ABSTRACT
Women with triple-negative breast cancer (TNBC) have poor prognosis because of the aggressive nature of the tumor, delayed diagnosis and non-specific symptoms in the early stages. Identification of novel specific TNBC serum biomarkers for screening and therapeutic purposes therefore remains an urgent clinical requirement.We obtained serum samples from a total of 380 recruited individuals split into mining and testing sets, with the aim of screening for reliable protein biomarkers from TNBC and non-TNBC (NTNBC) sera. Samples were assessed using mass spectrometry, followed by receiver operating characteristic (ROC), survival and hazard function curve as well as multivariate Cox regression analyses to ascertain the potential of the protein constituents as diagnostic and prognostic biomarkers for TNBC.We identified upregulated apolipoprotein C-I (apoC-I) with a validated positive effect on TNBC tumorigenesis, with confirmation in an independent test set and minimization of systematic bias by pre-analytical parameters. The apoC-I protein had superior diagnostic ability in distinguishing between TNBC and NTNBC cases. Moreover, the protein presented a more robust potential prognostic factor for TNBC than NTNBC. The apoC-I protein identified in this study presents an effective novel diagnostic and prognostic biomarker for TNBC, indicating that measurement of the peak intensity at 7785 Da in serum samples could facilitate improved early detection and estimation of postoperative survival prognosis for TNBC.
Abbreviations
ApoC-I | = | apolipoprotein C-I |
TNBC | = | triple-negative breast cancer |
NTNBC | = | non- triple-negative breast cancer |
MS | = | mass spectrometry |
SELDI-TOF-MS | = | surface-enhanced laser desorption/ionization time-of-flight mass spectrometry |
MALDI-TOF/TOF MS | = | matrix-assisted laser desorption/ionization time-of-flight/time-of-flight mass spectrometry |
ROC | = | receiver operating characteristic |
ELISA | = | Enzyme-Linked Immunosorbent Assay |
WB | = | protein gel blot |
Sq-PCR | = | semi-quantitative polymerase chain reaction |
QRT-PCR | = | quantitative real-time polymerase chain reaction |
Introduction
Breast cancer (BC), the second most common cancer type after lung cancer, is the most frequently occurring malignancy and the leading cause of cancer-related mortality in women.Citation1,2 Triple-negative BC (TNBC), which accounts for 12–17% of all BCs,Citation3 is associated with higher risk of early development of visceral metastasis and local recurrence, compared to other breast cancer subtypes.Citation4 In Asia, the burden of TNBC is substantial, partly due to the younger age at diagnosis of breast cancer.Citation5–7 TNBC is characterized by lack of estrogen receptor/progesterone receptor (ER/PgR) expression and absence of human epidermal growth factor receptor 2 (HER2) overexpression or amplification, resulting in insensitivity to hormone or trastuzumab treatment.Citation8 Currently, the most common treatment option for TNBC patients is cytotoxic chemotherapy.Citation9 However, high rate of early relapse Citation10 and poor long-term outcomes Citation11 are reported, even for patients who initially respond well to therapy. Since the early stages of BC are nearly asymptomatic, the majority of cases are diagnosed at the later stages when it is often too late for curative treatment due to the absence of specific and sensitive tests that may facilitate early screening and monitoring of cancerous states.Citation12 Hence, early accurate diagnosis and timely treatment are critical for improving long-term survival of TNBC patients. The current screening methods to detect breast tumors include clinical examination, ultrasound, and mammography.Citation13 Although mammography screening has made a substantial contribution to reducing BC mortality, this technique has several limitations, including poor diagnosis in dense breast tissue and insufficient screening facilities.Citation14–16 In addition, for mammography detection, breast tumors should be at least a few millimeters in size.Citation17 However, a tumor of this size already contains several hundred thousand cells, and given that a single cell can lead to the development of a whole tumor, the stage is likely to be advanced at the time of detection with mammography. Alternatively, magnetic resonance imaging (MRI) for BC detection may be particularly applicable in young women for whom mammographic screening is less effective due to lower sensitivity.Citation18
Genomic expression profiling is a highly reliable method for cancer classification and prognostication.Citation19,20 However, genes lack catalytic and signaling capabilities, and instead exert their effects through translation into active proteins. Moreover, key natural biological processes, such as RNA alternative splicing and post-translational modification of proteins, lead to inherent limitations in genomic discovery studies. Consequently, post-genomic “proteomic” projects correlating protein expression profiles with cancer progression are essential for complementary and comprehensive elucidation of BC biology. Proteins secreted from tumor tissues have a greater likelihood of reaching the systemic circulation and may therefore serve as potential biomarkers for early detection.Citation21 Serum proteomics is an important tool that can facilitate comprehensive and systematic elucidation of the serum proteome under both healthy and disease conditions as well as identification of serum protein markers used for disease diagnosis and prognosis. While serum is generated by coagulation, its proteome is vulnerable to the proteases involved in this cascade as well as the complement cascade activated upon blood clotting. Examination of various pre-analytical parameters, such as the sampling device used, clotting temperature and time, storage duration and temperature, and incubation temperature and handling, has confirmed the importance of uniform handling to exclude systematic pre-analytical inconsistencies. False discovery can exert a distinct influence on the serum proteome, potentially leading to significant differences between results, even with comparable patient populations and sample types under study.Citation22–26 New proteomic technologies that promote large-scale sample screening and facilitate identification of proteins associated with disease and treatment are developing rapidly.Citation27,28 Mass spectrometry (MS), a powerful proteomics tool, has evolved to a high-throughput level, allowing rapid and accurate analysis of several thousand proteins in a single study.Citation29–31 Gel-free MS-based "shotgun" quantitative proteomics, a commonly used approach, is more sensitive and accurate, compared to 2-dimensional gel electrophoresis-based techniques.Citation32 Sodium dodecyl sulfate-polyacrylamide gel electrophoresis (SDS-PAGE), followed by in-gel digestion, is a protein separation technique based on molecular weight.Citation33 Surface-enhanced laser desorption/ionization time-of-flight MS (SELDI-TOF-MS), based on selective binding of proteins with different physicochemical properties on protein chip arrays, has been successfully applied to uncover crucial molecular events in many tumor types, such as liver,Citation34–36 lung,Citation37–39 prostate Citation40–42 and ovarian cancers.Citation43 Recently, a new high-throughput workflow with matrix-assisted laser desorption/ionization time-of-flight/time-of-flight MS (MALDI-TOF/TOF MS) based on magnetic beads with different chemical chromatographic surfaces instead of protein chip arrays was established for the effective discovery and identification of serum peptides,Citation44 whereby proteins selectively bound to magnetic beads are eluted and analyzed with MALDI-TOF MS. Several studies have addressed the possibility of applying MS proteome analysis to diagnostics of TNBC, revealing protein patterns specific for patients with TNBC at either early or late clinical stages.Citation45–49 The peptide markers identified with differentiating patterns include glycolytic enzymes, cytokeratins, vimentin, fibronectin, L-plastin, galectin-3-binding protein, cathepsin D preproprotein, melanoma-associated antigen 4, vimentin, peroxiredoxin 5, keratins, heat-shock proteins and human leukocyte antigen-class proteins. Using this system, we have identified novel biomarkers for papillary thyroid carcinoma, Wilms tumor, and BC.Citation50–52 However, all the earlier studies employed MS-based screening and did not include completely independent test sets to examine substantial heterogeneity in data with regard to standardized pre-analytical sample handling protocols. Moreover, the utility of biomarkers in relation to early detection and prognostic evaluation was not assessed. Therefore, the identification of novel and specific TNBC serum biomarkers for screening and therapeutic purposes remains an urgent clinical requirement. In the current study we included an independent test set from a second hospital and minimization of systematic bias impact by the above pre-analytical parameters, with the aim of screening for reliable protein biomarkers from serum samples using SELDI-TOF-MS, followed by protein identification using MALDI-TOF/TOF MS, polymerase chain reaction (PCR)-and immunoassay-based assessment using receiver operating characteristics (ROC), survival and hazard function curve as well as multivariate Cox regression analyses to ascertain their potential utility as diagnostic and prognostic biomarkers for TNBC.
Results
Serum protein profiles and data processing
Serum samples from the mining set were analyzed and compared using SELDI-TOF-MS with the WCX2 chip. All MS data were baseline-subtracted and normalized using total ion current, and peak clusters generated with Biomarker Wizard software. Wilcoxon rank sum tests used to determine relative signal strength disclosed 11 differentially expressed proteins, including 5 upregulated and 6 downregulated protein peak intensities from preoperative NTNBC patient samples, compared with controls (). Nine differentially expressed proteins, including 4 upregulated and 5 downregulated protein peak intensities, were observed for TNBC patients, compared with controls (), and 7 differentially expressed proteins, including 3 upregulated and 4 downregulated protein peak intensities, for TNBC, compared with NTNBC patients (). From the random combination of protein peaks with remarkable variations, the support vector machine (SVM) screened out the model with maximum Youden index of predicted value, leading to the identification of a marker positioned at 7785 Da with continuous dynamic presence in NTNBC and TNBC patient sera in addition to control sera. The 7785 Da protein peak was significantly higher in TNBC patient sera, compared with NTNBC and control sera (TNBC, 1979.565 ± 110.836; NTNBC, 1404.859 ± 383.252; control, 1306.598 ± 319.451; F = 133.2, p < 0.0001; ), while no significant differences were evident between NTNBC and control sera (p = 0.053). In addition, the 7785 Da protein peak in TNBC progressively increased through clinical stages I, II, III and IV (stage I, 1713.011 ± 163.911; stage II, 2003.282 ± 109.985; stage III, 2260.943 ± 150.214; stage IV, 2552.328 ± 181.292; F = 115.4, p < 0.0001; ). In contrast, while no significant differences in the peak level were observed in NTNBC samples (stage I, 1437.489 ± 386.085; stage II, 1683.986 ± 453.388; stage III, 1645.736 ± 431.287; stage IV, 1280.696 ± 199.760; F = 2.7, p = 0.052; ). Using leave-one-out cross-validation, the 7785 Da fragment was used to effectively distinguish TNBC from NTNBC with an accuracy of 76.7% (115/150), sensitivity of 77.8% (70/90), and specificity of 75.0% (45/60).
Figure 1. Follow-up of all enrolled triple-negative breast cancer(TNBC) and non-triple-negative breast cancer(NTNBC) patients from the mining and blind testing sets and representative expression map from surface-enhanced laser desorption/ionization time-of-flight mass spectrometry(SELDI-TOF-MS) analysis of sera from control, NTNBC and TNBC patients in the mining set. (A) Kaplan-Meier survival curves for NTNBC and TNBC patients from the mining and blind testing sets. (B) Graph of Hazard Function analysis between NTNBC and TNBC cases in the mining and blind testing sets. (C) The peak at 7785 Da was significantly elevated in TNBC patient sera, compared with NTNBC and control sera in the mining set (p < 0.0001). (D) Representative expression of the peak at 7785 Da (left panel,arrow) and gel views(right panel, arrow)from control, NTNBC and TNBC patient sera in the mining set.****p ≤ 0.0001.
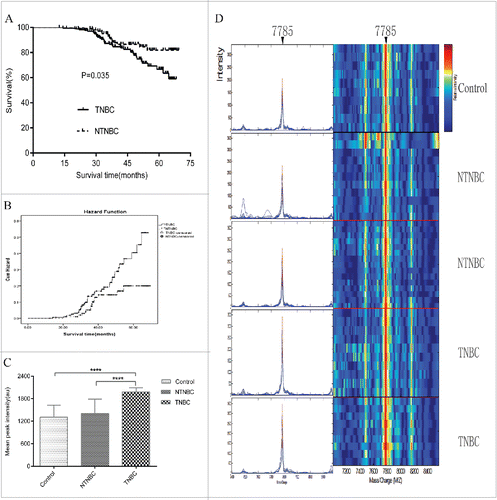
Figure 2. Typical expression map of SELDI-TOF-MS analysis of sera from different stages of TNBC and NTNBC. (A)Typical expression of the 7785 Da peak (left panel, arrow) and gel views (right panel, arrow) at different stages of TNBC (TNBC-I: patient with stage I TNBC; TNBC-II: patient with stage II TNBC; TNBC-III: patient with stage III TNBC; TNBC-IV: patient with stage IV TNBC). (B)The 7785 Da protein peak progressively increased with clinical stage in TNBC(p< 0.0001). (C) Typical expression of the 7785 Da protein (left panel, arrow) and gel views (right panel, arrow) at different stages of NTNBC (NTNBC-I: patient with stage I NTNBC; NTNBC-II: patient with stage II NTNBC; NTNBC-III: patient with stage III NTNBC; NTNBC-IV: patient with stage IV NTNBC). (D) The 7785 Da peak displayed no significant changes with increasing clinical stage in NTNBC(p = 0.052).** p ≤ 0.01,****p ≤ 0.0001.
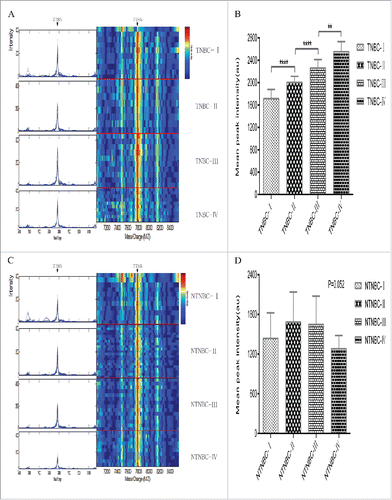
Table 1. Demographics of triple-negative breast cancer (TNBC) and non-triple-negative breast cancer (NTNBC) patients enrolled in the study.
Table 2. Comparison of protein peak intensities between controls and NTNBC patients in the mining set.
Table 3. Comparison of protein peak intensities between controls and TNBC patients in the mining set.
Table 4. Comparison of protein peak intensities between NTNBC and TNBC patients in the mining set.
Protein peak validation
The remaining 75 preoperative TNBC, 45 NTNBC and 50 control serum samples were analyzed as a blind testing set to validate the accuracy and validity of the 7785 Da protein marker identified from the mining set. The peak intensity of the 7785 Da protein in TNBC patients was consistently higher than that of NTNBC and control samples (TNBC, 1836.517 ± 171.128; NTNBC, 1495.170 ± 406.430; control, 1376.238 ± 360.759 ;F = 37.9, p < 0.0001; ), while no significant differences were detected between NTNBC and control sera (p = 0.062). The 7785 Da marker distinguished TNBC from NTNBC with 75.0% accuracy (90/120), 73.3% sensitivity (55/75), and 77.8% specificity (35/45).
Figure 3. Validation, identification and polymerase chain reaction (PCR) confirmation of the peak at 7785 Da. (A) Validation of the peak at 7785 Da in the blind testing set. (B)The intensity of the 7785 Da peak was higher in TNBC patient sera, compared with NTNBC and control sera in the blind testing set. (C)Representative matrix-assisted laser desorption/ionization time-of-flight/time-of-flight mass spectrometry(MALDI-TOF/TOF MS) map of peptide segments obtained after enzymatic digestion of protein and peptide segments with m/z of 7785 Da. (D) Ethidium bromide-stained semi-quantitative reverse transcription-PCR(RT-PCR) products of apoC-I mRNA in control, NTNBC and TNBC sera.Lanes 1–2: control; lanes 3–4: NTNBC; lanes 5–6: TNBC; ApoC-I gene expression (121bp) was analyzed via semi-quantitative RT-PCR, with β-actin (100 bp) as the internal control. (E)Representative quantitativereal-time PCR amplification plot of apoC-I in different serum samples; Rn, normalized report fluorescence. ((F) and G) Representative melting curves of apoC-I and β-actin during quantitative real-time PCR. (H) Relative apoC-I expression normalized to β-actin in different serum samples.****p ≤ 0.0001.
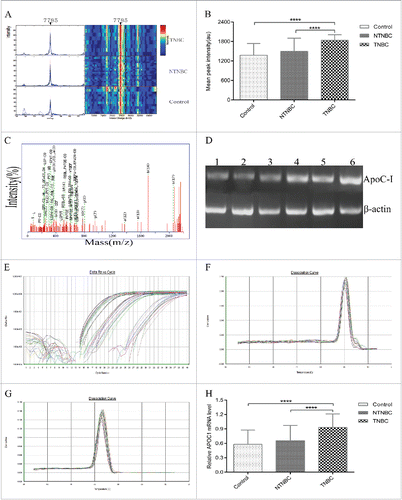
In-gel digestion and MALDI-TOF/TOF-MS identification of the candidate protein biomarker
Protein spots positioned at 7785 Da were excised from the gel with the Ettan Spot Picker, followed by digestion with trypsin and MALDI-TOF/TOF analysis (). The sequence of proteins and peptide segments with m/z of 7785 Da was identified as E.LFLSLPVLVG PAPAQGTPDLDKLKEFGNTLEDKARELSELSAK.G (complete sequences are not listed owing to patent pending status). Subsequent analysis using the MASCOT search program and NCBI database led to identification of the apolipoprotein C-I (apoC-I) peptide segment with a matching rate of 83.4% and matching score of 108.3 points ().
Table 5. Peptide sequences obtained after enzymatic digestion of protein and peptide segments with m/z of 7785 Da. Peptide sequences identified using the NCBI database are in bold.
Confirmation of candidate protein biomarker expression using sq-PCR, qRT-PCR, ELISA and WB
To further determine expression of the apoC-I deduced from the results of SELDI-TOF and MALDI-TOF/TOF MS, sq-PCR, qRT-PCR, ELISA and WB analyses were performed to examine apoC-I mRNA and protein expression using serum specimens from the testing set. Representative sq-PCR products from control, NTNBC and TNBC samples are shown in . Increased apoC-I mRNA expression was observed in TNBC, compared with NTNBC and control sera. Additionally, qRT-PCR was employed to examine apoC-I transcript levels in the 75 TNBC, 45 NTNBC and 50 control samples (). ApoC-I expression was significantly increased in TNBC, compared to NTNBC and control samples (control, 0.579 ± 0.295; NTNBC, 0.655 ± 0.316; TNBC, 0.929 ± 0.284; F = 24.4, p < 0.0001; ), but no significant differences were evident between NTNBC and control sera (p = 0.213) whereby ΔΔCt expression values were normalized to endogenous control β-actin gene and calculated relative to amplification of selected serum samples of TNBC patients, consistent with sq-PCR findings. Since full-length human apoC-I is 9.3 kDa, the m/z 7785 Da biomarker peptide with total sequence coverage > 83% represents the large fragment of apoC-I. Immunological analysis of this apoC-I was performed using an available antibody specific for the identified sequence. ELISA was additionally conducted to explore the apoC-I protein level in sera from the testing set. This biomarker was remarkably elevated in TNBC, compared with NTNBC and control sera (control, 0.302 ± 0.108; NTNBC, 0.404 ± 0.287; TNBC, 0.943 ± 0.575; F = 43.6, p < 0.0001; ), whereas no significant differences were observed between NTNBC and control sera (p = 0.232) whereby optical density (OD) ratios of apoC-I following normalization to a selected TNBC serum source were calculated and used as the vertical scale. WB data on apoC-I levels from the same serum samples with β-actin used as the loading control were consistent with ELISA findings (control, 0.299 ± 0.127; NTNBC, 0.343 ± 0.174; TNBC, 0.765 ± 0.204; F =134.3, p < 0.0001; ). As expected, no significant differences in the apoC-I band intensity were observed between NTNBC and control serum samples (p = 0.234).
Figure 4. Immunoassay-based confirmation of the diagnostic and prognostic value of the candidate protein biomarker based on Kaplan-Meier Survival and Hazard Function curve analyses. (A) Qualitative enzyme-linked immunosorbent assay (ELISA) confirmed SELDI-TOF and MALDI-TOF/TOF MS findings on the apoC-I protein biomarker in 50 control, 45 NTNBC and 75 TNBC samples. Expression of the apoC-I protein was significantly elevated in TNBC patient sera, compared with NTNBC and control sera. OD ratios of apoC-I following normalization to a selected TNBC serum source were calculated and used as the vertical scale. (B) Representative western blot analysis of the apoC-I protein biomarker in the same serum samples. Lanes 1–2: control; Lanes 3–4: NTNBC; Lanes 5–6: TNBC. β-Actin was used as a reference. (C) Grayscale scanning of protein gel blot bands, in which the ratio of the grayscale values of apoC-I to β-actin was used as the analyzed scale, revealed a similar trend to ELISA results. (D) Diagnostic value of apoC-I, determined using ROC curve, compared with NTNBC. (E) Kaplan-Meier survival curve for the 7785 Da peak in NTNBC patients divided into apoC-I-lower and apoC-I-higher groups from the testing set. (F) Graph of Hazard Function analysis between the apoC-I-lower and apoC-I-higher groups of NTNBC patients from the testing set. (G) Kaplan-Meier survival curve for the 7785 Da peak in TNBC patients divided into apoC-I-lower and apoC-I-higher groups from the testing set. (H) Graph of Hazard Function analysis between the apoC-I-lower and apoC-I-higher groups of TNBC patients from the testing set, supporting increased apoC-I peak intensity as a risk factor for poor prognosis.
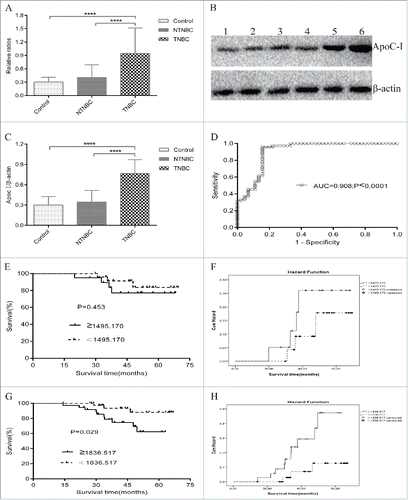
Diagnostic and prognostic value of the candidate protein biomarker
The relative intensity of the apoC-I peak in the testing set was used to determine its diagnostic and prognostic value. Sensitivities were determined from the results of 75 patients with TNBC and specificities from 45 NTNBC cases. Taking the histologically verified results in patients as the golden standard, the diagnostic value of apoC-I for TNBC, compared to that for NTNBC, was assessed by means of ROC curve, the AUC value of which was calculated as 0.908 (95% CI, 0.845–0.970; p < 0.0001; ).
Conventional prognostic factors were selected based on a priori knowledge of their associations with breast cancer survival, age at diagnosis, depth of tumor invasion, lymph node metastasis, distant metastasis, clinical stage, histological grade, tumor size and neoadjuvant chemotherapy. Multivariate Cox regression analysis including conventional prognostic variables and apoC-I peak intensity disclosed that the latter supports the utility of this protein as a strong independent prognostic factor for survival in TNBC (HR 4.326, 95% CI 1.176 – 15.918; χ2 = 4.857, p = 0.028), while the data did not reach statistical significance for NTNBC (HR 2.780, 95% CI 0.471 – 16.403; χ2 = 1.275, p = 0.259). No significant differences in survival time were evident between NTNBC patients with apoC-I peak intensities greater than or equal to the average relative intensity values, compared to those with apoC-I peak intensities lower than the average relative intensity values (apoC-I-higher, 60.0 m, 95% CI 52.8 – 67.2 m vs. apoC-I -lower, 64.7 m, 95% CI 59.6 – 69.7 m; HR 1.760, 95% CI 0.397 – 7.915; χ2 = 0.563, p = 0.453; ), based on Kaplan-Meier survival and hazard function analyses. In contrast, survival time for TNBC patients with apoC-I peak intensities greater than or equal to average relative intensity values were shorter than those of TNBC patients with peak intensities lower than the average relative intensity values (apoC-I-higher, 52.7 m, 95% CI 47.3 – 58.1 m vs. apoC-I-lower, 63.4 m, 95% CI 59.7 – 67.1 m; HR 3.778, 95% CI 1.133 – 10.010; χ2 = 4.744, p = 0.029; ), clearly indicating that higher apoC-I peak intensity is an unfavorable prognostic factor for TNBC.
Discussion
Patient blood, a dynamic reflection of physiological and pathological status, is a readily accessible matrix in which proteomes of multiple tissues can be analyzed. To date, several studies have identified potential candidates, but few have overcome validation and reproducibility issues to achieve clinical application.Citation53,54 To our knowledge, this is the first study to characterize apoC-I as a potential diagnostic and prognostic biomarker for TNBC based on MS across a broad spectrum of TNBC sera with further validation using an independent test set from a second hospital and minimization of systematic bias by pre-analytical parameters with a major impact on serum protein profiles. Protein chip MS led to the identification of a unique serum protein positioned at 7785 Da with continuous dynamic presence in NTNBC and TNBC patient sera in addition to control sera that effectively discriminated between TNBC and NTNBC patients with high sensitivity and specificity in the mining set. The 7785 Da protein panel screened using SVM was significantly upregulated in preoperative TNBC samples, compared with NTNBC and control sera, while no significant differences were evident between NTNBC and control sera, as validated in an independent testing set from a second hospital. While the specificity of the candidate protein biomarker was somewhat higher in the testing set than the mining set, sensitivity was slightly lower in the testing set. In addition to dynamic changes, the intensity of the 7785 Da protein peak in TNBC progressively increased at higher clinical stages but displayed no significant changes among all NTNBC stages, supporting the theory that excessive levels contribute to tumor progression. Using a combination of MALDI-TOF/TOF MS, PCR and immunological methods, the protein was identified as apoC-I secreted by the liver, the smallest member of the apolipoprotein family that forms a component of very low density lipoprotein (VLDL), chylomicrons (CM) and high density lipoprotein (HDL),Citation55 and promotes inhibition of lipoprotein lipase activity by factors, such as angiopoietin-like protein 4.Citation56 Despite the rapid evolution of MS-based proteomics methods, in terms of resolution power, mass accuracy, accurate quantification, and sequencing speed, together with parallel improvements in bioinformatics tools for analysis of large amounts of data, SELDI-TOF-MS we referred, popularly used in the first decade of 2000, still has a great impact on cancer proteomics to uncover crucial molecular events that drive malignant cells progression or response to therapy in recent years, and could pave the way for the identification of new therapeutic targets with higher sensitivity and resolving power.Citation57-60
Apolipoproteins (Apo-) bind lipids to form lipoproteins that transport these lipids through the lymphatic and circulatory systems. Serum and plasma lipoprotein metabolism are regulated and controlled by specific Apo- constituents of various lipoprotein classes, such as apoA-I, apoC-I, apoC-III, apo-H (β 2 glycoprotein), and others. ApoA-I, a regulator of tumor growth and metastasis, is involved in antiproliferative and proapoptotic activities via regulation of cancer cell differentiation,Citation61–63 supporting its potential not only as a biomarker but also a therapeutic agent in cancer. Hsu and coworkers recently demonstrated correlation of the dyslipidemia-associated apoA-I minor allele with unfavorable baseline characteristics in Chinese BC patients, and a 10-year follow-up study revealed poorest survival in patients carrying both minor alleles in the lymph node-negative group.Citation64 Emerging roles of apoC-III synthesized in the liver, and to a minor degree, small intestine, include directing the atherogenicity of high-density lipoprotein, intestinal dietary triglyceride trafficking and modulating insulin-secreting pancreatic β-cell survival and apoptosis via activation of the mitogen-activated protein kinase (MAPK) p38 and extracellular signal regulated protein kinases 1 and 2 (ERK1/2),Citation65,66 resulting in hyperglycemia, positive effects on proliferation and colony formation ability of BC cells, and high mammographic density-induced BC risk due to hypoinsulinemia.Citation67,68 An earlier study by Moore and colleagues provided evidence that common functional variations at the APOC1 gene locus increase susceptibility to renal cell carcinoma.Citation69 Takano et al.Citation70 compared pre- and postoperative serum protein profiles obtained from pancreatic cancer patients subjected to curative pancreatectomy using SELDI-TOF-MS, and identified 6420 and 6630 Da proteins as apoC-I, which correlated with poor prognosis. These results are relatable to our current findings. In this study, the authors further confirmed abundant expression of apoC-I in pancreas neoplastic epithelium and culture medium of the pancreatic cancer cell line in vitro, suggesting that cancer cells secrete apoC- I. Moreover, inhibition of apoC-I with short interfering RNA suppressed proliferation and induced apoptosis of pancreatic cancer cells, indicating that apoC-I contributes to the aggressive nature of pancreatic cancer through avoidance of spontaneous apoptotic cell death and should therefore serve as a useful novel therapeutic target. ApoC-I has additionally been reported to promote human aortic smooth muscle cell apoptosis by stimulating expression of caspase-3, a crucial executioner in the pathway leading to DNA fragmentation and apoptosis,Citation71,72 and identified as a putative BC biomarker using proteomic techniques, including SELDI-TOF-MS, MALDI-TOF/TOF-MS, isobaric tags for relative and absolute quantification (iTRAQ) and 2-dimensional nano-liquid chromatography coupled with tandem MS (2D-nanoLC-MS/MS).Citation57,73 However, in addition to systematic bias by pre-analytical parameters, these earlier reports employed MALDI-based screening and did not verify the results obtained with PCR- and immunoassay-based assays. PCR, ELISA and WB data from the current study confirmed that the apoC-I is dynamically regulated in NTNBC and TNBC sera, consistent with proteomic findings, suggesting that lipoprotein metabolism is dysregulated in TNBC. Remarkably, in our pilot experiment, apoC-I and apoC-I mimetic peptides enhanced growth of MDA-MB-231 cell cultures representing a ‘triple -negative’ breast cancer cell line subtype that is aggressive and has few treatment options, promoted proliferation in vitro and improved tumor growth in a MDA-MB-231 xenograft nude mouse model in vivo, in contrast to the MCF-7 (non-TNBC) cell line (data not shown). These data not only support the potential of apoC-I as a biomarker but also its therapeutic utility in TNBC, consistent with the viewpoint of Takano et al.Citation70 Further studies to determine whether apoC-I is secreted from TNBC cells and the molecular mechanisms underlying apoC-I-mediated inhibition of apoptosis in TNBC cells are warranted.
The diagnostic ability of the apoC-I determined based on ROC analysis revealed that the candidate protein biomarker has distinctly superior ability to distinguish patients with TNBC from those with NTNBC. Consistent with this result, Kaplan-Meier survival and hazard function as well as multivariate Cox regression analyses disclosed that the apoC-I presents a novel stronger prognostic factor for TNBC than NTNBC. Thus, measurement of the peak intensity at 7785 Da in serum should improve estimation of postoperative survival for TNBC patients. However, the identity of only a small proportion of detected peaks has been confirmed, and the roles of many of the identified proteins in TNBC development are not known as yet. Reliable validation of this potential TNBC biomarker in a larger, prospective clinical setting is necessary for translation to clinical application. In fact, previous experience suggests an expected timeline for biomarker succession to bedside translation averaging around 2 decades, a maturity phase which current proteomic efforts has not reached yet. Clearly, there is a lag between proteomic biomarker discovery and clinical use. Biomarker development runs through 5 step wise stages involving preclinical discovery, clinical assay development, retrospective longitudinal validation studies, prospective screening studies and finally randomized controlled studies. Current BC proteomics lie within the first 3 stages. In addition to the aforementioned pre-analytical factors contributing to this delay, clinical utility of any biomarker remains the biggest challenge.Clinical utility necessitates markers that will lead to improved patient outcomes and cost-effective care with minimal harm, which is best assessed by clinical trials. Additionally, further investigation is essential to determine whether this peptide is altered in cancers other than those of the liver. The issue of whether a causal link exists between TNBC and abnormal lipid metabolism is yet to be established, and complicated by the fact that the detected peak may be associated with inflammation rather than being specific for TNBC.
Materials and methods
Patients and serum samples
The study had been approved by the Zhengzhou University Ethics Committee, and written patient consent was obtained where appropriate from all participants. Serum samples from 380 individuals were included from the Division of Breast Surgery, the First Affiliated Hospital of Zhengzhou University, China, from July 2008 to April 2015 (mining set) and the Third Affiliated Hospital of Zhengzhou University, China, from September 2008 to June 2015 (testing set). The mining set consisted of preoperative serum samples from 90 patients with TNBC, 60 patients with non-TNBC (NTNBC) and 60 control subjects, including 25 with benign breast disease and 35 healthy donors. The blind testing set consisted of preoperative serum samples from 75 patients with TNBC, 45 patients with NTNBC and 50 control subjects, including 37 with benign lesions and 13 healthy donors, for the second study step. Patients were graded according to the modified Bloom–Richardson scoring system and staged based on the Union for International Cancer Control (UICC) TNM staging system (2010 edition). Tumors were classified as T1, tumor sizes ≤ 2 cm; T2, tumor sizes > 2 and ≤ 5 cm; T3, tumor sizes > 5 cm; T4, direct invasion into the chest wall (a) or skin (b) regardless of tumor size; T4c = T4a + T4b; T4d, inflammatory BC; N1, ipsilateral 1–3 lymph node-positive; N2, ipsilateral 4–9 lymph node-positive; N3, ipsilateral ≥ 10 lymph node-positive or ipsilateral supraclavicular lymph node metastases, and M1, distant metastasis. Patients with metastatic BC and axillary lymph node metastasis from other primary tumors were excluded. Clinicopathological data are summarized in . Patients with TNBC tended to be younger with higher tumor grade and larger tumor size, compared with the NTNBC group (p < 0.05). The large majority of postoperative patients received anthracyclines ± taxane-based adjuvant chemotherapy. Modified radical mastectomy was the most common type of mastectomy and was accompanied by at least a level II axillary dissection. Postmastectomy radiotherapy was indicated for patients presenting with 4 or more positive axillary lymph nodes, those with tumors measuring 5 cm or more in size, women with T4 disease and patients with positive surgical margins. Radiation was given to the chest wall and supraclavicular fossa (if nodespositive disease) using mega-voltage X-rays, delivering 50 Gy in 25 fractions over 5 d a week, for a period of 5 weeks. Patients treated with breast-conserving surgery received adjuvant chemotherapy followed by whole breast radiotherapy in which patients received 50 Gy in 25 fractions to the whole breast. Treatment was administered 5 d a week for a duration of 5 weeks followed by a 10 Gy boost to the tumor bed (5 fractions, daily over 1 week). All patients were treated with megavoltage photons and mostly planned using 3D conformal radiotherapy.The ipsilateral supraclavicular fossa was treated if patients had node-positive disease. Follow-up visits were performed at the outpatient clinic or via telephone interview every 3 months for the first 2 years, followed by every 6 months thereafter. Complete periodic follow-up of all BC patients from the mining and testing sets was recorded as of May 2015 (TNBC, 58.9 m, 95% CI 56.3–61.6 m vs. NTNBC, 63.9 m, 95% CI 61.3–66.6 m; χ2 = 4.431, p = 0.035; ).
In addition, patients did not have concomitant primary cancers and received no therapeutic intervention (including surgery, chemotherapy or hormone therapy) prior to sample collection. All participants were pre- or postoperatively histologically verified with BC or benign lesions via ultrasound-guided core needle biopsy or pathological examination by more than 2 senior pathologists. Data on estrogen receptors(ER), progesterone receptors(PR), and HER2/neu were obtained through standard clinical testing using immunohistochemistry (IHC) for ER and PR and the HerceptTest for HER2/neu. Pre-surgery fasting peripheral blood samples were collected in vacutainer tubes. Pre-analytical parameters, such as sampling device, clotting temperature and time, storage temperature and duration, incubation temperature and handling, were controlled by following an identical standard protocol for cases and controls. Normal, benign breast disease and BC serum samples were depleted of high molecular weight proteins via acetonitrile precipitation. Sera were incubated at room temperature for 1 h, centrifuged at 3000 rpm for 10 min, and stored at –80°C. For immunological confirmation of apolipoprotein C-I (apoC-I) and β-actin, a similar procedure using rabbit anti-apoC-I and anti-β-actin antibodies (Santa Cruz Biotechnology, Santa Cruz, CA) at a dilution of 1:400 overnight at 4°C, respectively, was employed. Details of SELDI-TOF-MS, bioinformatics, fractionation through tricine-sodium dodecyl sulfate-PAGE (TRICINE-SDS-PAGE), identification via MALDI-TOF/TOF MS and confirmation of candidate protein biomarkers with semi-quantitative polymerase chain reaction (sq-PCR), quantitative real-time polymerase chain reaction (qRT-PCR), enzyme-linked immunosorbent assay (ELISA) and western blot (WB) are presented in online Supplementary File 1.
Statistical analysis
Data were analyzed using the Zhejiang University Cancer Institute-Protein Chip Data Analysis System (ZUCIPDAS). The undecimated discrete wavelet transform (UDWT) method was applied to denoise the signals using Rice Wavelet Toolbox v. 2.4. Baseline correction was achieved by aligning spectra with a monotone local minimum curve, and mass calibration carried out by adjusting the intensity scale to 3 peaks present in all spectra. Peaks were filtered to maintain a signal-to-noise ratio (SNR) > 3, whereby SNR was defined as the ratio of the height of the peak above baseline to the wavelet-defined noise. To distinguish data from different groups, we used a nonlinear SVM classifier originally developed by Vladimir Vapnik with a radial-based function kernel, parameter Gamma of 0.6, and cost of constraint violation of 19. The leave-one-out crossing validation approach was applied to estimate the accuracy of this classifier. Quantitative variables, presented as means ± SD, were analyzed with unpaired Student's t-test and one-way ANOVA. Categorical variables were assessed using Pearson's χ2 test. ROC curves were utilized to assess the diagnostic value of candidate protein biomarkers. The prognostic value of conventional prognostic factors and 7785 Da peak intensity were assessed in multivariate analysis with the Cox proportional hazards model. Survival and hazard function curve analyses were performed using the Kaplan-Meier method, and significant levels assessed with the log-rank test. P-values were 2-sided and statistical significance set at p ≤ 0.05.
Disclosure of potential conflicts of interest
No potential conflicts of interest were disclosed.
Supplemental_Informations.doc
Download MS Word (55 KB)Acknowledgments
The authors are grateful to Professor Lijun Wang from the School of Foreign Languages of Zhengzhou University for language editing and polishing.
Funding
This work was supported by a grant (No.81172085) from the National Natural Science Foundation of China.
References
- Siegel RL, Miller KD, Jemal A. Cancer statistics, 2015. CA Cancer J Clin 2015; 65:5-29; PMID:25559415; http://dx.doi.org/10.3322/caac.21254
- DeSantis C, Ma J, Bryan L, Jemal A. Breast cancer statistics, 2013. CA Cancer J Clin 2014; 64:52-62; PMID:24114568; http://dx.doi.org/10.3322/caac.21203
- Foulkes WD, Smith IE, Reis-Filho JS. Triple-negative breast cancer. N Engl J Med 2010; 363:1938-48; PMID:21067385; http://dx.doi.org/10.1056/NEJMra1001389
- Lowery AJ, Kell MR, Glynn RW, Kerin MJ, Sweeney KJ. Locoregional recurrence after breast surgery: a systematic review by receptor phenotype. Breast Cancer Res Treat 2012; 133:831-41; PMID:22147079; http://dx.doi.org/10.1007/s10549-011-1891-6
- Pathy NB, Yip CH, Taib NA, Hartman M, Saxena N, Iau P, Bulgiba AM, Lee SC, Lim SE, Wong JE, et al. Singapore-Malaysia Breast Cancer Working Group. Breast cancer in a multi-ethnic Asian setting: results from the Singapore-Malaysia hospital-based breast cancer registry. Breast 2011; 20(Suppl 2):S75-80; PMID:21316967; http://dx.doi.org/10.1016/j.breast.2011.01.015
- Kwong A, Mang OW, Wong CH, Chau WW, Law SC. Hong Kong Breast Cancer Research Group. Breast cancer in Hong Kong, Southern China: the first population-based analysis of epidemiological characteristics, stage-specific, cancer-specific, and disease-free survival in breast cancer patients:1997–2001. Ann Surg Oncol 2011; 18:3072-8; PMID:21847700; http://dx.doi.org/10.1245/s10434-011-1960-4
- Raina V, Bhutani M, Bedi R, Sharma A, Deo SV, Shukla NK, Mohanti BK, Rath GK. Clinical features and prognostic factors of early breast cancer at a major cancer center in North India. Indian J Cancer 2005; 42:40-5; PMID:15805691; http://dx.doi.org/10.4103/0019-509X.15099
- Teng YH, Tan WJ, Thike AA, Cheok PY, Tse GM, Wong NS, Yip GW, Bay BH, Tan PH. Mutations in the epidermal growth factor receptor (EGFR) gene in triple negative breast cancer: possible implications for targeted therapy. Breast Cancer Res 2011; 13:R35; PMID:21457545; http://dx.doi.org/10.1186/bcr2857
- Amir E, Ocana A, Freedman O, Clemons M, Seruga B. Chemotherapy:dose-dense treatment for triple-negative breast cancer. Nat Rev Clin Oncol 2010; 7:79-80; PMID:20118978; http://dx.doi.org/10.1038/nrclinonc.2009.231
- Hama Y, Nakagawa K. Early distant relapse in early stage triple-negative breast cancer: usefulness of FDG-PET for diagnosis of distant metastases. Breast Cancer 2013; 20:191-3; PMID:20082162; http://dx.doi.org/10.1007/s12282-009-0195-8
- Carey L, Winer E, Viale G, Cameron D, Gianni L. Triple-negative breast cancer:disease entity or title of convenience?. Nat Rev Clin Oncol 2010; 7:683-92; PMID:20877296; http://dx.doi.org/10.1038/nrclinonc.2010.154
- de Gelder R, van As E, Tilanus-Linthorst MM, Bartels CC, Boer R, Draisma G, de Koning HJ. Breast cancer screening: evidence for false reassurance?. Int J Cancer 2008; 123:680-6; PMID:18484587; http://dx.doi.org/10.1002/ijc.23540
- Kroman NT, Grinsted P, Nielsen NS. Symptoms and diagnostic work-up in breast cancer. Ugeskr Laeger 2007; 169:2980-1; PMID:17953874
- Tabar L, Yen MF, Vitak B, Chen HH, Smith RA, Duffy SW. Mammography service screening and mortality in breast cancer patients: 20-year follow-up before and after introduction of screening. Lancet 2003; 361:1405-10; PMID:12727392; http://dx.doi.org/10.1016/S0140-6736(03)13143-1
- Elmore JG, Armstrong K, Lehman CD, Fletcher SW. Screening for breast cancer. JAMA 2005; 293:1245-56; PMID:15755947; http://dx.doi.org/10.1001/jama.293.10.1245
- Chérel P, Hagay C, Benaim B, De Maulmont C, Engerand S, Langer A, Talma V. Mammographic evaluation of dense breasts:techniques and limits. J Radiol 2008; 89:1156-68; PMID:18772800; http://dx.doi.org/10.1016/S0221-0363(08)73926-X
- Ding J, Warren R, Warsi I, Day N, Thompson D, Brady M, Tromans C, Highnam R, Easton D. Evaluating the effectiveness of using standard mammogram form to predict breast cancer risk: case-control study. Cancer Epidemiol Biomarkers Prev 2008; 17:1074-81; PMID:18483328; http://dx.doi.org/10.1158/1055-9965.EPI-07-2634
- Lord SJ, Lei W, Craft P, Cawson JN, Morris I, Walleser S, Griffiths A, Parker S, Houssami N. A systematic review of the effectiveness of magnetic resonance imaging (MRI) as an addition to mammography and ultrasound in screening young women at high risk of breast cancer. Eur J Cancer 2007; 43:1905-17; PMID:17681781; http://dx.doi.org/10.1016/j.ejca.2007.06.007
- Zeidan BA, Townsend PA. SELDI-TOF proteomic profiling of breast carcinomas identifies clinicopathologically relevant groups of patients similar to previously defined clusters from cDNA expression. Breast Cancer Res 2008; 10:107; PMID:18644101; http://dx.doi.org/10.1186/bcr2107
- Curtis C, Shah SP, Chin SF, Turashvili G, Rueda OM, Dunning MJ, Speed D, Lynch AG, Samarajiwa S, Yuan Y, et al. The genomic and transcriptomic architecture of 2,000 breast tumours reveals novel subgroups. Nature 2012; 486:346-52; PMID:22522925; http://dx.doi.org/10.1038/nature10983
- Kulasingam V, Diamandis EP. Strategies for discovering novel cancer biomarkers through utilization of emerging technologies. Nat Clin Pract Oncol 2008; 5:588-99; PMID:18695711; http://dx.doi.org/10.1038/ncponc1187
- Engwegen JY, Alberts M, Knol JC, Jimenez CR, Depla AC, Tuynman H, van Heukelem HA, Snel P, Smits ME, Cats A, et al. Influence of variations in sample handling on SELDI-TOF MS serum protein profiles for colorectal cancer. Proteomics Clin Appl 2008; 2:936-45; PMID:21136891; http://dx.doi.org/10.1002/prca.200780068
- Gast MC, van Gils CH, Wessels LF, Harris N, Bonfrer JM, Rutgers EJ, Schellens JH, Beijnen JH. Influence of sample storage duration on serum protein profiles assessed by surface-enhanced laser desorption/ionisation time-of-flight mass spectrometry (SELDI-TOF MS). Clin Chem Lab Med 2009; 47:694-705; PMID:19416081; http://dx.doi.org/10.1515/CCLM.2009.151
- Hsieh SY, Chen RK, Pan YH, Lee HL. Systematical evaluation of the effects of sample collection procedures on low-molecular-weight serum/plasma proteome profiling. Proteomics 2006; 6:3189-98; PMID:16586434; http://dx.doi.org/10.1002/pmic.200500535
- Timms JF, Arslan-Low E, Gentry-Maharaj A, Luo Z, T'Jampens D, Podust VN, Ford J, Fung ET, Gammerman A, Jacobs I, et al. Preanalytic influence of sample handling on SELDI-TOF serum protein profiles. Clin Chem 2007; 53:645-56; PMID:17303688; http://dx.doi.org/10.1373/clinchem.2006.080101
- Zeidan BA, Cutress RI, Murray N, Coulton GR, Hastie C, Packham G, Townsend PA. Proteomic analysis of archival breast cancer serum. Cancer Genomics Proteomics 2009; 6:141-7; PMID:19487543
- Smith L, Lind MJ, Welham KJ, Cawkwell L. Cancer Biology Proteomics Group. Cancer proteomics and its application to discovery of therapy response markers in human cancer. Cancer 2006; 107:232-41; PMID:16752413; http://dx.doi.org/10.1002/cncr.22000
- Diamond DL, Proll SC, Jacobs JM, Chan EY, Camp DG 2nd, Smith RD, Katze MG. HepatoProteomics: applying proteomic technologies to the study of liver function and disease. Hepatology 2006; 44:299-308; PMID:16871559; http://dx.doi.org/10.1002/hep.21318
- Wilhelm M, Schlegl J, Hahne H, Moghaddas Gholami A, Lieberenz M, Savitski MM, Ziegler E, Butzmann L, Gessulat S, Marx H, et al. Mass-spectrometry-based draft of the human proteome. Nature 2014; 509:582-7; PMID:24870543; http://dx.doi.org/10.1038/nature13319
- Nilsson T, Mann M, Aebersold R, Yates JR 3rd, Bairoch A, Bergeron JJ. Mass spectrometry in high-throughput proteomics: ready for the big time. Nat Methods 2010; 7:681-5; PMID:20805795; http://dx.doi.org/10.1038/nmeth0910-681
- Kim MS, Pinto SM, Getnet D, Nirujogi RS, Manda SS, Chaerkady R, Madugundu AK, Kelkar DS, Isserlin R, Jain S, et al. A draft map of the human proteome. Nature 2014; 509:575-81; PMID:24870542; http://dx.doi.org/10.1038/nature13302
- Tan HT, Lee YH, Chung MC. Cancer proteomics. Mass Spectrom Rev 2012; 31:583-605; PMID:22422534; http://dx.doi.org/10.1002/mas.20356
- George IS, Fennell AY, Haynes PA. Protein identification and quantification from riverbank grape, Vitis riparia: Comparing SDS-PAGE and FASP-GPF techniques for shotgun proteomic analysis. Proteomics 2015; 15:3061-5; PMID:25929842; http://dx.doi.org/10.1002/pmic.201500085
- Kim J, Kim SH, Lee SU, Ha GH, Kang DG, Ha NY, Ahn JS, Cho HY, Kang SJ, Lee YJ, et al. Proteome analysis of human liver tumor tissue by two-dimensional gel electrophoresis and matrix assisted laser desorption/ionization-mass spectrometry for identification of disease-related proteins. Electrophoresis 2002; 23:4142-56; PMID:12481271; http://dx.doi.org/10.1002/elps.200290032
- Zinkin NT, Grall F, Bhaskar K, Otu HH, Spentzos D, Kalmowitz B, Wells M, Guerrero M, Asara JM, Libermann TA, et al. Serum proteomics and biomarkers in hepatocellular carcinoma and chronic liver disease. Clin Cancer Res 2008; 14:470-7; PMID:18223221; http://dx.doi.org/10.1158/1078-0432.CCR-07-0586
- Feng Y, Tian ZM, Wan MX, Zheng ZB. Protein profile of human hepatocarcinoma cell line SMMC-7721: identification and functional analysis. World J Gastroenterol 2007; 13:2608-14; PMID:17552011; http://dx.doi.org/10.3748/wjg.v13.i18.2608
- Li C, Chen Z, Xiao Z, Wu X, Zhan X, Zhang X, Li M, Li J, Feng X, Liang S, et al. Comparative proteomics analysis of human lung squamous carcinoma. Biochem Biophys Res Commun 2003; 309:253-60; PMID:12943690; http://dx.doi.org/10.1016/j.bbrc.2003.08.001
- Au JS, Cho WC, Yip TT, Law SC. Proteomic approach to biomarker discovery in cancer tissue from lung adenocarcinoma among nonsmoking Chinese women in Hong Kong. Cancer Invest 2008; 26:128-35; PMID:18259942; http://dx.doi.org/10.1080/07357900701788031
- Xiao X, Liu D, Tang Y, Guo F, Xia L, Liu J, He D. Development of proteomic patterns for detecting lung cancer. Dis Markers 2003–2004; 19:33-9; PMID:14757945; http://dx.doi.org/10.1155/2003/278152
- Lin JF, Xu J, Tian HY, Gao X, Chen QX, Gu Q, Xu GJ, Song JD, Zhao FK. Identification of candidate prostate cancer biomarkers in prostate needle biopsy specimens using proteomic analysis. Int J Cancer 2007; 121:2596-605; PMID:17722004; http://dx.doi.org/10.1002/ijc.23016
- Adam BL, Qu Y, Davis JW, Ward MD, Clements MA, Cazares LH, Semmes OJ, Schellhammer PF, Yasui Y, Feng Z, et al. Serum protein fingerprinting coupled with a pattern-matching algorithm distinguishes prostate cancer from benign prostate hyperplasia and healthy men. Cancer Res 2002; 62:3609-14; PMID:12097261
- Qu Y, Adam BL, Yasui Y, Ward MD, Cazares LH, Schellhammer PF, Feng Z, Semmes OJ, Wright GL Jr. Boosted decision tree analysis of surface-enhanced laser desorption/ionization mass spectral serum profiles discriminates prostate cancer from noncancer patients. Clin Chem 2002; 48:1835-43; PMID:12324514
- Gagnon A, Kim JH, Schorge JO, Ye B, Liu B, Hasselblatt K, Welch WR, Bandera CA, Mok SC. Use of a combination of approaches to identify and validate relevant tumor associated antigens and their corresponding autoantibodies in ovarian cancer patients. Clin Cancer Res 2008; 14:764-71; PMID:18245537; http://dx.doi.org/10.1158/1078-0432.CCR-07-0856
- Villanueva J, Philip J, Entenberg D, Chaparro CA, Tanwar MK, Holland EC, Tempst P. Serum peptide profiling by magnetic particle-assisted, automated sample processing and MALDI-TOF mass spectrometry. Anal Chem 2004; 76:1560-70; PMID:15018552; http://dx.doi.org/10.1021/ac0352171
- Schulz DM, Böllner C, Thomas G, Atkinson M, Esposito I, Höfler H, Aubele M. Identification of differentially expressed proteins in triple-negative breast carcinomas using DIGE and mass spectrometry. J Proteome Res 2009; 8:3430-8; PMID:19485423; http://dx.doi.org/10.1021/pr900071h
- He J, Whelan SA, Lu M, Shen D, Chung DU, Saxton RE, Faull KF, Whitelegge JP, Chang HR. Proteomic-based biosignatures in breast cancer classification and prediction of therapeutic response. Int J Proteomics 2011; 2011:896476; PMID:22110952; http://dx.doi.org/10.1155/2011/896476
- Cabezón T, Gromova I, Gromov P, Serizawa R, Timmermans Wielenga V, Kroman N, Celis JE, Moreira JM. Proteomic profiling of triple-negative breast carcinomas in combination with a three-tier orthogonal technology approach identifies MageA4 as potential therapeutic target in estrogen receptor negative breast cancer. Mol Cell Proteomics 2013; 12:381-94; PMID:23172894; http://dx.doi.org/10.1074/mcp.M112.019786
- Lu M, Whelan SA, He J, Saxton RE, Faull KF, Whitelegge JP, Chang HR. Hydrophobic Proteome Analysis of Triple Negative and Hormone-Receptor-Positive-Her2-Negative Breast Cancer by Mass Spectrometer. Clin Proteomics 2010; 6:93-103; PMID:20930921; http://dx.doi.org/10.1007/s12014-010-9052-1
- Liu AN, Sun P, Liu JN, Yu CY, Qu HJ, Jiao AH, Zhang LM. Analysis of the differences of serum protein mass spectrometry in patients with triple negative breast cancer and non-triple negative breast cancer. Tumour Biol 2014; 35:9751-7; PMID:24972967; http://dx.doi.org/10.1007/s13277-014-2221-5
- Fan Y, Shi L, Liu Q, Dong R, Zhang Q, Yang S, Fan Y, Yang H, Wu P, Yu J, et al. Discovery and identification of potential biomarkers of papillary thyroid carcinoma. Mol Cancer 2009; 8:79; PMID:19785722; http://dx.doi.org/10.1186/1476-4598-8-79
- Wang J, Wang L, Zhang D, Fan Y, Jia Z, Qin P, Yu J, Zheng S, Yang F. Identification of potential serum biomarkers for Wilms tumor after excluding confounding effects of common systemic inflammatory factors. Mol Biol Rep 2012; 39:5095-104; PMID:22160518; http://dx.doi.org/10.1007/s11033-011-1305-1
- Fan Y, Wang J, Yang Y, Liu Q, Fan Y, Yu J, Zheng S, Li M, Wang J. Detection and identification of potential biomarkers of breast cancer. J Cancer Res Clin Oncol 2010; 136:1243-54; PMID:20237941; http://dx.doi.org/10.1007/s00432-010-0775-1
- Poste G. Bring on the biomarkers. Nature 2011; 469:156-7; PMID:21228852; http://dx.doi.org/10.1038/469156a
- Chung L, Baxter RC. Breast cancer biomarkers: proteomic discovery and translation to clinically relevant assays. Expert Rev Proteomics 2012; 9:599-614; PMID:23256671; http://dx.doi.org/10.1586/epr.12.62
- Puppione DL, Ryan CM, Bassilian S, Souda P, Xiao X, Ryder OA, Whitelegge JP. Detection of two distinct forms of apoC-I in great apes. Comp Biochem Physiol Part D Genomics Proteomics 2010; 5:73-9; PMID:20209111; http://dx.doi.org/10.1016/j.cbd.2009.12.003
- Larsson M, Vorrsjö E, Talmud P, Lookene A, Olivecrona G. Apolipoproteins C-I and C-III inhibit lipoprotein lipase activity by displacement of the enzyme from lipid droplets. J Biol Chem 2013; 288:33997-4008; PMID:24121499; http://dx.doi.org/10.1074/jbc.M113.495366
- Chung L, Moore K, Phillips L, Boyle FM, Marsh DJ, Baxter RC. Novel serum protein biomarker panel revealed by mass spectrometry and its prognostic value in breast cancer. Breast Cancer Res 2014; 16:R63; PMID:24935269; http://dx.doi.org/10.1186/bcr3676
- Chung L, Phillips L, Lin MZ, Moore K, Marsh DJ, Boyle FM, Baxter RC. A novel truncated form of S100P predicts disease-free survival in patients with lymph node positive breast cancer. Cancer Lett 2015; 368:64-70; PMID:26276712; http://dx.doi.org/10.1016/j.canlet.2015.07.046
- Sun L, Li L, Li Z, Hong S, Yang Q, Qu X, Kong B. Alterations in the serum proteome profile during the development of ovarian cancer. Int J Oncol 2014; 45:2495-501; PMID:25340615; http://dx.doi.org/10.3892/ijo.2014.2675
- De Bock M, Beguin Y, Leprince P, Willems E, Baron F, Deroyer C, Seidel L, Cavalier E, de Seny D, Malaise M, et al. Comprehensive plasma profiling for the characterization of graft-versus-host disease biomarkers. Talanta 2014; 125:265-75; PMID:24840443; http://dx.doi.org/10.1016/j.talanta.2014.03.017
- Zamanian-Daryoush M, Lindner D, Tallant TC, Wang Z, Buffa J, Klipfell E, Parker Y, Hatala D, Parsons-Wingerter P, Rayman P, et al. The cardioprotective protein apolipoprotein A1 promotes potent anti-tumorigenic effects. J Biol Chem 2013; 288:21237-52; PMID:23720750; http://dx.doi.org/10.1074/jbc.M113.468967
- Kim MY, Yoo BC, Cho JY. Ginsenoside-Rp1-induced apolipoprotein A-1 expression in the LoVo human colon cancer cell line. J Ginseng Res 2014; 38:251-5; PMID:25379004; http://dx.doi.org/10.1016/j.jgr.2014.06.003
- Su F, Kozak KR, Imaizumi S, Gao F, Amneus MW, Grijalva V, Ng C, Wagner A, Hough G, Farias-Eisner G, et al. Apolipoprotein A-I (apoA-I) and apoA-I mimetic peptides inhibit tumor development in a mouse model of ovarian cancer. Proc Natl Acad Sci USA 2010; 107:19997-20002; PMID:21041624; http://dx.doi.org/10.1073/pnas.1009010107
- Hsu MC, Lee KT, Hsiao WC, Wu CH, Sun HY, Lin IL, Young KC. The dyslipidemia-associated SNP on the APOA1/C3/A5 gene cluster predicts post-surgery poor outcome in Taiwanese breast cancer patients: a 10-year follow-up study. BMC Cancer 2013; 13:330; PMID:23829168; http://dx.doi.org/10.1186/1471-2407-13-330
- Kohan AB. Apolipoprotein C-III: a potent modulator of hypertriglyceridemia and cardiovascular disease. Curr Opin Endocrinol Diabetes Obes 2015; 22:119-25; PMID:25692924; http://dx.doi.org/10.1097/MED.0000000000000136
- Sol EM, Sundsten T, Bergsten P. Role of MAPK in apolipoprotein CIII-induced apoptosis in INS-1E cells. Lipids Health Dis 2009; 8:3; PMID:19196457; http://dx.doi.org/10.1186/1476-511X-8-3
- Adham SA, Al Rawahi H, Habib S, Al Moundhri MS, Viloria-Petit A, Coomber BL. Modeling of hypo/hyperglycemia and their impact on breast cancer progression related molecules. PLoS One 2014; 9:e113103; PMID:25401697; http://dx.doi.org/10.1371/journal.pone.0113103
- Kim BK, Chang Y, Ahn J, Jung HS, Kim CW, Yun KE, Kwon MJ, Suh BS, Chung EC, Shin H, et al. Metabolic syndrome, insulin resistance, and mammographic density in pre- and postmenopausal women. Breast Cancer Res Treat 2015; 153:425-34; PMID:26277917; http://dx.doi.org/10.1007/s10549-015-3544-7
- Moore LE, Brennan P, Karami S, Menashe I, Berndt SI, Dong LM, Meisner A, Yeager M, Chanock S, Colt J, et al. Apolipoprotein E/C1 locus variants modify renal cell carcinoma risk. Cancer Res 2009; 69:8001-8; PMID:19808960; http://dx.doi.org/10.1158/0008-5472.CAN-09-1734
- Takano S, Yoshitomi H, Togawa A, Sogawa K, Shida T, Kimura F, Shimizu H, Tomonaga T, Nomura F, Miyazaki M. Apolipoprotein C-1 maintains cell survival by preventing from apoptosis in pancreatic cancer cells. Oncogene 2008; 27:2810-22; PMID:18037960; http://dx.doi.org/10.1038/sj.onc.1210951
- McNeal CJ, Chatterjee S, Hou J, Worthy LS, Larner CD, Macfarlane RD, Alaupovic P, Brocia RW. Human HDL containing a novel apoC-I isoform induces smooth muscle cell apoptosis. Cardiovasc Res 2013; 98:83-93; PMID:23354389; http://dx.doi.org/10.1093/cvr/cvt014
- Kolmakova A, Kwiterovich P, Virgil D, Alaupovic P, Knight-Gibson C, Martin SF, Chatterjee S. Apolipoprotein C-I induces apoptosis in human aortic smooth muscle cells via recruiting neutral sphingomyelinase. Arterioscler Thromb Vasc Biol 2004; 24:264-9; PMID:14670935; http://dx.doi.org/10.1161/01.ATV.0000112036.72200.ac
- Opstal-van Winden AW, Krop EJ, Kåredal MH, Gast MC, Lindh CH, Jeppsson MC, Jönsson BA, Grobbee DE, Peeters PH, Beijnen JH, et al. Searching for early breast cancer biomarkers by serum protein profiling of pre-diagnostic serum; a nested case-control study. BMC Cancer 2011; 11:381; PMID:21871081; http://dx.doi.org/10.1186/1471-2407-11-381