ABSTRACT
Bladder cancer can range from noninvasive to invasive tumors. When non-muscle invasive bladder cancer (NMIBC) recurs, patients could endure long-term invasive malignancies with a high disease-specific death rate. Immune escape frequently results in tumor development, metastases, unfavorable prognosis, and failure of immunotherapy. Based on the median immune score, this study used ESTIMATE scores to evaluate 411 bladder cancer cases from TCGA-BLCA. Two hundred two ncRNAs were differentially expressed in two groups, where 29 candidates appeared to be associated with the overall survival of bladder cancer patients. LASSO algorithm was performed to establish the risk score model of 13-ncRNA. Risk scores were computed for cases in the training set, validation set, and TCGA-BLCA set; Poor prognosis in cases with higher risk scores was based on the training set, validating set, and TCGA-BLCA set. Among the 13 ncRNAs, IGF2BP2-AS1, MAGF-AS1, ARHGAP5-AS1, and LINC00942 were significantly correlated with the overall survival of bladder cancer patients. Pearson’s correlation analysis based on TCGA-BLCA identified 2093, 3107, 386, and 936 mRNAs co-expressed with IGF2BP2-AS1, MAGF-AS1, ARHGAP5-AS1, and LINC00942, respectively. Conclusively, the 13 ncRNA signature exhibited a feasible predictive prognostic value for bladder cancer patients. IGF2BP2-AS1 expression was higher in bladder cancer tissues and significantly correlated to immune-related factors, suggesting that IGF2BP2-AS1 represents a promising immune-related target for treating bladder cancer patients.
Background
Bladder cancer is the fourth most frequent type of cancer in males and a common type of cancer in females [Citation1,Citation2]. Bladder cancer can range from noninvasive/ unaggressive tumors that recur and admit patients to long-term monitoring to aggressive/invasive malignancies with a high disease-specific death rate [Citation3]. The common risk factors for bladder cancer include aging, male sex, and smoking [Citation4,Citation5]. Depending on the risk classification, the treatment of non-muscle invasive bladder cancer (NMIBC) is endoscopic excision followed by intravesical adjuvant treatment. Patients with high-grade NMIBC that do not respond to the potent bacillus Calmette-Guerin (BCG) intravesical immunotherapy are challenging to manage; therefore, various alternative therapies are being researched [Citation6]. More aggressive treatment with radical cystectomy and urinary diversions or trimodality therapy with maximum endoscopic excision, radiosensitizing chemotherapy, and radiotherapy is utilized to minimize the risk of metastases and disease-specific death rate in muscle-invasive bladder cancer patients [Citation7]. Immunotherapies using checkpoint inhibitors, targeted therapies, and antibody-drug conjugates are now available for some individuals at different stages of illness, resulting in rapid changes in the treatment of advanced cancer patients [Citation8].
When tumors form, the body’s immune system is activated to combat their progression. Lamentably, tumors utilize various strategies to postpone or avoid immune detection, a process called immune escape [Citation9,Citation10]. Immune escape frequently results in tumor development, metastases, unfavorable prognosis, and failure of immunotherapy. Multiple immune escape pathways have been reported, among which the most notable one is the recruitment of suppressive immune cells by tumors, such as Tregs and macrophages, thereby promoting immune escape [Citation8]. The most prominent immune checkpoints are cytotoxic T lymphocyte-associated protein 4 (CTLA-4), programmed cell death protein 1 (PD-1), and programmed death-ligand 1 (PD-L1), allowing tumors to escape immune surveillance [Citation11,Citation12]. In addition, cytokines, including vascular endothelial growth factor (VEGF) and transforming growth factor-beta (TGF-β), are also involved in immune escape [Citation13,Citation14]. Winerdal M. E. et al. [Citation15] showed that tumor-infiltrating CD4+ FOXP3+ T cells in urinary bladder cancer are phenotypically, functionally, and epigenetically represent an actual Treg population. Increased numbers of CD4' FOXP3' T-cells have been observed in the peripheral blood of patients with urinary bladder cancer and other forms of cancer [Citation16,Citation17]. Infiltration of CD3' and CD68' cells in bladder cancer is subtype-specific and affects the outcome of patients with muscle-invasive tumors [Citation18]. CD8' T effector and immune checkpoint signatures predict prognosis and responsiveness to immunotherapy in bladder cancer [Citation19]. Over the past few years, immunotherapies have attracted extensive attention as a promising approach to cancer treatment. In bladder cancer, immunotherapeutic targets are under development, and clinical studies currently underway have been reported [Citation20]. The FDA designated MPDL3280A, an anti-programmed cell death protein ligand 1 (anti-PD-L1) antibody, as a breakthrough therapy in 2014 for metastatic urothelial carcinoma. Since bladder cancer has long been considered immune responsive [Citation21], investigating immune escape-related factors would lead to new avenues in bladder cancer immunotherapy.
The human genome sequencing showed that protein-coding genes account for 3% of human DNA. Over 80% of our genome is actively transcribed into various RNAs without protein-coding potentials [Citation22,Citation23]. Non-coding RNAs are the name given to such transcripts (ncRNAs), which are generally classified into two groups: those longer than 200 nts (nucleotides), namely long non-coding RNAs (lncRNAs), and those less than 200 nts, namely small non-coding RNAs (sncRNAs). Small ncRNAs, particularly microRNAs (miRNAs), have been widely researched during the past 20 years, and their functions have been explained; however, the functional roles of lncRNAs are still poorly understood.
This study calculated the ESTIMATE scores of 411 bladder cancer cases from TCGA-BLCA using the Estimate algorithm. Also, the Least Absolute Shrinkage and Selection Operator (LASSO) algorithm was conducted to establish a risk score model and identify potential candidates who contributed to immune escape and are feasible in treating bladder cancer patients.
Materials and methods
Data source
The expression profile was obtained from TCGA-BLCA (download from UCSC Xena, https://xena.ucsc.edu/). The patients’ detailed information is shown in .
Table 1. Information of patients in the training set and validating set.
ESTIMATE algorithm and scoring
ESTIMATE algorithm-generated stromal, immune, and ESTIMATE scores using a single-sample gene set-enrichment analysis (ssGSEA) as previously described [Citation24,Citation25]. Gene expression values for a given sample were normalized and ordered following their rank. The empirical cumulative distribution functions (ECDFs) were calculated for the signature and remaining genes. Statistical data was generated using the integration of the difference between ECDF, similar to the one used in gene set enrichment analysis (GSEA), while based on absolute expression than differential expression. ssGSEA was defined using signatures associated with stromal tissue and immune cell infiltration as stromal and immune scores, and the stromal and immune scores were combined to form the “ESTIMATE score.” ESTIMATE scores were calculated for each case in TCGA-BLCA.
Survival analyses using the Kaplan–Meier method
Based on the median expression value, cases in TCGA-BLCA were grouped into high- and low-gene expression groups. The correlation of candidate genes with the overall survival of bladder cancer patients in TCGA-BLCA was analyzed using the Kaplan–Meier method and a two-tailed log-rank test.
Identification of prognostic signatures
The LASSO algorithm determined the coefficients of the candidate ncRNAs associated with the overall survival of bladder cancer patients, and a 13-ncRNA signature was constructed. The risk score for each patient’s prognostic signature was computed using the LASSO package of the R language, and the formula was as follows: risk score = expression of gene1 × β1gene1 + expression of gene2 × β2gene2 + expression of gene3 × β3gene3 [Citation26,Citation27]. In this study, the formula is:
Risk_score = Exp (RN7SL834P)*-2.3823401497055663+
Exp(AC114730.3)*-3.5620563734151176+
Exp(IGF2BP2-AS1)*13.604331297140993+
Exp(PAXIP1-AS1)*-0.5234230526513313+
Exp(LINC01281)*-34.816318700579814+
Exp(RNF139-AS1)*-3.173975476924686+
Exp(LINC00942)*0.8476240783342311+
Exp(LINC00539)*3.117912084903602+
Exp(ARHGAP5-AS1)*0.20019107602940134+
Exp(OGFR-AS1)*-4.346093589706715+
Exp(LENG8-AS1)*-0.4474753066998284+
Exp(TTC28-AS1)*-5.18286292836644+
Exp(MAFG-AS1)*1.6248303306924285+
Classification performance of the risk score model analyzed by ROC curve
The survival ROC package in R was utilized to construct the 1-, 2-, 3-, 4-, and 5-year ROC curves for predictive accuracy analysis. The association of clinical risk parameters with survival was determined using univariate and multivariate Cox regression analyses to establish genome-clinicopathologic nomograms. Based on the 13-ncRNA signature risk score, age, gender, and grade, Markers were used to develop genome-clinicopathologic nomograms.
Genome-clinicopathologic nomogram
Calibration and discrimination were used to assess model performance through a bootstrap method with 1000-iteration resampling. The calculation of consistency index (C index) was used for discrimination assessment, which is the model’s ability to distinguish between patients who survived and those who did not. Then, the accuracy of actual observed rates and the predicted survival probability was estimated via plotting the calibration curves of the nomogram for 1-, 3-, and 5-year survival probability.
Clinical tissue samples
A total of 9 paired low-grade bladder cancer and adjacent normal tissues (age 59.4 ± 17.0 year, F/M is 1/8) and 9 paired high-grade bladder cancer and adjacent normal tissues (age 64.8 ± 8.1 year, F/M is 1/8) were collected from patients who underwent primary surgical resection at the Xiangya Hospital of Central South University (Changsha, China). All procedures were approved by the Research Ethics Committee of the Xiangya Hospital of Central South University (Changsha, China), and written informed consent was obtained from all patients.
Fluorescence in situ hybridization (FISH) and immunohistochemistry (IHC) assays
Tissue samples were processed and sliced into 3-μm thick specimens for FISH and IHC assays. For FISH analysis of IGF2BP2-AS1, A Specific DIG-labeled lncRNA IGF2BP2-AS1 probe (5’- CCCTCTGAGATTTTCTTTGTTTCTGCTGTTACCTTGACC-3’) was obtained from Servicebio tech (Wuhan, China). After digestion with proteinase K, the sections were prehybridized with a hybridization solution and then incubated with a 500 nM IGF2BP2-AS1 probe in a hybridization buffer overnight at 42°C. After being blocked with rabbit serum, sections were incubated with mouse anti-DIG-HRP for 50 min at 37°C. Then, cells were incubated with Cy3-TSA reagent for 5 min at room temperature in the dark. Finally, the sections were stained with DAPI for 5 min at room temperature. The sections were observed by fluorescence microscopy (Olympus). IGF2BP2-AS1 showed red inflorescence. The average fluorescence was measured by ImageJ software (NIH, USA).
IHC staining was performed according to standard indirect immunoperoxidase methods (Elite ABC kit, Vector Laboratories, Burlingame, CA, USA). Mouse monoclonal antibody or rabbit polyclonal antibody to human PD1, Foxp3, CD68, CD3, and CD8 protein was employed for the detection of PD-1 (ab52587, Cambridge, MA, USA), Foxp3 (CSB-PA15229A0Rb, Cusabio, Wuhan, China), CD68 (25,747-1-AP, Proteintech, Wuhan, China), CD3 (ab8090, Abcam,), and CD8 (ab217344, Abcam). Diaminobenzidine was utilized as a chromogen. Negative controls were obtained by isotype primary antibody incubation. Cellular staining was scored as follows: 0 (staining in <10%, negative), 1 (faint or moderate staining in 10 ~ 50%, weak), 2 (intense staining in 10 ~ 50%, moderate), or 3 (staining in ≥50%, strong). The average score was calculated from 8 independent fields.
Statistical analyses
SPSS (version 22.0) and R language (version 3.5.1; https://www.r-project.org/) were utilized for all statistical analyses. P < 0.05 indicated significant differences. Any missing clinical data resulted in eliminating the entire sample from the analysis. OS referred to the time interval between the date of diagnosis and death. DSS is the percentage of people in a study or treatment group who did not die of a specific disease within a specified time. The comparison of mean value among continuous variables was conducted using t-test analysis. Survival analyses of the high- and low-risk groups were performed using the Kaplan-Meier test, followed by two-sided log-rank tests in the R language to compare survival curves.
Results
Differential non-coding RNAs associated with immune microenvironment scores based on TCGA
For identifying differentially expressed non-coding RNAs associated with the immune microenvironment of bladder cancer, integrative bioinformatics analyses were performed, and the process is shown in . Based on 411 bladder cancer cases with clinical information and expression profile information in TCGA-BLCA, the Estimate algorithm was performed to evaluate the Estimate score of each patient. Bladder cancer cases were classified into high- (N = 206) and low-Estimate (N = 205) score subgroups. Differentially expressed non-coding RNAs (ncRNAs) between these two groups were analyzed, and a total of 202 ncRNAs with significant differences (|logFC|>1, P < 0.05) were identified, including 187 up-regulated and 15 down-regulated (). Kaplan–Meier method and a two-tailed log-rank test were conducted to identify ncRNAs linked to bladder cancer patients’ OS; 29 differential ncRNAs were correlated with immune microenvironment scores (Table S1). Based on these 29 ncRNAs, we classified the 411 bladder cancer patients from the TCGA-BLCA into two categories: Cluster1 (N = 213) and Cluster2 (N = 198), using consistent clustering analysis (). According to the survival analysis and Estimate algorithm score, bladder cancer patients in Cluster1 had a significantly better prognosis () and relatively lower malignancy ()) than Cluster2.
Figure 2. Differential non-coding RNAs associated with immune microenvironment scores based on TCGA (a) ESTIMATE algorithm was performed to calculate the ESTIMATE scores for each case in TCGA-BLCA. Cases were grouped using the median ESTIMATE score as the cutoff. Differentially expressed ncRNAs in two groups were analyzed and shown. (b-c) Consensus clustering was performed to divide the cases into two clusters; overall survival in these two clusters was analyzed using the Kaplan–Meier method and a two-tailed log-rank test. (d) Tumor purity ratio of cases in two clusters. (e) ESTIMATE scores of cases in two clusters. (f) Immune scores of cases in two clusters. (g) Stromal scores of cases in two clusters.
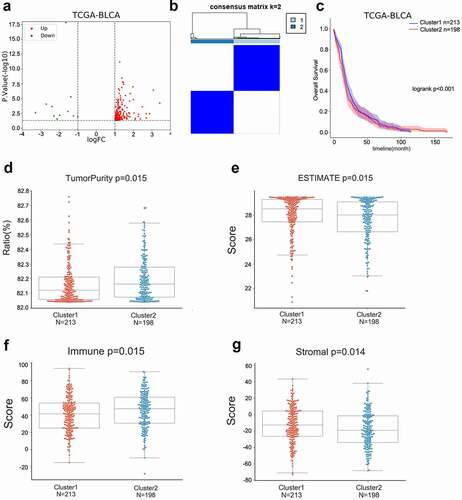
LASSO algorithm was employed to establish a risk score model based on TCGA-BLCA
Based on machine learning, among 411 bladder cancer cases, 406 bladder cancer patients in TCGA-BLCA with complete prognostic information and expression profile information were separated into training group (N = 203) and validation group (N = 203). The LASSO algorithm evaluated the coefficients of 29 ncRNAs and found that 13 were linked to the OS of patients with bladder cancer in the training set ()). Thus, we established a 13-ncRNA risk score model. The risk score model was used to calculate the risk score for each case in the training set, validating set, or TCGA-BLCA; cases in the training set, validating set, or TCGA-BLCA were divided into two groups based on the median risk score. As inferred by the Kaplan–Meier method and a two-tailed log-rank test, Poor prognosis was observed in cases with higher risk scores based on the training set, validating set, or TCGA-BLCA set ()). Using the ROC curve [Citation28], we examined the risk score model’s prediction efficiency. ROC curves determined the prediction efficiency of the risk score model at 1-, 3-, 5-, 8-, and 10-year OS in bladder cancer patients based on TCGA-BLCA. As inferred from , the risk score-based curve showed satisfactory predictive efficiency.
Figure 3. LASSO algorithm was performed to construct a risk score model based on TCGA-BLCA (a-b) The coefficients of candidate ncRNAs were calculated by multivariate Cox regression using LASSO. (c-e) The risk score for each case in the training set, validating set, or TCGA-BLCA was calculated using the risk score model. Cases in the training set, validating set, or TCGA-BLCA were divided into two groups using the median risk score as the cutoff, and the correlation between risk score and bladder cancer patients’ overall survival was analyzed using the Kaplan–Meier method and a two-tailed log-rank test. (f) ROC curves showed the predictive efficiency of the risk score model for 1-, 3-, 5-, 8-, and 10-year overall survival (OS) in bladder cancer patients based on TCGA-BLCA.
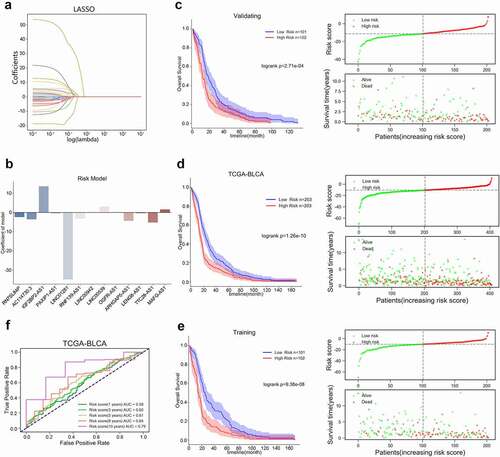
Univariate and multivariate Cox risk regression model analyzing the prognostic potential of clinical characteristics and risk score model
Next, using a multivariate Cox proportional hazard regression model, we analyzed the clinical properties within the high-risk group and low-risk group. A univariate and multivariate Cox risk regression model based on TCGA-BLCA was employed to analyze the correlation of age, gender, stage, and risk score with bladder cancer. and Table S2 showed that, according to TCGA-BLCA, age, stage, and the risk score could predict patients’ OS. Moreover, based on TCGA-BLCA, risk scores were significantly higher in dead cases compared with alive cases (), in cases with no treatment responses compared with cases with treatment response (), in cases with the progressive disease compared with complete remission cases (), and in cluster2 compared with Cluster1 ().
Figure 4. Univariate and multivariate Cox risk regression model analyzing the prognostic value of clinical characteristics and risk score model (a) The correlation of age, gender, stage, and risk score with bladder cancer patients’ overall survival was analyzed using a univariate and multivariate Cox risk regression model based on TCGA-BLCA. Risk scores in cases in different vital statuses (alive vs. dead) (b), with different treatment responses (response vs. no response) (c), with different treatment outcomes (complete remission vs. progressive disease) (d), and different clusters (cluster 1 vs. cluster 2) (e) based on TCGA-BLCA.
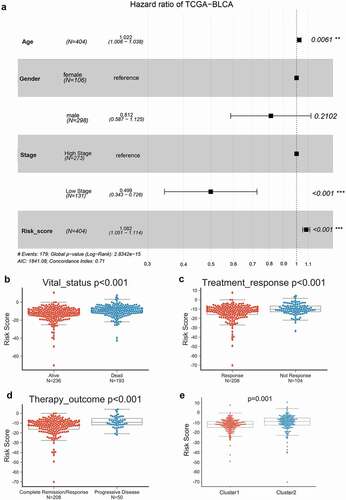
Univariate and multivariate Cox risk regression models identified ncRNAs correlated with the prognosis of bladder cancer patients
To further identify ncRNAs predicting bladder cancer patients’ prognosis, the correlation of RN7SL834P, AC114730.3, IGF2BP2-AS1, PAXIP1-AS1, LINC01281, RNF139-AS1, LINC00942, LINC00539, ARHGAP5-AS1, OGFR-AS1, LENG8-AS1, TTC28-AS1, or MAGF-AS1 expression with bladder cancer patients’ overall survival was analyzed using a univariate and multivariate Cox risk regression model based on TCGA-BLCA. and Table S3 show that IGF2BP2-AS1, MAGF-AS1, ARHGAP5-AS1, and LINC00942 were significantly correlated with bladder cancer patients’ overall survival. The Kaplan–Meier method and a two-tailed log-rank test were employed to analyze the correlation of IGF2BP2-AS1, MAGF-AS1, ARHGAP5-AS1, or LINC00942 with bladder cancer patients’ overall survival. ) shows that lower IGF2BP2-AS1, MAGF-AS1, ARHGAP5-AS1, or LINC00942 expression was correlated with better prognosis in bladder cancer patients.
Figure 5. Univariate and multivariate Cox risk regression model identifying ncRNAs correlated with the prognosis of bladder cancer patients (a) The correlation of RN7SL834P, AC114730.3, IGF2BP2-AS1, PAXIP1-AS1, LINC01281, RNF139-AS1, LINC00942, LINC00539, ARHGAP5-AS1, OGFR-AS1, LENG8-AS1, TTC28-AS1, or MAGF-AS1 expression with bladder cancer patients’ overall survival was analyzed using a univariate and multivariate Cox risk regression model based on TCGA-BLCA. (b-e) The correlation of IGF2BP2-AS1, MAGF-AS1, ARHGAP5-AS1, or LINC00942 with bladder cancer patients’ overall survival was analyzed using the Kaplan–Meier method and a two-tailed log-rank test.
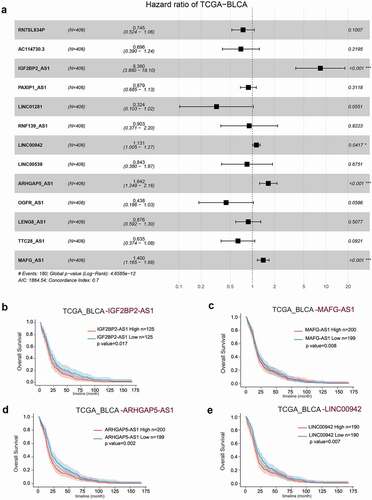
Enriched signaling pathways of ncRNA co-expressed mRNAs
The comprehension of these ncRNAs’ signaling pathways and functions was determined by analyzing the co-expressed mRNAs of IGF2BP2-AS1, MAGF-AS1, ARHGAP5-AS1, or other factors LINC00942 using Pearson’s correlation analysis based on TCGA-BLCA. shows that 2093, 3107, 386, and 936 mRNAs were co-expressed with IGF2BP2-AS1, MAGF-AS1, ARHGAP5-AS1, and LINC00942, respectively, based on TCGA-BLCA as inferred by Pearson correlation analysis (|r|>0.2, p < 0.05).
Figure 6. Co-expressed mRNAs of IGF2BP2-AS1, MAGF-AS1, ARHGAP5-AS1, or LINC00942 were analyzed using Pearson’s correlation analysis concerning the TCGA-BLCA.
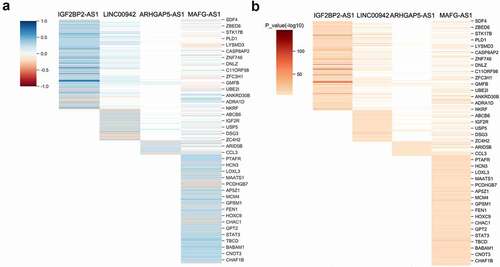
Next, we selected the top 100 co-expressed mRNAs with the most significant P values for functional analysis. The online metascape analysis found that IGF2BP2-AS1 might be associated with cellular glycolipidation, stimulus-response, glucuronide metabolic process, and drug metabolism (). MAFG-AS1 might be associated with cell cycle regulation, monosaccharide catabolic processes, antigen response, and bacterial invasion of epithelial cells (). ARHGAP5-AS1 might be related to cell division, intracellular transport regulation, synthesis, degradation of glycogen, mitochondrial construction, and localization of membrane proteins (). LINC00942 might be related to biological oxidation, epithelial cell differentiation, growth and development, and NRF2 pathway regulation ().
IGF2BP2-AS1 is associated with bladder cancer grade and the levels of immune-related factors in bladder cancer tissues
The FISH assay was performed to confirm the expression and localization of IGF2BP2-AS1 in low- and high-grade bladder cancer and non-cancerous samples. shows that IGF2BP2-AS1 expression was significantly higher in bladder cancer samples, either low- or high-grade, than in non-cancerous normal controls. Moreover, the IGF2BP2-AS1 level was higher in high-grade bladder cancer samples than in low-grade cancer samples (). As aforementioned, PD1, Foxp3, CD3, CD68, and CD8 are critical immune-related markers or immunotherapy targets for bladder cancer [Citation15–21]; therefore, the expression levels of immune microenvironment related proteins PD1, Foxp3, CD68, CD3, and CD8 were examined in low- and high-grade bladder cancer and non-cancerous samples. ) shows that the Foxp3, CD3, and CD8 levels were down-regulated in cancer samples compared with those in non-cancerous controls. They were more evidently down-regulated in high-grade cancer samples compared with low-grade samples. In contrast, PD1 and CD68 were up-regulated in cancer samples compared with those in non-cancerous controls, more significantly up-regulated in high-grade cancer samples than low-grade samples. Pearson’s correlation analysis was performed to validate the correlation between IGF2BP2-AS1 and these factors. IGF2BP2-AS1 was negatively correlated with Foxp3, CD3, and CD8 ()), whereas it was positively correlated with CD68 and PD-1, respectively ()).
Figure 8. The IGF2BP2-AS1 expression was determined by FISH in low-grade bladder cancer tissues, high-grade bladder cancer tissues, and adjacent normal tissues. ** p < 0.01, # p < 0.05.
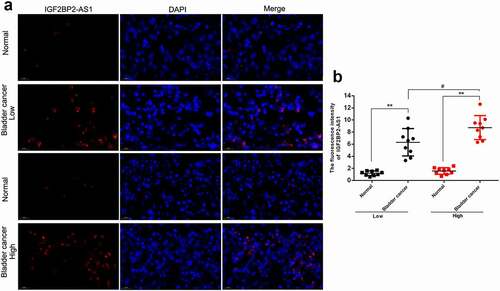
Figure 9. IHC staining (a) showed the expression of CD3 (b), CD8 (c), CD68 (d), FOXP3 (e) and PD-1 (f) in low grade bladder cancer tissues, high grade bladder cancer tissues and adjacent normal tissues. ** p < 0.01, # p < 0.05, ## p < 0.01.
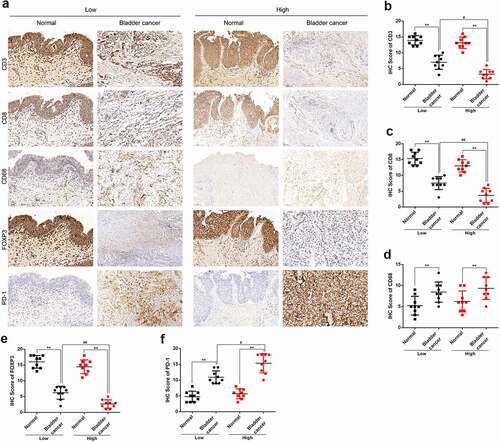
Figure 10. The expression correlation between IGF2BP2-AS1 and immune-related factors in low-grade bladder cancer tissues (black dot) and high-grade bladder cancer tissues (red dot). (a) IGF2BP2-AS1 and CD3; (b) IGF2BP2-AS1 and CD8; (c) IGF2BP2-AS1 and CD68; (d) IGF2BP2-AS1 and FOXP3; (e) IGF2BP2-AS1 and PD-1.
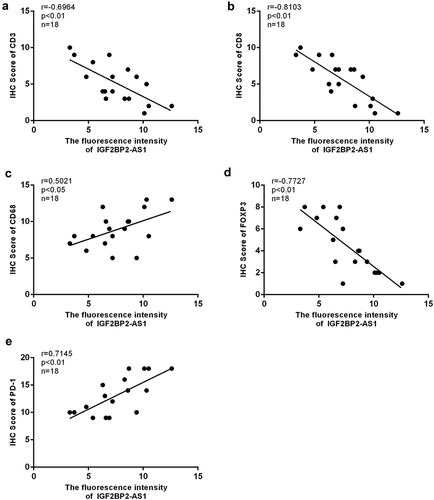
Discussion
In this study, ESTIMATE scores were evaluated for 411 bladder cancer cases from TCGA-BLCA, and the cases were grouped based on the median immune score. A total of 202 ncRNAs were differentially expressed in two groups, and 29 candidates appeared to be associated with the overall survival of bladder cancer patients. LASSO algorithm was performed, and a 13-ncRNA risk score model was established. Risk scores were computed for cases in the training set, validating set, and TCGA-BLCA set, and the prognosis for cases with higher risk scores was poorer based on the training set, validating set, or TCGA-BLCA set. ROC curves indicated the satisfactory prediction efficiency of the risk score model. Among the 13 ncRNAs, IGF2BP2-AS1, MAGF-AS1, ARHGAP5-AS1, and LINC00942 were significantly correlated with bladder cancer patients’ overall survival. Pearson’s correlation analysis based on TCGA-BLCA identified 2093, 3107, 386, and 936 mRNAs co-expressed with IGF2BP2-AS1, MAGF-AS1, ARHGAP5-AS1, and LINC00942, respectively. Co-expressed mRNAs were associated with cell metabolism, division, and differentiation. As the highest HR lncRNA, IGF2BP2-AS1 was up-regulated in bladder cancer tissues and positively related to immune-related factors levels.
Tomczak et al. [Citation29] created the ESTIMATE method (Estimation of STromal and Immune cells in MAlignant Tumor tissues Using Expression data), which computed immune and stromal scores to predict nontumor cell infiltration by evaluating particular gene expression signatures. Since then, by performing the single-sample gene set-enrichment analysis (ssGSEA), we calculated stromal and immune scores to predict the level of infiltrating stromal and immune cells using the ESTIMATE algorithm. [Citation24]. The ESTIMATE algorithm efficiently predicts stromal immune scores and tumor purity [24, 30], making it a suitable tool for evaluating bladder cancer patients’ outcomes based on gene expression profiles. Herein, the ESTIMATE scores for 411 bladder cancer cases in TCGA-BLCA were evaluated. After dividing these cases into two groups (low vs. high ESTIMATE score group), we analyzed differentially expressed ncRNAs and found 29 significantly differentially expressed ncRNAs correlated with bladder cancer patients’ OS. Consensus clustering of 411 bladder cancer cases from TCGA-BLCA based on survival analysis and ESTIMATE scores showed that cases in cluster1 had a better prognosis and lower malignancy than cluster 2, indicating that differentially expressed ncRNAs might play a role in bladder cancer progression in an immune-related way.
Recently, researchers have focused on integrated bioinformatics analysis identifying the gene expression patterns of bladder cancer and developing gene signatures linked with bladder cancer prognosis. For instance, Logistic LASSO regression based on BI-RADS descriptors and CDD outperformed SL in predicting the presence of bladder cancer. Cao et al. [Citation30] discovered a new EMT-associated gene signature that promotes tumor growth, functions as a negative independent prognostic factor, and might contribute to customized counseling and therapy in bladder cancer. Chen et al. [Citation31] indicated that m6A RNA methylation regulators could contribute to the tumor development of bladder cancer, and a risk signature containing three selected m6A RNA methylation regulators might be a potential prognostic biomarker guiding customized therapy for bladder cancer patients. In this study, the LASSO algorithm evaluated the coefficients of 29 ncRNAs, and 13 were correlated with the OS of bladder cancer patients in the training set. Thus, a 13-ncRNA risk score model was established. Through survival analysis, the risk score was significantly associated with the prognosis of bladder cancer patients, and the ROC curve also confirmed the satisfactory prediction efficiency.
As aforementioned, ncRNAs, particularly long non-coding RNAs (lncRNAs), have critical functions in bladder cancer development [Citation32,Citation33]. A series of critical lncRNAs, including H19, UCA1, and MALAT1, have been shown to participate in bladder cancer cell phenotype and regulate networks governing EMT (epithelial-to-mesenchymal transition) in the invasion-metastasis cascade during bladder cancer progression [Citation33]. Moreover, MALAT1, PCAT-1, and SPRY4-IT1, as well as exosomal H19 and PTENP derived from blood serum and urine, have been proposed as underlying noninvasive biomarkers [Citation33]. The 13 ncRNAs that comprise the risk score model were subsequently applied for a univariate and multivariate Cox risk regression analysis to identify ncRNAs associated with bladder cancer patients’ overall survival. IGF2BP2-AS1, MAGF-AS1, ARHGAP5-AS1, and LINC00942 were significantly correlated with bladder cancer patients’ overall survival. LINC00942 has been recognized as a component of a ferroptosis-related long non-coding RNAs (FRlncRNAs) signature [Citation34] and an immune-related lncRNA signature [Citation35]. MAGF-AS1 is recognized as an oncogenic lncRNA in bladder cancer [Citation36–38]. At the same time, the roles of IGF2BP2-AS1 and ARHGAP5-AS1 in bladder cancer remain unclear.
To comprehend the roles and possible mechanisms of IGF2BP2-AS1, MAGF-AS1, ARHGAP5-AS1, and LINC00942 in bladder cancer, co-expressed mRNAs of these four ncRNAs were analyzed and subjected to Metascape online analysis. IGF2BP2-AS1 might be associated with cellular glycolipidation, stimulus-response, glucuronide metabolic process, and drug metabolism. Notably, in the cancer-immune microenvironment, stimulus-response is vital. Neoantigens cause the immune system to activate naive T cells, counteracting the anticancer cytotoxic response. In conjunction with the TCR/antigen-mediated stimulus, accessory stimulatory or inhibitory molecules provide a secondary signal that modifies the intensity of the activation signal at the immunological synapse [Citation39]. IGF2BP2-AS1 is a lncRNA located in the IGF2BP2 antisense strand, whereas IGF2BP2 is one of the RNA binding proteins (RBPs), the most critical post-transcriptional regulatory protein. Notably, IGF2BP2 was significantly up-regulated in bladder cancer J82, T24, 5637, and RT4 cells [Citation40]. IGF2BP2 enhances various types of cancer [Citation41,Citation42], and IGF2BP2-AS1 could modulate the expression level of IGF2BP, thereby affecting bladder cancer onset and progression. MAFG-AS1 might be associated with cell cycle regulation, monosaccharide catabolic processes, antigen response, and bacterial invasion of epithelial cells.
Similarly, antigen response is a critical event in the cancer-immune microenvironment [Citation39]. The effective presentation of tumor antigens by antigen-presenting cells and identification of these antigens by MHC I and II are required for T-cell activation. T-cells recognize MHC-bound antigens and use co-stimulatory factors to enhance T-cell proliferation [Citation43]. The CTLA-4 and PD-1/PD-L1 pathways are crucial in tumor evasion by suppressing the immune response [Citation44]. ARHGAP5-AS1 might be related to cell division, regulation of intracellular transport, synthesis and degradation of glycogen, mitochondrial construction, and localization of membrane proteins. It has been previously demonstrated that impaired autophagy of lncRNA ARHGAP5-AS1 could promote chemotherapy resistance in gastric cancer [Citation45]. Another group reported contradicting findings that ARHGAP5-AS1 suppresses the migration of breast cancer cells by stabilizing SMAD7 protein [Citation46]. LINC00942 might be engaged in oxidation, epithelial cell differentiation, growth and development, and NRF2 pathway regulation. In the Keap1f/f/Ptenf/f lung cancer mice model, the immune milieu was dramatically changed by Keap1 and Pten deletion, and tumor regression was achieved by inhibiting immune checkpoints [Citation47]. Although the specific functions of the NRF2 pathway regulation in bladder cancer have not been investigated, its correlation with immune checkpoint inhibition in non-small cell lung cancer suggests the potential role in bladder cancer immune microenvironment.
Tumor-infiltrating lymphocytes (TILs) and tumor-associated macrophages are vital representatives of the tumor immune microenvironment; they have been shown to provide prognostic and predictive biomarkers in many cancers, including breast cancer [Citation48,Citation49], colorectal cancer [Citation50,Citation51], sarcomas [Citation52], and urothelial carcinoma [Citation18]. PD1, Foxp3, CD3, CD68, and CD8 are critical immune microenvironment markers or immunotherapy targets for bladder cancer [Citation15–21]. In this study, a total of 4 T-cell markers, CD3 for T cells in general, CD8 for CTLs, FOXP3 for Tregs [Citation53], and PD1 + T lymphocytes [Citation54], and macrophage-specific marker CD68 for macrophages [Citation55] were evaluated using IHC to evaluate infiltrating immunological cells in bladder cancer. In bladder cancer samples, especially high-grade samples, the levels of T-cell markers (except for PD1 + T lymphocytes) were significantly down-regulated while the macrophage marker was up-regulated. Moreover, IGF2BP2-AS1 was negatively correlated with T-cell markers except for PD1, whereas positively correlated with macrophage infiltration. This indicates that in most cases high IGF2BP2-AS1 expression was accompanied by high macrophage infiltration and T-cell reduction. Regarding PD1 and its ligand, programmed cell death 1 ligand 1 (PD-L1, CD274, B7-H1), they comprise one of the main immune checkpoint pathways that downregulates immune activity [Citation56]. When PD-1 binds one of its two known ligands, PD-L1 and programmed cell death 1 ligand 2 (PD-L2, CD273), T-cell function is downregulated as manifested by decreased IL2 and IFNγ production. Thus, IGF2BP2-AS1 might contribute to bladder cancer progression through affecting tumor immune microenvironment, which needs functional experimental investigations.
Conclusions
Conclusively, the 13-ncRNA signature has an excellent predictive value in the prognosis of bladder cancer patients. IGF2BP2-AS1 represents a promising immune-related target for treating bladder cancer patients.
Authors’ contributions
KD wrote this manuscript with contributions from all authors and developed the conceptual design of the study. ZZ and YH contributed to the clinical sample collection and data. XH directed all aspects of the project, from concept, design, and engineering to experimentation. All the authors have reviewed and approved the final manuscript.
Ethics approval and consent to participate
All applicable Medical Ethics Committee of Xiangya Hospital Central South University. Application No. 202104145.
Availability of data and materials
All data generated or analyzed during this study are included in this published article.
Supplemental Material
Download MS Word (22.6 KB)Disclosure statement
No potential conflict of interest was reported by the author(s).
Supplementary material
Supplemental data for this article can be accessed online at https://doi.org/10.1080/15384101.2022.2103898
Additional information
Funding
References
- Richters A, Aben KKH, Kiemeney L. The global burden of urinary bladder cancer: an update. World J Urol. 2020;38(8):1895–1904.
- Siegel RL, Miller KD, Jemal A. Cancer statistics, 2019. CA Cancer J Clin. 2019;69:7–34.
- Kirkali Z, Chan T, Manoharan M, et al. Bladder cancer: epidemiology, staging and grading, and diagnosis. Urology. 2005;66:4–34.
- Shariat SF, Sfakianos JP, Droller MJ, et al. The effect of age and gender on bladder cancer: a critical review of the literature. BJU Int. 2010;105:300–308.
- Freedman ND, Silverman DT, Hollenbeck AR, et al. Association between smoking and risk of bladder cancer among men and women. JAMA. 2011;306:737–745.
- Chang SS, Boorjian SA, Chou R, et al. Diagnosis and treatment of non-muscle invasive bladder cancer: AUA/SUO guideline. J Urol. 2016;196:1021–1029.
- Wallis CJ, Mahar AL, Choo R, et al. Second malignancies after radiotherapy for prostate cancer: systematic review and meta-analysis. BMJ. 2016;352:i851.
- Liu Y, Cao X. Immunosuppressive cells in tumor immune escape and metastasis. J Mol Med (Berl). 2016;94:509–522.
- Sanmamed MF, Chen L. A paradigm shift in cancer immunotherapy: from enhancement to normalization. Cell. 2018;175:313–326.
- Sanmamed MF, Chen L. A paradigm shift in cancer immunotherapy: from enhancement to normalization. Cell. 2019;176(3):677.
- Nakanishi J, Wada Y, Matsumoto K, et al. Overexpression of B7-H1 (PD-L1) significantly associates with tumor grade and postoperative prognosis in human urothelial cancers. Cancer Immunol Immunother. 2007;56(8):1173–1182.
- Chen DS, Mellman I. Elements of cancer immunity and the cancer-immune set point. Nature. 2017;541(7637):321–330.
- Mariathasan S, Turley SJ, Nickles D, et al. TGFbeta attenuates tumour response to PD-L1 blockade by contributing to exclusion of T cells. Nature. 2018;554(7693):544–548.
- Fukumura D, Kloepper J, Amoozgar Z, et al. Enhancing cancer immunotherapy using antiangiogenics: opportunities and challenges. Nat Rev Clin Oncol. 2018;15(5):325–340.
- Winerdal ME, Krantz D, Hartana CA, et al. Urinary bladder cancer tregs suppress MMP2 and potentially regulate invasiveness. Cancer Immunol Res. 2018;6(5):528–538.
- Wolf AM, Wolf D, Steurer M, et al. Increase of regulatory T cells in the peripheral blood of cancer patients. Clin Cancer Res. 2003;9(2):606–612.
- Loskog A, Ninalga C, Paul-Wetterberg G, et al. Human bladder carcinoma is dominated by T-regulatory cells and Th1 inhibitory cytokines. J Urol. 2007;177(1):353–358.
- Sjodahl G, Lovgren K, Lauss M, et al. Infiltration of CD3(+) and CD68(+) cells in bladder cancer is subtype specific and affects the outcome of patients with muscle-invasive tumors. Urol Oncol. 2014;32(6):791–797.
- Chen X, Xu R, He D, et al. CD8(+) T effector and immune checkpoint signatures predict prognosis and responsiveness to immunotherapy in bladder cancer. Oncogene. 2021;40:6223–6234.
- Kim JW, Tomita Y, Trepel J, et al. Emerging immunotherapies for bladder cancer. Curr Opin Oncol. 2015;27:191–200.
- Brandau S, Suttmann H. Thirty years of BCG immunotherapy for non-muscle invasive bladder cancer: a success story with room for improvement. Biomed Pharmacother. 2007;61:299–305.
- Consortium EP. An integrated encyclopedia of DNA elements in the human genome. Nature. 2012;489:57–74.
- Djebali S, Davis CA, Merkel A, et al. Landscape of transcription in human cells. Nature. 2012;489:101–108.
- Yoshihara K, Shahmoradgoli M, Martinez E, et al. Inferring tumour purity and stromal and immune cell admixture from expression data. Nat Commun. 2013;4:2612.
- Bindea G, Mlecnik B, Tosolini M, et al. Spatiotemporal dynamics of intratumoral immune cells reveal the immune landscape in human cancer. Immunity. 2013;39:782–795.
- Liu L, Lin J, He H. Identification of potential crucial genes associated with the pathogenesis and prognosis of endometrial cancer. Front Genet. 2019;10:373.
- Wang X, Fu X, Zhang J, et al. Identification and validation of m(6)A RNA methylation regulators with clinical prognostic value in Papillary thyroid cancer. Cancer Cell Int. 2020;20:203.
- Hanley JA, McNeil BJ. The meaning and use of the area under a receiver operating characteristic (ROC) curve. Radiology. 1982;143:29–36.
- Tomczak K, Czerwinska P, Wiznerowicz M. The Cancer Genome Atlas (TCGA): an immeasurable source of knowledge. Contemp Oncol (Pozn). 2015;19:A68–77.
- Cao R, Yuan L, Ma B, et al. An EMT-related gene signature for the prognosis of human bladder cancer. J Cell Mol Med. 2020;24:605–617.
- Chen Y, Wang X. Wang X. miRDB: an online database for prediction of functional microRNA targets. Nucleic Acids Res. 2019;48:D127–D31.
- Li Y, Li G, Guo X, et al. Non-coding RNA in bladder cancer. Cancer Lett. 2020;485:38–44.
- Cao Y, Tian T, Li W, et al. Long non-coding RNA in bladder cancer. Clin Chim Acta. 2020;503:113–121.
- Cui Y, Zhou Z, Chai Y, et al. Identification of a nomogram from ferroptosis-related long noncoding RNAs signature to analyze overall survival in patients with bladder cancer. J Oncol. 2021;2021:8533464.
- Zhang L, Li L, Zhan Y, et al. Identification of Immune-Related lncRNA signature to predict prognosis and immunotherapeutic efficiency in bladder cancer. Front Oncol. 2020;10:542140.
- Li D, Zhong S, Zhu Z, et al. LncRNA MAFG-AS1 promotes the progression of bladder cancer by targeting the miR-143-3p/COX-2 axis. Pathobiology. 2020;87:345–355.
- Xiao M, Liu J, Xiang L, et al. MAFG-AS1 promotes tumor progression via regulation of the HuR/PTBP1 axis in bladder urothelial carcinoma. Clin Transl Med. 2020;10(8):e241.
- Tang C, Wu Y, Wang X, et al. LncRNA MAFG-AS1 regulates miR-125b-5p/SphK1 axis to promote the proliferation, migration, and invasion of bladder cancer cells. Hum Cell. 2021;34:588–597.
- Russell BL, Sooklal SA, Malindisa ST, et al. The tumor microenvironment factors that promote resistance to immune checkpoint blockade therapy. Front Oncol. 2021;11:641428.
- Wu Y, Liu Z, Wei X, et al. Identification of the functions and prognostic values of RNA binding proteins in bladder cancer. Front Genet. 2021;12:574196.
- Barghash A, Golob-Schwarzl N, Helms V, et al. Elevated expression of the IGF2 mRNA binding protein 2 (IGF2BP2/IMP2) is linked to short survival and metastasis in esophageal adenocarcinoma. Oncotarget. 2016;7(31):49743–49750.
- Ye S, Song W, Xu X, et al. IGF2BP2 promotes colorectal cancer cell proliferation and survival through interfering with RAF-1 degradation by miR-195. FEBS Lett. 2016;590(11):1641–1650.
- Schumacher TN, Schreiber RD. Neoantigens in cancer immunotherapy. Science. 2015;348:69–74.
- Freeman GJ, Long AJ, Iwai Y, et al. Engagement of the PD-1 immunoinhibitory receptor by a novel B7 family member leads to negative regulation of lymphocyte activation. J Exp Med. 2000;192(7):1027–1034.
- Zhu L, Zhu Y, Han S, et al. Impaired autophagic degradation of lncRNA ARHGAP5-AS1 promotes chemoresistance in gastric cancer. Cell Death Dis. 2019;10:383.
- Wang CL, Li JC, Zhou CX, et al. Long non-coding RNA ARHGAP5-AS1 inhibits migration of breast cancer cell via stabilizing SMAD7 protein. Breast Cancer Res Treat. 2021;189(3):607–619.
- Best SA, De Souza DP, Kersbergen A, et al. Synergy between the KEAP1/NRF2 and PI3K pathways drives non-small-cell lung cancer with an altered immune microenvironment. Cell Metab. 2018;27(4):935–43 e4.
- Romagnoli G, Wiedermann M, and Hubner F, et al. Morphological evaluation of Tumor-Infiltrating Lymphocytes (TILs) to investigate invasive breast cancer immunogenicity, reveal lymphocytic networks and help relapse prediction: a retrospective study. Int J Mol Sci. 2017;18:1936.
- Kuroda H, Jamiyan T, Yamaguchi R, et al. Tumor microenvironment in triple-negative breast cancer: the correlation of tumor-associated macrophages and tumor-infiltrating lymphocytes. Clin Transl Oncol. 2021;23:2513–2525.
- Ye L, Zhang T, Kang Z, et al. Tumor-infiltrating immune cells act as a marker for prognosis in colorectal cancer. Front Immunol. 2019;10:2368.
- Narayanan S, Kawaguchi T, Peng X, et al. Tumor infiltrating lymphocytes and macrophages improve survival in microsatellite unstable colorectal cancer. Sci Rep. 2019;9:13455.
- Dancsok AR, Gao D, Lee AF, et al. Tumor-associated macrophages and macrophage-related immune checkpoint expression in sarcomas. Oncoimmunology. 2020;9(1):1747340.
- Fontenot JD, Gavin MA, Rudensky AY. Foxp3 programs the development and function of CD4+CD25+ regulatory T cells. Nat Immunol. 2003;4:330–336.
- Kather JN, Suarez-Carmona M, Charoentong P, et al. Topography of cancer-associated immune cells in human solid tumors. Elife. 2018;7. DOI:10.7554/eLife.36967.
- Holness CL, Simmons DL. Molecular cloning of CD68, a human macrophage marker related to lysosomal glycoproteins. Blood. 1993;81:1607–1613.
- Baumeister SH, Freeman GJ, Dranoff G, et al. Coinhibitory pathways in immunotherapy for cancer. Annu Rev Immunol. 2016;34:539–573.