In this reply to our commentators, we respond to ethical concerns raised about the potential use (or misuse) of personalized LLMs for academic idea and prose generation, including questions about career pressures, conformity, (lack of) meaning, bias, homogeneity of output, and lowered standards. We also highlight further potential benefits raised by a minority of commentators, including benefits relating to equity and diversity. We advocate a co-creation model for guiding human use of LLMs—whether personalized or standard—to produce and share new ideas or content. Thus, in concert with the impressive generative capacities of LLMs, humans must play a role in creating, crafting, curating, and communicating any resulting material, remaining responsible for such vital aspects as meaning-making, imbuing of intention, showing creativity in prompt design and elaboration of initial outputs, careful editing, vetting, fact-checking, and other necessary contributions.
OVERVIEW
We are grateful for the thoughtful responses to our recent target article, “AUTOGEN: A personalized large language model for academic enhancement—Ethics and proof of principle” (Porsdam Mann et al. Citation2023a). In that article, we explored the potential of personalized large language models (LLMs) fine-tuned on one or more individuals’ previously published writings for academic idea and prose generation. As a proof of principle, we generated four such fine-tuned models, which we dubbed AUTOGENs (AI Unique Tailored Output GENerators), and informally evaluated their performance in drafting introductions to hypothetical or existing research papers based on a title and abstract. Finding that our AUTOGEN models outperformed the base GPT-3 model, we surveyed a range of ethical risks and potential benefits associated with the use of personalized LLMs for academic idea and prose generation.
The Open Peer Commentaries (OPCs) presented thoughtful critiques of our ideas and also highlighted potential opportunities associated with the use of personalized LLMs in an academic context.Footnote1 Our reply is organized around three main categories: Concerns, Suggestions, and Opportunities. In the first of these—Concerns—we respond to about half of the OPCs. These papers add depth to some of our concerns, or describe new ones, regarding the introduction of AUTOGENs to bioethical, and wider, scholarship. In the second and third categories, we reflect primarily on the contributions by Nyholm (Citation2023b), Varma (Citation2023), Zohny (Citation2023), and McMillan (Citation2023), each of which, in addition to bringing new ideas, explores the positive potential of these models in greater depth than was possible in our original paper. We conclude by providing an update on our recent and ongoing work with AUTOGENs.
CONCERNS
One concern noted by several commentators was the potentially negative effects of widespread AUTOGEN use on academic publishing.
Laacke and Gauckler (Citation2023) argue that the use of personalized LLMs in bioethics risks decreasing quality, perpetuating biases, enforcing conformity pressures, exacerbating inequalities, reducing diversity, and compromising rather than enhancing academic work and ethical analysis. They question whether increased productivity translates to ethical progress and innovation, warn of potential rebound effects like increased publication pressure, and state that LLMs currently lack capabilities essential for critical, reflective, and discursive ethical practice. Overall, Laacke and Gauckler express skepticism about the benefits of AUTOGENs and similar LLMs in bioethics, instead emphasizing their potential to reproduce flaws, undermine integrity, and frustrate the essential functions of ethical inquiry.
Like Laacke and Gauckler, Ostertag (Citation2023) cautions against the use of AUTOGEN-style LLMs in bioethics. He argues that LLM-generated texts inherently lack meaning due to the absence of communicative intent. This is problematic, according to Ostertag, because reading published writings necessarily involves a degree of trust in its accuracy, given the limitations on the reader’s time and the presumed competence of editors and authors. This trust may lead readers to falsely treat LLM-generated texts as meaningful, coherent, and produced in good faith, which is problematic due to the known occurrence of fabrications and falsehoods in generated text.
Resnik and Hosseini (Citation2023) similarly worry that AUTOGENs may negatively impact the integrity of academic publishing by making it easier to “to write about ill-conceived ideas or repackage … old ideas without improving the extant literature” (50). They argue that AUTOGENs’ relative difficulties “in making creative and insightful connections between concepts” may worsen the already concerning trend toward conservatism in academic publishing by encouraging mediocrity in research. Besides issues related to novelty and creativity, Resnik and Hosseini point to AUTOGENs’ propensity toward factual mistakes and their presumed tendency to replicate the biases and weaknesses contained in their training material (e.g., in our previously published papers). These may be particularly difficult to identify because they deal with ethical frameworks, ideological and cultural assumptions, and values that may be difficult to define and identify. Like other contributors, Resnik and Hosseini share the concerns articulated in our original paper as to AUTOGENs’ likely effects in increasing publication pressure and the Matthew effect, according to which those who already enjoy the benefits of scholarly productivity are likely to see the greatest benefit from AUTOGEN use (because they have published more and therefore have more material with which to fine-tune their models).
Erler’s (Citation2023) contribution touches on many of the same issues. He notes the potential of AUTOGENs to increase publication pressures; worries about the implications for peer review processes that are already problematically overloaded, and for scholars’ ability to keep up with the literature; and extends our argument about potential homogenization of writing styles by pointing to the risk of increasingly homogenous thinking styles. While more of a worry for generic than personalized LLMs, Erler argues that increasing reliance on generative AI models for inspiration may lead to “a self-reinforcing feedback loop” in which LLMs increasingly shape the topics, and perhaps also the responses, chosen by academics.
We agree with many of the concerns raised and are thankful to the authors for adding depth to several issues we touched on in the original paper and for adding new ones. However, some worries appear to us inapt or exaggerated, or insufficiently tempered by the possibility of deliberately introducing measures to mitigate some of the risks identified.
For example, Laacke and Gauckler question whether LLMs “will be able to adequately paraphrase or interpret argumentative texts for the purpose of overview and summarization” (62). In our experience, these are among the tasks at which LLMs excel. Research on summarization tasks has found similar or greater performance for LLMs as compared to humans in both specialist and general domains (Van Veen et al. Citation2023; Zhang et al. Citation2023). To illustrate, we asked Anthropic’s Claude 2 to summarize and provide an overview of Laacke and Gauckler’s commentary. The result is the summary paragraph of their OPC provided earlier in this section (note that all other text was written manually). While not perfect, it seems to us to be a relatively comprehensive and fair overview.
Here we should also clarify a related point raised by Laacke and Gauckler. They state that we, in our original article, claim “that given the ‘analytic strength’ and ‘originality’ [of LLMs], the use of LLMs is not merely permissible but an attractive and morally desirable example of human-technological co-creation” (61). However, the full quote for our statement conveys our intended meaning better than the partial quote supplied by Laacke and Gauckler. We wrote: “In brief, when human beings evaluate and transform the results of LLMs, particularly building on their analytic strength or originality, the use of LLMs is not merely permissible but an attractive and morally desirable example of human-technological co-creation” (Porsdam Mann et al. Citation2023a, 37) Thus, our point was not that LLMs as such display impressive analytic strength or originality, but rather that when humans build or improve on these factors, the result can be a desirable example of co-creation.
Laacke and Gauckler also note that “one’s writing reflects not only an individual writing style and intellectual strengths, but also one’s intellectual weaknesses, including prejudice, blind spots, and fallacies” (62). Therefore, AUTOGENs may reproduce these weaknesses and biases. This is a worry shared, and also raised by, Resnik and Hosseini (Citation2023). The insight appears to us as likely correct. However, this would appear also to be a problem for manual writing. Indeed, one promising use of LLMs in academia is tasking them with identifying such biases and weaknesses (Ganguli et al. Citation2023). This solution, for what it’s worth, also applies equally to generated or manually produced text.
Finally, Laacke and Gauckler claim that AUTOGENs and LLMs generally lack the ability to “integrate the perspectives of those people who are or could be affected [by] the moral issues in question, may it be via empirical representation, surrogacy or empathy,” (62) presumably because empathy implicitly requires consciousness. While it is true that LLMs are not—on most leading theories of consciousness—conscious and thus cannot subjectively experience emotions such as empathy, recent work demonstrates that LLMs can be better than humans at producing text which integrates differing viewpoints into consensus statements (Bakker et al. Citation2022), and can produce text which is judged to be as or more empathic than human-generated text (Sorin et al. Citation2023). With respect to surrogacy, in other work, we have explored the idea of augmenting surrogate decision-making (Earp et al. Citation2024) or medical consent-taking (Allen et al. Citation2024) with fine-tuned LLMs—but always with humans “in the loop” (Zohny et al. Citation2024). We are not sure what Laacke and Gauckler mean by “empirical representation” in this context, but if it is demographic, descriptive, or other empirical data on the perspectives in question, we see no reason why LLMs should not be able to integrate such information.
Ostertag (Citation2023) argues that generated text cannot convey meaning due to a lack of communicative intent. As Ostertag himself acknowledges, there are examples of generated texts which incontrovertibly do carry meaning. Ostertag’s example is an automated email message acknowledging receipt of another email. To this may be added many others, such as automated traffic and weather reports, track-and-trace alerts, programs that generate diagnostic reports based on medical data, financial report software, and so on. In Ostertag’s view, these examples carry meaning because they can be directly tied to the psychological state of a language speaker with communicative intent. As “nothing analogous happens in LLMs,” the “resulting text cannot be said to be meaningful in the way that humanly-produced inscriptions and utterances are meaningful” (92).
This argument seems to us unconvincing for three reasons. First, Ostertag’s argument relies on the assumption that meaningful language necessarily involves a particular kind of explanation referring to intentional states and linguistic knowledge. This is problematic. It is true that the intention of a writer is relevant to the meaning of a text (Grice Citation1969). Yet that text may also have a separate, literal meaning (Grice Citation1968), or a meaning derived from context (Pavlick Citation2023). Young children can make meaningful statements without being conscious of intending to convey that meaning in any explicit psychological sense. More generally, meaning can derive from functions, as in the case of medical diagnostic software or a legal contract (Kar and Radin Citation2019). Moreover, in some contexts meaning can change over time while the original communicative intent remains static. In international law, for example, the original intention of the drafters of and signatories to a treaty is only one relevant factor in the interpretation of that agreement. Among the other factors are context, the ordinary (as opposed to intended) meaning of terms, general legal principles such as proportionality, as well as subsequent practice in relation to the agreement (i.e., its functional effects) (United Nations Citation1969 Articles 31–32).
Second, LLMs are trained on huge corpora of human statements, many of which were produced by linguistically competent agents with communicative intent. Thus, they do encapsulate a form of linguistic knowledge, albeit not in a conscious way. LLMs are not random word generators. Their stochastic range is limited by the patterns of text in their training data (Piantadosi and Hill Citation2022). Indeed, the fundamental function of LLMs is precisely to identify these patterns and produce new text that also fits them. What distinguishes current models from older ones is an increased ability to do just that.
Third, and most importantly, Ostertag’s critique leaves out a crucial component: the role of the human user of an LLM. As we stated in our original paper, our envisioned use of AUTOGENs is as part of a larger process of co-creation. Any generated text should be subject to rigorous vetting, including fact-checking and editing (Earp et al. under review). Even if one were to accept Ostertag’s argument based on the lack of communicative intent in relation to AUTOGEN-generated text, this would no longer hold for co-created text in which one or more humans has manipulated and interrogated the raw outputs. An editor critically examines generated text and makes necessary changes, if any, to ensure that the text meets the standards and requirements for its intended purpose. Even if the text undergoes no changes through this editing process, the fact that it has been reviewed and affirmed signifies that the editor has assumed responsibility for the text in question, transforming it from draft to final form. Using Ostertag’s terminology, we could say that the vetting process infuses the text with the editor’s own communicative intent. Irrespective of whether one accepts Ostertag’s argument, such a process of vetting imbues meaning. So long as generated text is rigorously vetted and improved in a process of co-creation, users may continue to place epistemic trust in the text they read.
This point is also relevant to several of the other critiques. As we noted in our original paper, and as emphasized by several of the OPCs (Laacke and Gauckler, Ostertag, Resnik and Hosseini), LLMs including AUTOGENs are prone to hallucination and factual inaccuracies. Relatedly, the higher performance of AUTOGENs on areas close to those discussed in its training data runs the risk of encouraging repackaging of old ideas, thus diluting the literature. Combined with AUTOGENs’ current difficulties in generating novel and creative ideas, these authors worry that the overall effect of both AUTOGEN use and general (non-personalized) LLM use will lead to declining standards and quality of work rather than facilitating productivity or progress.
While both general and personalized LLMs do indeed produce output that often contains factual errors, this need not translate into lower standards or quality of work. As in the case of communicative intent, the editing stage can and should be used to rectify errors and omissions. Importantly, Resnik and Hosseini themselves write that “[t]he problems we have pointed out in this commentary have mostly to do with widespread and indiscriminate use of AUTOGEN” (51). They note that some uses of AUTOGEN, “such as summarizing the opinions of an expert panel or focus group or writing encyclopedia entries or lecture notes” may “not threaten the integrity of scholarly writing” (51). While we agree with Resnik and Hosseini on this point, we believe that it is not the type of writing so much as the adherence or otherwise to quality standards that determines the impact of LLM use on the integrity of scholarly writing. Careful and judicious use of AUTOGENs, followed by rigorous vetting and iterative improvement in a process of co-creation, could, regardless of the type of writing used, be appropriate and ethically unproblematic so long as normal quality standards are adhered to. As Erler states in his contribution, “even novel and valuable content can conceivably be produced via a judicious mix of contributions from both humans and AI. A more constructive approach might simply lie in upholding proper standards of quality in research: that is, standards researchers can only meet by substantially contributing to the end result, and not by re-using a LLM’s response to prompts more or less as is” (Erler Citation2023, 94).
However, other worries related to increasing competitive pressure appear to us valid and important. It is indeed likely that any increase in productivity enabled by AUTOGENs will translate into higher expectations and a further increase in publish-or-perish dynamics. The extent to which this is problematic will depend in part on whether AUTOGEN-enabled productivity gains translate into bioethical and wider academic progress. If so, any effects on competition will need to be balanced against the wider societal interest in increasing the fruits of academic inquiry (Porsdam Mann et al. Citation2018). If not, any increased competitive pressure is inherently troublesome. In any case, whether benefits outweigh costs and risks will depend on implementation and oversight. If, as Erler wisely suggests, journal editors, funders, and employers accept responsibility for upholding standards of quality over quantity, the impact of competitive pressures introduced by productivity-enhancing tools such as AUTOGENs should not compromise the quality of published work. This is, however, a separate worry from their impacts on individual expectations. The only way to properly address this issue, which predates LLM use and is a much larger problem, is to radically change funding and evaluation standards. However, here too implementation is important. What one does with time freed up through increased productivity is a matter of personal choice, even if constrained by expectations. As we noted in our original contribution, any increases in productivity could be used to produce the same output in less time, thus potentially enabling a move to a four-day work week. Nothing dictates that additional time must be spent on more work.
SUGGESTIONS
In the title of our original paper, as well as in a footnote, we suggested that the literature on the ethics of enhancement might contain useful lessons for the ethics of AUTOGEN use. In his OPC, as well as in a recent article (Nyholm Citation2023a), Nyholm (Citation2023b) probes this suggestion in depth. Abstracting away from issues concerning hallucinations and accuracy, Nyholm suggests that a fully competent AUTOGEN might be more akin to an “independent academic agent of its own, whose output should rather be treated like the outputs of a digital twin coauthor—like a form of academic clone—rather than as something the researcher themselves can take credit for and claim to be the enhanced output of their own current efforts” (45). To substantiate this claim, Nyholm offers two thought experiments. In the first, he notes that in real cases where scholars produce writings in the style of a specific academic (Nyholm uses Kripke as an example) during that academic’s lifetime, this does not intuitively count as an enhancement of that scholar’s work. In the second, Nyholm asks us to consider a scholar who (secretly) relies entirely on an AUTOGEN to produce work which, due to advanced impairments, they can no longer carry out themselves. Would such a model still count as an enhancement of the impaired author’s work when used by others, for example after the original author’s death? If not, suggests Nyholm, then it is difficult to see why its use by a living author should count as such.
In his OPC, McMillan (Citation2023) notes a similar case, drawing on Argentinian author Jorge Luis Borges. In one of his essays, Borges reflected on the distinction between himself as an abstract entity—i.e., the author of Borges’s works—and himself as a concrete, living individual person. Indeed, writes McMillan, Borges the author has ‘outlived’ Borges the person and might continue to do so for hundreds of years. Against this backdrop, McMillan, like Nyholm, asks what we should make of hypothetical future works created by an LLM in the style of Borges the author. Unlike Nyholm, McMillan suggests that if “Borges the person had trained an LLM and was happy for it to continue writing on his behalf once he had passed, perhaps the causal connection would be strong enough to consider those works his” (44).
These commentaries point to an important aspect of LLM use in general, namely their impact on credit and authorship standards (Porsdam Mann et al. Citation2023b). These are deep questions whose philosophical implications will have to be worked out in due course. One relevant aspect we have not yet considered is the extent to which an AUTOGEN’s response is determined by the fine-tuning process as opposed to its original, general training. Where the fine-tuning dataset has a dominant influence, AUTOGEN outputs may more closely mimic the individual’s writing and argumentation style. On the other hand, in cases where the general training plays a more significant role than the fine-tuning data (for example, because an individual has not written enough articles to establish a large database) the AUTOGEN’s responses may be less personalized and more reflective of the broader patterns, including biases, present in the general training data. It thus appears that personalization is a matter of degree. In the case of the AUTOGEN models reported on here and in our original paper, the degree appeared to us significant. Nevertheless, this is an important area for further normative and experimental work.
There are urgent practical and pragmatic policy questions with respect to authorship and credit standards which need to be addressed before the more metaphysical questions can be entirely sorted out. From a policy perspective, it seems to us that in cases of impairment as mentioned by Nyholm—and by Varma (Citation2023), on which more below—fairness and inclusion would motivate allowing individuals to claim credit for the products of their AUTOGENs, even if the models carry out the lion’s share of the work.
Whether unimpaired individuals should likewise receive full credit for, and be considered authors of, texts produced by their digital twins depends, we suggest, on the extent to which they have contributed to the end product in a process of co-creation. There are interesting parallels here to group-based methods of text production. Nyholm (Citation2023b) asks us to consider the following case: “a researcher produces new material by letting scholars of their past work generate new ideas and texts for them, and then edits this output slightly and puts their name on it. Surely, this would not really count as an academic enhancement of the researcher. It would at best be coauthored work, where the other scholars deserve the main credit” (45). This parallel is interesting as, to a significant extent, it describes actual work practices in much of the biomedical sciences. A principal investigator or lab leader will have students and younger colleagues produce work which is often based on their previous efforts. This work is then edited by the principal investigator, who attaches their name, typically as senior author, to the manuscript. Similar work practices exist in the world of art: many Renaissance masters employed whole studios or warehouses of assistants (Tietze Citation1939), a practice carried on by some contemporary artists (Liedtke Citation2004).
What these dynamics mean for attribution of authorship and credit is not straightforward. While in the case of the biomedical principal investigator, the result is indeed a coauthored work, in the world of art it is the senior artist who takes all the credit. Either approach seems possible. As a matter of policy, it seems to us that there is a significant difference between work produced by an AUTOGEN-style personalized model trained on one’s past work versus a similar model trained on another person’s work. While it would be problematic for, say, the present authors to claim authorship for a piece of work produced using a Nyholm-based AUTOGEN, it appears less problematic for Nyholm himself to do so. An important disanalogy between the parallel case and the AUTOGEN case is that there are fewer considerations of fairness: AUTOGENs are not conscious and are not the type of entity that benefits from credit allocation. While there are legitimate concerns regarding whether an AUTOGEN user deserves full credit, there are no equivalent concerns regarding the fairness of allocating, or failing to allocate, credit to an AUTOGEN (as there is in relation to the artists and biomedical scientists in the parallel case).
As we describe in more detail below, it seems to us that there are strong policy considerations in favor of recognizing work produced by AUTOGENs in the case of impairments. For unimpaired academics, our position is that credit should be allocated and authorship acknowledged in proportion to the quality and degree of co-creation involved.
OPPORTUNITIES
In our original paper, we noted the potential for AUTOGEN-style LLMs to be used by academics who may have difficulties expressing themselves following health- or age-related declines in physical or mental function. Varma (Citation2023) picks up on this idea, adding depth and nuance based in part on personal experience. Varma’s commentary also notes the potential for AUTOGENs to enable entry into academia for individuals who would otherwise face insurmountable barriers for similar reasons. Importantly, Varma notes that these benefits may not be available to all individuals facing impairments in their ability to produce academic writing due to the variety of such impairments, some of which may prevent them from accessing and using LLMs. Furthermore, Varma notes that the utility of LLMs to help individuals express themselves is in large part reliant on these individuals’ preexisting skills, some of which may be impaired by an overreliance on LLMs.
We thank Varma for adding such insight to this important aspect of AUTOGEN-style personalized LLMs. We agree that further research should be carried out to find ways of making these models more accessible to individuals with impairments. We are also grateful for the point, not noted in our original paper, that LLMs may help individuals overcome what might otherwise have been prohibitive barriers to entry into academia (as opposed to allowing existing academics to work despite impairments). While Varma raises this point in relation to general LLMs, there is an interesting synergy here with Zohny’s (Citation2023) suggestion that allowing personalization on senior academics’ work could help address worries related to Matthew effects (i.e., that the benefits of AUTOGEN may be greater for those who have already published many articles). An individual who, due to impairments, has not written many articles cannot train a high-functioning AUTOGEN. However, it would seem to us relatively unproblematic for such an individual to train a personalized LLM based, for example, on a senior colleague’s or advisor’s publications, with permission, with the expectation that the training data would periodically be updated as the individual produces writings of their own.
Zohny’s (Citation2023) OPC is remarkable for turning several of our worries on their head. Contrary to our suggestion that AUTOGEN-style personalized LLMs may worsen Matthew effects, Zohny points to the potential leveling effects of LLMs fine-tuned by junior scholars on the work of more senior colleagues. Against the obvious concerns this raises in relation to intellectual property, Zohny notes that writing styles are not themselves protected and that the use of others’ articles may be permissible under fair and transformative use exceptions. With respect to our concerns about homogenization, Zohny points out that homogenizing effects may in fact be preferable, given the often-opaque writing of individual academics and the generally clear and lucid style of LLMs.
In our view, perhaps the most important insight made by Zohny is the potential for personalized consumption, rather than production, of academic works, a powerful idea not explored in our original paper. Whether generic or fine-tuned for this purpose, the use of LLMs to present ideas and text in interactive ways easily modifiable by user preferences has the potential to greatly facilitate understanding and to revolutionize interfaces to knowledge more generally. Using LLMs such as Claude 2 or GPT-4, it is possible to upload papers and to interact with them, asking the model to explain the major concepts in any way one desires. For example, we asked Claude 2 to provide a one-sentence summary of Zohny’s paper understandable by a five-year-old: “Zohny says using big kids’ writing to train a writing robot could help little kids write better, but adults need to talk about getting the good things like clearer writing without the bad things like everyone writing the same way.” Using GPT-4’s advanced data analysis tool, we generated visual outlines of Zohny’s paper (), flash cards with its major points, and even a PowerPoint presentation (Appendix). We also gave Claude 2 and GPT-4 information about ourselves and our learning styles and asked the models to tailor their summaries of Zohny’s points to these preferences. This is a fascinating novel application of our personalization idea with potentially enormous beneficial consequences for individual learning and research.
Figure 1. Visual representation of Zohny (Citation2023) generated by GPT-4’s advanced data analysis tool.
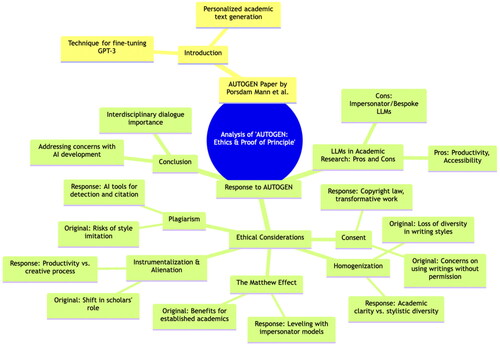
ADDITIONAL WORK ON AUTOGEN
Following the recent introduction of fine-tuning for GPT-3.5, we updated our AUTOGEN models and are currently carrying out follow-up experiments using these new models. Since GPT-3.5 is a version of GPT-3 which is itself fine-tuned to follow instructions in a chat format, we did not expect—and have so far not observed—significant differences in performance. However, the AUTOGEN-3.5 models appear to outperform the AUTOGEN-3 models in two respects: one, the newer models more consistently produce text in an appropriate format and with somewhat greater coherence. In other words, there appears to be less trailing off of performance with increased length of text (a problem we noted with respect to the original AUTOGEN models). Second, the 3.5 models are better able to deal with prompts outside our original format (detailed in the original paper). We remain excited to update our AUTOGEN models on GPT-4, as well as to try fine-tuning on the same data using other LLMs.
In a second line of work, we are investigating public moral attitudes toward the use of personalized versus generic LLMs to produce beneficial versus harmful content (Earp et al. under review). Preliminary results, based on studies of four populations (US, UK, China, and Singapore), suggest that people are willing to assign more credit for positive outcomes (e.g., a blogpost full of useful information) co-created by a human and a personalized LLM like AUTOGEN than for identical outcomes co-created by a human and a generic LLM like ChatGPT. Many participants took note of the fact that, for personalized LLMs compared to generic LLMs, the output reflected the author’s past skill, creativity, or effort, and this contributed to a sense that they deserved more credit for the current output as well. For harmful outcomes (e.g., a blog post full of disinformation), participants assigned high levels of blame to the human user regardless of LLM-type, explaining that the human user had a responsibility to edit and vet the blog post before publishing it, regardless of the source of material. This coincides with our theoretical analysis above.
CONCLUSION
We are grateful, again, to all of the OPC authors, both critical and sympathetic, for taking our proposal seriously and helping move the debate forward in a productive way. We believe that many of the concerns can be addressed through the concept of co-creation, where the human actively engages with the input and output of personalized LLMs. Of course, this is still just the start of a conversation that will, in the coming months and years, require the full participation of a wide range of stakeholders as the technological possibilities in this space continue to grow exponentially. We asked one of our AUTOGEN models to write the final sentence, and we endorse its conclusion: “Rather than trying to stop or restrict further development, we believe that the academic community should engage in co-creation to ensure that the technology is used responsibly and for the greatest possible benefit.”
Zohnys_Paper_Response_Presentation.pptx
Download MS Power Point (33.8 KB)ACKNOWLEDGMENTS
The authors thank Spencer Bentley for his help with fine-tuning the AUTOGEN-3.5 series.
DISCLOSURE STATEMENT
JS is a Partner Investigator on an Australian Research Council grant LP190100841 which involves industry partnership from Illumina. He does not personally receive any funds from Illumina. JS is a Bioethics Committee consultant for Bayer.
Additional information
Funding
Notes
1 In what follows, we use the term “personalized LLMs” to refer to LLMs that have been adapted to text produced by or describing a specific individual or group of individuals. We use “AUTOGEN” to refer specifically to the type of personalized LLM discussed in our paper, that is, a GPT-3 (or higher) model trained on the published academic output of an individual.
REFERENCES
- Allen, J. W., B. D. Earp, J. Koplin, and D. Wilkinson. 2024. Consent-GPT: Is it ethical to delegate procedural consent to conversational AI? Journal of Medical Ethics 50:77–83. doi:10.1136/jme-2023-109347.
- Bakker, M. A., M. J. Chadwick, H. R. Sheahan, M. H. Tessler, L. Campbell-Gillingham, J. Balaguer, N. McAleese, A. Glaese, J. Aslanides, M. M. Botvinick, et al. 2022. Fine-tuning language models to find agreement among humans with diverse preferences. arXiv. doi:10.48550/arXiv.2211.15006.
- Earp, B. D., S. Porsdam Mann, M. A. Khan, Y. Chu, J. Savulescu, P. Liu, and I. Hannikainen. Under review. Personalizing AI reduces credit-blame asymmetries across cultures.
- Earp, B. D., S. Porsdam Mann, J. Allen, S. Salloch, V. Suren, K. Jongsma, M. Braun, D. Wilkinson, W. Sinnott-Armstrong, A. Rid, et al. 2024. A personalized patient preference predictor for substituted judgment in healthcare: Technically feasible and ethically desirable. The American Journal of Bioethics. Online ahead of print. doi: 10.1080/15265161.2023.2296402.
- Erler, A. 2023. Publish with AUTOGEN or perish? Some pitfalls to avoid in the pursuit of academic enhancement via personalized large language models. The American Journal of Bioethics 23 (10):94–6. doi:10.1080/15265161.2023.2250291.
- Ganguli, D., A. Askell, N. Schiefer, T. I. Liao, K. Lukošiūtė, A. Chen, A. Goldie, A. Mirhoseini, C. Olsson, D. Hernandez, et al. 2023. The capacity for moral self-correction in large language models. arXiv. doi:10.48550/arXiv.2302.07459.
- Grice, H. P. 1968. Utterer’s meaning, sentence-meaning, and word-meaning. Foundations of Language 4 (3):225–42.
- Grice, H. P. 1969. Utterer’s meaning and intention. The Philosophical Review 78 (2):147–77. doi:10.2307/2184179.
- Kar, R. B., and M. J. Radin. 2019. Pseudo-contract and shared meaning analysis. Harvard Law Review 132 (4):1135–219.
- Laacke, S., and C. Gauckler. 2023. Why personalized large language models fail to do what ethics is all about. The American Journal of Bioethics 23 (10):60–3. doi:10.1080/15265161.2023.2250292.
- Liedtke, W. 2004. Rembrandt’s “workshop” revisited. Oud Holland – Quarterly for Dutch Art History 117 (1–2):48–73. doi:10.1163/187501704X00278.
- McMillan, J. 2023. Generative AI and ethical analysis. The American Journal of Bioethics 23 (10):42–4. doi:10.1080/15265161.2023.2249852.
- Nyholm, S. 2023a. Artificial intelligence and human enhancement: Can AI technologies make us more (artificially) intelligent? Cambridge Quarterly of Healthcare Ethics 33 (1):76–88. doi:10.1017/S0963180123000464.
- Nyholm, S. 2023b. Is academic enhancement possible by means of generative AI-based digital twins? The American Journal of Bioethics 23 (10):44–7. doi:10.1080/15265161.2023.2249846.
- Ostertag, G. 2023. Meaning by courtesy: LLM-generated texts and the illusion of content. The American Journal of Bioethics 23 (10):91–3. doi:10.1080/15265161.2023.2249851.
- Pavlick, E. 2023. Symbols and grounding in large language models. Philosophical Transactions of the Royal Society A: Mathematical, Physical and Engineering Sciences A381:1–19. doi:10.1098/rsta.2022.0041.
- Piantadosi, S. T., and F. Hill. 2022. Meaning without reference in large language models, arXiv.org. Accessed January 12, 2024. https://arxiv.org/abs/2208.02957v2.
- Porsdam Mann, S., P. de Lora Deltoro, T. Cochrane, and C. Mitchell. 2018. Is the use of Modafinil, a pharmacological cognitive enhancer, cheating? Ethics and Education 13 (2):251–67. doi:10.1080/17449642.2018.1443050.
- Porsdam Mann, S., B. D. Earp, N. Møller, S. Vynn, and J. Savulescu. 2023a. AUTOGEN: A personalized large language model for academic enhancement—ethics and proof of principle. The American Journal of Bioethics 23 (10):28–41. doi:10.1080/15265161.2023.2233356.
- Porsdam Mann, S., B. D. Earp, S. Nyholm, J. Danaher, N. Møller, H. Bowman-Smart, J. Hatherley, J. Koplin, M. Plozza, D. Rodger, et al. 2023b. Generative AI entails a credit–blame asymmetry. Nature Machine Intelligence 5 (5):472–5. doi:10.1038/s42256-023-00653-1.
- Resnik, D. B., and M. Hosseini. 2023. The impact of AUTOGEN and similar fine-tuned large language models on the integrity of scholarly writing. The American Journal of Bioethics 23 (10):50–2. doi:10.1080/15265161.2023.2250276.
- Sorin, V., D. Brin, B. Yiftach, E. Konen, A. Charney, A., G. Nadkarni, and E. Klang. 2023. Large language models (LLMs) and empathy – a systematic review. medRxiv 2023.08.07.23293769.
- Tietze, H. 1939. Master and workshop in the Venetian renaissance. Parnassus 11 (8):34–45. doi:10.2307/772019.
- United Nations. 1969. Vienna convention on the Law of Treaties (adopted 23 May 1969, entry into force 27 January 1980) 1155 UNTS 331 (VCLT).
- Varma, S. 2023. Large language models and inclusivity in bioethics scholarship. The American Journal of Bioethics 23 (10):105–7. doi:10.1080/15265161.2023.2250286.
- Van Veen, D., C. Van Uden, L. Blankemeier, J.-B. Delbrouck, A. Aali, C. Bluethgen, A. Pareek, M. Polacin, E. P. Reis, A. Seehofnerová, et al. 2023. Clinical text summarization: Adapting large language models can outperform human experts. Research Square doi: 10.21203/rs.3.rs-3483777/v1.
- Zhang, T., F. Ladhak, E. Durmus, P. Liang, K. McKeown, and T. B. Hashimoto. 2023. Benchmarking large language models for news summarization, arXiv.org. Accessed January 11, 2024. https://arxiv.org/abs/2301.13848v1.
- Zohny, H. 2023. Reimagining scholarship: A response to the ethical concerns of AUTOGEN. The American Journal of Bioethics 23 (10):96–9. doi:10.1080/15265161.2023.2250315.
- Zohny, H., S. Porsdam Mann, B. D. Earp, and J. McMillan. 2024. Generative AI and medical ethics: The state of play. Journal of Medical Ethics. https://www.researchgate.net/publication/376757174_Generative_AI_and_Medical_Ethics_The_State_of_Play.