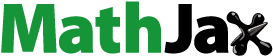
ABSTRACT
High throughput technologies opened a new era in biomedicine by enabling massive analysis of gene expression at both RNA and protein levels. Unfortunately, expression data obtained in different experiments are often poorly compatible, even for the same biologic samples. Here, using experimental and bioinformatic investigation of major experimental platforms, we show that aggregation of gene expression data at the level of molecular pathways helps to diminish cross- and intra-platform bias otherwise clearly seen at the level of individual genes. We created a mathematical model of cumulative suppression of data variation that predicts the ideal parameters and the optimal size of a molecular pathway. We compared the abilities to aggregate experimental molecular data for the 5 alternative methods, also evaluated by their capacity to retain meaningful features of biologic samples. The bioinformatic method OncoFinder showed optimal performance in both tests and should be very useful for future cross-platform data analyses.
Introduction
Next generation sequencing (NGS), Microarray hybridization (MH) and high throughput proteomic techniques opened a new era in biomedicine by enabling large-scale analysis of gene expression at both the RNA and protein levels.Citation1 Multiple experimental platforms based on different principles and using different reagents were developed for these tasks.Citation1 According to the International Aging Research Portfolio, over 8 billion dollars in government funding have been spent on research projects involving high throughput gene expression analysis since 1993.Citation2 This resulted in tens of thousands of publications. Unfortunately, gene expression data obtained using different experimental platforms are poorly compatible with each other even when obtained using the same biosamples. For example, a generally weak correlation between NGS and microarray gene expression data has been reported.Citation3 Therefore, a new data processing method is badly needed to enable data harmonization among different platforms and experiments.Citation4,Citation5
Recently we showed that aggregation of gene expression data into molecular pathways, each containing dozens or hundreds of gene products, may help to solve the problem of poor data compatibility among different experimental platforms.Citation3 NGS and microarray data obtained for the same transcripts showed generally low correlation (< 0.2) when examined at the level of individual genes. However, these correlations improved dramatically, up to 0.9, when activation of 90 molcular pathways was analyzed instead.Citation3 The output measure was a Pathway Activation Strength (PAS), which positively reflects the degree of pathway activation. The PAS makes it possible to quantity different processes such as molecular signaling, metabolism, DNA repair and cytoskeleton reorganization, based on gene expression data. These processes determine cell fate by governing growth, differentiation, proliferation, migration, survival and death.Citation6,Citation7 Molecular modeling of intracellular pathways has been performed for more than 2 decades.Citation8,Citation9 A plethora of molecular pathways have been discovered and cataloged, each containing different numbers of gene products.Citation10,Citation11 Pathway activation strength was also found to be a better marker of human tissue types,Citation12 Citation13 and tumor response to chemotherapy treatment.Citation14-16 Several approaches were published by us and others to assess the activation of signaling pathways, basing on large scale molecular dataCitation7,Citation17,Citation18 These methods take into account different factors like the extent of differential gene expression, architecture of molecular pathways, and the roles of individual gene products in a pathway (e.g., activator/repressor).Citation17,Citation18 For example, a method we used to minimize discrepancies between the NGS and microarray platforms, termed OncoFinder, relies on differential gene expression and the known roles in a pathway, but does not take into account pathway architecture, i.e. the position of a gene product in a pathway.Citation18
In spite of this progress, it is not known, what factors influences improvement of information stability after aggregation of gene expression profiles into pathway-based values for activation assessment. It is also unclear which bioinformatic algorithms provide better PAS outputs for cross-platform data stability. Additionally, PAS algorithms have not yet been applied to the high throughput proteomic data.
In this study, we applied data aggregation methods to transcriptomic information obtained using the Affymetrix HG U133 Plus 2.0, the Illumina HT12 bead array, the Agilent 1M array, the llumina Genome Analyzer platforms, and to proteomic data from the Orbitrap Velos and XL mass spectrometer platforms. We confirmed that for both transcriptomic and proteomic expression levels, the PAS approach provided more stable results than the expression of individual genes. To explain this phenomenon, we created a biomathematical model simulating error acquisition in individual gene expression and in PAS-based approaches. In agreement with the experimental data, in the mathematical model PAS methods produced significantly more stable results under most conditions. This model also predicts the optimal size of a molecular pathway and ideal parameters of the normalizing (control) set of gene expression data.
To make further tests for the predictions of our biomathematical model, we designed a new experimental gene expression array using the CustomArray microchip platform (USA), which enables direct electrochemical synthesis of oligonucleotide probes on a blank array. We compared results for the 7 human kidney cancer tissue samples independently profiled by the 2 laboratories on this customized array and on the commercial Illumina HT12 bead array platform. In agreement with the theoretical model, gene expression features differed significantly among the platforms for the same biosamples, while PAS values remained highly correlated. Therefore, gene expression data aggregated at the PAS level appears to be the method of choice for cross-platform data comparisons, including both transcriptomic and proteomic approaches.
We next explored the capacity of 5 most popular PAS calculation methods, OncoFinder,Citation18 TAPPA (Topology analysis of pathway phenotype association),Citation19 Topology-Based Score (TBScore),Citation20 Pathway-Express,Citation21 and SPIA (Signal pathway impact analysis)Citation22 to generate stable and biologically relevant data. We used the MicroArray Quality Control (MAQC) data setCitation4 that includes expression data for 4 biologic samples profiled in 15 replicates on major commercial microarray platforms. The abilities of the various PAS methods to increase correlation between transcriptomic features of the same biosamples examined using different experimental platforms were tested. We also checked whether different PAS scoring methods were able to retain biologic features after data harmonization using a generally accepted cross-platform harmonization procedure XPN.Citation23 We found that the OncoFinder method showed the optimal performance in both tests.
Results
Cross-platform processing of transcriptomic and proteomic data
We processed transcriptomic and proteomic data to establish pathway activation strength (PAS) profiles corresponding to intracellular molecular pathways. Our OncoFinder method for PAS calculation was shown to diminish the cross-platform variation between the MH and NGS data.Citation3 OncoFinder has previously been applied to many human and non-human systems including cell culture, leukemia and solid cancers, fibrosis, asthma, Hutchinson Gilford and Age-Related Macular Degeneration Disease.Citation24-27 The PAS for a given pathway (p) is calculated as follows,Citation18, , where the functional role of the nth gene product in the pathway is indicated by the activator/repressor role (ARR), which equals 1 for an activator, –1 for a repressor, and intermediate values -0,5; 0,5 and 0 for gene products having intermediate repressor, activator, or unknown roles, respectively. The CNRn value (case-to-normal ratio) is the ratio of the expression level of gene n in the sample under investigation to the average expression level in the control samples. A positive PAS value indicates activation of a pathway, and a negative value indicates repression.
In the current work, the OncoFinder-assisted analysis was performed with 271 molcular pathways (Supplementary table S1). The topological structure for these pathways was taken from the SABiosciences portal (http://www.sabiosciences.com/pathwaycentral.php), which is one of the best-annotated databases for signaling pathway topology; for convenience, large pathways were split into smaller functional sub-pathways that describe certain physiologic processes. The ARR flags for each gene/gene product in each pathway were set manually, according to their role in activation of the main effector gene product in such a pathway.
Building pathway activation profiles and assessment of batch effects
To identify if the OncoFinder technique may improve gene expression analysis by eliminating batch effects, we profiled a set of human clinical bladder cancer tissue samples using the same experimental platform (Illumina human HT 12 v4 bead arrays) in 2 different laboratories. We investigated gene expression profiles generated from 17 bladder cancer samples and 7 normal bladder tissue samples. Eight cancer and 4 normal samples were analyzed in Dr. Kovalchuk's laboratory in Lethbridge (Canada), and 9 cancer and 3 normal bladder tissue samples were analyzed in Dr. Buzdin's laboratory in Moscow (Russia). The gene expression data were deposited in the GEO database (http://www.ncbi.nlm.nih.gov/geo/) with accession numbers GSE52519 and GSE65635.
In agreement with previous reports,Citation28 the normalized gene expression showed significant batch effects with data from different laboratories clearly clustered on a Principal Component Analysis (PCA) plot (). However, the PAS data formed a single merged cluster (). The principle component variability was 4–6 times smaller for the PAS data ( and ).
Figure 1. Bladder carcinoma data sets assessed at the level of individual gene expression and pathway activation. (A) principal component analysis (PCA) plot for transcriptomes from data sets obtained in Russia (red dots) and Canada (black dots), at the level of individual gene expression. (B) PCA plot at the level of molecular pathway activation. (C) hierarchical clustering dendrogram of the data sets obtained in Russia (marked white) and Canada (marked blue), at the level of molecular pathway activation.
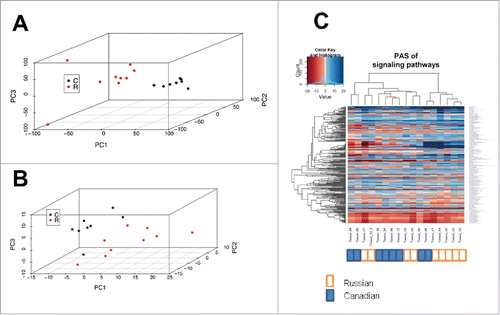
Similarly, using PAS values these 2 sets of samples formed mixed groups on a hierarchical cluster heatmap (). The Canadian samples were labeled 55 – 72; the Russian samples X1 – X8. Some sub-clusters are evidently formed by the samples coming from the different sets, e.g., by samples X5, X8, 69, 68 and X1. (). These data show that data aggregation at the PAS level is sufficient to suppress the batch effect in gene expression comparisons.
Mathematical modeling of data aggregation effects
We investigated the hypothesis that the apparently higher robustness of OncoFinder PAS scoring compared with single gene expression, is due to the cumulative nature of the former. PAS is the sum of multiple mathematical terms that correspond to each individual gene product participating in a pathway. Model calculations showed that this cumulative effect is able to reduce stochastic noise.
In the model, we included 271 pathways with variable numbers of gene products. We assumed that the expression level of every gene product could be measured using 2 different methods, say X and Y, corresponding to different experimental platforms (e.g., MH and NGS). Each method introduces errors into the determination of gene expression level, and these errors are independent. A Monte Carlo trial was performed as follows: we simulated both biased CNR (with a median value of 1.5) and unbiased CNR with a median value of 1; both biased and unbiased CNR values were distributed log-normally. We explored both noisy and exact expression profiling methods, to allow whether measurement procedures introduce errors in the true expression values. The 4 scenarios of the stochastic simulations (labeled A to D) are shown in .
Table 1. Cross-platform comparisons for modeling the data aggregation effect.
For each scenario, we calculated the benefit ratio , where Cp and Cg are the correlation coefficients between the results obtained using methods X and Y, using pathway-based (PAS), and individual gene product-based log CNR values, respectively. For each subset of genes in a pathway, we performed 100 Monte Carlo stochastic simulations and then computed the mean values of Cp and Cg using the R statistical package. The greater R > 1, the higher the benefit from using PAS instead of individual gene expression for the cross-platform comparisons; R < 1 means operating at the individual gene product level is better than the PAS level.
For biased expression profiles, scenarios A and B of , (), the PAS method shows much better agreement between the results obtained using different methods, compared with the individual gene expression levels. The data aggregation advantage of PAS is especially strong when both expression methods are noisy (scenario A). In scenario B, when one method is exact, the benefit of pathway data aggregation is lower. This is caused mainly by higher expression correlation already at the level of individual gene products (). However, the advantages of PAS remain considerable for pathways that contain at least 10 gene products (). For shorter pathways, the data aggregation effect is gradually decreased, and the R ratio reflecting the benefit of using PAS values, trends toward 1.
Figure 2. Ratio of pathway-related and gene-related correlation coefficients between results obtained using hypothetical methods X and Y, as a function of the median gene number, N, in a pathway for 4 scenarios: (A, blue) – biased expression profile, noisy method Y; (B, red) – biased expression profile, exact method Y; (C, green) – unbiased expression profile, noisy method Y; (D, magenta) – unbiased expression exact method Y. The method X is always condsidered noisy
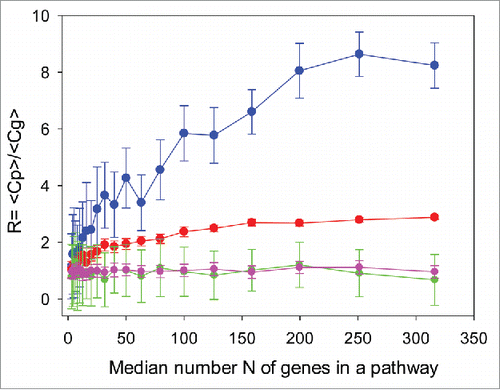
Figure 3. Distributions of values obtained during random trials using 2 different expression profiling methods X (horizontal axis) and Y (vertical axis). Median number of gene products in a pathway is 100. Left column: logCNR for individual gene products, method Y vs method X. Right column: PAS scoring method Y vs method X. Blue dots: scenario A (biased expression profile, noisy method Y). Red dots: scenario B (biased expression profile, exact method Y). Green dots: scenario C (unbiased expression profile, noisy method Y). Magenta dots: scenario D (unbiased expression profile, exact method Y). Method X is always considered noisy.
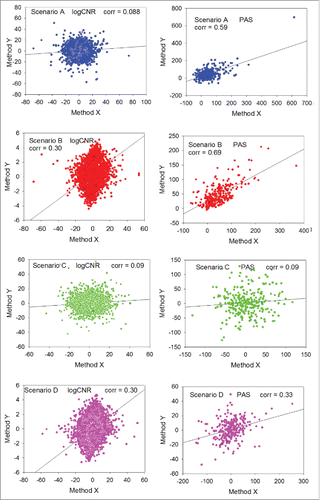
For unbiased transcription profiles, with median relative gene expression levels equal to 1, the data aggregation effect is completely lost (scenarios C and D). Here, the mean value for each gene product component of the PAS score is zero; consequently, the expected PAS is also zero, and the relative data variation is the same at the gene product and the PAS level.
The simulations clearly elucidate how the cumulative nature of PAS suppresses cross-platform data variation and batch effects. They show that there is a significant advantage of using PAS to compare platforms, when at least one platform is noisy. This should apply to most if not all existing high throughput experimental platforms, and it should be seen when experimental expression data are compared. The simulations demonstrate that PAS calculations are advantageous for biased transcriptomes and proteomes and virtually useless for unbiased ones. Unbiased data sets are too similar to the control group used as the reference to calculate CNR values. This means that the PAS approach will be especially useful when the expression signature in the sample under study is very different from that of the control samples. This finding may help to identify appropriate control samples for decreasing expression data noise. Finally, this model shows that the higher is the number of gene products in a pathway, the greater the benefit of shifting from individual gene/protein expression to PAS data For example, the mean number of gene products in the OncoFinder database is 68 per pathway, and the model predicts about a 4.5–fold decrease in data variation at the PAS level in the biased noisy-noisy scenario, which may explain the success of the OncoFinder approach in various applications.Citation3
Experimental model of cross-platform comparisons
In transcriptomic methods, batch effects arise from errors introduced at the stages of RNA purification, library preparation and amplification, hybridization and reading of arrays.Citation29 We investigated whether the OncoFinder PAS algorithm can suppress batch effects introduced by cross-platform comparisons. At the same time, we assessed if the algorithm works efficiently for formalin-fixed, paraffin-embedded (FFPE) tissue samples. Seven FFPE tissue blocks isolated from human renal carcinomas were profiled using 2 independent experimental platforms. The first was the Illumina HT 12 v4 bead array system optimized for FFPE tissues. The second was a customized microchip system developed using the CustomArray (USA) technology of direct on-chip electrochemical oligonucleotide synthesis. The custom arrays had 3775 oligonucleotide probes corresponding to 2214 human gene products involved in 271 intracellular signaling pathways (Supplementary table S1, sheet PAS_renal). The custom arrays, used the original oligonucleotide probe sequences of the Illumina HT 12 v4 platform, but shortened by 5 nucleotides at the 5′ end and by 5 nucleotides at the 3′ end. Quantile-normalized gene expression data were deposited into the GEO database with the accession numbers GSE65637 and GSE65639. The differences between the Illumina and the Custom platforms included shorter oligonucleotide probe sequences, different library preparation protocols and different hybridization signal development and reading methods (Supplementary Fig. S1). The Custom method for library preparation for FFPE tissue profiling was quite distinct from Illumina and identical to that used by the Agilent MH platform (Supplementary Fig. S1B, C, and E) with the sole exception that biotinylated rather than fluorescently labeled DNA is used at the terminal stage (Supplementary Fig. S1B and E).
To compare with the renal carcinoma samples, we used GEO data set GSE4997230 containing 6 normal kidney samples to normalize the expression data and calculate PAS. The normalized CNR expression data and PAS values are shown on Supplementary table S1, sheets PAS_Renal and logCNR_Renal. At the level of individual gene products, we observed relatively low correlations (0.2–0.3) between the same transcriptomes profiled using the 2 platforms (; Supplementary table S2). In contrast, at the PAS level the correlations were strong, varying from 0.84 to 0.91 (; Supplementary table S2).
These results experimentally confirm the hypothesis that data aggregation at the PAS level increases the stability of cross-platform expression data and that the advantage of PAS is retained for FFPE samples.
Data aggregation effects assessed on different RNA and protein expression profiles
We investigated quantitative aspects of the effect of data aggregation on several data sets where the same samples were profiled using different expression platforms (, Supplementary table S2, referencesCitation31-36).
Table 2. Transcriptomic and proteomic data sets used to assess data aggregation effects.
We observed 2 trends for the behavior of the benefit ratio . In model calculations, we observed a crucial role of expression profile bias between the case and normal samples for successful data aggregation of genes into pathways (, ). We introduce a measure of such bias, termed
, where
and
are the mean and standard deviation, respectively, of the set of log CNR values obtained for a given sample using the experimental platform i. The results of the model calculation ( and , scenarios A and B) suggest that, even for the same values of β the ratio R may be different depending on Cg (correlation at the individual gene product level): the higher is Cg, the lower is the ratio R at equal β.
With a discrimination threshold for Cg chosen as equal to 0.25 between low-correlated and the considerably correlated samples, we can see the clear clusters of data for aggregation effect (, blue dots for low and red dots for considerably correlated samples. Note that the 2 clusters of data depending on the Cg threshold are seen for both transcriptome-to-transcriptome and transcriptome-to-proteome comparisons.
The data obtained suggests that when β is low, the R is hardly distinguishable from 1; however, when β exceeds a threshold, the increase of R becomes statistically significant. Finally, these results also demonstrate that transcriptomic and proteomic profiles demonstrate more compatible results at the molecular pathway level rather than on the level of individual gene products.
Comparison of PAS scoring methods according to their capacities in data aggregation
We compared the abilities of 5 popular PAS scoring methods to express an advantageous data aggregation effect when the activation of molecular pathways is compared instead of individual gene products. For the 7 renal carcinoma samples discussed above, we calculated R using alternative PAS scoring methods: OncoFinder,Citation18 topology analysis of pathway phenotype association, TAPPA,Citation19 topology-based score (TB),Citation20 pathway-express (PE),Citation21 and signaling pathway impact analysis (SPIA)Citation22 methods (Supplementary tables S3, S4). These methods differ in the factors used to evaluate the importance of distinct gene products in pathway activation.
Only 3 of the methods, OncoFinder, PE and SPIA, showed a substantial data aggregation effect (R) ranging from 2–2.3. Other methods showed lack of any positive effect ().
Different methods for PAS scoring show different properties in retention of biologic features
Cross-platform data comparison has the potential to become an extremely useful tool in contemporary biomedicine and bioinformatics. Although the application of PAS methods has the ability to restore correlations between different expression data sets, the absolute values of PAS may differ between platforms. To overcome this inconsistency, several cross-platform harmonizationFootnote1
Figure 4. Correlation between transcriptomic data obtained for the same representative renal carcinoma specimen using the Illumina HT12 (ordinate) and CustomArray (abscissa) microarray platforms. The panels represent (from left to right) correlation between the oligonucleotide expression tags, correlations at the level of individual genes, and correlation at the level of molecular pathways.
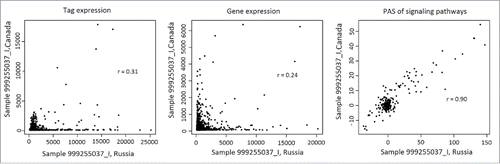
We used the results of the Microarray quality control project (MAQC)Citation4 as a model data set for this study. The MAQC project investigated 4 types of samples (A-D; each sample profiled in 15 technical replicates) using different microarray devices. Type A samples were taken from the Stratagene Universal Human Reference RNA; type B samples – from the Ambion Human Brain Reference RNA. Type C and D samples were obtained by combining samples A and B in mass ratios 75:25 for C, and 25:75 for D, respectively.
After XPN harmonization of gene expression profiling using the Agilent Whole Human Genome Oligo and Affymetrix Human Genome U133 Plus 2.0 platforms, we applied different methods of PAS scoring (Supplementary table S5) using the samples of type A as normal. The probability densities of the Euclidean distances between the PAS vectors calculated for the 3 samples (B, C, and D) differ greatly depending on the PAS scoring method used (). In such an assay, an ideal PAS scoring method should make distinctions between samples depending primarily on the sample types, rather than on the experimental platform used. A satisfactory PAS calculation method, therefore, should demonstrate a unimodal distribution of the PAS-PAS distances, without any significant deviations. If the distribution of PAS-PAS distances is bimodal or multimodal, this points to the inability to eliminate platform-specific bias even at the pathway level. Only the OncoFinder and TAPPA methods were able to eliminate the cross-platform bias for all 3 sample types ().
Figure 5. Dependence of the data aggregation effect (R) on the minimal expression profile bias β. Left panel: transcriptome-to-transcriptome comparisons for the same samples using different experimental platforms. Right panel: transcriptome-to-proteome comparisons for the same samples. The Cg threshold between the samples low and considerably correlated at the gene level was chosen as equal to 0.25; blue dots: low correlation at gene product level; red dots: considerable correlation at gene product level.
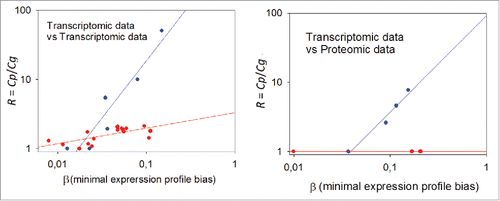
Figure 6. Data aggregation effect R for 5 pathway activation scoring methods (OncoFinder (OF), TAPPA, TBScore (TB), Pathway-Express (PE), and SPIA) on the renal carcinoma data set.
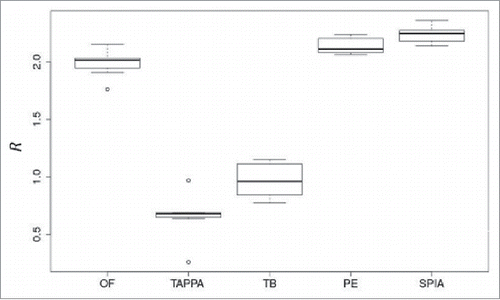
Figure 7. Distribution of Euclidean distances between the PAS vectors for different sample types taken from the MAQC data set (marked as B, C, and D) using different methods of PAS scoring. A unimodal distribution indicates lack of significant difference between within-platform and cross-platform distances. A bimodal distribution means that the cross-platform PAS distance (upper mode in the violin plots) is essentially higher that the within-platform distance. See text for descriptions of the different scoring methods.
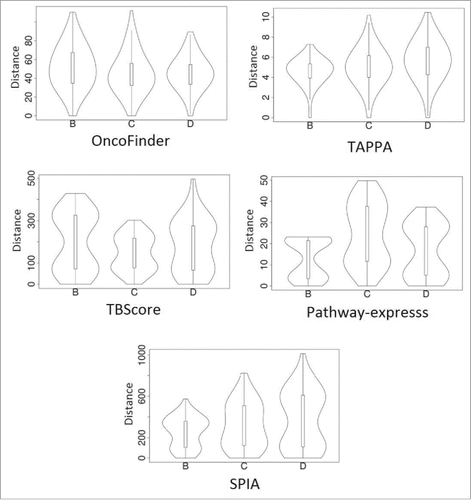
Hierarchical clustering (dendrograms shown in Supplementary Fig. S2) demonstrates that only the OncoFinder and TAPPA methods enabled clustering of the PAS vectors exclusively according to biologic sample type. Thus, among the 5 PAS scoring algorithms tested, only OncoFinder showed effective data aggregation with efficient retention of biologic information in 3 independent tests ().
Discussion
High throughput gene expression may produce both random and systematic errors, arising from the steps in RNA or protein purification, library preparation and/or amplification, hybridization, sequencing, mass spectrometry, reading arrays, and mapping and annotation of the readsCitation29,Citation39-42 It is generally hard to identify the types of errors and to find out which kind of experimental protocol provides more reliable data. While the measured concentration of each individual gene product may be in error, we show in this report that combining sufficient numbers of these concentrations into a pathway-oriented framework apparently generates significantly more stable data. We also tested whether OncoFinder and other PAS scoring methods can improve expression data to suppress batch effects, the unwanted variation in gene expression measurements on the same experimental platform made at different times, which frequently originate from the limitation in the number of samples that can be processed at once in a single experiment.Citation43 Batch effects also hinder the combination of different experimental data sets. Batch effects are almost inevitable.Citation28 By limiting analyses to single data sets, one frequently must use an insufficient number of samples, which leads to high false-negative rates.Citation28 Eliminating batch effects enables larger data sets, and provides more statistical power to subsequent analyses.Citation28
Here, using the Illumina HT12 bead array platform to profile human cancer samples, we demonstrate that the PAS scoring technology OncoFinder effectively suppresses batch effects present in the individual gene expression measurements (). OncoFinder efficiently increases expression data stability from all major experimental platforms, for both fresh and formalin-fixed, paraffin-embedded tissue samples ().
Various publicly available repositories of gene expression data embrace the full spectrum of normal and pathological conditions for the majority of known human diseases.Citation44,Citation45 Unfortunately, batch effects, which bias the expression profiles, hamper the joint analysis of most of this data obtained using different experimental settings.
Discrepancies in data obtained on the same and different experimental platforms, must be addressed by different methods, termed normalization and harmonization, respectively. For intra-platform normalization, more attention is paid to equilibration of scaling factors, while cross-platform harmonization must address the type of distribution of output intensities for each gene. Exiting methods for intra-platform normalization include quantile normalizationCitation46 and frozen robust multi-array analysis (FRMA)Citation47 for microarray data, and the DESeq methodCitation48 for next-generation sequencing.
Methods for cross-platform harmonization, such as distance-weighted discrimination (DWD),Citation49 cross-platform normalization (XPN),Citation23 and platform-independent latent Dirichlet allocation (PLIDA),Citation50 provide deep restructuring or signal intensity redistribution for the entire set of genes profiled. As a rule, the cross-platform harmonization involves data clustering and finding similarity regions among results obtained using different platforms, to strengthen similarity during the harmonization process.
Unfortunately, current normalization and harmonization methods hardly distinguish between artifacts introduced by batch effects and the real biologic differences. Additional tools are needed to improve normalization and harmonization procedures. We demonstrate here for most major transcriptomic and proteomic commercial platforms that data aggregation at the level of molecular pathways has the potential to reduce greatly the bias in the data sets under comparison. Since each pathway may contain hundreds of different gene products, transition from single gene products to the whole pathway level may restore biologically significant correlations.
We propose a term data aggregation effect for such restoration of biologic correlation at the pathway level. We created a mathematical model that simulates it and identifies the necessary conditions for its applicability. Sample expression profiles must be biased compared with control samples, i.e., the transcriptional signatures of the case samples must differ significantly from the normal ones (). The strength of the data aggregation effect grows with the number of gene products in a molecular pathway. The data aggregation effect is especially strong when the initial correlation between the expression data are weak ( and ). Finally, the choice of PAS scoring method affects the data aggregation effect. On a model data set, the OncoFinder, Pathway-Express and SPIA algorithms result in a considerable data aggregation effect, while TAPPA and TB-Score don't (). Only OncoFinder and TAPPA were able to preserve the biologic features on the model data set MAQC after cross-platform harmonization, while with Pathway-Express, SPIA and TB-Score methods, platform-introduced bias features still dominated the output expression signatures (). Thus, among the 5 PAS scoring methods tested here, the OncoFinder algorithm showed the best efficiency and accuracy (), which makes OncoFinder a method of choice for many applications using high-throughput analysis of gene expression at the RNA or protein levels.
Table 3. Comparison of PAS scoring methods using functional and statistical tests.
Of course, from one hand such an aggregation effect is not unexpected, taking into account the law of large numbers and central limit theorem, which are well-known for a few centuries. However, as we found in this study, different pathway activation scoring methods demonstrate very different performance.
Another concern may be addressed to the fact that transition from gene-based values to pathway-based ones inevitably causes irreversible loss of information. Indeed, so far not all genes have been attributed to certain pathways. However, if the high throughput gene-based information is still insufficiently reliable, then accumulation of such information within the molecular pathways is clearly beneficial.
In this study, we used the pathway structure database based on the SABioscience portal that includes 2426 individual gene products. To the date, this is still far from covering the full repertoire of protein-coding genes. However, in the future applications this part of the genome will grow with the progress of molecular interactomics. Nevertheless, we have demonstrated that gene expression data aggregation works efficiently for already-established molecular pathways. To clarify if this effect is linked with the physiologic coordination of gene products, in the future it would be important to compare these results with the randomly generated pathway-like gene sets.
In the future, it should also be possible to refine PAS methods to create universal platform-agnostic analytic tools. These tools have a huge potential to accelerate progress in genetics, physiology, biomedicine, molecular diagnostics and other applications by combining unbiased data from many sources and various experimental platforms.
Materials and methods
Ethics statement
The involvement of human subjects in the current work is not considered clinical research as defined by Russian Federal Service for Surveillance in Healthcare (Roszdravnandzor) and Canadian National Institute of Heath. All patients involved in the research have given the informed consent for the use of the bladder and renal cancer samples for this non-research. All the experimental methods were performed in accordance with the relevant guidelines.
Tissue collection and RNA isolation from fresh biosamples
Seven normal bladder and 17 bladder carcinoma specimens from patients treated at the P.A. Herzen Moscow Oncological Research Institute (HMORI; Moscow, Russia) were analyzed. Of these samples (cancer/normal), 9/3 were examined at the Shemyakin-Ovchinnikov Institute of Bioorganic Chemistry (IBC; Moscow, Russia) and 8/4 at the University of Lethbridge (UL; Alberta, Canada). All patients provided written informed consent to participate in this study. This study was approved by the local ethical committees at IBC, UL and HMORI. Tumor samples were obtained from patients who had undergone surgery for bladder carcinoma at the HMORI between 2009 and 2013. The median age of the cancer patients at the time of surgical tumor resection was 64 y (range 48–77 years). Tissue samples from non-cancer controls were collected from autopsies at the Department of Pathology at the Faculty of Medicine, Moscow State University. Both the tumors and normal tissues were evaluated by a pathologist to confirm the diagnosis and estimate the tumor cell numbers. All tumor samples used in this study contained at least 80% tumor cells. The median age of the healthy tissue donors was 45 y (range 20–71 years). Tissue samples were stabilized in RNAlater (Qiagen, Germany) and then stored at −80°C. Frozen tissue was homogenized in TRIzol Reagent (Life Technologies, USA), and RNA was isolated following the manufacturer's protocol. Purified RNA was dissolved in RNase-free water and stored at −80°C.
Microarray profiling of gene expression in fresh biosamples
Total RNA was extracted using TRIzol Reagent and then reverse-transcribed to cDNA and cRNA using the Ambion TotalPrep cRNA Amplification Kit (Invitrogen, USA). The cRNA concentration was quantified and adjusted to 150 ng/ml using an ND-1000 Spectrophotometer (NanoDrop Technologies, USA). 750 ng of each RNA library was hybridized onto the bead arrays.
Gene expression experiments were performed by Genoanalytica (Moscow, Russia) and the O. Kovalchuk Laboratory (Lethbridge, Canada) using the Illumina HumanHT-12v4 Expression BeadChip (Illumina, Inc.). This gene expression platform contains more than 25,000 annotated genes and more than 48,000 probes derived from the National Center for Biotechnology Information RefSeq (build 36.2, release 22) and the UniGene (build 199) databases. The expression data were deposited in the GEO database (http://www.ncbi.nlm.nih.gov/geo/), accession numbers GSE52519 and GSE65635.
Synthesis of microarrays
A B3 synthesizer (CustomArray, USA) was used for oligonucleotide probe synthesis on the CustomArray ECD 4 × 2K/12K slides. Synthesis was performed according to the manufacturer's recommendations. At least 3 replicates of total 3823 unique oligonucleotide probes of 40 nucleotides in length for 2278 genes were placed on each chip.
Library preparation and hybridization
RNA was extracted from freshly frozen tissue samples or samples stored in stabilizing buffer solutions using the standard protocol for TRIzol reagent (Life Technologies). RNA extraction from FFPE samples was performed using the RecoverAll™ Total Nucleic Acid Isolation Kit for FFPE.
Complete Whole Transcriptome Amplification WTA2 Kit (Sigma) was used for reverse transcription and library amplification. The manufacturer's protocol was modified by adding to amplification reaction a dNTP mix containing biotinylated dUTP, resulting to a final proportion dTTP/biotin-dUTP of 5:1.
Hybridization was performed according to the CustomArray ElectraSense™ Hybridization and Detection protocol. The hybridization mix contained 2.5 ug of labeled DNA library, 6X SSPE, 0.05% Tween-20, 20mM EDTA, 5x Denhardt solution, 100 ng/ul sonicated calf thymus gDNA, and 0,05% SDS. The chip was incubated in the hybridization mix overnight at 50°C. The hybridization efficiency was detected electrochemically using CustomArray ElectraSense™ Detection Kit and ElectraSense™ 4 × 2K/12K Reader. The chip was designed using the Layout Designer software (CustomArray, USA).
Functional annotation of gene expression data
The SABiosciences (http://www.sabiosciences.com/pathwaycentral.php) signaling pathways knowledge base was used to determine structures of intracellular pathways, as described previously.Citation51
OncoFinder
We applied the original OncoFinder algorithmCitation18 for functional annotation of the primary expression data and for calculating PAS scores. The microarray gene expression data were quantile normalized according to Bolstad et al.Citation40 The formula used to calculate the PAS for a given sample and a given pathway p is as follows:
Here the case-to-normal ratio, CNRn, is the ratio of the expression level of gene n in the sample under investigation to the average expression level of that gene in the control group of samples. The Boolean flag of BTIF (beyond tolerance interval flag) equals one or zero when the CNR value has simultaneously passed or not passed, respectively, the 2 criteria that indicate a significantly perturbed expression level from an essentially normal expression level. The first criterion is that the expression level of the sample lies within the tolerance interval, with p < 0.05. The second criterion is whether the CNR value lies outside the cut-off limits, i.e., either CNR < 2/3 or CNR > 3/2. ARRnp, the discrete value of the activator/repressor role equals the following fixed values: −1, when the gene/protein n is a repressor of molecular pathway; 1, if the gene/protein n is an activator of pathway; 0, when the gene/protein n is known to be both an activator and a repressor of the pathway; and 0.5 and −0.5, respectively, tends to be an activator or a repressor of the pathway p, respectively.
Our approach to calculations of PAS implies 2 principal assumptions:
1. | Computational modeling of signal transduction processesCitation52-54 indicates that for most interacting proteins the concentration of their active forms, which are sufficient for downstream signaling, is much lower than the total abundance of the corresponding protein. In other words, signal transduction may be performed even at the very low level for most gene products. | ||||
2. | We stipulate that each pathway graph may be simplified up to the following structure that includes only 2 chain-like (linear) branches: one for sequential events that promote activation of whole pathway, and another for repressor sequential events. The adequacy of this quite radical approximation was shown before in comparison with the full-scaled kinetic model,Citation54 when all protein-protein interactions were described using the mass-action law along each edge of a highly branched pathway graph.Citation18 |
Under these conditions, we presume that all activator/repressor members have equal importance for the whole pathway, and come to the following formula for the overall signal outcome (SO) of a given pathway, . Here the multiplication is done over all possible activator and repressor proteins in the pathway, [AGEL]i and [RGEL]j are relative gene expression levels of activator (i) and repressor (j) members, respectively. To obtain an additive value, it is possible to take the logarithmic levels of gene expression, and thus come to a function of PAS.
The results for 271 pathways were obtained for each sample (see Supplementary table S1). Statistical tests used the R software package.
TAPPA (Topology analysis of pathway phenotype association)
Imagine а pathway graph, , where
is the set of graph nodes (vertices), and
is the set of graph edges.Citation19 The adjacency matrix is defined as follows,
, if
or
, and
, if
. A centered Z-scoring procedure was applied to the logarithmic gene expression matrix,
. The adjacency index for a pathway is defined as follows,
(2)
(2) where
is the number of genes in the pathway, and the double summation of over the
reveals whether the pathway has more up- or downregulated genes. The sign of xis + xjs indicates whether the whole pathway is up- or downregulated is calculated as
where
is the expected value of J over the set of samples that are considered normal.
TBScore (Topology-based score)
For a pathway p that has N nodes, the valueCitation20 , where the node value, NV, equals to zero if all the genes in the node i are non-differential genes, or equals to the sum of log-fold-changes of the differential genes in the node i. The gene is considered differentially expressed according to the state of the Boolean flag BTIF (as for the OncoFinder algorithm). The node weight, NWi, equals the number of downstream nodes for node i. To determine the value of NWi, we used the depth-first search methodCitation55 with labeling visited nodes to avoid the infinite cycling.
Pathway-Express (PE)
The PE-score for a pathway K was calculated as follows,Citation21,
The first term in this sum is the p-value for the probability to obtain the observed or a higher number Nd of differentially expressed genes (between the pools of case and normal samples) by random chance, assuming a hypergeometrical distribution for Nd. The second term is a summation over the perturbation factors for the all genes g of the pathway K,
Here is the signed difference of gene
logarithmic expression in a given sample compared with the expected value for the pool of normal samples. The latter term expresses the summation over all the genes γ that belong to the set Ug of the upstream genes for the gene g. The value of ndown (γ) denotes the number of downstream genes for gene γ. The weight factor
indicates the interaction type between γ and g:
if γ activates g, and
when γ inhibits g. Although the value of PF may be positive or negative, the overall score of PE is obligatory positive. The search for upstream/downstream genes is performed according to the depth-first search method, as in the TBScore method.
SPIA (Signal pathway impact analysis)
To obtain an estimator for pathway perturbation that is positive for an upregulated and negative for a downregulated pathway, use the second term in formula for the perturbation factor (PF) from the precious paragraph, resulting in the accuracy value, It can be shown that this accuracy vector may be expressed as follows,Citation22,
where
I is the identity matrix, and
The overall score for pathway pertubation calculated as: .
Statistical tests
Principal component analyses were performed using the MADE4 package.Citation56 Hierarchical clustering heat maps with Pearson distances and average linkage were generated using heatmap.2 function from the gplots package.Citation57
Mathematical modeling
We performed a Monte Carlo trial to investigate the data aggregation effect. We assumed that the number of genes in each pathway is distributed log-normally with the variable median number N. The case-to-normal-ratio (CNR) values for each gene were also sampled from the log-normal law, so that the value of log CNR had a normal distribution. When sampling CNR, we distinguished between biased and unbiased models of gene expression. For the biased model, the CNR distribution has a median value of 1.5, whereas for the unbiased model, the median CNR value is 1. The standard deviation of the mean log CNR value was set to 0.3 for both biased and unbiased models. The independent error produced by an experimental platform was also sampled stochastically. We simulated both the exact and noisy expression profiling methods. By the definition, exact methods did not introduce errors. For noisy methods, the error was chosen from the log-normal distribution, with a median value of 1.0. All the calculations were made using the R open source platform (version 3.1.2).
Analysis of published transcriptomic and proteomic data sets
Prior to analysis, all the microarray data were quantile normalized,Citation44 and the RNA-seq data were normalized using the DESeq package from Bioconductor software.Citation46 All gene products showing zero intensities were skipped to avoid aberrant data variations. Pearson correlation coefficients between the same samples examined using different expression profiling methods (e.g., proteome vs transcriptome or MH vs NGS) were calculated at 2 levels of data aggregation: first, at the level of distinct genes and gene products – namely for the value of log CNR (the so-called Cg correlation value); and, second, at the level of the whole pathways, for the PAS value (the Cp correlation coefficient). Then, the ratio was calculated for each sample.
Analysis of biologic relevance after cross-platform harmonization
Transcriptional profiles were obtained using the Agilent Whole Human Genome Oligo and Affymetrix Human Genome U133 Plus 2.0 array platforms. The transcriptomic data were cross-platform harmonized with the XPN methodCitation23 using the R package CONOR.Citation58 Then, the cross-harmonized (between the Agilent and Affymetrix platforms) gene expression profiles were used as the input data for the PAS calculations. For all the calculations, type A samples were used as normal, and type B, C and D samples – as cases.
Euclidean distances between the PAS vectors were used to determine whether the resulting PAS samples are grouped in agreement with their biologic properties (i.e., biologic sample types B, C and D compared with A), or according to the experimental platform used to investigate them (i.e., Agilent or Affymetrix microarray platform). The cluster dendrograms and violin plots were drawn using the R packages dendextend and vioplot, respectively.
Disclosure of potential conflicts of interest
No potential conflicts of interest were disclosed.
KCCY_S_1361068.zip
Download Zip (5.2 MB)Acknowledgements
The work was supported by the internal research grant of National Research Centre “Kurchatov Institute”, Moscow, Russia, as well as by the Presidium of the Russian Academy of Sciences program “Biodiversity”. The authors thank the First Oncology Research and Advisory Center (Moscow, Russia) for the support in preparation of this manuscript. We would like to thank Alex Kim and ASUS for equipment and support of this research.
Notes
1 In the current article, we apply the term normalization to any method for within-platform batch effect elimination, and harmonization when such procedure is performed for the cross-platform comparison, although the mathematical methods for both the former and latter procedures may be different.
References
- Kumar D, Bansal G, Narang A, Basak T, Abas T, Dash D. Integrating transcriptome and proteome profiling: Strategies and applications. Proteomics. 2016;6:2533-44. PMID:27343053. doi:10.1002/pmic.201600140
- Zhavoronkov A, Cantor CR. Methods for structuring scientific knowledge from many areas related to aging research. PLoS One. 2011;6:e22597. doi:10.1371/journal.pone.0022597. PMID:21799912
- Buzdin AA, Zhavoronkov AA, Korzinkin MB, Roumiantsev SA, Aliper AM, Venkova LS, Smirnov PY, Borisov NM. The OncoFinder algorithm for minimizing the errors introduced by the high-throughput methods of transcriptome analysis. Front Mol Biosc. 2014;1:8. PMID:25988149. doi:10.3389/fmolb.2014.00008
- MAQC Consortium. The MicroArray Quality Control (MAQC) project shows inter- and intra-platform reproducibility of gene expression measurements. Nat Biotechnol. 2006;24:1151-61. PMID:16964229
- Zhang L, Zhang J, Yang G, Wu D, Jiang L, Wen Z, Li M. Investigating the concordance of Gene Ontology terms reveals the intra- and inter-platform reproducibility of enrichment analysis. BMC Bioinformatics. 2013;14:143. doi:10.1186/1471-2105-14-143. PMID:23627640
- Diederich M, Cerella C. Non-canonical programmed cell death mechanisms triggered by natural compounds. Semin Cancer Biol. 2016;S1044-579X:30021-29. PMID:27262793. doi:10.1016/j.semcancer.2016.06.001
- Zhavoronkov A, Buzdin AA, Garazha AV, Borisov NM, Moskalev AA. Signaling pathway cloud regulation for in silico screening and ranking of the potential geroprotective drugs. Front Genet. 2014;5:49. doi:10.3389/fgene.2014.00049. PMID:24624136
- Kholodenko BN, Demin OV, Moehren G, Hoek JB. Quantification of short term signaling by the epidermal growth factor receptor. J Biol Chem 1999;274:30169-81. PMID:10514507. doi:10.1074/jbc.274.42.30169
- Hanahan D, Weinberg RA. The hallmarks of cancer. Cell. 2000;100:57-70. doi:10.1016/S0092-8674(00)81683-9. PMID:10647931
- Croft D, Mundo AF, Haw R, Milacic M, Weiser J, Wu G, Caudy M, Garapati P, Gillespie M, Kamdar MR et al. The Reactome pathway knowledge base. Nucleic Acids Res. 2014;42:D472-77. doi:10.1093/nar/gkt1102. PMID:24243840
- Nakaya A, Katayama T, Itoh M, Hiranuka K, Kawashima S, Moriya Y, Okuda S, Tanaka M, Tokimatsu T, Yamanishi Y et al. KEGG OC: a large-scale automatic construction of taxonomy-based ortholog clusters. Nucleic Acids Res. 2013;41:D353-57, doi:10.1093/nar/gks1239. PMID:23193276
- Borisov NM, Terekhanova NV, Aliper AM, Venkova LS, Smirnov PY, Roumiantsev S, Korzinkin MB, Zhavoronkov AA, Buzdin AA. Signaling pathways activation profiles make better markers of cancer than expression of individual genes. Oncotarget. 2014;5:10198-205. doi:10.18632/oncotarget.2358. PMID:25415353
- Lezhnina K, Kovalchuk O, Zhavoronkov AA, Korzinkin MB, Zabolotneva AA, Shegay PV, Sokov DG, Gaifullin NM, Rusakov IG, Aliper AM. Novel robust biomarkers for human bladder cancer based on activation of intracellular signaling pathways. Oncotarget. 2014;5:9022-32. doi:10.18632/oncotarget.2493. PMID:25296972
- Zhu Q, Izumchenko E, Aliper AM, Makarev E, Paz K, Buzdin AA, Zhavoronkov AA, Sidransky D. Pathway activation strength is a novel independent prognostic biomarker for cetuximab sensitivity in colorectal cancer patients. Hum Genome Var. 2015;2:15009. doi:10.1038/hgv.2015.9. PMID:27081524
- Venkova L, Aliper A, Suntsova M, Kholodenko R, Shepelin D, Borisov N, Malakhova G., Vasilov R, Roumiantsev S, Zhavoronkov A, Buzdin A. Oncotarget. 2015;6:27227-32728. doi:10.18632/oncotarget.4507. PMID:26317900
- Artemov A, Aliper A, Korzinkin, M, Lezhnina K, Jellen L, Zhukov N, Roumiantsev S, Gaifullin N, Zhavoronkov A, Borisov N, Buzdin A. A method for predicting target drug efficiency in cancer based on the analysis of signaling pathway activation. Oncotarget. 2015;6:29347-56. doi:10.18632/oncotarget.5119. PMID:26320181
- Khatri P, Sirota M, Butte AJ. Ten years of pathway analysis: current approaches and outstanding challenges. PLoS Comput Biol. 2012;8:e1002375. doi:10.1371/journal.pcbi.1002375. PMID:22383865
- Buzdin AA, Zhavoronkov AA, Korzinkin MB, Venkova LS, Zenin AA, Smirnov PY, Borisov NM. Oncofinder, a new method for the analysis of intracellular signaling pathway activation using transcriptomic data. Front Genet. 2014;5:55. doi:10.3389/fgene.2014.00055. PMID:24723936
- Gao, S, Wang X. TAPPA: topological analysis of pathway phenotype association. Bioinformatics. 2007;23:3100-02. doi:10.1093/bioinformatics/btm460. PMID:17890270
- Ibrahim MA, Jassim S, Cawthorne MA, Langlands K. A topology-based score for pathway enrichment. J Comput Biol. 2012;19:563-73. doi:10.1089/cmb.2011.0182. PMID:22468678
- Draghici S, Khatri P, Tarca AL, Amin K, Done A, Voichita C, Georgescu C, Romero R. A systems biology approach for pathway level analysis. Genome Res. 2007;17:1537-45. doi:10.1101/gr.6202607. PMID:17785539
- Tarca AL, Draghici S, Khatri P, Hassan SS, Mittal P, Kim JS, Kim CJ, Kusanovic JP, Romero R. A novel signaling pathway impact analysis. Bioinformatics. 2009;25:75-82. doi:10.1093/bioinformatics/btn577. PMID:18990722
- Shabalin AA, Tjelmeland H, Fan C, Perou CM, Nobel AB. Merging two gene-expression studies via cross-platform normalization. Bioinformatics. 2008;24:1154-60. doi:10.1093/bioinformatics/btn083. PMID:18325927
- Makarev E, Izumchenko E, Aihara F, Wysocki PT, Zhu Q, Buzdin A, Sidransky D, Zhavo-ronkov A, Atala A. Common pathway signature in lung and liver fibrosis. Cell Cycle. 2016;15:1667-73. doi:10.1080/15384101.2016.1152435. PMID:27267766
- Artcibasova AV, Korzinkin MB, Sorokin MI, Shegay PV, Zhavoronkov AA, Gaifullin N Alekseev BY, Vorobyev NV, Kuzmin DV, Kaprin АD, et al. MiRImpact, a new bioinformatic method using complete microRNA expression profiles to assess their overall influence on the activity of intracellular molecular pathways. Cell Cycle. 2016;15:689-98. doi:10.1080/15384101.2016.1147633. PMID:27027999
- Alexandrova E, Nassa G, Corleone G, Buzdin A., Aliper AM, Terekhanova N, Shepelin D, Zhavoronkov A, Tamm M, Milanesi L, et al. Large-scale profiling of signaling pathways reveals an asthma specific signature in bronchial smooth muscle cells. Oncotarget. 2016;7:25150-61. doi:10.18632/oncotarget.7209. PMID:26863634
- Lebedev TD, Spirin PV, Suntsova MV, Ivanova AV, Buzdin AA, Prokofjeva MM, Rub-tsov PM, Prassolov VS. Receptor tyrosine kinase KIT may regulate expression of genes involved in spontaneous regression of neuroblastoma. Mol Biol (Mosk). 2015;49:1052-1055. doi:10.7868/S0026898415060154. PMID:26710790
- Lazar C, Meganck S, Taminau J, Steenhoff D, Coletta A, Molter C, Weiss-Solís DY, Duque R, Bersini H, Nowé A. Batch effect removal methods for microarray gene expression data integration: a survey. Brief Bioinform. 2013;14:469-90. doi:10.1093/bib/bbs037. PMID:22851511
- Risso D, Schwartz K, Sherlock G, Dudoit S. GC-content normalization for RNA-Seq data. BMC Bioinformatics. 2011;12:480. doi:10.1186/1471-2105-12-480. PMID:22177264
- Karlsson J, Holmquist Mengelbier L, Ciornei CD, Naranjo A, O'Sullivan MJ, Gisselsson D. Clear cell sarcoma of the kidney demonstrates an embryonic signature indicative of a primitive nephrogenic origin. Genes Chromosomes Cancer. 2014;53:381-91. doi:10.1002/gcc.22149. PMID:24488803
- Van Delft J, Gaj S, Lienhard J, Albrecht MW, Kirpiy A, Brauers K, Claes-sen S, Lizarraga D, Lehrach H, Herwig R, Kleinjans J. RNA-seq provides new insights in the transcriptome responses induced by the carcinogen benzo[a]pyrene. Toxicological sciences. 2012;130:427-39. doi:10.1093/toxsci/kfs250. PMID:22889811
- Xu X, Zhang Y, Williams J, Antoniou E, McCombie WR, Wu S, Zhu W, Davidson NO, De-noya P, Li E. Parallel comparison of Illumina RNA-Seq and Affymetrix microarray platforms on transcriptomic profiles generated from 5-aza-deoxy-cytidine treated HT-29 colon cancer cells and simulated datasets. BMC Bioinformatics. 2013;14:S1. doi:10.1186/1471-2105-14-S9-S1. PMID:23902433
- Kim SC, Jung Y, Park J, Cho S, Seo C, Kim J, Kim P, Park J, Seo J, Kim J, et al. A high-dimensional, deep-sequencing study of lung adenocarcinoma in female never-smokers. PLoS One. 2013;8:e55596. doi:10.1371/journal.pone.0055596. PMID:23405175
- Yang W, Ramachandran A, You S, Jeong H, Morley S, Mulone MD, Log-vinenko T, Kim J, Hwang D, Freeman MR, Adam RM. Integration of proteomic and transcriptomic profiles identifies a novel PDGF-MYC network in human smooth muscle cells. Cell Commun Signal. 2014;12:44. doi:10.1186/s12964-014-0044-z. PMID:25080971
- Cabezas-Wallscheid N, Klimmeck D, Hansson J, Lipka DB, Reyes A, Wang Q, Weich-enhan D, Lier A, von Paleske L, Renders S, et al. Identification of regulatory networks in HSCs and their immediate progeny via integrated proteome, transcriptome, and DNA methylome analysis. Cell Stem Cell. 2014;15:507-22. doi:10.1016/j.stem.2014.07.005
- Hara Y, Kawasaki N, Hirano K, Hashimoto Y, Adachi J, Watanabe S, Tomonaga T. Quantitative proteomic analysis of cultured skin fibroblast cells derived from patients with triglyceride deposit cardiomyovasculopathy. Orphanet J Rare Dis. 2013;8:197. doi:10.1186/1750-1172-8-197. PMID: 24360150
- Warnat P, Eils R, Brors B. Cross-platform analysis of cancer microarray data improves gene expression based classification of phenotypes. BMC Bioinformatics. 2005;6:265. doi: 10.1186/1471-2105-6-265. PMID:16271137
- Hsu MJ, Chang YC, Hsueh HM. Biomarker selection for medical diagnosis using the partial area under the ROC curve. BMC Res Notes. 2014;7:25. doi:10.1186/1756-0500-7-25. PMID:24410929
- Chalaya T, Gogvadze E, Buzdin A, Kovalskaya E, Sverdlov ED. Improving specificity of DNA hybridization-based methods. Nucleic Acids Res. 2004;32:e130. doi:10.1093/nar/gnh125. PMID:15371554
- Shugay M, Britanova OV, Merzlyak EM, Turchaninova MA, Mamedov Z, Tuganbaev TR, Bo-lotin DA, Staroverov DB, Putintseva EV, Plevova K, et al. Towards error-free profiling of immune repertoires. Nat Methods. 2014;11:653-655. doi:10.1038/nmeth.2960. PMID:24793455
- Aiello D, Casadonte F, Terracciano R, Damiano R, Savino R, Sindona G, Napoli A. Targeted proteomic approach in prostatic tissue: a panel of potential biomarkers for cancer detection. Oncoscience. 2016;3:220-41. doi:10.18632/oncoscience.313. PMID:27713912
- Borras C, Abdelaziz KM, Gambini J, Serna E, Inglés M, de la Fuente M, Garcia I, Matheu A, Sanchís P, Belenguer A, Errigo A, Avellana JA, Barettino A, Lloret-Fernández C, Flames N, Pes G, Rodriguez-Mañas L, Viña J. Human exceptional longevity: transcriptome from centenarians is distinct from septuagenarians and reveals a role of Bcl-xL in successful aging. Aging (Albany NY). 2016;8:3185-208. doi:10.18632/aging.101078. PMID:27794564
- Demetrashvili N, Kron K, Pethe V, Bapat B, Briollais L. How to deal with batch effect in sequential microarray experiments? Mol Inform. 2010;29:387-393. doi:10.1002/minf.200900019. PMID:27463194
- Cancer Genome Atlas Research Network. Comprehensive genomic characterization defines human glioblastoma genes and core pathways. Nature. 2008;455:1061-68. doi:10.1038/nature07385. PMID:18772890
- Jones P, Côté RG, Martens L, Quinn AF, Taylor CF, Derache W, Hermjakob H, Apweiler R. PRIDE: a public repository of protein and peptide identifications for the proteomics community. Nucleic Acids Res. 2006;34:D659-63. doi:10.1093/nar/gkj138. PMID:16381953
- Bolstad BM, Irizarry RA, Astrand M, Speed TP. A comparison of normalization methods for high density oligonucleotide array data based on variance and bias. Bioinformatics. 2003;19:185-193. PMID:12538238. doi:10.1093/bioinformatics/19.2.185.
- McCall MN, Bolstad BM, Irizarry RA. Frozen robust multiarray analysis (fRMA). Biostatistics. 2010, 11:242-53. doi:10.1093/biostatistics/kxp059. PMID:20097884
- Anders S, Huber W. Differential expression analysis for sequence count data. Genome Biol. 2010;11:R106. doi:10.1186/gb-2010-11-10-r106. PMID:20979621
- Huang H, Lu X, Liu Y, Haaland P, Marron JS. R/DWD: distance-weighted discrimination for classification, visualization and batch adjustment. Bioinformatics. 2012;28:1182-83. doi:10.1093/bioinformatics/bts096. PMID:22368246
- Deshwar, A.G., Morris, Q. (2014) PLIDA: cross-platform gene expression normalization using perturbed topic models. Bioinformatics, 30, 956-61, 10.1093/bioinformatics/btt574. PMID:24123674
- Spirin PV, Lebedev TD, Orlova NN, Gornostaeva AS, Prokofjeva MM, Nikitenko NA, Dmit-riev SE, Buzdin AA, Borisov NM, Aliper AM, et al. Silencing AML1-ETO gene expression leads to simultaneous activation of both pro-apoptotic and proliferation signaling. Leukemia. 2014;28:2222-8. doi:10.1038/leu.2014.130. PMID:24727677
- Birtwistle MR, Hatakeyama M, Yumoto N, Ogunnaike BA, Hoek JB, Kholodenko BN. Ligand-dependent responses of the ErbB signaling network: experimental and modeling analyses. Mol Syst Biol. 2007;3:144. doi:10.1038/msb4100188. PMID:18004277
- Borisov N, Aksamitiene E, Kiyatkin A, Legewie S, Berkhout J, Maiwald T, Kaimach-nikov NP, Timmer J, Hoek, JB, Kholodenko B.N. Systems-level interactions between insulin-EGF networks amplify mitogenic signaling. Mol Syst Biol. 2009;5:256. doi:10.1038/msb.2009.19. PMID:19357636
- Kuzmina NB, Borisov NM. Handling complex rule-based models of mitogenic cell signaling (On the example of ERK activation upon EGF stimulation). Intl Proc Chem Biol Envir Engng. 2011;5:76-82. doi:10.7763/ipcbee.2011.v5.17
- Even Sh. Graph Algorithms. Ed. by G. Even. Cambridge, UK: Cambridge University Press; 2011. ISBN-13: 978-0521736534. ISBN-10: 0521736536
- Culhane AC, Thioulouse J, Perrière G, Higgins DG. MADE4: an R package for multivariate analysis of gene expression data. Bioinformatics. 2005;21:2789-90. doi:10.1093/bioinformatics/bti394. PMID:15797915
- Scales M, Jäger R, Migliorini G, Houlston RS, Henrion MY. VisPIG–a web tool for producing multi-region, multi-track, multi-scale plots of genetic data. PLoS One. 2014;9:e107497. doi:10.1371/journal.pone.0107497. PMID:25208325
- Rudy J, Valafar F. Empirical comparison of cross-platform normalization methods for gene expression data. BMC Bioinformatics. 2011;12:46. doi:10.1186/1471-2105-12-467. PMID:21291543