ABSTRACT
Circulating tumor DNA (ctDNA) is utilized for molecular profiling of cancers, and is under investigation for a growing number of applications based on the assumption that ctDNA levels faithfully reflect disease burden. Our objective was to investigate whether patient and tumor characteristics may impact ctDNA detection or levels and the prognostic significance of ctDNA levels or mutations. We performed a retrospective cohort analysis of a comprehensively annotated cohort of 561 patients at a National Cancer Institute–designated comprehensive cancer center with advanced solid cancers who underwent ctDNA testing using a commercial targeted next-generation sequencing assay. ctDNA detection in advanced cancers was associated with older age, non-obese body mass index, and diabetes, but not with tumor diameter, volume, lesion number, or other pathological features. Regression models indicate that no more than 14.3% of the variance in ctDNA levels between patients was explained by known clinical factors and disease burden. Even after adjusting for established prognostic factors and tumor burden, ctDNA levels were associated with worse survival among patients without prior systemic therapy, while ctDNA mutations were associated with survival among patients who previously received systemic treatment. These findings uncover clinical factors that affect ctDNA detection in patients with advanced cancers and challenge the convention that ctDNA is a surrogate for tumor burden. Our study also indicates that the prognostic value of ctDNA levels and mutations are independent of tumor burden and dependent on treatment context.
Introduction
Cell-free DNA (cfDNA) shed from cancer cells termed circulating tumor DNA (ctDNA) are detectable in liquid biopsies from patients with early and advanced-stage cancers using various technologies.Citation1–4 Early observations demonstrating that ctDNA declines after surgical treatment and are detectable at a higher frequency among advanced-stage cancers than early-stage cancers supported a close association between ctDNA and tumor burden, with some studies even indicating a linear relationship.Citation1,Citation2,Citation5–12 Accordingly, ctDNA has been employed as a surrogate for tumor burden and liquid biopsies are currently under investigation as early detection, pharmacodynamic, and minimal residual disease markers.Citation8,Citation13–19 However, large-scale studies across many cancer types show that 10–40% of late-stage cancers have no detectable ctDNA.Citation1,Citation5,Citation6,Citation12,Citation20–23 Different methodologies for ctDNA detection used in these studies and evidence that ctDNA can capture the genetic heterogeneity of metastatic cancers including subclonal mutations not apparent from single-site tissue biopsies suggest that undetectable ctDNA levels cannot be wholly attributed to sampling bias or pervasive deficiencies in any one ctDNA assay.Citation12,Citation24–26 Although ctDNA biology remains poorly understood, the abundance of ctDNA is determined by mechanisms governing its production, release into circulation, stability in blood, and clearance.Citation27 Thus, other factors beyond tumor mass or cell divisions are likely to be important determinants of ctDNA detectability. This is supported by evidence that cfDNA concentrations are influenced by various aspects of human biology and disease including demographics, chronic comorbidities, inflammatory states, and tissue injury.Citation28–32
We hypothesized that patient and tumor characteristics may influence ctDNA detection across cancer types. Elucidation of such determinants would be critical to understanding the limitations of liquid biopsies for individual patients and guide the interpretation of ctDNA analyses for an increasing number of clinical applications. Herein, we examined a comprehensively annotated cohort of 561 patients with advanced cancers who underwent liquid biopsies analyzed by targeted next-generation sequencing.
Patients and Methods
Patient data and samples
This retrospective cohort analysis included consecutive eligible patients seen at the University of Texas Southwestern Harold C. Simmons Comprehensive Cancer Center and Parkland Health & Hospital System, who had liquid biopsies analyzed using the Guardant360 CDx assay. Eligible patients included all individuals with either unresectable or metastatic solid cancers. Collection of liquid biopsies was performed as part of routine clinical care. As such, liquid biopsies were generally performed in place of molecular profiling of tumor tissue for most patients. Hematology and blood chemistry test values were only abstracted if laboratory tests were drawn the same day as the liquid biopsy. Acute phase reactants and serum tumor markers were abstracted if laboratory tests were drawn within 2 weeks of the liquid biopsy. Characteristics of tumor pathology were abstracted from pre-systemic treatment biopsy or resection specimens. Disease site and tumor burden data were abstracted from radiographic images including computed tomography and magnetic resonance imaging performed within 4 weeks of the liquid biopsy. The sum of all diameters was the aggregate of the longest diameter on either the axial, sagittal, or coronal plane of all lesions. To calculate tumor volumes, 3-deminsional measurements for each lesion were used to calculate the volume of an ellipsoid (4/3 x π x width x length x height) which has been shown to approximate tumor volumes.Citation33,Citation34 This study was performed in accordance with Good Clinical Practices and the Declaration of Helsinki and approved by the University of Texas Southwestern Institutional Review Board.
Liquid biopsy and ctDNA sequencing
Liquid biopsies were collected according to the Guardant360 Clinical Blood Collection Kit instructions (Guardant Health, Inc.).Citation9,Citation35 As previously described, the Guardant360 CDx assay is a targeted high throughput hybridization-based capture technology for the detection of single nucleotide variants, insertions, and deletions in 73 genes by paired-end synthesis-sequencing using the NextSeq 500 and/or HiSeq 2500 platforms (Illumina, Inc.).Citation9,Citation35 The complete list of genes and variants analyzed by the assay is viewable under the GTR Test ID GTR000527948.6. Putative germline mutations including variants identified by allele fractions between 40% and 60%, prior annotation as germline mutations, and manual review as indicated in clinical reports were excluded from our analysis.
Statistical analysis
Odds ratio for undetectable ctDNA was assessed by univariable logistic regression for all variables and multivariable logistic regression for variables with significant associations in univariable analysis. Linear regression using the ordinary least squares method was used to assess the coefficient of determination of univariable and multivariable models. Overall survival was determined from the date of the initial liquid biopsy to the time of death or most recent follow-up. Kaplan–Meier survival curves were compared using log-rank tests. Unadjusted and adjusted hazard ratios were determined using Cox regression models. The proportional hazard assumption was tested by including a time-dependent covariate in regression models and results were only reported if the covariate was not significantly associated with the outcome, indicating that the assumption was not violated. SPSS Statistics 23 (IBM) and GraphPad Prism 8 were used for statistical analysis.
Results
Identifying factors associated with undetectable ctDNA
Among 561 patients with unresectable or metastatic solid cancers who underwent liquid biopsies and NGS of ctDNA using the Guardant360 CDx assay, the median age was 68 years (interquartile range 60–75), 54.2% were male, and 42.2% had newly diagnosed disease (). We defined undetectable ctDNA as the absence of any somatic mutation by liquid biopsy testing, and found that 70 patients (12% of the entire cohort) had undetectable ctDNA, which is comparable to rates reported in prior studies.
Table 1. Characteristics of patients with ctDNA testing
For each patient, a comprehensive panel of clinical, tumor, and treatment characteristics including demographics, comorbidities, hematology and chemistry lab tests, pathological characteristics, disease sites, tumor measurements, and treatment history was curated (). Variables were systematically tested in univariable logistic regression analyses, which showed that age, history of smoking, diabetes, metastatic disease, bone disease, number of organs involved, diameter of the largest and all lesions, and progression of disease at the time of liquid biopsy were associated with ctDNA detection (). In contrast, obesity was significantly associated with undetectable ctDNA (odds ratio, 3.46; p < .01). A multivariable analysis including cancer type as a covariate revealed that only clinical factors, namely age, obesity, and diabetes, remained statistically significant predictors of ctDNA status. No multicollinearity was detected among the aforementioned variables related to tumor burden including the number of organs involved, diameter of the largest lesion, diameters of all lesions, and number of lesions based on the determination of variance inflation factors using linear regression models (all factors being less than 4).Citation36 Identical univariable and multivariable analyses were performed after excluding synonymous mutations or variants of unknown significance, which confirmed that age was associated with ctDNA detection, while obesity was associated with undetectable ctDNA. Given the possibility that prior treatment may impact ctDNA detection, we conducted logistic regression analysis of patients who had ctDNA collected prior to any systemic therapy, which similarly showed that obesity was negatively associated with ctDNA detectability (odds ratio, 2.52; p = .03), while age or tumor burden parameters were not significantly associated in univariable or multivariable analyses.
Figure 1. Patient and tumor characteristics associated with ctDNA detection. Variables relating to clinical factors, tumor biology, and treatment history were abstracted for 561 patients with advanced stage cancers to assess for predictors of ctDNA detection using the Guardant360 CDx assay. The odd ratios (OR) of undetectable ctDNA were determined using univariable and multivariable logistic regression analyses
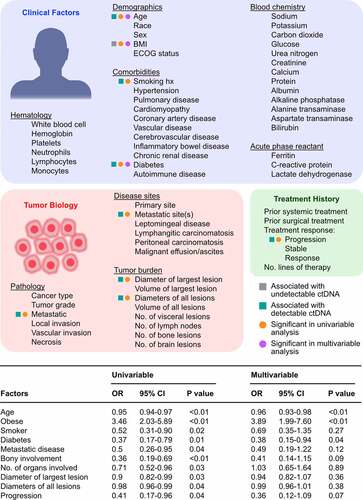
Among the most frequently mutated genes linked to clonal hematopoiesis of the indeterminate potential (CHIP) including DNMT3A, TET2, TP53, and CEBPA, only TP53 is assessed in the Guardant360 CDx assay. We repeated our regression analysis after excluding 20 patients who only had ctDNA mutations in TP53 and no other genes. Multivariable analysis showed that age (odds ratio, 0.96; p < .01), obesity (odds ratio, 3.61; p < .01), and diabetes (odds ratio, 0.41; p = .03) remained significant predictors of undetectable ctDNA.
Examining the relationship between ctDNA levels and tumor burden
The lack of an association between tumor characteristics and ctDNA detection in our cohort led us to evaluate the relationship between ctDNA levels and tumor characteristics as pathologic factors including disease burden have been associated with ctDNA abundance.Citation1,Citation5,Citation6,Citation25–27,Citation37 We quantitated ctDNA levels using three methods that have been applied in past studies including the maximal variant allele frequency (VAF) of any somatic mutation, the mean VAF of all somatic mutations, and the sum of VAF of somatic mutations.Citation6,Citation38–43 We assessed the relationship between ctDNA levels and either the sum of the largest diameters for all lesions, the standard measurement for gauging responses in clinical practice, and the volume of all lesions, a metric which provides a more faithful reflection of disease burden. While there was a positive correlation between maximal VAF levels with the diameters (Pearson r, 0.38; p < .01) or volume (Pearson r, 0.30; p < .01) of all lesions, this relationship was associated with a large degree of scatter as observed in bivariate plots (). Consistent with a limited association between ctDNA levels and tumor burden, univariable linear regression analyses demonstrated that the explained variance of ctDNA levels by the diameter or volume of all lesions was only 14.3% and 9.3%, respectively. The explained variance of ctDNA levels calculated as the mean VAF or sum of VAF by the diameters or volume of all lesions was also poor (Supplementary Figure 1). In addition, the association between ctDNA levels and tumor burden was limited across cancer types with the coefficient of determinations ranging from 6.5% for lung cancers to 21.9% for pancreatic cancers (Supplementary Figure 2). Notably, the explained variance of ctDNA levels was even lower, ranging from 0% to 7% for other parameters of disease burden including the number of lesions, number of nodal metastases, and the diameter and volume of the dominant lesion (Supplementary Figure 3).
Figure 2. Pathologic characteristics and tumor burden are poorly associated with ctDNA levels. (a) Scatter plots depict the relationship between ctDNA levels and tumor burden defined as the diameters of all lesions or the volume of all lesions. The gray line depicts the best-fitting line. The inset shows the same plots with axes in log scale to demonstrate the degree of scattering across ctDNA values and tumor burden measurements. (b) Multivariable linear regression analyses adjusting for cancer type were used to assess the effects of variables with significant associations in univariable linear regressions on ctDNA abundance defined as maximal VAF, mean VAF, or sum of VAFs. Standardized coefficients denoting the fraction of variance explained by each model is shown the bar chart
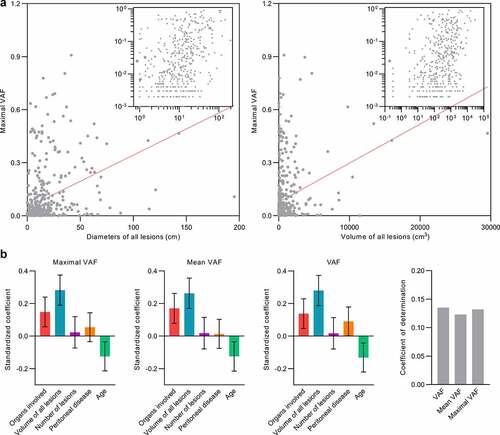
We used multivariable linear regression analysis to assess independent associations between ctDNA level and different tumor burden parameters that were significant predictors in univariable analyses including the number of organs involved, the volume of all lesions, the number of lesions, and the presence of peritoneal disease (). Given the collinearity detected between the diameters and volume of all lesions, we chose to include only the volume of all lesions as a covariate in multivariable models as it is a more accurate estimate of tumor mass. Among predictors of ctDNA detection, age but not BMI or diabetes was significantly associated with ctDNA levels in univariable analyses, and therefore age was also included in the final model. Multivariable regression analysis adjusting for cancer type demonstrated that the number of organs involved and volume of all lesions remained positively associated with ctDNA levels, while the opposite was observed for age (). Similar results were seen when ctDNA was calculated as the mean or maximal VAF (). However, the coefficient of determination for any model never exceeded 14.5%, indicating that the variance of ctDNA levels across patients is poorly explained by known clinical factors or tumor biology (). There were also no statistically significant correlations detected between ctDNA levels and acute phase reactants and tumor markers with the exception of prostate-specific antigen (r = 0.29, p = .04)(Supplementary Figure 4).
In our cohort, six patients had three serial liquid biopsies obtained throughout their treatments, which allowed us to assess intraindividual variation in ctDNA levels and tumor burden longitudinally. In three patients, ctDNA kinetics largely paralleled changes in tumor burden ( and Supplementary Fig. 5). In contrast, three patients demonstrated discordant trends in ctDNA levels and tumor burden ( and Supplementary Fig. 5).
Figure 3. Longitudinal analysis of intraindividual relationships between ctDNA and tumor burden. Kinetics in ctDNA levels and tumor volumes for patients who had serial liquid biopsies. Representative axial slices from computed tomography scans are shown
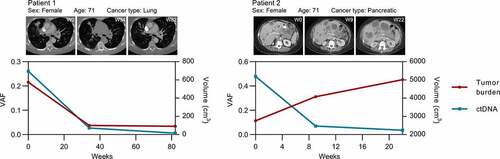
ctDNA levels are independent prognostic biomarkers
There are conflicting data regarding the prognostic relevance of ctDNA detectability, and whether the abundance or number of ctDNA mutations is independently associated with survival.Citation44–48 In addition, prior studies have not assessed whether ctDNA is a prognostic biomarker after accounting for tumor burden. Using the Kaplan–Meier analysis and log-rank test, we found that patients with undetectable ctDNA had a median overall survival of 68.4 months versus 15.6 months for patients with detectable ctDNA (chi-square, 11.1; p < .001) (). When patients with detectable ctDNA were segregated by ctDNA levels greater or less than the median maximal VAF value, patients with ctDNA levels less than the median were noted to have longer overall survival (21.7 versus 10.8 months; chi square, 24.5; p < .001) (Supplementary Fig. 6A). Patients with mean VAF or the sum of all VAFs less than the median were similarly associated with longer overall survival (Supplementary Fig. 6B and 6 C). We also segregated patients into quartiles by ctDNA levels, which showed that overall survival was shortest for patients in the third (11 months) and fourth quartiles (10.5 months), followed by patients in the second quartile (18.6 months), and then patients in the first quartile (35.4 months) ().
Figure 4. ctDNA is an independent prognostic biomarker in advanced cancers. (a) Kaplan-Meier survival curves for patients with detectable and undetectable ctDNA. The median overall survival for patients with detectable and undetectable ctDNA was 15.6 and 68.4 months, respectively. p < .001 by the Log-rank test. (b) Kaplan-Meier survival curves for patients stratified by ctDNA level quartiles. The median overall survival for patients in the first, second, third, and fourth quartiles was 11, 10.5, 18.6 and 35.4 months, respectively. p < .001 by the Log-rank test. (c) Kaplan-Meier survival curves for patients stratified by the number of gene mutations. The median overall survival for patients with 1, 2, 3–5, and >5 mutations was 48.2, 20.8, 11.1 and 11.1 months, respectively. p < .001 by the Log-rank test. (d) Kaplan-Meier survival curves for patients stratified by tumor volume quartiles. The median overall survival for patients in the first, second, third, and fourth quartiles was 11, 10.5, 18.6 and 35.4 months, respectively. p < .001 by the Log-rank test. (e) Adjusted hazard ratios (HRs) of death with 95% confidence intervals for patients stratified by systemic treatment exposure was determined by multivariable Cox regression models adjusted for cancer type. Models include all variables listed as factors
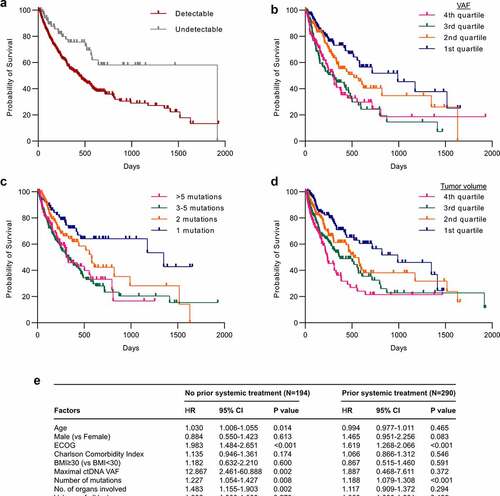
Among patients with detectable ctDNA, a higher ctDNA mutation count (stratified by the median or quartiles) was associated with worse survival ( and Supplementary Fig. 6D).
Greater tumor volumes (stratified by the median or quartiles) were also associated with worse survival ( and Supplementary Fig. 6E). We used multivariable Cox regression models to determine whether ctDNA levels, mutation number, and tumor volumes were independent prognostic factors. Patients were initially stratified by whether they had received prior systemic treatment to assess whether the timing of ctDNA collection at baseline or after treatment exposure would impact its prognostic value. Additional variables included in multivariable Cox regression models included factors significantly associated with an increased risk of death in univariable analyses including age, male sex, ECOG score, Charlson Comorbidity Index, number of organs involved, and number of lesions as these. In multivariable analyses adjusting for cancer type among systemic treatment naïve patients, increasing age, ECOG score, ctDNA levels, number of ctDNA mutations, and number of organs involved were significantly associated with an increased risk of death (). Among patients previously treated with systemic therapies, only ECOG score, and number of ctDNA mutations, but not ctDNA levels, were associated with overall survival (). While small sample sizes limited the statistical power of analyses of individual cancer types, we note that similar findings were observed in multivariable Cox regression analyses of patients with non-small cell lung cancer and other cancer types (Supplementary Fig. 7).
Discussion
Elucidating clinical and pathologic factors that impact ctDNA is crucial to assessing the clinical validity and utility of ctDNA as a biomarker. Herein, we systematically interrogated a large and comprehensively annotated cohort of patients with advanced cancers who underwent ctDNA analysis using Guardant360 CDx, a highly sensitive and clinically validated assay.Citation35
Our analysis indicates that patient characteristics including age, obesity, and diabetes rather than tumor biology are key determinants of ctDNA detectability. While the precise mechanisms of these associations remain to be elucidated, host factors may impact the distribution, stability, and metabolism of ctDNA.Citation27 This is supported by prior findings demonstrating the impact of maternal health on fetal cfDNA detection and variation in cfDNA levels as a result of changes in physiology and diseased states.Citation28–32,Citation49–54 In fact, multiple studies have shown that maternal weight and age are negatively associated with fetal cfDNA detection, and these prior findings are akin to our results.Citation52–54 Our analysis does not exclude technical causes of undetectable ctDNA given constraints on sensitivity for any ctDNA assay including error rates of DNA polymerase for polymerase chain reaction-based methods and sequencing errors in NGS.Citation55 In addition, it is unclear whether patients with undetectable ctDNA using Guardant360 CDx includes those with ctDNA levels below the threshold of detection or those with a complete absence of ctDNA.
The limited association between ctDNA levels and parameters encompassing different facets of cancer growth in our patient cohort calls into question the widely held assumption that ctDNA reflects tumor burden.Citation2,Citation6,Citation7,Citation10,Citation19,Citation56 This discrepancy may be in part attributed to the smaller sample sizes of prior studies and methodological differences in ctDNA quantification, but also alludes to additional undefined or poorly appreciated patient or tumor characteristics that regulate ctDNA. Despite our efforts to capture major characteristics of tumor biology, not every aspect could be accounted for in our dataset. For instance, while the generation of ctDNA has been largely attributed to cell turnover, ctDNA may be shed by non-passive processes including release through exosomes, which are not readily detected by the Guardant360 CDx assay.Citation27,Citation55,Citation57 It is also unknown whether ctDNA is derived from all subclones, or if certain cells within the tumor hierarchy or within geographic locales such as invasive edges may contribute disproportionately to ctDNA levels.Citation27 This brings into the question whether subgroups of cancers with inflammatory phenotypes such as a high number of infiltrating lymphocytes or the tumor microenvironment may influence ctDNA levels. In addition, the abundance of specific ctDNA fragments may be influenced by molecular characteristics of cancer cells as the representation of the genome by cfDNA is dictated by nucleosome occupation which is in turn regulated by transcriptional and epigenetic processes.Citation58,Citation59 Our longitudinal analysis of ctDNA in six patients with at least three serial measurements to allow correlative analyses with tumor measurements should only be considered exploratory, but nonetheless supports our primary findings that ctDNA does not strictly reflect tumor burden.
Our study indicates that the prognostic values of ctDNA levels and mutations are independent of tumor burden and context dependent. Specifically, ctDNA levels are only prognostic when collected at baseline, while ctDNA mutation counts are prognostic even after prior exposure to systemic treatment. The mechanism underlying the association of ctDNA mutation counts with survival in treated patients remains unclear, but may be explained by the fact that treatment induced selection of subclones may lead to greater genetic heterogeneity. Inconsistent results in past studies describing the prognostic value of ctDNA or lack thereof may be attributed to varying sample sizes, adjustment for a limited number of confounding variables, and other methodological differences.Citation23,Citation26,Citation37,Citation38,Citation44–48 It is unclear if the association between ctDNA and survival is correlative or causative, but it is possible that ctDNA has functional effects based on evidence that cfDNA from various sources are ligands for toll-like receptors and may be internalized by cells.Citation60–62 Given that tumor mutation burden may be associated with cancer prognosis and impact ctDNA, it remains to be investigated whether tumor mutation burden contributes to the prognostic value of ctDNA mutations and abundance. Whether this type of treatment also affects the prognostic value of ctDNA is also unknown, although prior studies including patients who received chemotherapies, targeted therapies, or immunotherapies similarly showed that baseline ctDNA levels are prognostic.Citation15,Citation37,Citation63
Strengths of our analysis include its large sample size, which exceeds that of many prior studies, comprehensive clinical annotations, and the inclusion of major solid cancer types. In addition, we provide a thorough characterization of tumor biology including precise volumetric measurements rather than just lesion diameters as assessed in past studies. While our study incorporates a large panel of clinical and biological factors, missing data for a few variables such as race may limit the power to detect significant associations. Other limitations of our study includes the lack of a comparison of ctDNA to matched molecular profiling of tissue specimens, which is due to the fact that in nearly all instances liquid biopsies were performed in place of tissue biopsies. Thus, a lack of detectable ctDNA could be attributed to scarce mutations in the tumor itself or mutations not captured in the gene panel analyzed by the Guardant360 CDx assay. However, several studies including a recent rapid autopsy study comparing multi-site tissue and ctDNA sequencing indicate that cfDNA analysis may sensitively capture all if not most clonal mutations in patients and may even be superior to single biopsies in the detection of driver gene mutations.Citation64
Given the association between age and CHIP, it is expected that a non-trivial fraction of patients in our cohort who are predominantly older aged will have CHIP and CHIP mutations may thus confound ctDNA analyses. However, a couple of factors mitigate the contribution of CHIP in our analyses including the fact that the most common genes associated with CHIP including DNMT3A, TET2, ASXL1, PPM1D, SF3B1, ASXL2, GNB1, CBL, and SRSF2 are not assessed in the Guardant360 CDx assay.Citation65,Citation66 Nonetheless, several genes with mutations associated with CHIP including TP53, JAK2, IDH1/2, and GNAS are assessed in the Guardant360 CDx assay. A sensitivity analysis was conducted by excluding cases who only had mutations in TP53 detected in ctDNA because there were no cases where JAK2, IDH1/2, and GNAS mutations were the only mutations detected in ctDNA, and we found no differences in the subsequent results from our primary analysis. It should also be noted that a pan-cancer analysis of 10,719 patients across 30 solid cancer types in The Cancer Genome Atlas shows a substantially increased frequency of TP53, IDH1/2, and GNAS mutations in solid cancers than in CHIP, corroborating the likely nominal contribution of CHIP mutations to our analyses.Citation65,Citation67
Another limitation of this study is the number of genes analyzed by the Guardant360 CDx assay, and it remains to be investigated whether a larger gene panel with equivalent depth of sequencing coverage may lead to similar results. In addition, somatic mutations in nonmalignant cells have now been observed in an increasing number of tissue types, and whether these may be another source of mutations in cfDNA remains to be clarified.Citation68
Author contributions
D.H. conceived the project, collected data, performed data analyses, and wrote the manuscript. D.E.G., and M.S.B contributed patient data. M.E., D.E.G., and M.S.B participated in data interpretation and contributed to the final editing and approval of the manuscript.
Supplemental Material
Download MS Word (2.2 MB)Acknowledgments
We thank Dr. Hao Zhu for the discussions and review of the manuscript.
Disclosure statement
D.E.G. reports advisory or consulting roles for Bristol-Myers Squibb, Karyopharm, and Catalyst and receives research funding from AstraZeneca, BerGenBio, Karyopharm, and 3V Biosciences. D.H., M.E., and M.S.B. declare no competing interests.
Supplementary material
Supplemental data for this article can be accessed on the publisher’s website
Additional information
Funding
References
- Bettegowda C, Sausen M, Leary RJ, Kinde I, Wang Y, Agrawal N, Bartlett BR, Wang H, Luber B, Alani RM, et al. Detection of circulating tumor DNA in early- and late-stage human malignancies. Sci Transl Med. 2014;6(224):224ra24. doi:10.1126/scitranslmed.3007094.
- Diehl F, Schmidt K, Choti MA, Romans K, Goodman S, Li M, Thornton K, Agrawal N, Sokoll L, Szabo SA, et al. Circulating mutant DNA to assess tumor dynamics. Nat Med. 2008;14(9):985–990. doi:10.1038/nm.1789.
- Forshew T, Murtaza M, Parkinson C, Gale D, Tsui DW, Kaper F, Dawson S-J, Piskorz AM, Jimenez-Linan M, Bentley D, et al. Noninvasive identification and monitoring of cancer mutations by targeted deep sequencing of plasma DNA. Sci Transl Med. 2012;4(136):136ra68. doi:10.1126/scitranslmed.3003726.
- Diehl F, Li M, Dressman D, He Y, Shen D, Szabo S, Diaz LA, Goodman SN, David KA, Juhl H, et al. Detection and quantification of mutations in the plasma of patients with colorectal tumors. Proc Natl Acad Sci U S A. 2005;102(45):16368–16373. doi:10.1073/pnas.0507904102.
- Magbanua MJM, Swigart LB, Wu HT, Hirst GL, Yau C, Wolf DM, Tin A, Salari R, Shchegrova S, Pawar H, et al. Circulating tumor DNA in neoadjuvant-treated breast cancer reflects response and survival. Ann Oncol. 2021;32(2):229–239. 10.1016/j.annonc.2020.11.007
- Abbosh C, Birkbak NJ, Wilson GA, Jamal-Hanjani M, Constantin T, Salari R, Le Quesne J, Moore DA, Veeriah S, Rosenthal R, et al. Phylogenetic ctDNA analysis depicts early-stage lung cancer evolution. Nature. 2017;545(7655):446–451. doi:10.1038/nature22364.
- Sanmamed MF, Fernandez-Landazuri S, Rodriguez C, Zarate R, Lozano MD, Zubiri L, Perez-Gracia JL, Martín-Algarra S, González A. Quantitative cell-free circulating BRAFV600E mutation analysis by use of droplet digital PCR in the follow-up of patients with melanoma being treated with BRAF inhibitors. Clin Chem. 2015;61(1):297–304. doi:10.1373/clinchem.2014.230235.
- Chaudhuri AA, Chabon JJ, Lovejoy AF, Newman AM, Stehr H, Azad TD, Khodadoust MS, Esfahani MS, Liu CL, Zhou L. Early Detection of Molecular Residual Disease in Localized Lung Cancer by Circulating Tumor DNA Profiling. Cancer Discov. 2017;7(12):1394–1403. doi:10.1158/2159-8290.CD-17-0716.
- Odegaard JI, Vincent JJ, Mortimer S, Vowles JV, Ulrich BC, Banks KC, Fairclough SR, Zill OA, Sikora M, Mokhtari R, et al. Validation of a Plasma-Based Comprehensive Cancer Genotyping Assay Utilizing Orthogonal Tissue- and Plasma-Based Methodologies. Clin Cancer Res. 2018;24(15):3539–3549. doi:10.1158/1078-0432.CCR-17-3831.
- Strijker M, Soer EC, de Pastena M, Creemers A, Balduzzi A, Beagan JJ, Busch OR, Delden OM, Halfwerk H, Hooft JE, et al. Circulating tumor DNA quantity is related to tumor volume and both predict survival in metastatic pancreatic ductal adenocarcinoma. Int J Cancer. 2020;146(5):1445–1456. doi:10.1002/ijc.32586.
- Iijima Y, Hirotsu Y, Amemiya K, Ooka Y, Mochizuki H, Oyama T, Nakagomi T, Uchida Y, Kobayashi Y, Tsutsui T, et al. Very early response of circulating tumour-derived DNA in plasma predicts efficacy of nivolumab treatment in patients with non-small cell lung cancer. Eur J Cancer. 2017;86:349–357. doi:10.1016/j.ejca.2017.09.004.
- Zill OA, Banks KC, Fairclough SR, Mortimer SA, Vowles JV, Mokhtari R, Gandara DR, Mack PC, Odegaard JI, Nagy RJ, et al. The Landscape of Actionable Genomic Alterations in Cell-Free Circulating Tumor DNA from 21,807 Advanced Cancer Patients. Clin Cancer Res. 2018;24(15):3528–3538. doi:10.1158/1078-0432.CCR-17-3837.
- Oxnard GR, Thress KS, Alden RS, Lawrance R, Paweletz CP, Cantarini M, Yang JCH, Barrett JC, Jänne PA. Association Between Plasma Genotyping and Outcomes of Treatment With Osimertinib (AZD9291) in Advanced Non-Small-Cell Lung Cancer. J Clin Oncol. 2016;34(28):3375–3382. doi:10.1200/JCO.2016.66.7162.
- Said R, Guibert N, Oxnard GR, Tsimberidou AM. Circulating tumor DNA analysis in the era of precision oncology. Oncotarget. 2020;11(2):188–211. doi:10.18632/oncotarget.27418.
- Parikh AR, Mojtahed A, Schneider JL, Kanter K, Van Seventer EE, Fetter IJ, Thabet A, Fish MG, Teshome B, Fosbenner K. Serial ctDNA Monitoring to Predict Response to Systemic Therapy in Metastatic Gastrointestinal Cancers. Clin Cancer Res. 2020;26(8):1877–1885. doi:10.1158/1078-0432.CCR-19-3467.
- Lipson EJ, Velculescu VE, Pritchard TS, Sausen M, Pardoll DM, Topalian SL, Diaz Jr LA. Circulating tumor DNA analysis as a real-time method for monitoring tumor burden in melanoma patients undergoing treatment with immune checkpoint blockade. J Immunother Cancer. 2014;2:42. 10.1186/s40425-014-0042-0
- Moding EJ, Liu Y, Nabet BY, Chabon JJ, Chaudhuri AA, Hui AB, Bonilla RF, Ko RB, Yoo CH, Gojenola L, et al. Circulating tumor DNA dynamics predict benefit from consolidation immunotherapy in locally advanced non-small-cell lung cancer. Nat Cancer. 2020;1(2):176–183. doi:10.1038/s43018-019-0011-0.
- Giroux Leprieur E, Herbretau G, Dumenil C, Julie C, Giraud V, Labrune S, Dumoulin J, Tisserand J, Emile J-F, Blons H, et al. Circulating tumor DNA evaluated by Next-Generation Sequencing is predictive of tumor response and prolonged clinical benefit with nivolumab in advanced non-small cell lung cancer. Oncoimmunology. 2018;7(5):e1424675. doi:10.1080/2162402X.2018.1424675.
- Lee JH, Long GV, Boyd S, Lo S, Menzies AM, Tembe V, Guminski A, Jakrot V, Scolyer RA, Mann GJ, et al. Circulating tumour DNA predicts response to anti-PD1 antibodies in metastatic melanoma. Ann Oncol. 2017;28(5):1130–1136. doi:10.1093/annonc/mdx026.
- Song Y, Hu C, Xie Z, Wu L, Zhu Z, Rao C, Liu L, Chen Y, Liang N, Chen J, et al. Circulating tumor DNA clearance predicts prognosis across treatment regimen in a large real-world longitudinally monitored advanced non-small cell lung cancer cohort. Transl Lung Cancer Res. 2020;9(2):269–279. doi:10.21037/tlcr.2020.03.17.
- Chin YM, Takahashi Y, Chan HT, Otaki M, Fujishima M, Shibayama T, Miki Y, Ueno T, Nakamura Y, Low S. Ultradeep targeted sequencing of circulating tumor DNA in plasma of early and advanced breast cancer. Cancer Sci. 2021;112(1):454–464
- Wilson HL, D’Agostino RB Jr., Meegalla N, Petro R, Commander S, Topaloglu U, et al. The Prognostic and Therapeutic Value of the Mutational Profile of Blood and Tumor Tissue in Head and Neck Squamous Cell Carcinoma. Oncologist. 2020;26(2):e279-e289. doi:10.1002/onco.13573. .
- Pairawan S, Hess KR, Janku F, Sanchez NS, Mills Shaw KR, Eng C, Damodaran S, Javle M, Kaseb AO, Hong DS, et al. Cell-free Circulating Tumor DNA Variant Allele Frequency Associates with Survival in Metastatic Cancer. Clin Cancer Res. 2020;26(8):1924–1931. doi:10.1158/1078-0432.CCR-19-0306.
- Wyatt AW, Annala M, Aggarwal R, Beja K, Feng F, Youngren J, Foye A, Lloyd P, Nykter M, Beer TM. et al. Concordance of Circulating Tumor DNA and Matched Metastatic Tissue Biopsy in Prostate Cancer. J Natl Cancer Inst. 2017;109(12):10.1093/jnci/djx118
- Shatsky R, Parker BA, Bui NQ, Helsten T, Schwab RB, Boles SG, Kurzrock R. Next-Generation Sequencing of Tissue and Circulating Tumor DNA: the UC San Diego Moores Center for Personalized Cancer Therapy Experience with Breast Malignancies. Mol Cancer Ther. 2019;18(5):1001–1011. doi:10.1158/1535-7163.MCT-17-1038.
- Maron SB, Chase LM, Lomnicki S, Kochanny S, Moore KL, Joshi SS, Landron S, Johnson J, Kiedrowski LA, Nagy RJ. Circulating Tumor DNA Sequencing Analysis of Gastroesophageal Adenocarcinoma. Clin Cancer Res. 2019;25(23):7098–7112. doi:10.1158/1078-0432.CCR-19-1704.
- lWan JCM, Massie C, Garcia-Corbacho J, Mouliere F, Brenton JD, Caldas C, Pacey S, Baird R, Rosenfeld N. Liquid biopsies come of age: towards implementation of circulating tumour DNA. Nat Rev Cancer. 2017;17:223–238.
- Naumann DN, Hazeldine J, Dinsdale RJ, Bishop JR, Midwinter MJ, Harrison P, Hutchings SD, Lord JM. Endotheliopathy is associated with higher levels of cell-free DNA following major trauma: a prospective observational study. PLoS One. 2017;12(12):e0189870. doi:10.1371/journal.pone.0189870.
- Duvvuri B, Lood C. Cell-Free DNA as a Biomarker in Autoimmune Rheumatic Diseases. Front Immunol. 2019;10:502. doi:10.3389/fimmu.2019.00502.
- Li X, Hu R, Luo T, Peng C, Gong L, Hu J, Yang S, Li Q. Serum cell-free DNA and progression of diabetic kidney disease: a prospective study. BMJ Open Diabetes Res Care. 2020;8(1):e001078. doi:10.1136/bmjdrc-2019-001078.
- Polina IA, Ilatovskaya DV, DeLeon-Pennell KY. Cell free DNA as a diagnostic and prognostic marker for cardiovascular diseases. Clin Chim Acta. 2020;503:145–150. doi:10.1016/j.cca.2020.01.013.
- Teo YV, Capri M, Morsiani C, Pizza G, Faria AMC, Franceschi C, Neretti N. Cell-free DNA as a biomarker of aging. Aging Cell. 2019;18(1):e12890. doi:10.1111/acel.12890.
- Tirumani SH, Shinagare AB, O’Neill AC, Nishino M, Rosenthal MH, Ramaiya NH. Accuracy and feasibility of estimated tumour volumetry in primary gastric gastrointestinal stromal tumours: validation using semiautomated technique in 127 patients. Eur Radiol. 2016;26(1):286–295. doi:10.1007/s00330-015-3829-6.
- Tomayko MM, Reynolds CP. Determination of subcutaneous tumor size in athymic (nude) mice. Cancer Chemother Pharmacol. 1989;24(3):148–154. doi:10.1007/BF00300234.
- Lanman RB, Mortimer SA, Zill OA, Sebisanovic D, Lopez R, Blau S, Collisson EA, Divers SG, Hoon DSB, Kopetz ES. Analytical and Clinical Validation of a Digital Sequencing Panel for Quantitative, Highly Accurate Evaluation of Cell-Free Circulating Tumor DNA. PLoS One. 2015;10(10):e0140712. doi:10.1371/journal.pone.0140712.
- Zuur AF, Ieno EN, Elphick CS. A protocol for data exploration to avoid common statistical problems. Methods Ecol Evol. 2010;1(1):3–14. doi:10.1111/j.2041-210X.2009.00001.x.
- Zhang Q, Luo J, Wu S, Si H, Gao C, Xu W, Abdullah SE, Higgs BW, Dennis PA, van der Heijden MS, et al. Prognostic and Predictive Impact of Circulating Tumor DNA in Patients with Advanced Cancers Treated with Immune Checkpoint Blockade. Cancer Discov. 2020;10(12):1842–1853. doi:10.1158/2159-8290.CD-20-0047.
- Bachet JB, Blons H, Hammel P, Hariry IE, Portales F, Mineur L, Metges J-P, Mulot C, Bourreau C, Cain J, et al. Circulating Tumor DNA is Prognostic and Potentially Predictive of Eryaspase Efficacy in Second-line in Patients with Advanced Pancreatic Adenocarcinoma. Clin Cancer Res. 2020;26(19):5208–5216. doi:10.1158/1078-0432.CCR-20-0950.
- Jiang J, Ye S, Xu Y, Chang L, Hu X, Ru G, Guo Y, Yi X, Yang L, Huang D, et al. Circulating Tumor DNA as a Potential Marker to Detect Minimal Residual Disease and Predict Recurrence in Pancreatic Cancer. Front Oncol. 2020;10:1220. doi:10.3389/fonc.2020.01220.
- An Y, Guan Y, Xu Y, Han Y, Wu C, Bao C, Zhou B, Wang H, Zhang M, Liu W, et al. The diagnostic and prognostic usage of circulating tumor DNA in operable hepatocellular carcinoma. Am J Transl Res. 2019;11:6462–6474.
- Kato S, Okamura R, Baumgartner JM, Patel H, Leichman L, Kelly K, Sicklick JK, Fanta PT, Lippman SM, Kurzrock R, et al. Analysis of Circulating Tumor DNA and Clinical Correlates in Patients with Esophageal, Gastroesophageal Junction, and Gastric Adenocarcinoma. Clin Cancer Res. 2018;24(24):6248–6256. doi:10.1158/1078-0432.CCR-18-1128.
- Li J, Jiang W, Wei J, Zhang J, Cai L, Luo M, Wang Z, Sun W, Wang S, Wang C, et al. Patient specific circulating tumor DNA fingerprints to monitor treatment response across multiple tumors. J Transl Med. 2020;18(1):293. doi:10.1186/s12967-020-02449-y.
- Velimirovic M, Juric D, Niemierko A, Spring L, Vidula N, Sa W, Medford A, Parikh A, Malvarosa G, Yuen M, et al. Rising Circulating Tumor DNA As a Molecular Biomarker of Early Disease Progression in Metastatic Breast Cancer. JCO Precision Oncology. 2020;1246–1262.4. 10.1200/PO.20.00117
- Madic J, Kiialainen A, Bidard FC, Birzele F, Ramey G, Leroy Q, Frio TR, Vaucher I, Raynal V, Bernard V, et al. Circulating tumor DNA and circulating tumor cells in metastatic triple negative breast cancer patients. Int J Cancer. 2015;136(9):2158–2165. doi:10.1002/ijc.29265.
- Tie J, Cohen JD, Wang Y, Li L, Christie M, Simons K, Elsaleh H, Kosmider S, Wong R, Yip D, et al. Serial circulating tumour DNA analysis during multimodality treatment of locally advanced rectal cancer: a prospective biomarker study. Gut. 2019;68(4):663–671. doi:10.1136/gutjnl-2017-315852.
- Cohen JD, Li L, Wang Y, Thoburn C, Afsari B, Danilova L, Douville C, Javed AA, Wong F, Mattox A, et al. Detection and localization of surgically resectable cancers with a multi-analyte blood test. Science. 2018;359(6378):926–930. doi:10.1126/science.aar3247.
- Vu P, Khagi Y, Riviere P, Goodman A, Kurzrock R. Total Number of Alterations in Liquid Biopsies Is an Independent Predictor of Survival in Patients With Advanced Cancers. JCO Precis Oncol. 2020 Mar 24;4:PO.19.00204. doi: 10.1200/PO.19.00204.
- PN D, Tm B, Vj B, Chua W, YF M, Lynch D, Po J, Luk AWS, Caixeiro N, de Souza P, et al. The predictive and prognostic significance of liquid biopsy in advanced epidermal growth factor receptor-mutated non-small cell lung cancer: a prospective study. Lung Cancer. 2019;134:187–193. doi:10.1016/j.lungcan.2019.06.021.
- Stawski R, Walczak K, Perdas E, Wlodarczyk A, Sarniak A, Kosielski P, Meissner P, Budlewski T, Padula G, Nowak D, et al. Decreased integrity of exercise-induced plasma cell free nuclear DNA – negative association with the increased oxidants production by circulating phagocytes. Sci Rep. 2019;9(1):15970. doi:10.1038/s41598-019-52409-w.
- Jing RR, Wang HM, Cui M, Fang MK, Qiu XJ, Wu XH, Qi J, Wang Y-G, Zhang L-R, Zhu J-H, et al. A sensitive method to quantify human cell-free circulating DNA in blood: relevance to myocardial infarction screening. Clin Biochem. 2011;44(13):1074–1079. doi:10.1016/j.clinbiochem.2011.06.083.
- Tsai NW, Lin TK, Chen SD, Chang WN, Wang HC, Yang TM, Lin Y-J, Jan C-R, Huang C-R, Liou C-W, et al. The value of serial plasma nuclear and mitochondrial DNA levels in patients with acute ischemic stroke. Clin Chim Acta. 2011;412:476–479. doi:10.1016/j.cca.2010.11.036.
- Wang E, Batey A, Struble C, Musci T, Song K, Oliphant A. Gestational age and maternal weight effects on fetal cell-free DNA in maternal plasma. Prenat Diagn. 2013;33(7):662–666. doi:10.1002/pd.4119.
- Scott FP, Menezes M, Palma-Dias R, Nisbet D, Schluter P, Da Silva Costa F, McLennan AC. Factors affecting cell-free DNA fetal fraction and the consequences for test accuracy. J Matern Fetal Neonatal Med. 2018;31(14):1865–1872. doi:10.1080/14767058.2017.1330881.
- Chan N, Smet ME, Sandow R, Da Silva Costa F, McLennan A. Implications of failure to achieve a result from prenatal maternal serum cell-free DNA testing: a historical cohort study. BJOG. 2018;125:848–855. doi:10.1111/1471-0528.15006.
- Diaz LA Jr., Bardelli A. Liquid biopsies: genotyping circulating tumor DNA. J Clin Oncol. 2014;32(6):579–586. doi:10.1200/JCO.2012.45.2011.
- Avanzini S, Kurtz DM, Chabon JJ, Moding EJ, Hori SS, Gambhir SS, et al. A mathematical model of ctDNA shedding predicts tumor detection size. Sci Adv. 2020;6(50):eabc4308. doi: 10.1126/sciadv.abc4308.
- Stroun M, Lyautey J, Lederrey C, Olson-Sand A, Anker P. About the possible origin and mechanism of circulating DNA apoptosis and active DNA release. Clin Chim Acta. 2001;313(1–2):139–142. doi:10.1016/S0009-8981(01)00665-9.
- Ulz P, Thallinger GG, Auer M, Graf R, Kashofer K, Jahn SW, Abete L, Pristauz G, Petru E, Geigl JB. Inferring expressed genes by whole-genome sequencing of plasma DNA. Nat Genet. 2016;48(10):1273–1278. doi:10.1038/ng.3648.
- Snyder Matthew W, Kircher M, Hill Andrew J, Daza Riza M, Cell-free SJ. DNA Comprises an In Vivo Nucleosome Footprint that Informs Its Tissues-Of-Origin. Cell. 2016;164:57–68. doi:10.1016/j.cell.2015.11.050.
- Dvorakova M, Karafiat V, Pajer P, Kluzakova E, Jarkovska K, Pekova S, Krutílková L, Dvořák M. DNA released by leukemic cells contributes to the disruption of the bone marrow microenvironment. Oncogene. 2013;32(44):5201–5209. doi:10.1038/onc.2012.553.
- Garcia-Olmo DC, Dominguez C, Garcia-Arranz M, Anker P, Stroun M, Garcia-Verdugo JM, García-Olmo D. Cell-free nucleic acids circulating in the plasma of colorectal cancer patients induce the oncogenic transformation of susceptible cultured cells. Cancer Res. 2010;70(2):560–567. doi:10.1158/0008-5472.CAN-09-3513.
- Nishimoto S, Fukuda D, Higashikuni Y, Tanaka K, Hirata Y, Murata C, Kim-Kaneyama J-R, Sato F, Bando M, Yagi S. Obesity-induced DNA released from adipocytes stimulates chronic adipose tissue inflammation and insulin resistance. Sci Adv. 2016;2(3):e1501332. doi:10.1126/sciadv.1501332.
- Lee JH, Menzies AM, Carlino MS, McEvoy AC, Sandhu S, Weppler AM, Diefenbach RJ, Dawson S-J, Kefford RF, Millward MJ, et al. Longitudinal Monitoring of ctDNA in Patients with Melanoma and Brain Metastases Treated with Immune Checkpoint Inhibitors. Clin Cancer Res. 2020;26(15):4064–4071. doi:10.1158/1078-0432.CCR-19-3926.
- Pereira B, Chen CT, Goyal L, Walmsley C, Pinto CJ, Baiev I, Allen R, Henderson L, Saha S, Reyes S, et al. Cell-free DNA captures tumor heterogeneity and driver alterations in rapid autopsies with pre-treated metastatic cancer. Nat Commun. 2021;12(1):3199. doi:10.1038/s41467-021-23394-4.
- Jaiswal S, Fontanillas P, Flannick J, Manning A, Grauman PV, Mar BG, Lindsley RC, Mermel CH, Burtt N, Chavez A, Jaiswal S, Fontanillas P, Flannick J, Manning A, Grauman PV, Mar BG, et al, Age-related clonal hematopoiesis associated with adverse outcomes. N Engl J Med. 2014;371(26):2488–2498. 10.1056/NEJMoa1408617
- Razavi P, Li BT, Brown DN, Jung B, Hubbell E, Shen R, Abida W, Juluru K, De Bruijn I, Hou C, et al. High-intensity sequencing reveals the sources of plasma circulating cell-free DNA variants. Nat Med. 2019;25(12):1928–1937. doi:10.1038/s41591-019-0652-7.
- Gibson CJ, Lindsley RC, Tchekmedyian V, Mar BG, Shi J, Jaiswal S, Bosworth A, Francisco L, He J, Bansal A, et al. Clonal Hematopoiesis Associated With Adverse Outcomes After Autologous Stem-Cell Transplantation for Lymphoma. J Clin Oncol. 2017;35(14):1598–1605. doi:10.1200/JCO.2016.71.6712.
- Martincorena I. Somatic mutation and clonal expansions in human tissues. Genome Med. 2019;11(1):35. doi:10.1186/s13073-019-0648-4.