Abstract
Objective: This article explores the effects of earlier emergency medical services (EMS) or automatic collision notification (ACN) and EMS arrival on passenger/driver survivability within the short time frame following traffic crashes.
Methods: Survival analysis techniques are used extensively in this study, because traffic crash and EMS data are closely associated with time. The Kaplan-Meier estimator and life curves are applied to compare the survival rates between 2 or more conditions (e.g., earlier verus late EMS notification); The Weibull model with 3 parameters is used to predict mortality over time; furthermore, the Cox proportional hazard model explores multiple risk factors related to traffic mortality.
Results: Based on Fatality Analysis Reporting System (FARS) data from 2005 to 2009, Kaplan-Meier life curves clearly showed the benefits associated with earlier notifications (approximately 1.84% fatality reduction within a time frame of 6 h after a crash) and earlier arrivals, and the Weibull model with 3 parameters reasonably predicted the fatality trends. The relative risks (RRs) associated with collision notification, arrival, location, and age were obtained from a multiple Cox regression model, and the relatively higher fatality hazard (2.4% higher) associated with the later notification of more than 1 min was studied in detail.
Conclusions: This article obtains the driver/passenger survival probabity differences over time under different conditions of collision notifications, EMS arrivals, and crash locations; furthermore, this analysis provides an estimation of the lives that could potentially be saved (approximately 154 to 290 per year) due to earlier ACN.
Introduction
Recent Fatality Analysis Reporting System (FARS) data indicate that there are approximately 33,000 traffic fatalities per year in the United States (33,883 in 2009, 32,999 in 2010, and 32,367 in 2011), and it has been a continuing and challenging effort for both automotive manufacturers and government agencies to reduce traffic fatalities by all possible means and new techniques. Automatic collision notification (ACN) systems have been available from some automobile manufacturers since the late 1990s. One benefit of these systems is that they enable emergency response personnel to receive earlier notification and location information related to a car crash. In crashes of moderate (usually requiring an air bag deployment) or greater severity, ACN systems initiate a call, often routed through a third-party telematics provider (e.g., OnStar, Agero), that notifies emergency personnel of the existence and location of the crash. More recently, these ACN systems have evolved to advanced ACN (AACN) systems that have all of the capabilities of prior ACN systems, together with the additional capability of transmitting relevant vehicle crash data (change in velocity or delta V, seat belt use, direction of impact), and these capabilities from ACN and AACN can be used to predict the severity of a crash and the associated potential for severe injuries. The current study only considers the time-based aspect of earlier notification that is applicable to both ACN and AACN systems.
Prior extensive research has attempted to document the potential that ACN may have in reducing motor vehicle crash fatalities. In a study with a relatively small fleet of vehicles equipped with ACN, Kanianthra et al. (2001) documented that emergency medical service (EMS) providers received notifications within 2 min of a crash in all cases for vehicles equipped with ACN, whereas 20 percent of nonequipped vehicles took over 5 min. Their study estimated that 240 to 765 lives could be saved with ACN based on time distribution of fatalities in FARS and confirmed in the National Automotive Sampling System–Crashworthiness Data System. Clark and Cushing (Citation2002) estimated the potential benefits of ACN through analysis of 1997 FARS data. Using survival analysis techniques, they estimated the fatality numbers within several time intervals or “states” (from crash to notification, from crash or notification to EMS arrival, and after arrival) while assuming notification times to be 1 min for all. A model matching FARS fatalities only estimated a total annual reduction of 421 fatalities (1.5%), and the second model, using an estimate for the total number of incapacitating injuries in the United States, estimated a reduction of 1674 fatalities (6.0%). Lahausse et al. (2008) estimated potential lives saved in Australia with full implementation of ACN, and their study used various data sources for urban and rural crashes. They also attempted to estimate ACN benefits given a change in EMS notification time to be 1 min for all. The average EMS notification time reduction was 6 min for rural areas and 3 min for urban areas. Based on these reductions it was estimated that approximately 11 percent of passenger vehicle occupant fatalities (41 urban and 63 rural) would be saved with full introduction of ACN. The European Commission's eCall program (European Commission Citation2009) published a final report regarding its crash notification–related efforts. Their report included a summary of a series of 10 studies looking at the potential impact of crash notification in Europe. As with this study, the benefits were limited to those that related to ACN systems (i.e., earlier notification) and not the expanded benefits that may be possible with AACN. The estimated motor vehicle crash occupant fatality reduction with ACN in the cited studies ranged from 1 to 12 percent.
Existing research has not yet dealt with the relative comparisons of different conditions statistically with sufficiently larger data sizes; for instance, to compare earlier versus later notification outcomes or earlier versus later arrival outcomes. Some relatively older data (FARS 1997) may not reflect today's EMS call status well (Clark and Cushing Citation2002), and the work done by Toyota (2011) provides clear comparisons of notification and arrival times with and without ACN but also proposed prior assumptions on the possible correlations between EMS notification, timeliness of arrival, and survivability, most of which have not yet been substantiated with real-world data (e.g., FARS 2005–2009). Though the authors are inspired by earlier research (Clark and Cushing Citation2002; Kanianthra et al. 2001; Lahausse et al. 2008; Toyota 2011), more effort is still needed to understand complicated ACN technique such as
• | What the overall trend in probability of survival of drivers/ passengers in passenger cars or light trucks is and the desired data samples chosen for this purpose. | ||||
• | What the impact of earlier or automatic notification on occupant survivability—with quantitative examples and P values (e.g., notification within 1 minute) is. Is there a potential reduction in fatalities with effective ACN applied to all vehicles, and how many reductions? | ||||
• | Survival rate comparisons of varied EMS arrival times and the impact of earlier EMS arrival on survivability—with quantitative examples (e.g., EMS response within 15 min). | ||||
• | The comparison of EMS response time and survivability in rural versus urban areas, especially focusing on the importance of timely EMS arrival in sparsely populated rural areas. | ||||
• | The exploration of relationship between the traffic fatality hazard with several risk factors, such as later notification, rural area, and older age, simultaneously. |
The earlier notification with earlier hospitalization may all play a significant role in mitigating the effects of the injuries suffered in a crash. Research has also indicated that proximity to advanced trauma care may be a key factor in mitigating injury outcome (National Highway Traffic Safety Administration Citation2011). Because time is of the essence in EMS response to such situations, driver/passenger survival rates can vary significantly between different time conditions (e.g., time elapsed before or after the notification call). This is especially exacerbated in the case of single-vehicle crashes in rural areas that may incapacitate a lone vehicle occupant, which may lower the likelihood an early call to emergency services. Therefore, the time-to-event survival analysis technique is used extensively in this research to address the time effect. This study explores the relative comparisons between different conditions or groups, such as survival rates with earlier notification versus late notification and survivability in rural areas versus urban areas. For this purpose of relative comparison, the concept of relative risk (RR) in survival analysis is used, along with the application of Kaplan-Meier life curves (Kaplan and Meier Citation1958) and the Cox proportional hazard model (Cox Citation1972). Further, the Weibull model (Abernethy Citation1999; Allison Citation1999), unlike the nonparametric approach of Kaplan-Meier curves but with 3 parameters, is used to plot the fatality rate over time and predict future trends. A larger data set from the National Highway Traffic Safety Administration's FARS (2005–2009), with relatively new statistics (compared to one year of data from FARS 1997 studied by Clark and Cushing [Citation2002]) on EMS calls and arrivals, was used in this study. Hence, the primary objective of this study was to assess the benefits of earlier notification within 1 min and potential lives saved and to better understand the complicated ACN technique from 3 different perspectives of survival probability curves (nonparametric Kaplan-Meier estimator), fatality trend prediction (parametric Weibull plot), and multiple risk analysis (Cox model).
Data Descriptive Summary
Research suggests that EMS is most helpful for drivers and passengers of passenger cars and light trucks if received within 6 h following a crash (Clark and Cushing Citation2002). Data were compiled using the 2005–2009 FARS data with specific evaluation of the 6-h postcrash time frame (EMS notification/arrival within 6 h after a crash; data with missing notification/arrival information were excluded).
Data cleaning was performed in FARS data verification and time variable coding by the authors. For each crash coded in FARS, the following times are recorded: crash, EMS notification, EMS arrival at scene of crash, EMS arrival at hospital, and time of death (see for definitions of time events and time intervals). These time–date values were used to generate the time intervals needed for the time-to-event or survival analysis. Note that only crashes with complete time–date information were used. See for the data flowchart for this study. During the time frame of 6 h postcrash, there were 59,049 fatalities associated with known EMS notification and arrival times (see ; approximately 53% of the data sample), and 17,187 died instantly.
Fig. 1 Crash time events and time elapsed between crash (C), notification (N), arrival (A), and death (D) (color figure available online).
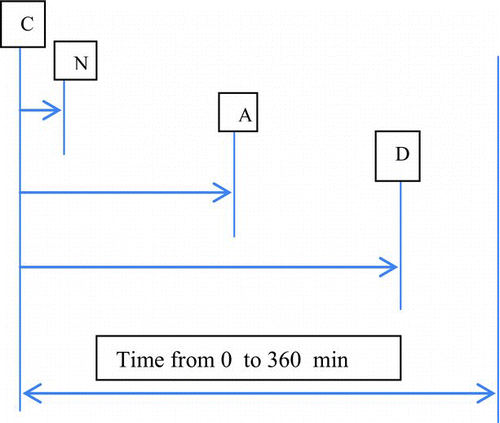
Table 1 FARS 2005–2009 population sampled in study
displays the remaining percentage of those 59,049 fatalities, those who were alive at the scene, over time. At time 0, there were 17,187 instantaneous deaths (29.1% of 59,049 total deaths, and the survival probability dropped from 100% to around 71% at time 0, as shown in ). Furthermore, the calculations associated with indicate the following vital information: during the relatively short time frame from crash to EMS notification ( is again helpful for understanding the time events and intervals of a typical crash), there were 20,295 fatalities (including 17,187 instantaneous deaths at time 0 and 3108 between time zero and the call); after EMS notification there were 38,754 fatalities (during the interval of notification to death). During the time frame of crash to EMS arrival, there were 25,289 fatalities and after arrival there were 33,760.
Fig. 2 Time between crash and death within 6 h after a crash with inj_SEV = 4 (17,187 instantaneous deaths at time = 0 and 59,049 total deaths at time = 360 min) (color figure available online).
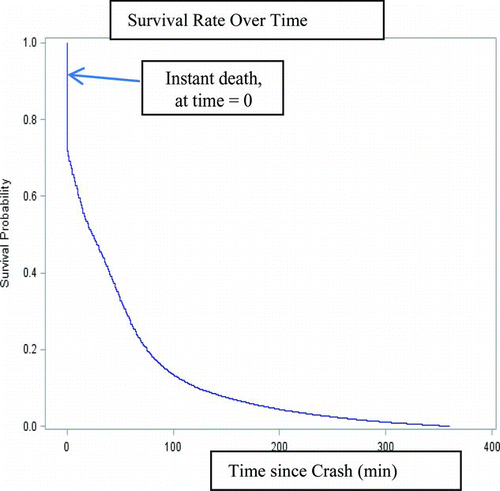
FARS uses the key injury variable of “inj_SEV” to indicate injury severity as follows: 0 = no injury; 1 = possible injury; 2 = nonincapacitating evident injury; 3 = incapacitating injury; 4 = fatal injury; 5 = injured, severity unknown; 6 = died prior to crash; and 9 = unknown. For the purposes of this study, injury severities of 3 (incapacitating injury) and 4 (fatal injury) are focused on in detail.
Though 17,187 instantaneous deaths (time 0) were not included in the analysis of EMS effects (because EMS intervention is not beneficial), shows the overall descriptive data summary for the remaining 41,862 deaths, together with the comparison of rural versus urban areas, within the 6-h time window following a crash (with known notification and arrival information). Furthermore, FARS data results with and without missing data are compared, and displays the distribution between rural and urban areas without missing data (64% rural occupants). If missing data were also included (overall data), 61 percent of car occupants were in rural areas and 39 percent were in urban areas. If ACN were available to all vehicles and a 1 min notification is assumed for all, the average EMS notification time reduction would be 6.15 min for rural areas and 3.32 min for urban areas. This reduction in notification times would agree reasonably well with the result of Lahausse et al. (2008).
Table 2 Overall, rural, and urban EMS response times since crash (EMS call, arrival, and time to death, in minutes)
To analyze the survival rate or proportion (i.e., people alive at the scene among all populations within a certain study time frame), the data set in this study included crashes that resulted in at least one fatality, and the results should not be generalized to the whole crash population. The values of inj_SEV = 3, 4 were used in this study, because these 2 categories require EMS help the most. There were 44,003 incapacitating injury cases that were matched with 41,862 fatalities under the same conditions (within 6 h after a crash and with known EMS information) in this cohort study or follow-up study (Breslow and Day Citation1987), and a typical cohort study is described in to compare the subsequent occurrence of traffic injury severities between 2 groups with different EMS response statuses.
Table 3 Typical cohort study of notification versus traffic severity within 6 h after crash (inj_SEV = 3, 4)
Comparing Survival Rates Using the Kaplan-Meier Estimator
It is of great interest to observe the driver/passenger survival probability, S(t), as it varies over time after traffic crashes. One of the most useful tools for comparing the survival probability over time for each minute after a crash was proposed by Kaplan and Meier (Citation1958) and is widely used for medical research and reliability engineering.
The Kaplan-Meier estimator, or life curve, at any time is described by the following formula:
To compare the traffic fatality relative risk or differences between different blocks or groups (such as earlier calls versus late calls), the log-rank test, which compares the Kaplan-Meir life curves and obtains statistical significance with P values, was used. Various factors that may affect traffic fatalities are explored by following three hypotheses related to EMS notification, EMS arrival, and EMS in rural versus urban areas. The SAS Proc LifeTest (SAS Institute, Cary, NC) was used for the calculations (Hosmer and Lemeshow Citation1999; Kaplan and Meier Citation1958).
Hypothesis 1: Earlier Notification May Save Additional Lives
In order to study the survival rates within 6 h (study or research time) following a crash, 44,003 incapacitating injury cases were matched in this cohort study (Kaplan and Meier Citation1958) with 41,862 fatalities under the same conditions, and the total sample was then statistically randomized (each case had the same probability) and blocked (divided into 2 groups based on EMS notification time). Those with survival times longer than 6 h were regarded as censored data or people who were still alive within the current study time. illustrates the concepts of converting calendar time to study time and randomization. The status alive or censored is also relative and changes over time.
The total study sample was first divided into 2 blocks or groups: the preferred or earlier group was “Call ≤1 min after crash and call time is before the time of death”; the rest belonged to a second, nonpreferred group, for the purpose of understanding the effect of earlier notification on fatalities. The survival rate difference between the 2 groups with earlier (≤1 min) or late (>1 min) notifications was explored using Kaplan-Meier life curves as EquationEq. (1), , and display the survival rate curves of the 2 groups within the study time (6 h postcrash). The P value from log-rank test was less than .0001.
Interpreting and Estimating the Additional Lives Saved Due to Earlier Notification From and
As seen in , the earlier notification group (≤1 min) had a cumulative survival rate (alive/total) of 52.14 percent, 1.47 percent higher than the late notification group (>1 min) within 6 h after a crash. Hence, if the late group had made the EMS calls within 1 min like the earlier group did, the possible additional lives saved would be approximately 1.47 percent × (52,335) = 769; this reduction of 769 deaths represents 1.84 percent of the original 41,862 deaths within a time frame of 6 h after a crash during the 5 years between 2005 and 2009, or 154 per year (i.e., the survival rate for the late group would be (26,519 + 769)/52,335 = 52.14 percent, the same as the earlier notification group in ). Data related to notification and arrival times were missing from about 47 percent of the sample population (). If we assume that the missing data would share similar trends, the additional lives saved could have been 290 per year from 2005 to 2009.
Table 4 Survivors and fatalities within 6 h after crash (inj_SEV = 3, 4, P < .0001)
Here are 5 additional points about the earlier notification effect and the analysis method:
1. | If the EMS cutoff time were longer than 6 h in this study, the study population would be slightly larger than the population shown in , although most people died within the first few hours, as seen in : the proper EMS cutoff time depends on when the life curve becomes stabilized or flat after a crash. This method derived the fatality reductions or lives potentially saved due to ACN from a cross-comparison of the survival probabilities of 2 paired life curves at a specific time of 6 h (360 min), and this comparison can also be made at any specific time—that is, 4, 5, or 6 h after a crash—within the time frame. Comparing the survival rate difference of 2 paired groups at a specific time is a common approach used in clinical trials and public health research (Hosmer and Lemeshow 1999). | ||||
2. | The EMS notification cutoff time of 1 min or 2 min may also lead to different estimations of lives saved. | ||||
3. | It is reasonable to assume that the whole FARS population (with or without EMS information within 6 h after crash) would have shared similar EMS response patterns (call/arrival/survival), although some small deviations may occur. For instance, approximately 64 percent of car occupants were rural residents with EMS info available (see ), and approximately 61 percent were rural residents overall if missing data were also included. | ||||
4. | If the study sample were larger to include incapacitating injuries, fatal injuries, nonincapacitating evident injuries, and injured but severity unknown, the data sizes of the earlier and late notifications would also be larger and might contain richer EMS and survival information. , including only injury levels 3 and 4, provides a more conservative and realistic estimation (Clark and Cushing Citation2002). | ||||
5. | This calculation came from 5 years of FARS data; therefore, the means and especially deviations may be significantly different from the result from data for only one year. The data blocking for the preferred and nonpreferred groups, based on notification and arrival (following Hypothesis 2), may also differ from the data divisions done by other researchers; again, as point 1 indicates, this analysis derived the fatality reductions due to ACN from a cross-comparison of the survival probabilities of 2 paired life curves at a specific same time after crash, which may differ from the approaches used by others. Other potential sources of different estimates of potential lives saved with ACN include FARS data with EMS verification and recordation, various time frames after a crash, and statistical calculation (Clark and Cushing Citation2002; Kanianthra et al. 2001; Lahausse et al. 2008; Toyota 2011). |
Hypothesis 2: Earlier EMS Arrival May Save Additional Lives
Similarly, it is hypothesized that earlier EMS arrival may significantly improve crash survivability, based on real-world data. The results from real-world data also indicated that earlier notifications would not always guarantee either earlier arrivals or better survival chances, although there was a correlation between the EMS notification and arrival with a correlation coefficient of 0.37 (). The variable of EMS arrival was hence treated as one independent variable for survival rate comparison. The matched data sample, including the injury levels incapacitating injury (inj_SEV = 3) and fatal injury (inj_SEV = 4), was statistically randomized (each case had the same probability) and blocked (divided into 2 groups based on EMS arrival times) as shown in the following example: the preferred group was defined as “EMS arrival ≤15 min and arrival time was before the time of death”; the rest were defined as the nonpreferred group. The cutoff arrival time of 15 min was suggested by the data in , because the average arrival time was approximately 16 min for the overall population.
Table 5 Correlation between notification and arrival (correlation coefficient = 0.37)
Kaplan-Meier curves were plotted as seen in , and the P value from the log-rank test that compared the 2 groups was less than .0001. provides a cumulative summary of the 6 h following crashes. From and , the preferred group or earlier arrival group (≤15 min) had a cumulative survival rate of 53.89 percent, 6.69 percent higher than the late group (>15 min) at t = 6 h. Therefore, if the late arrival group had also received EMS treatment within 15 min, the possible additional lives saved would also be approximately 6.69 percent × (32,817) = 2195, and this reduction of 2,195 fatalities was approximately 5.2 percent of the original 41,862 deaths of within a time frame of 6 h after a crash during 2005 to 2009, or 439 per year (825 per year if all missing data were included).
Fig. 5 EMS arrivals versus occupant survival rates within 6 h (inj_SEV = 3, 4, P < .0001) (color figure available online).
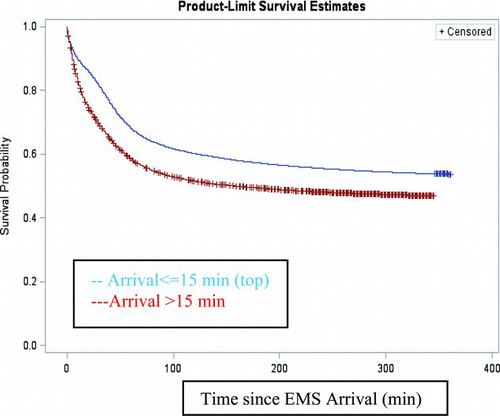
Table 6 Survivors and fatalities within 6 h after a crash (inj_SEV = 3, 4, P < .0001)
Hypothesis 3: Earlier EMS Arrival May Be Especially Vital to Urban Area
Urban areas and rural areas may differ in EMS responses, as shown in (the notification times were 7.15 and 4.32 min for rural and urban areas, respectively). The life curves shown in indicate that the survival rates were similar between the rural and urban areas (P = .19), although the traffic crash notifications and EMS arrival conditions were different. , , and all show similar trends. More explorations will be given in Cox modeling when multiple risk factors are considered simultaneously.
Fig. 6 Occupant survival rates for rural versus urban EMS (inj_SEV = 3, 4, P = .19) (color figure available online).
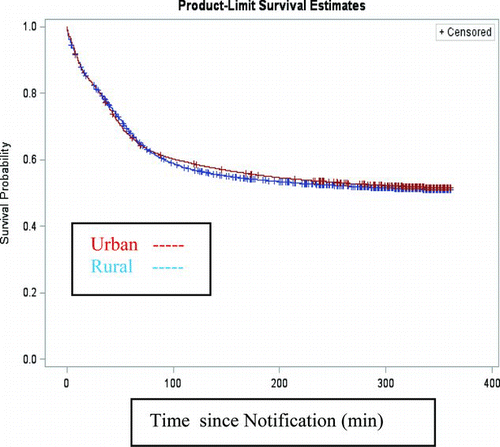
However, the focus of this analysis was to verify the benefit of earlier EMS service to rural areas where more occupants lived from FARS 2005–2009 data, and explores such EMS details using the rural crash data alone, highlighting the importance of timely EMS service to injured people in rural areas. evaluates the impact of earlier EMS arrival on rural areas (6.39% higher survival probability), and the P value for comparison between the 2 groups (≤15 min and arrival before death, vs. >15 min) in rural areas was less than .0001. Furthermore, displays the distribution of the rural population (64%) and the urban population (36%) if missing data were also included; approximately 61 percent of the population lived in rural areas, and timely EMS service to rural areas becomes even more important.
Table 7 Rural versus urban survival rate comparison (inj_SEV = 3, 4, P = .19)
The statistical results yielded from Kaplan-Meier life curves (as seen in – and –) are useful when examining one single factor; if several traffic risk factors, such as time of notification, time of arrival, person's age, etc., are considered simultaneously, the Cox proportional hazard model with multiple risk factors (Cox Citation1972) would be more insightful. In addition, the life curve proposed by Kaplan-Meier needs no assumption of statistical distribution and has no parameters (a so-called nonparametric model), which is not helpful for making predictions in future trends. In contrast, the Weibull model, can reasonably predict future trends with 2 or 3 parameters, as discussed in the following.
Predicting Mortality Rate Using Weibull Plot
As stated earlier, one of objectives was to better understand the traffic fatality and complicated ACN technique from different perspectives. The occupant survivability, S(t), which has been discussed using nonparametric Kaplan-Meier life curves, can be viewed from a different perspective, and S(t) is closely related to the probability of a fatality over time after a crash described by the Weibull model, F(t), equals to 1 − S(t) approximately for the same data. The probability of a fatality, F(t), can be studied in detail and expressed using the following Weibull model equation (Abernethy Citation1999):
Table 8 Survivors and fatalities within 6 h after a crash (inj_SEV = 3, 4, P < .0001), rural areas only
Using FARS 2005–2009 data with earlier notification (≤1 min) only, displays the fatality rate over time (1–360 min) with a cumulative fatality rate of approximately 47.5 percent (or 52.5% survival probability) and the data fitting results of α (scale), β (slope), and t 0 (time shift), which can be used for future trend predictions as well. The data fit for the Weibull model indicates the value of the time shift (threshold) as 1.0 s, which is the time of high peak fatalities. SAS Proc Reliability and LifeReg were used for calculation (Allison Citation1999).
Multiple Relative Risk Model
The goal of this study was to explore the relative comparison of passenger vehicle occupant survival rates, S(t), with one risk factor alone or with several risk factors simultaneously. Cox (Citation1972) proposed a model to consider multiple risk factors simultaneously, and the hazard function, h(t), was introduced. This fatality hazard function, h(t), is actually closely related to the occupant survival probability, S(t), described by Kaplan-Meier life curves, and the fatality hazard function provides a relative change rate of survival probability according to the following equation:
The main focus of this study, however, was to explore the effects of EMS notification, especially arrival, and location on crash survivability, and not all possible risk factors were considered in this very limited model. SAS Proc PHREG was used for calculation (Allison Citation1999; Cox Citation1972).
Because there was a correlation between the notification and arrival as shown in (correlation coefficient of 0.37), 2 reduced models, based on EquationEq. (4), were run separately to avoid the collinearity issue, with the factors age, call, and location and age and arrival. If age, call, and location factors were used, the results indicated that older people (age > 60) have an approximately 6 percent higher crash fatality risk than people under 60 years old (RR = 1.059, P < .0001). Later crash notification (>1 min) could result in a 2.4 percent higher fatality risk than earlier notification within 1 min (RR = 1.024, P = .0215). People in rural areas may have the same crash fatality likelihood as people in urban areas (RR = 1.003, P = .80). If only age and arrival were used, the results indicated that older people have an approximately 7.6 percent higher crash fatality risk (RR = 1.076, P < .0001), and later EMS arrival (>15 min) could result in a 25 percent higher fatality risk (RR = 1.251, P < .0001). Again, the data were divided into 2 groups, preferred and nonpreferred, based on notification, arrival, or rural/urban, and the calculations were based on the same data set including incapacitating injuries and fatal injuries only.
Further, the Cox model may provide more details on risk factor interactions, notification time shift, and more, but not all possible risk factors were explored in this particularly limited Cox modeling. More advanced research using the Cox model, including FARS 2010–2011 data and perhaps including more levels of injury severities, will be performed in the future.
Discussion and Conclusions
Using the survival analysis technique to study the effects of traffic crash notification and EMS arrival is still relatively new, but the nature of EMS data is closely associated with time, making it more suitable for survival analysis than other statistical approaches. Though FARS 2005–2009 data had limited information about EMS notification and arrival, the data did suggest certain patterns and facts that can be very useful for future studies:
• | From this analysis, earlier EMS or ACN could save the lives of additional drivers and passengers of passenger cars and light trucks—approximately 154 to 290 lives per year—when a time window of 6 h after a crash is considered. | ||||
• | Effective ACN and earlier EMS arrival significantly improved crash survivability, and earlier notification (≤1 min) would generally result in earlier EMS arrival and the correlation between the 2 was studied. Kaplan-Meier curves clearly demonstrate the survival differences between 2 paired groups whose EMS statuses differed—for example, the benefits associated with earlier notifications within 1 min (approximately 1.84% fatality reduction within a time frame of 6 h after a crash)—and the benefits associated with earlier arrival. The fatality reductions or lives potentially saved due to ACN were estimated from a cross-comparison of the survival probabilities of 2 paired life curves at one specific time after a crash. This survival rate comparison is a common approach used in clinical trials and pulic health research. | ||||
• | We did not discover a significant difference in survivability between urban and rural areas overall. However, the study indicates that earlier EMS arrival would save additional lives in rural areas, which is consistent with the overall data pattern, and overall 61 percent of car occupants lived in rural areas if missing data were also included. | ||||
• | The Weibull model with 3 parameters provided a reasonably good fit for fatality data and can be used to predict fatality trends (unlike Kaplan-Meier curves, which include no parameters), and the Cox model is useful for exploring multiple risk factors simultaneously as well as time-dependent variables (e.g., earlier versus late notification). The relatively higher fatality hazard associated with later notification (2.4% higher) was explored in detail. | ||||
• | Compared to previous research, this study's data sample size was larger, with 5 years of data, and the data sample included more current statistics that reflect current phone call and EMS statuses; More important, this research provides details on relative risks, and relative group comparisons were provided with quantitative examples and P values. The traffic fatality reductions due to ACN were estimated from a cross-comparison of the survival probabilities of 2 paired life curves at one specific time after a crash. Furthermore, no prior assumptions were made in this study regarding the possible correlations among EMS notifications, arrivals, and survival time and chance, but the benefits and effects of earlier notification/arrival on survival chances were derived from the original FARS 2005–2009 data, with the aid of an advanced survival analysis technique. Overall, this study assessed the benefits of earlier notification within 1 min and potential lives saved with a larger and more current data and provides a better understanding of the complicated ACN technique from 3 different perspectives of survival probability curves (nonparametric Kaplan-Meier estimator), fatality trend predictions (parametric Weibull model), and multiple risk analysis (Cox model), inspired by extensive earlier research on dynamic survival analyses and ACN/AACN fields. |
Acknowledgments
This article not subject to US copyright law
References
- Abernethy , R . 1999 . The New Weibull Handbook. 3rd ed. , Warrendale, PA: Society of Automotive Engineers .
- Allison , P . 1999 . Survival Analysis Using SAS: A Practical Guide , Cary, NC: SAS Publishing .
- Breslow , N and Day , N . 1987 . Statistical Methods in Cancer Research II: Analysis of Cohort Studies , Lyon , , France : International Agency of Cancer Study .
- Clark , D E and Cushing , B M . 2002 . Predicted effect of automatic crash notification on traffic mortality . Accid Anal Prev. , 34 : 507 – 513 .
- Cox , D R . 1972 . Regression models and life tables—with discussions . J R Stat Soc. , 34 : 187 – 220 .
- Crowley , J and Hu , M . 1977 . Covariance analysis of heart transplant survival data . J Am Stat Assoc. , 78 : 27 – 36 .
- European Commission . 2009 . Impact assessment on the introduction of the eCall service in all new type-approved vehicles in Europe, including liability/legal issues . Available at: http://ec.europa.eu/information_society/activities/esafety/doc/studies/ecall/ecall_final_report.pdf. Accessed April 16, 2013.
- Hosmer , D and Lemeshow , S . 1999 . Applied Survival Analysis—Regression Modeling of Time to Event Data , New York: John Wiley & Sons .
- Kanianthra , J , Carter , A and Preziotti , G . Enhancing post-crash vehicle safety through an automatic collision notification system . Paper presented at: 17th International Technical Conference on the Enhanced Safety of Vehicles; June 4–7, 2001; Amsterdam, The Netherlands ,
- Kaplan , E L and Meier , P . 1958 . Nonparametric estimation from incomplete observations . J Am Stat Assoc. , 261 : 719 – 724 .
- Lahausse , J A , Fildes , B N , Page , Y and Fitzharris , M P . The potential for automatic crash notification systems to reduce road fatalities . Paper presented at: 52nd Annual Conference of the Association for the Advancement of Automotive Medicine; October 6–8, 2008; San Diego, CA ,
- National Highway Traffic Safety Administration . 2011 . Analysis of the Proximity of Fatal Motor Vehicle Crash Locations to the Availability of Helicopter Emergency Medical Service Response NHTSA Research Note DOT HS 811-542, Washington, DC
- Toyota . EMS and Notification Study, Centers for Disease Control and Prevention . Paper presented at: AACN and Field Triage of Injury Patients Update Meeting; November 2, 2011; Atlanta, GA ,