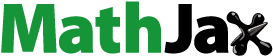
ABSTRACT
Fibrosis, a progressive accumulation of extracellular matrix components, encompasses a wide spectrum of distinct organs, and accounts for an increasing burden of morbidity and mortality worldwide. Despite the tremendous clinical impact, the mechanisms governing the fibrotic process are not yet understood, and to date, no clinically reliable therapies for fibrosis have been discovered. Here we applied Regeneration Intelligence, a new bioinformatics software suite for qualitative analysis of intracellular signaling pathway activation using transcriptomic data, to assess a network of molecular signaling in lung and liver fibrosis. In both tissues, our analysis detected major conserved signaling pathways strongly associated with fibrosis, suggesting that some of the pathways identified by our algorithm but not yet wet-lab validated as fibrogenesis related, may be attractive targets for future research. While the majority of significantly disrupted pathways were specific to histologically distinct organs, several pathways have been concurrently activated or downregulated among the hepatic and pulmonary fibrosis samples, providing new evidence of evolutionary conserved pathways that may be relevant as possible therapeutic targets. While future confirmatory studies are warranted to validate these observations, our platform proposes a promising new approach for detecting fibrosis-promoting pathways and tailoring the right therapy to prevent fibrogenesis.
Introduction
Fibrosis, the thickening and scarring of connective tissue due to accumulation of extracellular matrix molecules, is a common feature associated with chronic tissue inflammation.Citation1 Chronic fibrotic diseases often result in widespread distortion of normal tissue architecture and account for up to 45% of deaths in the developed world.Citation2 While fibrosis can affect all major tissues, idiopathic pulmonary fibrosis (IPF)Citation3 and hepatic cirrhosisCitation4 are among the more common fibrotic diseases, and as such, are associated with an unmet clinical need.
Studies of organ fibrosis have flourished over the last decade and common features of fibrosis across different tissues have been previously described. For example, while tissue fibroblasts are normally heterogeneous, pathologic myofibroblasts exhibit similar histological phenotype and molecular characteristics in lung, kidney or liver fibrosis, suggesting that fundamental, conserved pathogenic pathways are common in fibrotic organs.Citation5 Despite multiple efforts to assess the gene expression or phosphoproteomics signatures associated with fibrosis in several distinct organs,Citation6 no reliable markers of clinical utility have been identified and to date, no approved anti-fibrotic therapies have been discovered.Citation2
It was previously suggested that development of advanced fibrosis across different organs may be associated with unique levels of signaling pathway activation,Citation7 however the complex signaling network in fibrotic diseases have been largely overlooked and the broad understanding of fundamental molecular pathways across multiple fibrotic organs is still lacking. The pathogenesis of fibrotic disease regardless of etiology shares common features including altered epithelial-mesynchymal cell interactions, inflammation, and proliferation of fibroblasts as a result of chronic activation of the myofibroblast cell type.Citation8 A line of evidence suggests that these events may result from alterations in common regulatory pathways, such as TGFβ-SMAD2/3 signaling that drives cellular transdifferentiation to myofibroblasts and abnormal extracellular matrix deposition, and PI3K–AKT signaling that contributes to myofibroblast resistance to apoptosis.Citation8,9 Although evidence supporting the concept of core fibrosis pathways is strong and compelling, as summarized in a recent review article by Rockey et al.,Citation9 most of the recent data were gathered across many single-organ studies and a direct comparison of pathway signatures from human liver vs. lung fibrosis have not been reported previously.
To further investigate this hypothesis we used a novel software suite, Regeneration Intelligence, for quantitative and qualitative analysis of the intracellular signaling pathway activation (SPA)Citation10,11 based on the transcriptomic data from 32 hepatic and 13 IPF patients. The intracellular SPA analysis is a universal method, which may be used to analyze any physiological, stress, malignancyCitation12 and other perturbed conditions at the molecular level. In contrast to other existing techniques for aggregation and generalization of the gene expression data for individual samples, our method distinguishes the positive/activator and negative/repressor role of every gene product in each of the distinct signaling pathways analyzed and determines its pathway activation score (PAS).Citation10
Results
Regeneration Intelligence detects major conserved pathways involved in lung and liver fibrogenesis
Using stringent criteria (p < 0.0005 has been chosen to restrict out analysis to the most significantly dysregulated pathways), our analysis showed that the PAS values of 61 and 41 distinct pathways, in hepatic and pulmonary fibrotic samples respectively, were significantly dysregulated compared to controls (Table S1). In both tissues, our comprehensive analysis revealed alterations in signaling pathways that are commonly associated with fibrosis, further supporting their potential functional role in fibrogenesis, and confirming the success of our conservative filtering approach. For example, among the top 20 most significantly altered signaling axis in hepatic patients, we found MAPK/ERK, PAK/P38, integrins/ILK, IL6 and AKT pathways (Table S1). The MAPK/ERK signaling cascade is a major pathway controlling cellular processes associated with fibrogenesis, including growth, proliferation, and surviva,Citation13 whereas PAK/P38 signaling plays a key role in pro-fibrogenic epithelial–mesenchymal transition (EMT),Citation14 and was shown to be upregulated in response to lysoposphatidic acid during organ fibrogenesis.Citation15,16 Moreover, ERK and p38 MAPK pathways are principally related with the TGFβ signaling,Citation17 which plays a central role in fibrotic disorders by inducing multiple pro-fibrogenic and immunosuppressive effects in various distinct organs including liver.Citation18 Several studies have shown that TGFβ also mediates PI3K/AKT signaling in fibrosis models.Citation19 Similarly, integrin-linked kinase (ILK), the member of the signal transduction cascade between integrins and growth factor receptors, is also involved in the activation of ERK and p38 MAPK signaling during the development of hepatic fibrosis.Citation20 Finally, IL6 supports fibrogenesis by shifting acute inflammation into a more chronic pro-fibrotic state through induction of Th1 cell responses as a consequence of recurrent inflammation.Citation21 Furthermore, as a potent activator of the JAK-STAT signaling, IL6 may play a crucial role during hepatic stellate cell activation and transdifferentiation into myofibroblasts, the key events in liver fibrogenesis.Citation22
Similarly to liver, the well-established fibrosis-associated pathways, such as those involving ILK, PAK and ERK, were also found among the 20 most significantly altered signaling pathways in IPF patients ( and Table S1). EGFR signaling pathway was also found to be disrupted (Table S1). Aberrant EGFR signaling is associated with the early stage pathogenesis of lung fibrosis, cancer and numerous airway hypersecretory diseases,Citation23 and it therefore provides an attractive therapeutic target for reduction of fibrogenesis in human organsCitation24 and animal models.Citation23
Figure 1. Intercross of signaling pathways in liver and lung fibrosis reveals common fibrosis pathway signature. Gene expression data derived from lung dataset GES2052 and liver data set GES45941 was analyzed to estimate Pathway Activation Scores (PAS) using corresponding fibrosis-free tissues as a reference. Only pathways with PAS p-values less than 0.0005 have been selected. (A). Venn diagram illustrates the upregulated pathways which are overlapping in lung and liver fibrotic tissues. (B). Venn diagram illustrates the downregulated pathways which are overlapping in lung and liver fibrotic tissues. (C) Distribution of PAS values of 22 shared signaling pathways (results from A and B) in fibrotic lung and liver tissue samples.
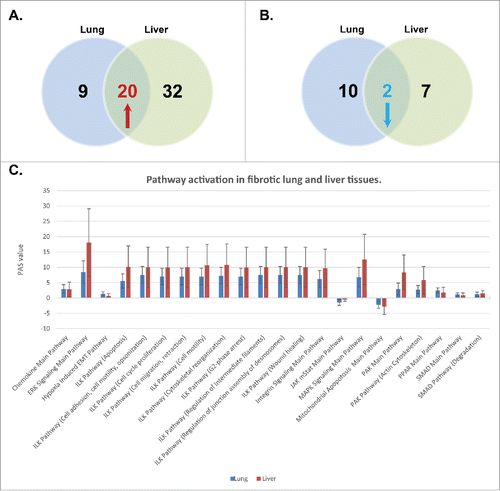
While the association of the above signaling pathways with fibrosis is not new, the observation that Regeneration Intelligence is able to detect well characterized molecular processes involved in fibrogenesis of both liver and lung, is a reasonable validation of the utility of the software, and supports the credibility of the pathway activation score estimation as a promising analytical approach.
Shared pathway signatures in liver and lung fibrosis
Surprisingly, while our data showed that the majority of significantly disrupted pathways are specific to histologically distinct organs (Table S1), several pathways and their major signaling branches have been concurrently activated (20 pathways) or downregulated (2 pathways) among the hepatic and pulmonary fibrosis samples (, Table S2). While larger confirmatory studies are warranted to further validate our observations, it is tempting to speculate that these pathways may represent fibrosis-associated “core pathways”, essential to convert an initial stimulus to the development of fibrosis in most of the fibrotic patients. Whereas versatile pathways specific to each of the organs tested are regulatory pathways, which can influence the core pathways but do not directly convert the initial stimulus into the basic component of fibrosis.Citation2
The downregulation of mitochondrial apoptosis pathway and JAK/STAT pathway in fibrotic samples (, Table S2) further supports the credibility of our approach, since myofibroblast resistance to apoptosis is a cardinal feature of chronic fibrotic diseases involving diverse organ systems,Citation25 and protective role of JAK/STAT signaling has been demonstrated in renalCitation26 and hepaticCitation22 fibrosis. All of the upregulated signaling cascades shown (, Table S2) have cross-talk to induce complex networks that regulate inflammation and fibrosis in various human organs, and are often triggered by the exposure of effector cells to circulating or locally produced molecules that stimulate the biosynthesis and secretion of extracellular matrix proteins. Interestingly, an extensive transactivation has been observed between several pathways, such as ERK/MAPK,Citation17 integrins/ILK,Citation27 PPAR,Citation28 PAK,Citation29 SMAD,Citation30 hypoxia/EMT,Citation31 and TGFβ signaling, a potent stimulator of the synthesis of extracellular matrix proteins, which is important in virtually all types of fibrosis.Citation32 These findings further support the concept that TGFβ is a harbinger of fibrogenic cascade activation.Citation9 Although in animal models it was shown that TGFβ could cause fibrosis independently, a complete blockade of TGFβ was associated with substantial toxicity.Citation32 Due to the complexity of TGFβ signaling, this raises the question of whether specific targeting of fibrosis-promoting downstream pathway branches will be safer and more efficient then a pan-TGFβ blockade. Although given the limited availability of well-annotated gene expression datasets we cannot answer this important question, this work emphasizes that our approach may help to elucidate the complex fibrosis-promoting pathways and unravel their most critical branches for potential drug targeting.
Analysis of fibrosis-associated pathways with IPA Ingenuity and MetaCore
We next performed parallel analyses using QIAGEN's Ingenuity Pathway Analysis (IPA®,QIAGEN Redwood City, www.qiagen.com/ingenuity) and MetaCore from Thomson Reuters (http://thomsonreuters.com/en/products-services/pharma-life-sciences/pharmaceutical-research/metacore.html) software with the same input data sets utilized to elucidate fibrosis-associated pathways with Regeneration Intelligence toolset and compared data generated by these alternative methods with our results. IPA and Metacore software each produced 2 tables which contain list of pathways significantly enriched in either lung or liver fibrosis. Using the same statistical criteria (p < 0.0005) as has been used for selection of pathways obtained by Regeneration Intelligence, IPA Ingenuity pathway analysis revealed that 18 and 8 distinct pathways, in hepatic and pulmonary fibrotic samples respectively, were significantly enriched compared to controls (Tables S3 and S4). Several fibrosis-associated pathways (stellate cell activation and signaling involving LXRCitation33 and RXRCitation34) were enriched in fibrotic patients according to the IPA analysis. However, such well-characterized fibrogenesis-driving molecular processes as TFGβ-SMAD, PAK/P38, integrins/ILK or PI3K/AKT were neither detected in lung nor in liver fibrosis patient cohorts. Stellate cell activation’ was the only pathway concurrently enriched across hepatic and pulmonary fibrosis samples, perhaps due to erroneous assignment of liver specific pathways to non-hepatic tissues.
MetaCore suite identified 10 significantly enriched pathways in each of the tissues analyzed. MetaCore analysis revealed crucial basic component of fibrosis signaling network in both pulmonary and hepatic samples, such as IL-1,Citation35,36 IL-10,Citation37,38 TGFβCitation9,18,39 and WNTCitation40,41 signaling pathways and components of EMT mediating networkCitation42,43 (Tables S5 and S6). Nevertheless, no shared pathways concurrently enriched in liver and lung fibrosis have been identified.
Although using strict criteria, results generated by MetaCore and IPA ingenuity analysis revealed pathways relevant to organ fibrosis, neither platform was able to identify systemic fibrosis signature common to both distinct organs.
Discussion
An understanding of the protein signaling architecture in fibrogenesis is of critical importance for the development of new therapeutic approaches and identification of predictive and prognostic biomarkers. Numerous studies have used genomic- and phosphoproteomics-based approaches to characterize the molecular underpinnings of fibrogenesis,Citation6,9 however a systematic comprehensive analysis of the signaling pathways activation in distinct fibrotic organs has never been done.
In this work we used the new bioinformatics software suite for the analysis of intracellular signaling pathway activation using transcriptomic data, for quantitative and qualitative comparison of the signaling pathway activation in lung and liver fibrosis. Due to its universal applicability, this platform was established as a potent in-silico drug screening and efficacy prediction tool.Citation10,11,44
By utilizing our approach we were able to detect some of the major conserved molecular processes involved in fibrogenesis, such as those involving TFGβCitation32, IL6Citation45,46 and ILKCitation47 signaling. This is a reasonable validation of the utility of the software, which suggests that our platform provides a rational bio-mathematical framework for studying signaling pathway alterations driving the fibrogenetic processes. Furthermore, the fact that virtually all pathways that passed our stringent threshold (p < 0.0005) were previously experimentally validated as core fibrotic processes, suggests that some of the next-tier pathways (0.0005 < p < 0.005) identified by our algorithm but not yet wet-lab validated as fibrogenesis related, may be attractive targets for future research (Table S1).
Although the concept that fibrosis follows many common pathways across diverse organs was recently described,Citation9 most of the evidence was gathered across many single-organ studies and to the best of our knowledge, our work for the first time directly compares the pathway signatures from human liver and lung fibrosis. We acknowledge that due to the limited samples size, our findings are exciting but exploratory, and are therefore restricted to hypothesis generation. However, given the difficulty of obtaining gene expression data of well annotated, clinically relevant fibrotic specimens, the datasets used in this work represent a unique model to study pathways alterations that drive lung and liver fibrogenesis.
During the past 2 decades, systematic collections of pathway data from experimental studies have been compiled by several databases in both academic and commercial settings and various computational scoring platforms that can project gene expression data into a network of molecular signaling have been proposed for integrative pathway analysis.Citation48,49 Despite significant advancements, several obvious drawbacks and challenges - such as lack of information regarding the pathway down- or upregulation, inconsistent pathways annotation, difficulties to understand the obtained values and inconsistency of the data coming from the various types of equipment - may lead to the incorrect interpretation of the underlying biological processes. Therefore, it is reasonable to assume, that the abovementioned problems could contribute to the inability of IPA-Ingenuity and MetaCore software suits to identify common fibrosis signatures in distinct fibrotic tissues. Specifically, inconsistency and/or redundancy in pathway names annotations and number of pathway components may result in different statistical weights assigned to these pathways, and subsequently lead to the inability to detect signaling pathways shared between different biological systems. This motivated us to create a method which allows accurate and robust cross-platform analysis of gene expression data obtained using different high-throughput techniques and enables scientists to characterize the functional states of transcriptomes more accurately than before.Citation44,50-52 Nevertheless, given the difficulty to unify inputs from different existing signaling pathways databases (UniProt, HPRD, QIAGEN SABiosciences, WikiPathways, Ariadne Pathway Studio, SPIKE, Reactome, KEGG and others) used by other software that conduct pathway analyses (such those tested here), the direct comparison of the data generated by different approaches should be addressed with caution.
One of the main advantages of the proposed pathway scoring utility is the ability to predict the up- or downregulation for every differentially dysregulated signaling pathway. Since target-based drug discovery largely focuses on gain-of-function mutations and upregulation of biological processes, unveiling the directionality of signaling pathways that may be responsible for the fibrogenesis could provide the foundation for a target-based therapeutic intervention at the pathway level.
In conclusion, our work further supports the theory that conserved signaling elements may be shared in various fibrotic organs, and provides new evidence of evolutionary conserved pathways that may be relevant as possible therapeutic targets. Future work using the Regeneration Intelligence platform could shed light into molecular mechanisms responsible for the development, maintenance and progression of advanced fibrosis, and may provide further insight into its fundamental biologic mechanisms.
Materials and methods
Microarray data sets
We carefully reviewed several publicly available transcriptomic repository databases for the microarray-profiled human biopsy samples of fibrosis affected lung and liver tissues and appropriate controls which served as a reference for PAS calculation. Only two datasets met our inclusion criteria (clinical material obtained via biopsy or surgical resection): GSE2052 (http://www.ncbi.nlm.nih.gov/geo/query/acc.cgi?acc=GSE2052), contains the information on 24 lung samples profiled using Amersham Biosciences CodeLink Uniset Human I Bioarray arrays. This cohort includes 13 samples obtained from patients diagnosed with idiopathic pulmonary fibrosis (criteria of the American Thoracic Society and European Respiratory Society) who underwent pulmonary transplant, and 11 histologically normal lung tissue samples resected from lung cancer patients.Citation53 The second set, GSE49541 (http://www.ncbi.nlm.nih.gov/geo/query/acc.cgi?acc=GSE49541) contains data for 72 samples obtained via diagnostic biopsy in nonalcoholic fatty liver disease (NAFLD) patients. Samples in this cohort were scored into various fibrotic stages according to Pathology Committee of the NASH Clinical Research Network. Biopsies scored as fibrosis stage 0–1 (fibrosis-free/very limited fibrosis were used as control cases in this study. 32 samples diagnosed as severe/advanced fibrosis (stage 3–4) we referred to as fibrotic liver cases.Citation54 Transcriptomic data in this set was obtained using Affymetrix Human Genome U133 Plus 2.0 Array.
Expression data pre-processing
All microarray data preprocessing steps were performed in R version 3.1.0 using packages from Bioconductor. Microarray raw data files were background adjusted and quantile normalized.
Dimensionality reduction of data sets
Obtained gene expression values for all replicates were combined into 2 matrices (for fibrosis and control samples, respectively), which were then subjected to the procedure of non-negative matrix factorization. The leading contributions to both fibrosis and control sample matrices had the form of a n x m rank 1 matrix, where n is the number of samples and m is the number of genes represented in the transcriptome. As such matrices are the tensor products of 2 n- and m-component vectors, the m-component vectors were considered as the transcriptional signature vectors of control and fibrosis samples, respectively.
Pathway activation score (PAS) calculation
Preprocessed and dimensionality-reduced gene expression data were loaded into Regeneration Intelligence, a proprietary software suite developed by Atlas Regeneration, Inc. The software enables calculation of the Pathway Activation Score (PAS) for each of the 271 pathways analyzed, a value which serves as a quantitative measure of differential pathway activation between the 2 states. Regeneration Intelligence software suite is a cloud based implementation of topological gene expression aggregation algorithm,Citation10 optimized for the needs of stem cells research and regenerative medicine. The algorithm utilizes the following formula to evaluate pathway activation:
Here, CNRn is the ratio of the expression level of a gene n in the pathologic sample and in the control; BTIFn is a value of beyond tolerance interval flag, which equals 0 (if 0.67<CNR<1.5) or 1 (if CNR<0.67 or CNR>1.5); and ARR (activator/repressor role) serves as a discrete value which equals to either −1, −0.5, 0, 0.5 or 1 depending on the topological role (activator or repressor) of the particular gene/protein in the signaling pathway p, respectively.Citation10,11 Results for the pathway activation scores for 271 pathways, which were estimated for each sample can be found in supplement materials (Table S1). Pathways with positive PAS values are upregulated, while negative PAS values correspond to down-regulated pathways.
Disclosure of potential conflicts of interest
No potential conflicts of interest were disclosed.
Supplemental Files
Download Zip (222.9 KB)References
- Hernandez-Gea V, Friedman SL. Pathogenesis of liver fibrosis. Annu Rev Pathol 2011; 6:425-56; PMID:21073339; http://dx.doi.org/10.1146/annurev-pathol-011110-130246
- Mehal WZ, Iredale J, Friedman SL. Scraping fibrosis: expressway to the core of fibrosis. Nat Med 2011; 17:552-3; PMID:21546973; http://dx.doi.org/10.1038/nm0511-552
- Raghu G, Weycker D, Edelsberg J, Bradford WZ, Oster G. Incidence and prevalence of idiopathic pulmonary fibrosis. Am J Respir Crit Care Med 2006; 174:810-6; PMID:16809633; http://dx.doi.org/10.1164/rccm.200602-163OC
- Sass DA, Chang P, Chopra KB. Nonalcoholic fatty liver disease: a clinical review. Dig Dis Sci 2005; 50:171-80; PMID:15712657; http://dx.doi.org/10.1007/s10620-005-1267-z
- Zeisberg M, Kalluri R. Cellular mechanisms of tissue fibrosis. 1. Common and organ-specific mechanisms associated with tissue fibrosis. Am J Physiol Cell Physiol 2013; 304:C216-25; PMID:23255577; http://dx.doi.org/10.1152/ajpcell.00328.2012
- Eddy AA. Scraping fibrosis: UMODulating renal fibrosis. Nat Med 2011; 17:553-5; PMID:21546974; http://dx.doi.org/10.1038/nm0511-553
- Yu Z, Xie M, Fan X, Jia J. Interferon alpha2b Increases MMP-13 and IL-10 expression in Kupffer cells through MAPK signaling pathways. Hepatogastroenterology 2015; 62:350-4; PMID:25993756.
- Nanthakumar CB, Hatley RJ, Lemma S, Gauldie J, Marshall RP, Macdonald SJ. Dissecting fibrosis: therapeutic insights from the small-molecule toolbox. Nat Rev Drug Discov 2015; 14:693-720; PMID:26338155; http://dx.doi.org/10.1038/nrd4592
- Rockey DC, Bell PD, Hill JA. Fibrosis–a common pathway to organ injury and failure. N Engl J Med 2015; 372:1138-49; PMID:25785971; http://dx.doi.org/10.1056/NEJMra1300575
- Buzdin AA, Zhavoronkov AA, Korzinkin MB, Venkova LS, Zenin AA, Smirnov PY, Borisov NM. Oncofinder, a new method for the analysis of intracellular signaling pathway activation using transcriptomic data. Front Genet 2014; 5:55; PMID:24723936; http://dx.doi.org/10.3389/fgene.2014.00055
- Buzdin AA, Zhavoronkov AA, Korzinkin MB, Roumiantsev SA, Aliper AM, Venkova LS, Smirnov PY, Borisov NM. The OncoFinder algorithm for minimizing the errors introduced by the high-throughput methods of transcriptome analysis. Front Mol Biosci 2014; 1:8; PMID:25988149; http://dx.doi.org/10.3389/fmolb.2014.00008
- Zhu Q, Izumchenko E, Aliper AM, Makarev E, Paz K, Buzdin AA, Zhavoronkov AA, Sidransky D. Pathway activation strength is a novel independent prognostic biomarker for cetuximab sensitivity in colorectal cancer patients. Human Genome Variation 2015; 2; http://dx.doi.org/10.1038/hgv.2015.9
- Madala SK, Schmidt S, Davidson C, Ikegami M, Wert S, Hardie WD. MEK-ERK pathway modulation ameliorates pulmonary fibrosis associated with epidermal growth factor receptor activation. Am J Respir Cell Mol Biol 2012; 46:380-8; PMID:22021337; http://dx.doi.org/10.1165/rcmb.2011-0237OC
- Sebe A, Masszi A, Zulys M, Yeung T, Speight P, Rotstein OD, Nakano H, Mucsi I, Szaszi K, Kapus A. Rac, PAK and p38 regulate cell contact-dependent nuclear translocation of myocardin-related transcription factor. FEBS Lett 2008; 582:291-8; PMID:18154735; http://dx.doi.org/10.1016/j.febslet.2007.12.021
- Swaney JS, Chapman C, Correa LD, Stebbins KJ, Bundey RA, Prodanovich PC, Fagan P, Baccei CS, Santini AM, Hutchinson JH, et al. A novel, orally active LPA(1) receptor antagonist inhibits lung fibrosis in the mouse bleomycin model. Br J Pharmacol 2010; 160:1699-713; PMID:20649573; http://dx.doi.org/10.1111/j.1476-5381.2010.00828.x
- Pyne NJ, Dubois G, Pyne S. Role of sphingosine 1-phosphate and lysophosphatidic acid in fibrosis. Biochim Biophys Acta 2013; 1831:228-38; PMID:22801038; http://dx.doi.org/10.1016/j.bbalip.2012.07.003
- Derynck R, Zhang YE. Smad-dependent and Smad-independent pathways in TGF-beta family signalling. Nature 2003; 425:577-84; PMID:14534577; http://dx.doi.org/10.1038/nature02006
- Gressner AM, Weiskirchen R, Breitkopf K, Dooley S. Roles of TGF-beta in hepatic fibrosis. Front Biosci 2002; 7:d793-807; PMID:11897555; http://dx.doi.org/10.2741/gressner
- Nishida M, Okumura Y, Sato H, Hamaoka K. Delayed inhibition of p38 mitogen-activated protein kinase ameliorates renal fibrosis in obstructive nephropathy. Nephrol Dial Transplant 2008; 23:2520-4; PMID:18515792; http://dx.doi.org/10.1093/ndt/gfn309
- Zhang Y, Ikegami T, Honda A, Miyazaki T, Bouscarel B, Rojkind M, Hyodo I, Matsuzaki Y. Involvement of integrin-linked kinase in carbon tetrachloride-induced hepatic fibrosis in rats. Hepatology 2006; 44:612-22; PMID:16941698; http://dx.doi.org/10.1002/hep.21315
- Fielding CA, Jones GW, McLoughlin RM, McLeod L, Hammond VJ, Uceda J, Williams AS, Lambie M, Foster TL, Liao CT, et al. Interleukin-6 signaling drives fibrosis in unresolved inflammation. Immunity 2014; 40:40-50; PMID:24412616; http://dx.doi.org/10.1016/j.immuni.2013.10.022
- Kong X, Horiguchi N, Mori M, Gao B. Cytokines and STATs in Liver Fibrosis. Front Physiol 2012; 3:69; PMID:22493582; http://dx.doi.org/10.3389/fphys.2012.00069
- Vallath S, Hynds RE, Succony L, Janes SM, Giangreco A. Targeting EGFR signalling in chronic lung disease: therapeutic challenges and opportunities. Eur Respir J 2014; 44:513-22; PMID:24435005; http://dx.doi.org/10.1183/09031936.00146413
- Fuchs BC, Hoshida Y, Fujii T, Wei L, Yamada S, Lauwers GY, McGinn CM, DePeralta DK, Chen X, Kuroda T, et al. Epidermal growth factor receptor inhibition attenuates liver fibrosis and development of hepatocellular carcinoma. Hepatology 2014; 59:1577-90; PMID:24677197; http://dx.doi.org/10.1002/hep.26898
- Johnson A, DiPietro LA. Apoptosis and angiogenesis: an evolving mechanism for fibrosis. FASEB J 2013; 27:3893-901; PMID:23783074; http://dx.doi.org/10.1096/fj.12-214189
- Koike K, Ueda S, Yamagishi S, Yasukawa H, Kaida Y, Yokoro M, Fukami K, Yoshimura A, Okuda S. Protective role of JAK/STAT signaling against renal fibrosis in mice with unilateral ureteral obstruction. Clin Immunol 2014; 150:78-87; PMID:24333535; http://dx.doi.org/10.1016/j.clim.2013.11.003
- Serrano I, McDonald PC, Lock FE, Dedhar S. Role of the integrin-linked kinase (ILK)/Rictor complex in TGFbeta-1-induced epithelial-mesenchymal transition (EMT). Oncogene 2013; 32:50-60; PMID:22310280; http://dx.doi.org/10.1038/onc.2012.30
- Deng YL, Xiong XZ, Cheng NS. Organ fibrosis inhibited by blocking transforming growth factor-beta signaling via peroxisome proliferator-activated receptor gamma agonists. Hepatobiliary Pancreat Dis Int 2012; 11:467-78; PMID:23060391; http://dx.doi.org/10.1016/S1499-3872(12)60210-0
- Zhang YE. Non-Smad pathways in TGF-beta signaling. Cell Res 2009; 19:128-39; PMID:19114990; http://dx.doi.org/10.1038/cr.2008.328
- Leask A, Abraham DJ. TGF-beta signaling and the fibrotic response. FASEB J 2004; 18:816-27; PMID:15117886; http://dx.doi.org/10.1096/fj.03-1273rev
- Hanna C, Hubchak SC, Liang X, Rozen-Zvi B, Schumacker PT, Hayashida T, Schnaper HW. Hypoxia-inducible factor-2alpha and TGF-beta signaling interact to promote normoxic glomerular fibrogenesis. Am J Physiol Renal Physiol 2013; 305:F1323-31; PMID:23946285; http://dx.doi.org/10.1152/ajprenal.00155.2013
- Pohlers D, Brenmoehl J, Loffler I, Muller CK, Leipner C, Schultze-Mosgau S, Stallmach A, Kinne RW, Wolf G. TGF-beta and fibrosis in different organs - molecular pathway imprints. Biochim Biophys Acta 2009; 1792:746-56; PMID:19539753; http://dx.doi.org/10.1016/j.bbadis.2009.06.004
- Mallat A, Lotersztajn S. The liver X receptor in hepatic stellate cells: a novel antifibrogenic target? J Hepatol 2011; 55:1452-4; PMID:21782759; http://dx.doi.org/10.1016/j.jhep.2011.05.018
- Wang Z, Xu J, Zheng Y, Chen W, Sun Y, Wu Z, Luo M. Effect of the regulation of retinoid X receptor-alpha gene expression on rat hepatic fibrosis. Hepatol Res 2011; 41:475-83; PMID:21518404; http://dx.doi.org/10.1111/j.1872-034X.2011.00794.x
- Pan LH, Ohtani H, Yamauchi K, Nagura H. Co-expression of TNF alpha and IL-1 beta in human acute pulmonary fibrotic diseases: an immunohistochemical analysis. Pathol Int 1996; 46:91-9; PMID:10846556; http://dx.doi.org/10.1111/j.1440-1827.1996.tb03584.x
- Gieling RG, Wallace K, Han YP. Interleukin-1 participates in the progression from liver injury to fibrosis. Am J Physiol Gastrointest Liver Physiol 2009; 296:G1324-31; PMID:19342509; http://dx.doi.org/10.1152/ajpgi.90564.2008
- Sziksz E, Pap D, Lippai R, Beres NJ, Fekete A, Szabo AJ, Vannay A. Fibrosis Related Inflammatory Mediators: Role of the IL-10 Cytokine Family. Mediators Inflamm 2015; 2015:764641; PMID:26199463; http://dx.doi.org/10.1155/2015/764641
- Sun L, Louie MC, Vannella KM, Wilke CA, LeVine AM, Moore BB, Shanley TP. New concepts of IL-10-induced lung fibrosis: fibrocyte recruitment and M2 activation in a CCL2/CCR2 axis. Am J Physiol Lung Cell Mol Physiol 2011; 300:L341-53; PMID:21131395; http://dx.doi.org/10.1152/ajplung.00122.2010
- Fernandez IE, Eickelberg O. The impact of TGF-beta on lung fibrosis: from targeting to biomarkers. Proc Am Thorac Soc 2012; 9:111-6; PMID:22802283; http://dx.doi.org/10.1513/pats.201203-023AW
- Morrisey EE. Wnt signaling and pulmonary fibrosis. Am J Pathol 2003; 162:1393-7; PMID:12707021; http://dx.doi.org/10.1016/S0002-9440(10)64271-X
- Miao CG, Yang YY, He X, Huang C, Huang Y, Zhang L, Lv XW, Jin Y, Li J. Wnt signaling in liver fibrosis: progress, challenges and potential directions. Biochimie 2013; 95:2326-35; PMID:24036368; http://dx.doi.org/10.1016/j.biochi.2013.09.003
- Wells RG. The epithelial-to-mesenchymal transition in liver fibrosis: here today, gone tomorrow? Hepatology 2010; 51:737-40; PMID:20198628.
- Chapman HA. Epithelial-mesenchymal interactions in pulmonary fibrosis. Annu Rev Physiol 2011; 73:413-35; PMID:21054168; http://dx.doi.org/10.1146/annurev-physiol-012110-142225
- Borisov NM, Terekhanova NV, Aliper AM, Venkova LS, Smirnov PY, Roumiantsev S, Korzinkin MB, Zhavoronkov AA, Buzdin AA. Signaling pathways activation profiles make better markers of cancer than expression of individual genes. Oncotarget 2014; 5:10198-205; PMID:25415353; http://dx.doi.org/10.18632/oncotarget.2548
- Le TT, Karmouty-Quintana H, Melicoff E, Le TT, Weng T, Chen NY, Pedroza M, Zhou Y, Davies J, Philip K, et al. Blockade of IL-6 Trans signaling attenuates pulmonary fibrosis. J Immunol 2014; 193:3755-68; PMID:25172494; http://dx.doi.org/10.4049/jimmunol.1302470
- O'Reilly S, Ciechomska M, Cant R, van Laar JM. Interleukin-6 (IL-6) trans signaling drives a STAT3-dependent pathway that leads to hyperactive transforming growth factor-beta (TGF-beta) signaling promoting SMAD3 activation and fibrosis via Gremlin protein. J Biol Chem 2014; 289:9952-60; PMID:24550394; http://dx.doi.org/10.1074/jbc.M113.545822
- Kavvadas P, Kypreou KP, Protopapadakis E, Prodromidi E, Sideras P, Charonis AS. Integrin-linked kinase (ILK) in pulmonary fibrosis. Virchows Arch 2010; 457:563-75; PMID:20857141; http://dx.doi.org/10.1007/s00428-010-0976-7
- Khatri P, Sirota M, Butte AJ. Ten years of pathway analysis: current approaches and outstanding challenges. PLoS Comput Biol 2012; 8:e1002375; PMID:22383865; http://dx.doi.org/10.1371/journal.pcbi.1002375
- Jin L, Zuo XY, Su WY, Zhao XL, Yuan MQ, Han LZ, Zhao X, Chen YD, Rao SQ. Pathway-based analysis tools for complex diseases: a review. Genomics Proteomics Bioinformatics 2014; 12:210-20; PMID:25462153; http://dx.doi.org/10.1016/j.gpb.2014.10.002
- Venkova L, Aliper A, Suntsova M, Kholodenko R, Shepelin D, Borisov N, Malakhova G, Vasilov R, Roumiantsev S, Zhavoronkov A, et al. Combinatorial high-throughput experimental and bioinformatic approach identifies molecular pathways linked with the sensitivity to anticancer target drugs. Oncotarget 2015; 6:27227-38; PMID:26317900; http://dx.doi.org/10.18632/oncotarget.4507
- Artemov A, Aliper A, Korzinkin M, Lezhnina K, Jellen L, Zhukov N, Roumiantsev S, Gaifullin N, Zhavoronkov A, Borisov N, et al. A method for predicting target drug efficiency in cancer based on the analysis of signaling pathway activation. Oncotarget 2015; 6:29347-56; PMID:26320181.
- Lezhnina K, Kovalchuk O, Zhavoronkov AA, Korzinkin MB, Zabolotneva AA, Shegay PV, Sokov DG, Gaifullin NM, Rusakov IG, Aliper AM, et al. Novel robust biomarkers for human bladder cancer based on activation of intracellular signaling pathways. Oncotarget 2014; 5:9022-32; PMID:25296972; http://dx.doi.org/10.18632/oncotarget.2493
- Wang XM, Zhang Y, Kim HP, Zhou Z, Feghali-Bostwick CA, Liu F, Ifedigbo E, Xu X, Oury TD, Kaminski N, et al. Caveolin-1: a critical regulator of lung fibrosis in idiopathic pulmonary fibrosis. J Exp Med 2006; 203:2895-906; PMID:17178917; http://dx.doi.org/10.1084/jem.20061536
- Moylan CA, Pang H, Dellinger A, Suzuki A, Garrett ME, Guy CD, Murphy SK, Ashley-Koch AE, Choi SS, Michelotti GA, et al. Hepatic gene expression profiles differentiate presymptomatic patients with mild versus severe nonalcoholic fatty liver disease. Hepatology 2014; 59:471-82; PMID:23913408; http://dx.doi.org/10.1002/hep.26661