Journal of the American College of Cardiology 76 (8):942–4. doi:10.1016/j.jacc.2020.07.001.
- Nebeker, C., R. J. Bartlett Ellis, and J. Torous. 2020. Development of a decision-making checklist tool to support technology selection in digital health research. Translational Behavioral Medicine 10 (4):1004–15. doi:10.1093/tbm/ibz074.
- Obermeyer, Z., B. Powers, C. Vogeli, and S. Mullainathan. 2019. Dissecting racial bias in an algorithm used to manage the health of populations. Science (New York, N.Y.) 366 (6464):447–53. doi:10.1126/science.aax2342.
- Office of Federal Register 2023. “45 CFR § 164.524 - Access of Individuals to Protected Health Information.” LII/Legal Information Institute. Accessed January 9, 2023. https://www.law.cornell.edu/cfr/text/45/164.524.
- Onnela, J.-P. 2021. Opportunities and challenges in the collection and analysis of digital phenotyping data. Neuropsychopharmacology: Official Publication of the American College of Neuropsychopharmacology 46 (1):45–54. doi:10.1038/s41386-020-0771-3.
- Onnela, J.-P., and S. L. Rauch. 2016. Harnessing smartphone-based digital phenotyping to enhance behavioral and mental health. Neuropsychopharmacology: Official Publication of the American College of Neuropsychopharmacology 41 (7):1691–6. doi:10.1038/npp.2016.7.
- Parcesepe, A. M., and L. J. Cabassa. 2013. Public stigma of mental illness in the United States: A systematic literature review. Administration and Policy in Mental Health and Mental Health Services Research 40 (5):384–99. doi:10.1007/s10488-012-0430-z.
- Presidential Commission for the Study of Bioethical Issues. 2013. “Anticipate and Communicate: Ethical Management of Incidental and Secondary Findings in the Clinical, Research, and Direct-to-Consumer Contexts.” https://bioethicsarchive.georgetown.edu/pcsbi/sites/default/files/FINALAnticipateCommunicate_PCSBI_0.pdf.
- Ravitsky, V., and B. S. Wilfond. 2006. Disclosing individual genetic results to research participants. The American Journal of Bioethics 6 (6):8–17. doi:10.1080/15265160600934772.
- Raymond, M. B., K. E. Cooper, L. S. Parker, and V. L. Bonham. 2021. Practices and attitudes toward returning genomic research results to low-resource research participants. Public Health Genomics 24 (5–6):241–52. doi:10.1159/000516782.
- Reeves, B., N. Ram, T. N. Robinson, J. J. Cummings, C. L. Giles, J. Pan, A. Chiatti, M. J. Cho, K. Roehrick, X. Yang, et al. 2021. Screenomics: A framework to capture and analyze personal life experiences and the ways that technology shapes them. Human-Computer Interaction 36 (2):150–201. doi:10.1080/07370024.2019.1578652.
- Reeves, B., T. Robinson, and N. Ram. 2020. Time for the human screenome project. Nature 577 (7790):314–7. doi:10.1038/d41586-020-00032-5.
- Ressler, K. J., and L. M. Williams. 2021. Big data in psychiatry: Multiomics, neuroimaging, computational modeling, and digital phenotyping. Neuropsychopharmacology 46 (1):1–2. doi:10.1038/s41386-020-00862-x.
- Richards, S., N. Aziz, S. Bale, D. Bick, S. Das, J. Gastier-Foster, W. W. Grody, M. Hegde, E. Lyon, E. Spector, et al. 2015. Standards and guidelines for the interpretation of sequence variants: A joint consensus recommendation of the American College of Medical Genetics and Genomics and the Association for Molecular Pathology. Genetics in Medicine 17 (5):405–24. doi:10.1038/gim.2015.30.
- Richards, C. Sue, S. Bale, D. B. Bellissimo, S. Das, W. W. Grody, M. R. Hegde, E. Lyon, and B. E. Ward. 2008. ACMG recommendations for standards for interpretation and reporting of sequence variations: Revisions 2007. Genetics in Medicine 10 (4):294–300. doi:10.1097/GIM.0b013e31816b5cae.
- Rodriguez-Villa, E., U. M. Mehta, J. Naslund, D. Tugnawat, S. Gupta, J. Thirtalli, A. Bhan, V. Patel, P. K. Chand, A. Rozatkar, et al. 2021. Smartphone Health Assessment for Relapse Prevention (SHARP): A digital solution toward global mental health. BJPsych Open 7 (1):e29. doi:10.1192/bjo.2020.142.
- “Sec. 13.04 MN Statutes - Rights of Subjects of Data.” 2022. Office of the Revisor of Statutes. https://www.revisor.mn.gov/statutes/cite/13.04.
- Secretary’s Advisory Committee on Human Research Protections. 2015. “SACHRP Recommendations.” HHS.Gov. 2015. https://www.hhs.gov/ohrp/sachrp-committee/recommendations/sachrp-recommendations/index.html.
- Sendelbach, S., and M. Funk. 2013. Alarm fatigue: A patient safety concern. AACN Advanced Critical Care 24 (4):378–86. quiz 387–88. doi:10.1097/NCI.0b013e3182a903f9.
- Sharac, J., P. McCrone, S. Clement, and G. Thornicroft. 2010. The economic impact of mental health stigma and discrimination: A systematic review. Epidemiologia e Psichiatria Sociale 19 (3):223–32. doi:10.1017/S1121189X00001159.
- Shen, F. X., B. C. Silverman, P. Monette, S. Kimble, S. L. Rauch, and J. T. Baker. 2022. An ethics checklist for digital health research in psychiatry: Viewpoint. Journal of Medical Internet Research 24 (2):e31146. doi:10.2196/31146.
- Shoemaker, J. M., Cole, C. Petree L. E., D. L. Helitzer, M. T. Holdsworth, John P. Gluck, and John P. Phillips. 2016. Evolution of universal review and disclosure of MRI reports to research participants. Brain and Behavior 6 (3):e00428. doi:10.1002/brb3.428.
- Sickel, A. E., J. D. Seacat, and N. A. Nabors. 2014. Mental health stigma update: A review of consequences. Advances in Mental Health 12 (3):202–15. doi:10.1080/18374905.2014.11081898.
- Smith, D. G. 2018. Digital phenotyping approaches and mobile devices enhance CNS biopharmaceutical research and development. Neuropsychopharmacology 43 (13):2504–5. doi:10.1038/s41386-018-0222-6.
- Social Security Administration 1974. “Privacy Act Of 1974, P.L. 93-579, (88 Stat. 1896), 5 U.S.C. 552a.” Compilation Of The Social Security Laws. Accessed December 31, 1974. https://www.ssa.gov/OP_Home/comp2/F093-579.html.
- Tayeh, M. K., M. Chen, S. M. Fullerton, P. R. Gonzales, S. J. Huang, L. J. Massingham, J. M. O’Daniel, D. R. Stewart, A. R. Stiles, and B. J. Evans. 2022. The designated record set for clinical genetic and genomic testing: A points to consider statement of the American College of Medical Genetics and Genomics (ACMG). Genetics in Medicine. Online ahead of print. doi:10.1016/j.gim.2022.11.010.
- The European Parliament and the Council of the European Union. 2016. “Regulation (EU) 2016/679 of the European Parliament and of the Council of 27 April 2016 on the Protection of Natural Persons with Regard to the Processing of Personal Data and on the Free Movement of Such Data, and Repealing Directive 95/46/EC (General Data Protection Regulation).” Website. Publications Office of the European Union. Accessed April 27, 2016. http://op.europa.eu/en/publication-detail/-/publication/3e485e15-11bd-11e6-ba9a-01aa75ed71a1.
- The Multi-Regional Clinical Trials (MRCT) Center. 2017. “Return of Individual Results Recommendations Document.” https://mrctcenter.org/wp-content/uploads/2017/12/2017-12-07-Return-of-Individual-Resullts-Recommendations-Document-V-1.2.pdf.
- The Multi-Regional Clinical Trials (MRCT) Center. 2023. “Return of Individual Researh Results.” 2023. https://mrctcenter.org/return-of-individual-results/.
- The National Commission for the Protection of Human Subjects of and Biomedical and Behavioral Research. 1979. The Belmont Report: Ethical Principles and Guidelines for the Protection of Human Subjects of Research. Bethesda, MD: Office for Human Research Protections. https://www.hhs.gov/ohrp/regulations-and-policy/belmont-report/read-the-belmont-report/index.html.
- Torous, J., M. V. Kiang, J. Lorme, and J.-P. Onnela. 2016. New tools for new research in psychiatry: A scalable and customizable platform to empower data driven smartphone research. JMIR Mental Health 3 (2):e16. doi:10.2196/mental.5165.
- Torous, J., H. Wisniewski, B. Bird, E. Carpenter, G. David, E. Elejalde, D. Fulford, S. Guimond, R. Hays, P. Henson, et al. 2019. Creating a digital health smartphone app and digital phenotyping platform for mental health and diverse healthcare needs: An interdisciplinary and collaborative approach. Journal of Technology in Behavioral Science 4 (2):73–85. doi:10.1007/s41347-019-00095-w.
- Torrado, J. C., Bettina S. Husebo, H. G. Allore, A. Erdal, Stein E. Fæø, H. Reithe, E. Førsund, Charalampos Tzoulis, and Monica Patrascu. 2022. Digital phenotyping by wearable-driven artificial intelligence in older adults and people with Parkinson’s disease: Protocol of the mixed method, cyclic activeageing study. PloS One 17 (10):e0275747. doi:10.1371/journal.pone.0275747.
- U.S. Department of Health & Human Services. 2020. “Individuals’ Right under HIPAA to Access Their Health Information 45 CFR § 164.524.” Text. HHS.Gov. January 2020. https://www.hhs.gov/hipaa/for-professionals/privacy/guidance/access/index.html.
- Vogel, D. L., R. L. Bitman, J. H. Hammer, and N. G. Wade. 2013. Is stigma internalized? The longitudinal impact of public stigma on self-stigma. Journal of Counseling Psychology 60 (2):311–6. doi:10.1037/a0031889.
- Vokinger, K. N., S. Feuerriegel, and A. S. Kesselheim. 2021. Mitigating bias in machine learning for medicine. Communications Medicine 1 (1):25. doi:10.1038/s43856-021-00028-w.
- Wilkins, C. H., B. M. Mapes, R. N. Jerome, V. Villalta-Gil, J. M. Pulley, and P. A. Harris. 2019. Understanding what information is valued by research participants, and why. Health Affairs 38 (3): 399–407. doi:10.1377/hlthaff.2018.05046.
- Wolf, S. M. 2013. Return of individual research results and incidental findings: Facing the challenges of translational science. Annual Review of Genomics and Human Genetics 14 (1):557–77. doi:10.1146/annurev-genom-091212-153506.
- Wolf, S. M., and B. J. Evans. 2018. Defending the return of results and data. Science 362 (6420):1255–1256. doi:10.1126/science.aaw1851.
- Wolf, S. M., F. P. Lawrenz, C. A. Nelson, J. P. Kahn, M. K. Cho, E. W. Clayton, J. G. Fletcher, M. K. Georgieff, D. Hammerschmidt, K. Hudson, et al. 2008. Managing incidental findings in human subjects research: Analysis and recommendations. Journal of Law, Medicine & Ethics 36 (2):219–48. doi:10.1111/j.1748-720X.2008.00266.x.
- Wong, C. A., A. F. Hernandez, and R. M. Califf. 2018. Return of research results to study participants: Uncharted and untested. JAMA 320 (5):435–6. doi:10.1001/jama.2018.7898.
- Wright, C. F., A. Middleton, J. C. Barrett, H. V. Firth, D. R. FitzPatrick, M. E. Hurles, and M. Parker. 2017. Returning genome sequences to research participants: Policy and practice. Wellcome Open Research 2 (February):15. doi:10.12688/wellcomeopenres.10942.1.
- Yanos, P. T., A. Lucksted, A. L. Drapalski, D. Roe, and P. Lysaker. 2015. Interventions targeting mental health self-stigma: A review and comparison. Psychiatric Rehabilitation Journal 38 (2):171–8. doi:10.1037/prj0000100.
- Young, J. L., J. Mak, T. Stanley, M. Bass, M. K. Cho, and H. K. Tabor. 2021. Genetic counseling and testing for Asian Americans: A systematic review. Genetics in Medicine 23 (8):1424–37. doi:10.1038/s41436-021-01169-y.
- Yu, J., J. Crouch, S. M. Jamal, H. K. Tabor, and M. J. Bamshad. 2013. Attitudes of African Americans toward return of results from exome and whole genome sequencing. American Journal of Medical Genetics. Part A 161A (5):1064–72. doi:10.1002/ajmg.a.35914.
- Zhong, A., B. Darren, B. Loiseau, L. Q. B. He, T. Chang, J. Hill, and H. Dimaras. 2021. Ethical, social, and cultural issues related to clinical genetic testing and counseling in low- and middle-income countries: A systematic review. Genetics in Medicine 23 (12):2270–80. doi:10.1038/s41436-018-0090-9.