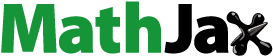
ABSTRACT
Muscle-invasive bladder urothelial carcinoma (MIBC) is a highly invasive cancer, which leads to prevalent recurrence and poor prognosis. Exploring the association of DNA methylation and the prognosis of MIBC will thus be of important value in clinical management and treatment. Bumphunter method and adaptive lasso regression were used to explore the relationship between different methylation regions (DMRs) and the prognosis of MIBC. Next, we constructed a risk prognosis model and validated this model. Moreover, the performance of this risk model was examined by using time-dependent receiver operating characteristic curve (ROC). We identified 58,449 different methylation sites and 490 different methylation regions. Among them, 11 DMRs were associated with the prognosis of MIBC through rigorous screening. Through the linear combination of 11 DMRs, a putative marker was developed, which can distinguish the survival risk in both the training dataset (HR = 2.58, 95% CI = (1.64, 4.05)) and the verification dataset (HR = 2.77, 95% CI = (1.25, 6.15)). Relatively high predictive values were observed from this model for training dataset (AUC = 0.791) and verification dataset (AUC = 0.668). Stratified analysis showed that the association was independent of gender. A nomogram was additionally generated to predict 5-year survival probability containing risk score and pathological stage. Its performance was evaluated by applying calibration curve. The methylation signature risk model based on 11 DMRs may be a reliable prognostic signature for MIBC, which provides new insights into development of individualized therapy for MIBC.
Introduction
Bladder cancer is a highly prevalent cancer arising from urothelial epithelium. 95% of bladder cancer are urothelial carcinoma, also called transitional cell carcinoma.Citation1 On average, 430,000 incident bladder cancer cases and 165,000 deaths are reported approximately each year worldwide.Citation2 Among them, about 25% of new cases are diagnosed as muscle invasive bladder urothelial carcinoma (MIBC), and 30–40% of nonmuscle invasive bladder urothelial carcinoma (NMIBC) will gradually infiltrate the muscular wall of the bladder to progress to MIBC.Citation3 The pathological grade of MIBC is usually higher and is more prone to distal metastasis, which brings about an unfavorable 5-year survival of being 30–50%.Citation4
In clinical practice, radical cystectomy of bladder in conjunction with neo-adjuvant therapy such as cisplatin is still the main treatment for MIBC.Citation5 But the response rate to cisplatin-based therapy is below 50%, and 63% of patients are forced to adjust doses because of toxicity from chemotherapy.Citation6,Citation7 As a result, the survival rate of MIBC has not improved in the past 30 years.Citation8 In consideration of the highly invasive nature and the genetic variability of MIBC, identifying important risk markers associated with MIBC prognosis at the molecular level will thus be of great value in exploring new individualized therapies and improving survival rates.
DNA methylation is a kind of epigenetic modification of human genome, which is related to several key processes, for example, genomic imprinting, repression of transposable elements, carcinogenesis, and so on.Citation9,Citation10 Association of DNA methylation and development of cancer has been reported in previous studies.Citation11–13 However, few studies have examined the role of a single DNA methylation site as prognostic and metastatic markers for MIBC among limited sample size, which hindered the generalization of findings to some extent.Citation14,Citation15 While existing evidences support that some functional changes are more related to the genomic region than to a single CpGs (5ʹ-C-phosphate-G-3ʹ) site.Citation16 Therefore, more attentions are suggested to be given to the abnormal methylation of gene region.
In this study, we used bumphunter algorithm to analyze the level 3 methylation data of 419 samples from The Cancer Genome Atlas (TCGA) database (https://portal.gdc.cancer.gov/) in order to screen differential methylation regions (DMRs) between tumor and normal tissues. Among them, we identified those DMRs related to overall survival, constructed a risk score model based on 11 DMRs in training dataset, and verified the model in validation dataset. Further, a time-dependent receiver operating characteristic (ROC) curve was applied to evaluate the predictive value of the 5-year survival rate of the model.
Material and methods
Data collection
We downloaded level 3 methylation data of 421 muscle invasive bladder urothelial carcinoma (MIBC) samples (Illumina Infinium Human DNA Methylation 450 platform, beta values) and the corresponding clinical information (GDC) (Bethesda, MD, USA). Among them, 400 were tumor samples and 21 were adjacent normal tissue samples. Meanwhile, batch information for methylation data were downloaded from MD Anderson Cancer Center.
Data preprocessing
The TCGA level 3 methylation data had been converted to a β value, representing the degree of methylation at the CpGs site. First, we used the ChIPseekerCitation17 R package to re-annotate the location of all probes based on the GRCh38 annotation file and the promoter region defined as upstream 2.0 kb to downstream 0.5kb of the transcriptional start site. Further, we deleted the XY chromosome probes, the SNP probes, the non-CpG site probes, the multiple matching probes, and the nonpromoter region probes. Meanwhile, we eliminated probes with missing values greater than 5% and applied the ChAMP pipeline to do the missing value filling, quality control, and BMIQCitation18 normalization.Citation19 Subsequently, we used ComBatCitation20 to correct for potential confounders, such as time and sampling location. Finally, 399 tumor samples and 20 normal samples with a total of 169,730 probes were included in the follow-up analysis. Also, we categorized the age into two groups (<60 and ≥60), divided pathological Stage into Stage II group and Stage III and IV group, and limited the follow-up time to 5 years (60 months) to investigate their effects on the prognosis.
Screening differential methylation regions
In order to reduce the heteroscedasticity, the β value was transformed into M value according to the formula .Citation21 First, the empirical Bayes method in limma R package was applied to screen the differentially methylated probes (DMPs) in both tumor and normal tissues.Citation22 Second, we identified tumor and normal differential methylation regions (DMRs) using bumphunter method,Citation23 in which clusters were defined by neighboring CpGs site of at an interval at most 500bp and 1,000 bootstrap samples were used to generate a null distribution of regions. Meanwhile, we used the 95% quantile as the cutoff value for the candidate regions and each candidate region had at least 7 CpGs probes. In addition, the false positive rate was controlled by using the family-wise error rate (FWER) in consideration for its conservation compared with the BH and FDR methods. Third, we annotated all DMRs gene coordinates using wANNOVA.Citation24 Forth, Kyoto Encyclopedia of Genes and Genomes (KEGG) pathway enrichment analysis were carried out to understand the underlying pathways of DMRs, using “clusterProfiler” R packageCitation25 based on a threshold of P < .05.
Model development and validation
The methylation level of each DMR was expressed by the average β value of the probes contained in the DMR. MIBC patients were randomly divided into a training dataset (n = 319) and a validation dataset (n = 80). First, we used univariate cox regression to screen DMRs in training dataset and found 20 DMRs that were correlated with overall survival. Second, adaptive lasso penalty cox regression (ALasso)Citation26 which avoided the problem of over fitting was performed to filter the important DMRs where the λ value was obtained by a 10-fold cross validation. Third, the risk model was established according to the formula (β is the ALasso cox regression coefficient and DMRs is the methylation level of DMR). Forth, patients were divided into high and low risk groups using the optimal cutoff value of the risk score, which was determined by the X‐tile software (version 3.6.1, Yale University, CT). Meanwhile, we validated the risk model in the validation dataset using the same cutoff value. Fifth, we conducted a time-dependent receiver operating characteristic (ROC) curve to evaluate the predictive value of the five-year survival rate of the model using the survival ROC package.Citation27 In addition, total dataset was separated by gender and Z test was used to compare the predictive value of the risk model between genders. Finally, we plotted nomogram based on the model-based risk and the participants’ clinical characteristics. Kaplan-Meier and log-rank method were used to test the survival differences of different risk groups.
Results
Patient characteristics
A total of 399 patients were included in this study after preprocessing and they were randomly divided into training datasets (n = 319) and validation datasets (n = 80). The demographic and clinical characteristics of both datasets are listed in . The median survival time were 35 months in training dataset, 22 months in validation dataset, and 33 months for all patients combined, respectively. Age, gender, race and pathological grade were comparable between training dataset and validation dataset (P > .05). However, the distribution of participants were significantly different by pathological stage between two datasets, with more high stages being in validation dataset (P = .013).
Table 1. Characteristics of training dataset and validation dataset
Identification of differential methylation regions
In this study, 58,449 differentially methylated probes (DMPs) were identified from 169,730 probes according to the criteria of “P (Benjamini adjusted P value) < 0.05.” Bumphunter algorithm was performed to analyze these DMPs in 399 MIBC samples and 20 normal samples. A total of 490 DMRs had been found based on the criteria of FWER being less than 0.05 and the number of probes being more than 7. KEGG pathway analysis showed that these DMRs were mainly involved in cAMP signaling pathway, Calcium signaling pathway, and transcriptional misregulation in cancer. ().
Risk model construction
We first used univariate Cox regression to evaluate the association of DMRs with overall survival in the training dataset, which generated a total of 23 differential DMRs (Supplementary table). Next, we used 10-fold cross-validation adaptive lasso cox regression to screen out stable DMRs combinations from 23 DMRs, from which 11 DMRs were identified and annotated, namely, ARHGDIB, SHANK2, PROM1, RASGRF2, SEC31B, PXDNL, TRIM27, GPR75-ASB3, GALR1, PP12613, and NRN1 (Supplementary table). Furthermore, we applied the ALasso cox regression coefficient to build a risk model as followed,
And the risk score was calculated for each patient. In the training dataset, patients were categorized into high-risk group when a risk score was> −2.69 and into low-risk group when a risk score was ≤ −2.69, using the optimal cutoff value determined by X-lite software. A worse overall survival was observed for the high-risk group (HR = 2.58, 95% CI = (1.64, 4.05)) with reference to the low-risk group after multiple adjustment (). The survival probability was depicted in the Kaplan-Meier survival curve plot (), In addition, we used the time-dependent ROC curve to evaluate the prediction accuracy of risk model in the training dataset, and the calculated area under the ROC curve was 0.791().
Table 2. Survival analysis for risk module with OS as endpoints in the training dataset and validation dataset
Risk model validation
In order to determine the stability of the risk model, we used the validation dataset to evaluate the constructed risk model. Verification dataset were divided into two groups, that is, high risk (n = 50) and low risk (n = 30) according to the same cutoff values as defined in the training dataset. An unfavorable overall survival were observed for high-risk group before (HR = 2.87, 95% CI = (1.36, 6.06)) and after (HR = 2.77, 95% CI = (1.25, 6.15)) multiple adjustment in comparison with low-risk group (, ). The prediction accuracy of the risk model was assessed (AUC = 0.668, ).
Subgroup analyses
In order to observe the impact of gender on the prognosis of MIBC, we evaluated the relative risk of high-risk group compared with low-risk group for total dataset (HR = 2.56, 95% CI = (1.73, 3.78)), male dataset (HR = 2.72, 95% CI = (1.71, 4.34)), and female dataset (HR = 2.18, 95% CI = (1.07, 4.44)), respectively (data not shown). The prediction accuracy (AUC value) was basically similar for different groups (0.762 for total dataset, 0.775 for male, and 0.718 for female, P = .441, )
Nomogram construction based on risk model and clinical covariates
In the training dataset, we found that pathologic stage and risk models were associated with overall survival in both univariate and multivariate analyses. Therefore, we developed a five year survival probability nomogram based on risk model and pathological stage ().
Figure 4. Methylation signature nomogram to predict the risk of survival in patients with MIBC. (a) Nomogram of methylation signature predict the 5-years Survival probability of MIBC. (b) Calibration curve for the OS nomogram model in training dataset. (c) Calibration curve for the OS nomogram model in validation dataset
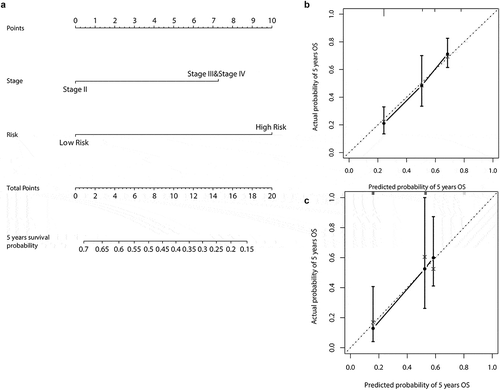
Discussion
The biological mechanism of bladder cancer has been widely studied for decades. Initially, most researches focused on the expression and biological function of a single gene.Citation28 With the introduction of high-throughput technology, researchers began to shift their attention to the association of DNA methylation with carcinogenesis.Citation29 Methylation is a dynamic chemical modification of DNA cytosine, which regulates biological development by inhibiting the transcription of genes.Citation30 Studies had shown that abnormal methylation of tumor suppressor gene and proto-oncogene promoter region played an important role in cancer occurrence and development.Citation11,Citation13,Citation31 In this study, we used publicly available data warehouses to perform genome-wide DNA methylation analysis at the gene region level and correlated it with clinical information to assess the relationship between aberrant methylation regions and the prognosis of MIBC.
In our study, we applied bumphunter method to compare methylation differences between tumor and normal samples and we identified 490 DMRs from a total of 58,449 DMPs. Further, univariate Cox regression was used to evaluate the relationship between each DMR and the prognosis of MIBC. In order to avoid collinearity and overfitting for our model, we therefore screened the DMRs that were strongly correlated with the prognosis of MIBC by performing the adaptive lasso method, an improvement of the traditional Lasso model for having overcome the limitation that the Oracle property was not satisfied.
Previous studies had explored the potential mechanism underlying the correlation between the identified single CpGs site with the unfavorable prognosis.Citation14,Citation15 We, here, identified a serial of DMRs which had been associated with carcinogenesis and cancer prognosis including ARHGDIB, SHANK2, PROM1, RASGRF2, TRIM27, GPR75-ASB3, GALR1, and NRN1. To date, direct evidences with carcinogenesis have not been reported for other DMRs, namely, SEC31B, PXDNL, and PP12613.
Among these markers identified, several had been related to the development of nervous system as well as nervous system tumorigenesis, recurrence, and prognosis. NRN1 (neuritin 1) encodes a protein of the neuritin family, which plays an important role in the development of the nervous system, the proliferation of stem cells, and the aging process. It had been shown that NRN1 played a key role in the occurrence and development of cancer by promoting the morphological changes of cells.Citation32,Citation33 PROM1 (prominin 1) maintains the properties of stem cells by inhibiting differentiation through encoding a pentaspan transmembrane glycoprotein. It was proved that the high expression of PROM1 was associated with the recurrence, metastasis and poor prognosis of nervous system tumors.Citation34,Citation35
GALR1 (galanin receptor 1), ARHGDIB (Rho GDP dissociation inhibitor beta) and SHANK2 (SH3 and multiple ankyrin repeat domains 2) has been reported to be associated with the prognosis of head and neck cancer, muscle-infiltrating bladder and prostate cancer, and oral squamous cell carcinoma, respectively. GALR1 exerts biological effects by interacting with specific G protein-coupled receptors. Studies have shown that aberrant methylation in the promoter region of GALR1 is associated with the occurrence, development, and poor prognosis of head and neck cancer. It may be that the hyper-methylation of GALR1 promoter region inhibits the expression of GALR1 mRNA, which leads to the loss of control of the expression of cyclin D1.Citation36 In addition, it has been reported that GALR1 affects the sensitivity of patients to chemotherapeutic drugs by participating in the mitogen-activated protein kinase (MAPK) signaling.Citation37 ARHGDIB is a member of Rho (or ARH) protein family, which is involved in cell signaling, proliferation, cytoskeletal organization, and secretion.Citation38 ARHGDIB was reported to prevent the metastasis of cancer by inhibiting the activation of Rho GTP enzyme.Citation39 Several studies from the United States have confirmed that the low expression of ARHGDIB is associated with metastasis and poor prognosis in muscle-infiltrating bladder and prostate cancer.Citation40–42 SHANK2 was reported to encode a synaptic protein in the Shank family that was involved in the development of the nervous system.Citation43 It has been also reported that SHANK2 promotes the invasion of cancer cells through synergistic action with cortactin, which is a protein involved in the cytoskeleton, and its high expression was related to the poor prognosis of oral cancer.Citation44,Citation45
Several other markers were previously observed to be relevant to tumorigenesis in different mechanisms. RASGRF2 (Ras protein specific guanine nucleotide releasing factor 2) encodes a calcium-regulated nucleotide exchange factor, which plays a key role in invasion, colonization, metastasis, and diffusion of tumor cells through the negative regulation of CDC42 signaling pathway.Citation46 TRIM27 (tripartite motif containing 27), a transcriptional inhibitor, mediates the regulation of thioredoxin binding protein 2 (TBP-2) by histone deacetylases (HDAC), which affects the susceptibility of cancer cells to oxidative stress and cisplatin.Citation47 GPR75-ASB3 and ABS3 encode the same protein by downregulating tumor necrosis factor R2 (TNF-R2) ubiquitin and degradation and affect tumor necrosis factor α (TNF-α) cell response and JNK signaling pathway, which is involved in the process of tumor cell proliferation, apoptosis, and metastasis.Citation48 To our knowledge, their association with cancer prognosis has not reported so far.
To date, SEC31B, PXDNL, and PP12613 methylation abnormality of promoter regions has not been reported to be related to carcinogenesis. We, among the first, observed in this study that aberrant methylation of SEC31B, PXDNL, and PP12613 promoter regions was associated with the prognosis of MIBC. Until recently, we know that SEC31B (SEC31 homolog B) encodes a protein of unknown function that mediates vesicular traffic from the endoplasmic reticulum, PXDNL (peroxidasin like) encodes a peroxidase that participates in basement membrane synthesis,Citation49 and PP12613 is a noncoding gene. The unknown mechanism underlying the process of carcinogenesis and pathological differentiation warrants to be further studies.
Compared with the study of Robertson et al, our study has the advantage of using multiple CpG sites over a single CpG site in the promoter region of the gene to better measure the association of the methylation level of that gene with MIBC survival. Meanwhile, we developed a new clinical survival risk prediction tool.Citation50 We also noted that Xie and colleagues had proposed a statistical method named GDP (Group lass regularized Deep learning for cancer Prognosis) which seems to be more efficient in some ways.Citation51 However, disadvantages existed for their method compared to adaptive lasso used in our study, such as, unsatisfied Oracle property, lack of clinical prior group knowledge and low sparsity within the group. We therefore constructed a new prognostic risk model based on gene methylation by used adaptive lasso method. Furthermore, some studies have shown that the prognosis of MIBC was different between genders and the worse prognosis was usually among women.Citation52 We therefore performed a gender-based subgroup analysis, and our results suggested that the risk model based on 11 DMRs was independent of gender. However, this study has its limitations. First, our data were abstracted from the public database, where treatment information was not available. Furthermore, lack of information about cancer recurrence prevents us from exploring the relationship between risk models and cancer recurrence. In addition, limited by the data acquisition, we only could verify the model internally, which limits the extrapolation of results.
Conclusion
We analyzed methylation data and clinical data from patients with MIBC. 11 abnormal methylation regions were identified and a prognostic risk model based on methylation markers was constructed and internally validated. Simultaneously, nomograms including methylated markers and clinical risk factors were established to predict the survival probability, which is believed to be valuable for the development of individualized treatment of MIBC.
Author contributions
Conception and design: Yueyi Feng, and Xiaochen Shu
Financial support: Xiaochen Shu
Collection and assembly of data: Yueyi Feng, Yiqing Jiang, Qingting Feng, Lingkai Xu, Yun Jiang
Data analysis and interpretation: Yueyi Feng, Yiqing Jiang, Qingting Feng, Lingkai Xu, Yun Jiang, Fang Meng, and Xiaochen Shu
Manuscript writing: Yueyi Feng, Yiqing Jiang, Fang Meng, and Xiaochen Shu
Final approval of manuscript: Yueyi Feng, Yiqing Jiang, Qingting Feng, Lingkai Xu, Yun Jiang, Fang Meng, and Xiaochen Shu
Disclosure of potential conflicts of interest
No potential conflicts of interest were disclosed.
Supplemental Material
Download MS Excel (13.6 KB)Acknowledgments
The corresponding author would be grateful for co-workers’ contributions including collecting, managing, maintaining, and analyzing data as well as writing and proofing the manuscript submitted.
Supplementary material
Supplemental data for this article can be accessed on the publisher’s website.
Additional information
Funding
References
- Sanli O, Dobruch J, Knowles MA, Burger M, Alemozaffar M, Nielsen ME, Lotan Y. 2017. Bladder cancer. Nat rev Dis Primers. 3:17022. doi:10.1038/nrdp.2017.22.
- Antoni S, Ferlay J, Soerjomataram I, Znaor A, Jemal A, Bray F. 2017. Bladder cancer incidence and mortality: A global overview and recent trends. Eur Urol. 71:96–108. doi:10.1016/j.eururo.2016.06.010.
- Burger M, Catto JW, Dalbagni G, Grossman HB, Herr H, Karakiewicz P, Kassouf W, Kiemeney LA, La Vecchia C, Shariat S, et al. 2013. Epidemiology and risk factors of urothelial bladder cancer. Eur Urol. 63:234–241. doi:10.1016/j.eururo.2012.07.033.
- Hautmann RE, Gschwend JE, de Petriconi RC, Kron M, Volkmer BG. Cystectomy for transitional cell carcinoma of the bladder: results of a surgery only series in the neobladder era. J Urol. 2006;176:486–492. discussion 491-2. doi:10.1016/j.juro.2006.03.038.
- Bellmunt J, Orsola A, Leow JJ, Wiegel T, De Santis M, Horwich A, Group EGW. 2014. Bladder cancer: ESMO practice guidelines for diagnosis, treatment and follow-up. Ann Oncol. 25(Suppl 3):iii40–8. doi:10.1093/annonc/mdu223.
- Von der Maase H, Hansen SW, Roberts JT, Dogliotti L, Oliver T, Moore MJ, Bodrogi I, Albers P, Knuth A, Lippert CM, et al. 2000. Gemcitabine and cisplatin versus methotrexate, vinblastine, doxorubicin, and cisplatin in advanced or metastatic bladder cancer: results of a large, randomized, multinational, multicenter, phase III study. J Clin Oncol. 18:3068–3077. doi:10.1200/JCO.2000.18.17.3068.
- Henrique R, Nunes SP, Jeronimo C. 2018. MSH2 expression and resistance to cisplatin in muscle-invasive bladder cancer: A mix of progress and challenges. Eur Urol. doi:10.1016/j.eururo.2018.11.014
- Berdik C. 2017. Unlocking bladder cancer. Nature. 551:S34–S35. doi:10.1038/551S34a.
- Moore LD, Le T, Fan G. 2013. DNA methylation and its basic function. Neuropsychopharmacology. 38:23–38. doi:10.1038/npp.2012.112.
- Arantes LM, de Carvalho AC, Melendez ME, Carvalho AL, Goloni-Bertollo EM. 2014. Methylation as a biomarker for head and neck cancer. Oral Oncol. 50:587–592. doi:10.1016/j.oraloncology.2014.02.015.
- Esteller M. 2008. Epigenetics in cancer. N Engl J Med. 358:1148–1159. doi:10.1056/NEJMra072067.
- Herman JG, Baylin SB. 2003. Gene silencing in cancer in association with promoter hypermethylation. N Engl J Med. 349:2042–2054. doi:10.1056/NEJMra023075.
- Ley TJ, Mardis ER, Ding L, Fulton B, McLellan MD, Chen K, Dooling D, Dunford-Shore BH, McGrath S, Hickenbotham M, et al. 2008. DNA sequencing of a cytogenetically normal acute myeloid leukaemia genome. Nature. 456:66–72. doi:10.1038/nature07485.
- Shivakumar M, Lee Y, Bang L, Garg T, Sohn KA, Kim D. 2017. Identification of epigenetic interactions between miRNA and DNA methylation associated with gene expression as potential prognostic markers in bladder cancer. BMC Med Genomics. 10:30. doi:10.1186/s12920-017-0269-y.
- Stubendorff B, Wilhelm K, Posselt K, Catto J, Hartmann A, Bertz S, Fussel S, Novotny V, Toma M, Gajda M, et al. 2019. A three-gene methylation marker panel for the nodal metastatic risk assessment of muscle-invasive bladder cancer. J Cancer Res Clin Oncol. doi:10.1007/s00432-018-02829-4.
- Lister R, Pelizzola M, Dowen RH, Hawkins RD, Hon G, Tonti-Filippini J, Nery JR, Lee L, Ye Z, Ngo QM, et al. 2009. Human DNA methylomes at base resolution show widespread epigenomic differences. Nature. 462:315–322. doi:10.1038/nature08514.
- Yu G, Wang LG, He QY. 2015. ChIPseeker: an R/Bioconductor package for ChIP peak annotation, comparison and visualization. Bioinformatics. 31:2382–2383. doi:10.1093/bioinformatics/btv145.
- Teschendorff AE, Marabita F, Lechner M, Bartlett T, Tegner J, Gomez-Cabrero D, Beck S. 2013. A beta-mixture quantile normalization method for correcting probe design bias in illumina infinium 450 k DNA methylation data. Bioinformatics. 29:189–196. doi:10.1093/bioinformatics/bts680.
- Morris TJ, Butcher LM, Feber A, Teschendorff AE, Chakravarthy AR, Wojdacz TK, Beck S. 2014. ChAMP: 450k chip analysis methylation pipeline. Bioinformatics. 30:428–430. doi:10.1093/bioinformatics/btt684.
- Johnson WE, Li C, Rabinovic A. 2007. Adjusting batch effects in microarray expression data using empirical Bayes methods. Biostatistics. 8:118–127. doi:10.1093/biostatistics/kxj037.
- Du P, Zhang X, Huang CC, Jafari N, Kibbe WA, Hou L, Lin SM. 2010. Comparison of beta-value and M-value methods for quantifying methylation levels by microarray analysis. BMC Bioinform. 11:587. doi:10.1186/1471-2105-11-587.
- Ritchie ME, Phipson B, Wu D, Hu Y, Law CW, Shi W, Smyth GK. 2015. limma powers differential expression analyses for RNA-sequencing and microarray studies. Nucleic Acids Res. 43:e47. doi:10.1093/nar/gkv007.
- Jaffe AE, Murakami P, Lee H, Leek JT, Fallin MD, Feinberg AP, Irizarry RA. 2012. Bump hunting to identify differentially methylated regions in epigenetic epidemiology studies. Int J Epidemiol. 41:200–209. doi:10.1093/ije/dyr238.
- Yang H, Wang K. 2015. Genomic variant annotation and prioritization with ANNOVAR and wANNOVAR. Nat Protoc. 10:1556–1566. doi:10.1038/nprot.2015.105.
- Yu GC, Wang LG, Han YY, He QY. 2012. clusterProfiler: an R package for comparing biological themes among gene clusters. Omics. 16:284–287. doi:10.1089/omi.2011.0118.
- Zhang HH, Lu WB. 2007. Adaptive lasso for Cox’s proportional hazards model. Biometrika. 94:691–703. doi:10.1093/biomet/asm037.
- Heagerty PJ, Lumley T, Pepe MS. 2000. Time-dependent ROC curves for censored survival data and a diagnostic marker. Biometrics. 56:337–344. doi:10.1111/j.0006-341X.2000.00337.x.
- Feng N, Wang Y, Zheng M, Yu X, Lin H, Ma RN, Shi O, Zheng X, Gao M, Yu H, et al. 2017. Genome-wide analysis of DNA methylation and their associations with long noncoding RNA/mRNA expression in non-small-cell lung cancer. Epigenomics. doi:10.2217/epi-2016-0120.
- Chan KC, Jiang P, Chan CW, Sun K, Wong J, Hui EP, Chan SL, Chan WC, Hui DS, Ng SS, et al. 2013. Noninvasive detection of cancer-associated genome-wide hypomethylation and copy number aberrations by plasma DNA bisulfite sequencing. Proc Natl Acad Sci U S A. 110:18761–18768. doi:10.1073/pnas.1313995110.
- Ambrosi C, Manzo M, Baubec T. 2017. Dynamics and context-dependent roles of DNA methylation. J Mol Biol. 429:1459–1475. doi:10.1016/j.jmb.2017.02.008.
- Liu S, Hausmann S, Carlson SM, Fuentes ME, Francis JW, Pillai R, Lofgren SM, Hulea L, Tandoc K, Lu J, et al. 2018. METTL13 methylation of eEF1A increases translational output to promote tumorigenesis. Cell. doi:10.1016/j.cell.2018.11.038.
- Naeve GS, Ramakrishnan M, Kramer R, Hevroni D, Citri Y, Theill LE. Neuritin: a gene induced by neural activity and neurotrophins that promotes neuritogenesis. Proc Natl Acad Sci U S A. 1997;94:2648–2653. doi:10.1073/pnas.94.6.2648.
- Le Jan S, Le Meur N, Cazes A, Philippe J, Le Cunff M, Leger J, Corvol P and Germain S. 2006. Characterization of the expression of the hypoxia-induced genes neuritin, TXNIP and IGFBP3 in cancer. FEBS Lett. 580:3395–3400. doi:10.1016/j.febslet.2006.05.011.
- Raso A, Mascelli S, Biassoni R, Nozza P, Kool M, Pistorio A, Ugolotti E, Milanaccio C, Pignatelli S, Ferraro M, et al. 2011. High levels of PROM1 (CD133) transcript are a potential predictor of poor prognosis in medulloblastoma. Neuro-oncology. 13:500–508. doi:10.1093/neuonc/nor022.
- Zhang W, Chen H, Lv S, Yang H. 2016. High CD133 expression is associated with worse prognosis in patients with glioblastoma. Mol Neurobiol. 53:2354–2360. doi:10.1007/s12035-015-9187-1.
- Misawa K, Ueda Y, Kanazawa T, Misawa Y, Jang I, Brenner JC, Ogawa T, Takebayashi S, Grenman RA, Herman JG, et al. 2008. Epigenetic inactivation of galanin receptor 1 in head and neck cancer. Clin Cancer Res. 14:7604–7613. doi:10.1158/1078-0432.CCR-07-4673.
- Stevenson L, Allen WL, Turkington R, Jithesh PV, Proutski I, Stewart G, Lenz HJ, Van Schaeybroeck S, Longley DB, Johnston PG. 2012. Identification of galanin and its receptor GalR1 as novel determinants of resistance to chemotherapy and potential biomarkers in colorectal cancer. Clin Cancer Res. 18:5412–5426. doi:10.1158/1078-0432.CCR-12-1780.
- Fagerberg L, Hallstrom BM, Oksvold P, Kampf C, Djureinovic D, Odeberg J, Habuka M, Tahmasebpoor S, Danielsson A, Edlund K, et al. 2014. Analysis of the human tissue-specific expression by genome-wide integration of transcriptomics and antibody-based proteomics. Mol Cell Proteomics. 13:397–406. doi:10.1074/mcp.M113.035600.
- Moissoglu K, McRoberts KS, Meier JA, Theodorescu D and Schwartz M A. 2009. Rho GDP dissociation inhibitor 2 suppresses metastasis via unconventional regulation of RhoGTPases. Cancer Res. 69:2838–2844. doi:10.1158/0008-5472.CAN-08-1397.
- Theodorescu D, Sapinoso LM, Conaway MR, Oxford G, Hampton GM, Frierson HF Jr. 2004. Reduced expression of metastasis suppressor RhoGDI2 is associated with decreased survival for patients with bladder cancer. Clin Cancer Res. 10:3800–3806. doi:10.1158/1078-0432.CCR-03-0653.
- Said N, Sanchez-Carbayo M, Smith SC, Theodorescu D. 2012. RhoGDI2 suppresses lung metastasis in mice by reducing tumor versican expression and macrophage infiltration. J Clin Invest. 122:1503–1518. doi:10.1172/JCI61392.
- Gildea JJ, Seraj MJ, Oxford G, Harding MA, Hampton GM, Moskaluk CA, Frierson HF, Conaway MR, Theodorescu D. RhoGDI2 is an invasion and metastasis suppressor gene in human cancer. Cancer Res. 2002;62:6418–6423.
- Peykov S, Berkel S, Schoen M, Weiss K, Degenhardt F, Strohmaier J, Weiss B, Proepper C, Schratt G, Nothen MM, et al. 2015. Identification and functional characterization of rare SHANK2 variants in schizophrenia. Mol Psychiatry. 20:1489–1498. doi:10.1038/mp.2014.172.
- Barros-Filho MC, Reis-Rosa LA, Hatakeyama M, Marchi FA, Chulam T, Scapulatempo-Neto C, Nicolau UR, Carvalho AL, Pinto CAL, Drigo SA, et al. 2018. Oncogenic drivers in 11q13 associated with prognosis and response to therapy in advanced oropharyngeal carcinomas. Oral Oncol. 83:81–90. doi:10.1016/j.oraloncology.2018.06.010.
- Freier K, Sticht C, Hofele C, Flechtenmacher C, Stange D, Puccio L, Toedt G, Radlwimmer B, Lichter P, Joos S. 2006. Recurrent coamplification of cytoskeleton-associated genes EMS1 and SHANK2 with CCND1 in oral squamous cell carcinoma. Genes Chromosomes Cancer. 45:118–125. doi:10.1002/gcc.20270.
- Calvo F, Sanz-Moreno V, Agudo-Ibanez L, Wallberg F, Sahai E, Marshall CJ, Crespo P. 2011. RasGRF suppresses Cdc42-mediated tumour cell movement, cytoskeletal dynamics and transformation. Nat Cell Biol. 13:819–826. doi:10.1038/ncb2271.
- Kato T, Shimono Y, Hasegawa M, Jijiwa M, Enomoto A, Asai N, Murakumo Y and Takahashi M. 2009. Characterization of the HDAC1 complex that regulates the sensitivity of cancer cells to oxidative stress. Cancer Res. 69:3597–3604. doi:10.1158/0008-5472.CAN-08-4368.
- Chung AS, Guan YJ, Yuan ZL, Albina JE, Chin YE. 2005. Ankyrin repeat and SOCS box 3 (ASB3) mediates ubiquitination and degradation of tumor necrosis factor receptor II. Mol Cell Biol. 25:4716–4726. doi:10.1128/MCB.25.11.4716-4726.2005.
- Peterfi Z, Geiszt M. 2014. Peroxidasins: novel players in tissue genesis. Trends Biochem Sci. 39:305–307. doi:10.1016/j.tibs.2014.05.005.
- Robertson AG, Kim J, Al-Ahmadie H, Bellmunt J, Guo G, Cherniack AD, Hinoue T, Laird PW, Hoadley KA, Akbani R, et al. 2017. Comprehensive molecular characterization of muscle-invasive bladder cancer. Cell. 171:540–556 e25. doi:10.1016/j.cell.2017.09.007.
- Xie G, Dong C, Kong Y, Zhong JF, Li M, Wang K. 2019. Group lasso regularized deep learning for cancer prognosis from multi-omics and clinical features. Genes (Basel). 10. doi:10.3390/genes10030240.
- Dobruch J, Daneshmand S, Fisch M, Lotan Y, Noon AP, Resnick MJ, Shariat SF, Zlotta AR, Boorjian SA. 2016. Gender and bladder cancer: A collaborative review of etiology, biology, and outcomes. Eur Urol. 69:300–310. doi:10.1016/j.eururo.2015.08.037.