ABSTRACT
Differences in human phenotypes and susceptibility to complex diseases are an outcome of genetic and environmental interactions. This is evident in diseases that progress through a common set of intermediate patho-endophenotypes. Precision medicine aims to delineate molecular players for individualized and early interventions. Functional studies of lymphoblastoid cell line (LCL) model of phenotypically well-characterized healthy individuals can help deconvolute and validate these molecular mechanisms. In this study, LCLs are developed from eight healthy individuals belonging to three extreme constitution types, deep phenotyped on the basis of Ayurveda. LCLs were characterized by karyotyping and immunophenotyping. Growth characteristics and response to UV were studied in these LCLs. Significant differences in cell proliferation rates were observed between the contrasting groups such that one type (Kapha) proliferates significantly slower than the other two (Vata, Pitta). In response to UV, one of the fast growing groups (Vata) shows higher cell death but recovers its numbers due to an inherent higher rates of proliferation. This study reveals that baseline differences in cell proliferation could be a key to understanding the survivability of cells under UV stress. Variability in baseline cellular phenotypes not only explains the cellular basis of different constitution types but can also help set priors during the design of an individualized therapy with DNA damaging agents. This is the first study of its kind that shows variability of intermediate patho-phenotypes among healthy individuals with potential implications in precision medicine.
Introduction
A complex interplay of genetics and environmental conditions leads to inter-individual differences in phenotypic outcomes and disease predispositions [Citation1,Citation2]. Precision medicine aims to identify genetic variations that help predict these differences [Citation3]. Strategies are being attempted to uncover variations that govern the differential response to the environment in healthy and diseased individuals [Citation4,Citation5]. Identifying variations that govern the differential response to the environment in healthy individuals prior to disease onset is still a challenge [Citation6–8]. This would be important for identifying predictive markers for actionable, early and individualized interventions.
Lymphoblastoid cell line (LCL) models of genetically characterized individuals such as the 1000 Genomes Project are being used to identify quantitative trait loci (eQTL) [Citation9]. In addition, cell lines of diseased individuals are also being used to uncover QTLs linked to differential drug response [Citation10,Citation11]. However, connecting these cellular differences to phenotypic outcomes requires newer and innovative approaches, such as functional phenomics that overlay information from multidimensional phenotypes for understanding biological differences [Citation12,Citation13].
In Ayurgenomics, multi-omics studies are carried out on healthy individuals that are classified on the basis of multi-system phenotypes, called “Prakriti” [Citation14]. Individuals in a population can be stratified into broadly seven types. Prakriti of an individual is invariant and governs health and disease trajectories including responsiveness to the environment. Out of the seven, Vata, Pitta and Kapha are the most contrasting types in phenotypes and susceptibilities [Citation15,Citation16]. Unsupervised clustering approaches have revealed distinct phenomic architecture of these contrasting constitutions and supervised machine learning and advanced statistical models could accurately predict these types [Citation14]. These constitution types exhibit differences at the genome-wide expression, genetic, epigenetic and microbiome levels [Citation15,Citation17–20]. Further, Prakriti types significantly differ with respect to variations in genes associated with response to double-stranded RNA, DNA-damaging agents, and hypoxia [Citation17,Citation21,Citation22].
These multi-omic differences prompted us to develop cellular models of extreme Prakriti types to elucidate differences in cellular phenotypes that might provide further scope for functional studies. We hypothesized that different Prakriti cell lines could respond differently to the same trigger through the same or distinct molecular networks. To address this, LCLs derived from healthy humans of extreme Prakriti types, identified from a cohort of a homogenous genetic background, were developed [Citation17]. During the LCL characterization, a surprising observation was made – there were striking differences in growth rates among the eight LCLs. Based on this intriguing observation, experiments were designed to establish the robustness of these differences in cell proliferation and probe their consequences in UV stress. This is the first study that reports a baseline difference in cell proliferation among healthy individuals that could contribute to variability in response to UV. These observations would be relevant in scenarios where altered rates of cell proliferation are a cause or consequence of disease outcomes and thus could help set priors for precision interventions.
Materials and methods
Sample collection and ethical clearance
The LCL lines were prepared from healthy individuals of extreme Prakriti types identified from the ayurgenomics cohort from Vadu [Citation14]. Ten male participants were selected based on detailed clinical phenotyping by Ayurveda clinicians. The study was approved by the KEMHRC ethics committee. With the written informed consent of the participants, 10 ml blood was drawn in heparin vacutainers, stored at room temperature and processed within 24 hours. Blood was tested for hepatitis B and HIV prior to the creation of cell lines. It was ensured that the participants were not under any medication at the time of sampling.
Peripheral blood mononuclear cells (PBMC) isolation
Blood was diluted with equal volume of phosphate-buffered saline (PBS), mixed by inversion and layered on Ficoll paque density gradient. The PBMCs were collected from the buffy layer after centrifugation at 400 g for 40 minutes. These were cultured in RPMI-1640 (AT060, Himedia) supplemented with 15% fetal bovine serum (FBS; Gibco, 10,270-106). The resultant PBMCs were then used for the generation of LCLs.
Lymphoblastoid cell line generation
Fresh PBMCs were infected with Epstein–Barr virus (EBV; viral stock was received as a kind gesture from Dr Odity Mukerjee’s lab, National Center for Biological Sciences, Bangalore, India) and incubated in RPMI-1640 supplemented with 15% FBS at 37 °C with 5% CO2 in a cell culture incubator for 3 hours. RPMI-1640 supplemented with 20% FBS and 1 µg/ml cyclosporine A (C3662, Sigma) was added in 1:1 ratio to the cellular media containing viral particles. Cells were incubated for 6 days without media supplementation. Subsequently, the cells were cultured for 3 to 4 weeks with regular media changes. The LCLs were maintained in RPMI-1640 supplemented with 15% FBS, 2 g/L sodium bicarbonate (S5761, Sigma), and 1× antibiotic-antimycotic (15,240-062, Gibco). Cells were maintained at 37 °C with 5% CO2 in a cell culture incubator. LCLs were successfully derived from eight individuals and used in subsequent experiments.
Morphology of the cells with respect to aggregates/clumps and rosette formation was recorded. Cellular clumps were disrupted by pipetting to ensure a multiclonal population. This population was considered to be the first passage of LCLs. Characterization and subsequent experiments were performed from the fifth passage.
Characterization of LCLs
(a) Karyotyping: Karyotyping of LCLs was performed by standard methods[Citation23]. Briefly, 2 × 106 cells were treated with 0.1 μg/ml of Colcemid (155,212–012, Gibco) for 30 minutes in normal culture conditions. They were subjected to a hypotonic solution of 0.075 M KCl for 30 minutes in a 37 °C water bath and fixed in fresh Carnoy’s fixative (3:1, methanol:acetic acid). Slides were prepared by pipetting two drops of cell suspension on slides held at 45°. Slides were heat fixed on a 45 °C hot plate for 2 minutes. Slides were aged for a day followed by trypsinization and staining with Giemsa stain for visualizing the chromosome banding pattern [Citation23]. (b) Immunophenotyping: In order to ensure that the LCLs originated primarily from B cells, immunophenotyping was carried out by flow cytometry analysis of B-cell-specific surface markers. 0.1 × 106 cells were harvested and washed with PBS for surface staining with lymphocyte-specific CD19 (B cell), CD3 (T cell), and CD56 (NK cell) marker antibodies (BD Biosciences). PBMCs with heterogeneous cells were used as positive controls. Non-specific binding of the antibody was blocked using FC receptor blocker (BD Biosciences) for 30 minutes on ice followed by incubation with the antibodies for 40 minutes on ice. Cells were washed with cold PBS twice and immediately analyzed by flow cytometry on BD LSR II. A minimum of 1 × 104 cells were recorded per sample for all flow cytometry analysis.
LCL growth curve assay
Growth assay of LCLs was performed using a non-lytic, luminescence-based method using the RealTime-Glo™ MT cell viability assay (G9711, Promega). The Realtime-Glo™ enzyme and substrate were added to the cell culture medium during seeding of cells. Cells were seeded at 10,000/100 µl media per well on a 96-well plate and incubated in normal culture conditions. Luminescence units were recorded at near regular hours for plotting the growth curves. Growth rate and doubling times were calculated from the log phase.
CFSE proliferation assay
Cell proliferation rates were estimated by tracing cells labeled with carboxyfluorescein succinimidyl ester (CFSE) across generations and compared between populations using CellTrace™ CFSE cell proliferation kit (C34554, Invitrogen) [Citation24]. The cells were stained with 5 µM CFSE and grown on 12-well plates at a seeding density of 1 × 105/ml. Readings were taken on day 0 and day 3 (72 hours). The cells fixed in 4% paraformaldehyde were resuspended in FACS buffer (PBS with 1% FBS) and analyzed by FACS on BD LSR II. The division index (DI) – the average number of cell divisions that a cell in the original population has undergone – was calculated using FlowJo software (BD Biosciences).
Cell cycle analysis after synchronization by double thymidine block
Cells were seeded at a density of 1 × 105/ml in media supplemented with 2 mM Thymidine (T9250-5G, Sigma) followed by 18 hours of growth. Cells were washed and grown in media without thymidine for 9 hours, followed by a 15-hour growth period in thymidine-supplemented media. The cells were harvested and fixed in 70% ethanol followed by permeabilization in 0.1% Triton-X 100 and RNase (40 ng/ml) treatment. Cell cycle phases were studied by staining the cells with propidium iodide and analyzing by flow cytometry.
Comparison of cell cycle stages using specific markers
The proportion of cells in different cell cycle phases was compared by Western blotting. We checked CDK2 Tyr15 phosphorylation for G1/S phase and histone 3 Ser10 phosphorylation for mitosis. Cell lysates were prepared at 24 hours of growth and SDS-PAGE was performed on 15% bis-acrylamide gel. Proteins were electrophoresed onto a PVDF membrane and blocked with 5% bovine serum albumin. The primary antibody used was a cocktail of rabbit anti-Cdk2 pTyr15, and rabbit anti-histone H3 pSer10 (1:1000 dilution, ab136810, Abcam). The secondary antibody was horseradish peroxidase conjugated goat anti-rabbit immunoglobulin G (1:10,000 dilution, sc-2004; Santa Cruz Biotechnology). Membranes were developed with the optiblot ECL detection kit (ab133406; Abcam) and imaged using a Chemi-Doc (ImageQuant LAS 500). Protein expression was measured by densitometry analysis of the bands using ImageJ and normalized to the density of the β-actin.
Ki67 assay
To estimate the number of proliferating cells, a 48-hour time course assay of Ki67 was carried out. Cells were cultured at 1 × 105 cells/ml in 12-well plates and one well of each LCL was harvested every 12 hours, from 0 to 48 hours. Cells were fixed in 4% paraformaldehyde, permeabilized with 0.1% Triton-X 100, and stained with 1:200 dilution of Ki67 antibody (11-5698-80; Invitrogen) for 40 minutes at RT. Cells were resuspended in the FACS buffer and analyzed on BD LSR II flow cytometer.
Cell proliferation and death in response to UV
1 × 105 CFSE stained cells were seeded per well in 12-well plates. The cells were exposed to 50 mJ/cm2 UV B and grown for 48 hours. Live and dead cells were counted using trypan blue staining. Cell proliferation was quantitated by flow cytometry analysis of CFSE-stained cells.
Statistical analysis
The normal distribution was checked using Shapiro–Wilk test. Pairwise comparisons of Vata versus Kapha, Vata versus Pitta and Pitta versus Kapha were carried out using Student’s t-test.
Results
Characterization of Prakriti-specific LCLs
LCLs showed a hallmark morphology as evidenced by the presence of uropods and the formation of aggregates in suspension culture, as seen through bright field microscopy (Figure S1). Karyotype analysis confirmed the presence of normal chromosome numbers, structure, and ploidy (Figure S2). Immunophenotyping affirmed LCLs to be of B cell origin, based on CD19-positive staining (Figure S3). Cells did not stain for the markers of T cells (CD3) and NK cells (CD56).
Differences in growth rate between Prakriti LCLs
The initial objective of the study was to observe cellular response to UV in LCLs, for which it was needed to work with equal cell densities. However, during initial experiments, a striking observation was made that the Vata and Pitta cell lines grow faster in comparison to Kapha and require more frequent media changes. This prompted the exploration of whether there were inherent differences in cell proliferation rates among the LCLs. Growth curves of LCLs revealed two groups of slow and fast-growing lines based on the average doubling time (36.6 hours). Interestingly, the three Kapha LCLs had doubling times greater than 36.6 hours and fell into the slow growing group (, b; Figure S4). The three Vata and two Pitta LCLs had doubling times less than 36.6 hours and fell into the fast-growing group (, b; Figure S4). This significantly differentiated Kapha from Vata and Pitta (, b). The growth curves were repeated multiple times as biological and technical replicates, i.e., a new stock from the same passage was revived for each experiment and the experiment was performed in triplicates.
Figure 1. Prakriti-specific growth differences in LCLs. (a) RealTime-Glo™ growth curves of LCLs. (b) Doubling times of the Kapha (K) to be significantly lower than Vata (V) and Pitta (P). Growth curves and box plots from biological and technical replicates [N = 3 (Vata and Kapha) and N = 2 (Pitta), n = 3, error bars denote ±SEM, unpaired t-test, ****p < 0.00001, ***p < 0.0001)]. (c) CFSE assay shows Kapha cell population dividing slower than Vata and Pitta (representative images of flow cytometry). (d) DI of Kapha is significantly lower than Vata and Pitta. Box plot from analysis of biological and technical replicates [N = 3 (Vata and Kapha) and N = 2 (Pitta), n = 3, error bars denote ±SEM, unpaired t-test, ***p < 0.0001)]
![Figure 1. Prakriti-specific growth differences in LCLs. (a) RealTime-Glo™ growth curves of LCLs. (b) Doubling times of the Kapha (K) to be significantly lower than Vata (V) and Pitta (P). Growth curves and box plots from biological and technical replicates [N = 3 (Vata and Kapha) and N = 2 (Pitta), n = 3, error bars denote ±SEM, unpaired t-test, ****p < 0.00001, ***p < 0.0001)]. (c) CFSE assay shows Kapha cell population dividing slower than Vata and Pitta (representative images of flow cytometry). (d) DI of Kapha is significantly lower than Vata and Pitta. Box plot from analysis of biological and technical replicates [N = 3 (Vata and Kapha) and N = 2 (Pitta), n = 3, error bars denote ±SEM, unpaired t-test, ***p < 0.0001)]](/cms/asset/f3e54fa9-4b6b-4e54-8530-59786d260dbe/kccy_a_1909884_f0001_oc.jpg)
Proliferation differences in cell populations of Prakriti LCLs
To investigate whether differences in growth rate are due to the entire population or only a certain fraction of cells, CFSE dye dilution method was used. The distribution of the cells across generations was studied at 72 hours of growth. Vata and Pitta LCLs were almost in the third generation of division whereas Kapha LCLs were in the second generation (, S5). We did not see any population of cells in the undivided peak, at 72 hours, which shows that all the cells in the population have undergone at least one round of division. The DI of Kapha LCLs is significantly lower than that of Vata and Pitta LCLs (). This shows that the entire cell population of Kapha LCLs divides slower than Vata and Pitta LCLs.
Cell cycle kinetics differ between Prakriti LCLs
To compare cell cycle kinetics, the synchronization of the eight LCLs was planned to follow the progression of cell populations through G1, S, and G2 phase by propidium iodide (PI) staining of DNA. A double thymidine block was used to synchronize the cells, which arrests cells at the boundary of G1/S. The same degree of synchronization was not achieved in all eight cell lines (Figure S6) which could allow the comparison of cell cycle progression. Other standard methods of synchronization were also attempted, for example, serum starvation for 24 hours, nocodazole treatment, etc., but they failed to get all the LCLs synchronized to the same phase. In an alternate strategy, cell cycle marker protein CDK2 (G1/S) and histone 3 (mitosis), were used for inferring differences in cell cycle kinetics. The G1/S phase marker CDK2 phosphorylation differed significantly between the three groups with levels in Vata<Pitta<Kapha at 24 hours of growth (, b). On the contrary, the mitosis marker histone 3 phosphorylation was significantly different between the three LCL groups with levels in Kapha<Vata<Pitta (, c). Thus, the results indicate that at 24 hours of growth, mitosis is lower in Kapha cell lines compared to others. However, it is interesting to note that even though Vata and Pitta show higher cell proliferation rates, the molecular mechanisms could be different.
Figure 2. Cell cycle marker proteins in Prakriti-specific LCLs. (a) Western blot (representative) for CDK2 pTyr15 (G2/S marker), histone H3 pSer10 (mitosis marker), and β-actin (loading control) at 24 hours of growth. (b) Western Blot quantitation showing a significant difference in CDK2 pTyr15 (G2/S marker) between Prakriti. (c) hHistone H3 pSer10 (mitosis marker) levels significantly differ between Prakriti [N = 3 (Vata and Kapha) and N = 2 (Pitta), n = 3, error bars denote ±SEM, unpaired t-test ****p < 0.00001, ***p < 0.0001, *p < 0.05]
![Figure 2. Cell cycle marker proteins in Prakriti-specific LCLs. (a) Western blot (representative) for CDK2 pTyr15 (G2/S marker), histone H3 pSer10 (mitosis marker), and β-actin (loading control) at 24 hours of growth. (b) Western Blot quantitation showing a significant difference in CDK2 pTyr15 (G2/S marker) between Prakriti. (c) hHistone H3 pSer10 (mitosis marker) levels significantly differ between Prakriti [N = 3 (Vata and Kapha) and N = 2 (Pitta), n = 3, error bars denote ±SEM, unpaired t-test ****p < 0.00001, ***p < 0.0001, *p < 0.05]](/cms/asset/be601326-7908-4597-9209-4dbdc6447b8c/kccy_a_1909884_f0002_oc.jpg)
To further explore whether the number of proliferating cells is lower in Kapha LCLs as compared to that in Vata and Pitta in a temporal manner, the cell proliferation marker Ki67 was traced in the cell lines over a 48-hour time course. The levels of Ki67-positive cells were higher in the Vata and Pitta LCLs along the entire time course (); however, significant differences were observed at the 12 hours (, c).
Figure 3. Ki67 proliferation marker differs between Prakriti types. (a) Percent of proliferating cells in LCL populations at 12 hours (representative flow cytometry plots). (b) Ki67 staining over the course of 48 hours of growth. (c) Kapha has significantly lower Ki67-positive cells at 12 h compared to Vata and Pitta [N = 3 (Vata and Kapha) and N = 2 (Pitta), n = 3, error bars denote ±SEM, unpaired t-test ***p < 0.0001, **p < 0.001)
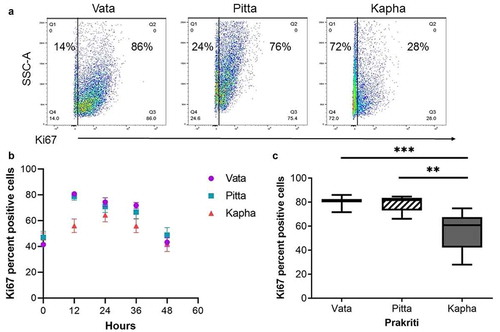
Effect of UV exposure on cell proliferation rates in Prakriti LCLs
It is known that cell cycle arrest ensues in response to UV or DNA damaging agents. So, the next question is how do LCLs of different Prakriti types, with inherent differences in baseline cell proliferation rates, respond to UV? Interestingly, it was found that the Vata LCLs are significantly more sensitive to UV than others (). Not only do they show significantly less percentage viability compared to Kapha () but also a significantly higher death percentage compared to Pitta and Kapha () in response to UV. The Kapha LCLs displayed significantly lower growth arrest compared to the Vata LCLs () 48-hours after UV exposure. Pitta LCLs did not show any significant differences compared to Vata but exhibited higher growth arrest compared to Kapha LCLs () in UV stress. All the LCLs show similar viable cell numbers after 48 hours of UV exposure (). However, the differences in UV response can only be appreciated by accounting for the baseline viability difference between them (). All the LCLs show growth arrest in response to UV, but the growth rate of Vata still remains higher than the Kapha cell lines (). This high growth rate helps the Vata LCLs to cope up with the higher cell death caused by UV () and recover their cell numbers ().
Figure 4. Effect of UV exposure on Prakriti-specific LCLs. (a) Percent cell viability, (b)viable cell number, (c) percent cell death, and (d) dead cell number of Prakriti-specific LCL types in response to UV stress. (e) Division index of Prakriti LCLs normalized to respective untreated controls. (f) Division index of control and UV exposed LCLs (N = 3 (Vata and Kapha) and N = 2 (Pitta), n = 3, error bars denote ±SEM, unpaired t-test ****p < 0.00001, **p < 0.001, *p < 0.05)
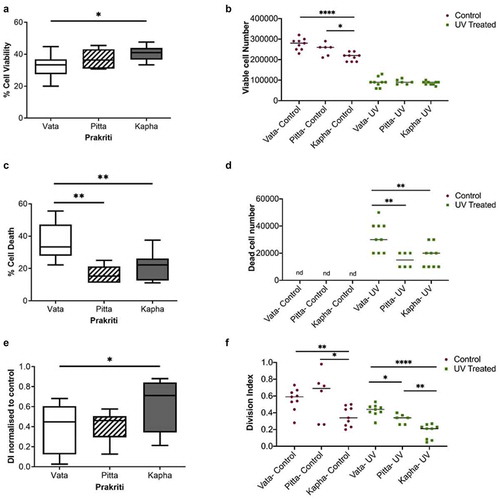
Discussion
This study reports a novel finding that cell proliferation rates differ significantly among LCLs derived from healthy individuals stratified phenotypically. Processes such as cell proliferation are thought to be homeostatic and not expected to vary systematically across individuals; the data from this research suggest otherwise. The LCLs studied here were derived from individuals of extreme and contrasting constitutions who differ with respect to multisystem and molecular attributes [Citation17–20,Citation25]. A core cellular phenotype is shown to be differentiating between these groups where Vata and Pitta have higher rates of cell proliferation compared to Kapha. However, Vata shows significantly more cell death in UV, than Pitta and Kapha. Despite its higher cell mortality, the baseline proliferation rates in Vata seem to provide a growth advantage to the surviving cells post UV treatment.
Cell proliferation is coupled to a variety of cellular and individual phenotypes, for instance, DNA repair [Citation26,Citation27]. Slow proliferation allows repair to be complete before the next cell division whereas fast proliferation allows coupling of DNA repair to replication and enables homology driven replication coupled repair process [Citation28]. Impaired cell turnover can lead to extensive cell accumulation, e.g., neoplastic and cancerous growth. Inappropriately triggered cell death can lead to excessive cell loss, e.g., neurodegenerative, autoimmune, and cardiovascular disorder [Citation29,Citation30]. Interestingly, neurons reenter cell cycles before apoptosis and impaired cell cycle regulation is a clear indicator of neurodegenerative disorders [Citation31]. Therefore, inherent differences in cell proliferation and repair in healthy states could contribute to inter-individual variability in disease and responsiveness. In this context, a recent study reports higher basal cell proliferation rate differences in LCLs derived from patients with bipolar disorder who respond to lithium chloride (LiCl) treatment. The response of control and patient LCLs to LiCl is shown to be dependent on these baseline proliferation differences [Citation32]. Higher cell proliferation has also been reported in the lymphocyte lines of Alzheimer’s disease [Citation33]. Could this be an early phenotype of predispositions? Assessment of cell proliferation also forms the basis for therapeutic interventions in cancer and its therapies. Without prior knowledge of baseline proliferation rates, enhanced killing of cancer cells by therapy might suggest a need for lower dosage or shorter treatment. However, priors set from the baseline data may suggest a higher dose or recurrent treatment to prevent relapse or predict differences in responsiveness.
The Prakriti types are an outcome of relative proportions of the three groups of physiological entities “Tridosha,” that govern the kinetic “Vata,” metabolic “Pitta” and structural “Kapha” components of the body. These proportions in Prakriti types are established at the time of embryonic development, and remain invariant throughout lifetime [Citation15,Citation25]. A previous report from the laboratory on the blood transcriptome from another cohort report differential enrichment of molecular pathways between the Prakriti types [Citation17]. Significant upregulation and enrichment of cell cycle genes between Vata and Kapha corroborates with the cellular differences reported here. The earlier work also revealed significant genetic differences in a subset of genes that govern cell proliferation, DNA damage response, apoptosis – AKT3, FAS, RAD51 between the Prakriti [Citation21,Citation34]. Hence, cellular proliferation rate seems to be a core cellular phenotype specific to Prakriti type. It is also a shared endophenotype for multiple triggers. Deconvolution of this pathway through Prakriti phenotypes might provide novel biological leads. For instance, here differences are observed among Prakriti types in the phosphorylation of CDK2, a candidate gene implicated in neuronal loss in Parkinson’s disease [Citation35].
The observations made here might help provide clues to some of the biological differences and inherent susceptibilities described by Prakriti. Noteworthy to mention are the barrier function differences with respect to UV that have been described in many studies [Citation36,Citation37]. Membrane activating agents have been shown to activate cell division [Citation38]. In Ayurveda, there are vivid descriptions of better barrier functions in Kapha. It might be possible that fast proliferation does not let the cell membrane stabilize as much as the slow proliferators, resulting in poor barrier function. Earlier research by the group has demonstrated such links between hypoxia response and hemostatic outcomes in Prakriti types. Individual’s bleeding outcomes were due to the inherently elevated hypoxia responsiveness in Pitta [Citation22]. In future studies, it is pertinent to explore whether differences in cell membrane properties could explain the response to UV in Kapha and consequent differences in cell proliferation rates.
These observations are preliminary and have to be carried out in larger sample sizes, cohorts and cellular models for generalizability. LCLs have the inherent limitations of not being readily amenable to transfection and therefore differentiated cell lineages from the same individual can be considered. Correlation of this core cellular phenotype across different pathological conditions in prospective cohorts is testable. This study also emphasizes the advantage of Prakriti-based stratification for predictive medicine and early interventional possibilities.
Conclusion
This first-of-its-kind study highlights the importance of the Aurgenomics approach in the development of cellular models for functional phenomics. This can be effective in integrating the expression readouts from system-level phenotypes to cellular outcomes in an individual-specific manner. Our observations open up tantalizing possibilities in precision medicine, for instance, taking into account the baselines of cell proliferation as priors might help resolve unexplainable outcomes and lead to individualized interventions. Noninvasive Prakriti-based stratification that focuses on individuality is what envisaged in affordable precision medicine initiatives.
Supplemental Material
Download MS Word (631.1 KB)Acknowledgments
We thank Odity Mukherjee for helping with the LCL facility, and the CSIR FACS Facility. This work was financially supported by the Ministry of AYUSH center of excellence (GAP0183) and CSIR funding by Trisutra (MLP901). Fellowship support was received from CSIR (Sumita), ICMR (Sunanda) and COE (Dayanidhi). We thank the field team of KEM Hospital Research Centre, Pune, for their assistance in data generation. Contribution from the participants is appreciated.
Disclosure statement
No potential conflict of interest was reported by the authors.
Supplementary material
Supplemental data for this article can be accessed here.
Additional information
Funding
References
- Kraft P, Yen Y-C, Stram DO, et al. Exploiting gene-environment interaction to detect genetic associations. Hum Hered. 2007;63:111–119.
- Eley TC, Sugden K, Corsico A, et al. Gene–environment interaction analysis of serotonin system markers with adolescent depression. Mol Psychiatry. 2004;9(10):908–915.
- Chen R, Mias G, Li-Pook-Than J, et al. Personal omics profiling reveals dynamic molecular and medical phenotypes. Cell. 2012;148(6):1293–1307.
- Yugi K, Kubota H, Hatano A, et al. How to reconstruct biochemical networks across multiple ‘omic’ layers. Trends Biotechnol. 2016;34(4):276–290.
- Baker SG. Identifying combinations of cancer markers for further study as triggers of early intervention. Biometrics. 2000;56(4):1082–1087.
- Hood L. Systems biology and p4 medicine: past, present, and future. Rambam Maimonides Med J. 2013;4(2):e0012–e0012.
- Brown SDM, Lad HV. The dark genome and pleiotropy: challenges for precision medicine. Mamm Genome. 2019;30(7–8):212–216.
- Braun E. The unforeseen challenge: from genotype-to-phenotype in cell populations. Rep Prog Phys. 2015;78:036602.
- The 1000 Genomes Project Consortium., Corresponding authors., Auton A, et al. A global reference for human genetic variation. Nature. 2015;526:68–74.
- Otsuka F, Tarone RE, Cayeux S, et al. Use of lymphoblastoid cell lines to evaluate the hypersensitivity to ultraviolet radiation in Cockayne syndrome. J Invest Dermatol. 1984;82(5):480–484.
- Zhang X, Gierman HJ, Levy D, et al. Synthesis of 53 tissue and cell line expression QTL datasets reveals master eQTLs. BMC Genomics. 2014;15(1):532.
- York LM. Functional phenomics: an emerging field integrating high-throughput phenotyping, physiology, and bioinformatics. J Exp Bot. 2019;70(2):379–386.
- Houle D, Govindaraju DR, Omholt S. Phenomics: the next challenge. Nat Rev Genet. 2010;11(12):855–866.
- Tiwari P, Kutum R, Sethi T, et al. Recapitulation of ayurveda constitution types by machine learning of phenotypic traits. PLoS One. 2017;12(10):e0185380.
- Prasher B, Gibson G, Mukerji M. Genomic insights into ayurvedic and western approaches to personalized medicine. J Genet. 2016;95(1):209–228.
- Prasher B, Varma B, Kumar A, et al. Ayurgenomics for stratified medicine: TRISUTRA consortium initiative across ethnically and geographically diverse Indian populations. J Ethnopharmacol. 2017;197:274–293.
- Prasher B, Negi S, Aggarwal S, et al. Whole genome expression and biochemical correlates of extreme constitutional types defined in Ayurveda. J Transl Med. 2008;6(48):48.
- Govindaraj P, Nizamuddin S, Sharath A, et al. Genome-wide analysis correlates ayurveda prakriti. Sci Rep. 2015;5(15786). DOI:10.1038/srep15786.
- Chaudhari D, Dhotre D, Agarwal D, et al. Understanding the association between the human gut, oral and skin microbiome and the Ayurvedic concept of prakriti. J. Biosci. 2019;44(5). DOI:10.1007/s12038-019-9939-6.
- Rotti H, Mallya S, Kabekkodu SP, et al. DNA methylation analysis of phenotype specific stratified Indian population. J. Transl. Med. 2015;13(151). DOI:10.1186/s12967-015-0506-0.
- Aggarwal S, Negi S, Jha P, et al. EGLN1 involvement in high-altitude adaptation revealed through genetic analysis of extreme constitution types defined in Ayurveda. Proc Natl Acad Sci. 2010;107(44):18961.
- Aggarwal S, Gheware A, Agrawal A, et al. Combined genetic effects of EGLN1 and VWF modulate thrombotic outcome in hypoxia revealed by ayurgenomics approach. J. Transl. Med. 2015;13(184). DOI:10.1186/s12967-015-0542-9.
- B. AU–, A. AU–, F. AU-T. Chromosome preparation from cultured cells. J Vis Exp. 2014;e50203. DOI:10.3791/50203
- Quah BJC, Warren HS, Parish CR. Monitoring lymphocyte proliferation in vitro and in vivo with the intracellular fluorescent dye carboxyfluorescein diacetate succinimidyl ester. Nat. Protoc. 2007;2(9):2049–2056.
- Abbas T, Kutum R, Pandey R, et al. Genetic differences between extreme and composite constitution types from whole exome sequences reveal actionable variations. bioRxiv. 2020. DOI:10.1101/2020.04.24.059006. 04.24.059006.
- Mjelle R, Hegre SA, Aas PA, et al. Cell cycle regulation of human DNA repair and chromatin remodeling genes. DNA Repair (Amst). 2015;30:53–67.
- Bhattacharya S, Srinivasan K, Abdisalaam S, et al. RAD51 interconnects between DNA replication, DNA repair and immunity. Nucleic Acids Res. 2017;45(8):4590–4605.
- Zhivotovsky B, Orrenius S. Cell cycle and cell death in disease: past, present and future. J. Intern. Med. 2010;268(5):395–409.
- Boehm M, Nabel E. The cell cycle and cardiovascular diseases. Prog Cell Cycle Res. 2003;5:19–30.
- Balomenos D, Martínez-A C. Cell-cycle regulation in immunity, tolerance and autoimmunity. Immunol Today. 2000;21(11):551–555.
- Park DS, Morris EJ, Greene LA, et al. G1/S cell cycle blockers and inhibitors of cyclin-dependent kinases suppress camptothecin-induced neuronal apoptosis. J Neurosci. 1997;17(1256):1256–1270.
- Paul P, Iyer S, Nadella RK, et al. Lithium response in bipolar disorder correlates with improved cell viability of patient derived cell lines. Sci Rep. 2020;10(7428). DOI:10.1038/s41598-020-64202-1.
- Ashok A, Naaz S, Kota LN, et al. Does retinoic acid reverse cell cycle dysregulation in Alzheimer’s disease lymphocytes? Asian J Psychiatr. 2019;39:174–177.
- Sethi TP, Prasher B, Ayurgenomics: MM. Way of threading molecular variability for stratified medicine. ACS Chem. Biol. 2011;6(9):875–880.
- Giovanni A, Wirtz-Brugger F, Keramaris E, et al. Involvement of cell cycle elements, cyclin-dependent kinases, pRb, and E2F·DP, in B-amyloid-induced neuronal death. J Biol Chem. 1999;274(27):19011–19016.
- Schwarz T. UV light affects cell membrane and cytoplasmic targets. J Photochem Photobiol B. 1998;44(2):91–96.
- Wlaschek M, Tantcheva-Poór I, Naderi L et al. Solar UV irradiation and dermal photoaging. J Photochem Photobiol B . 2001;63:41–51.
- Ingram LO, Fisher WD. Stimulation of cell division by membrane-active agents. Biochem Biophys Res Commun. 1973;50:200–210.