Abstract
Objective: Objective evaluation methods of time history signals are used to quantify how well simulated human body responses match experimental data. As the use of simulations grows in the field of biomechanics, there is a need to establish standard approaches for comparisons. There are 2 aims of this study. The first is to apply 3 objective evaluation methods found in the literature to a set of data from a human body finite element model. The second is to compare the results of each method, examining how they are correlated to each other and the relative strengths and weaknesses of the algorithms.
Methods: In this study, the methods proposed by Sprague and Geers (magnitude and phase error, SGM and SGP), Rhule et al. (cumulative standard deviation, CSD), and Gehre et al. (CORrelation and Analysis, or CORA, size, phase, shape, corridor) were compared. A 40 kph frontal sled test presented by Shaw et al. was simulated using the Global Human Body Models Consortium midsized male full-body finite element model (v. 3.5). Mean and standard deviation experimental data (n = 5) from Shaw et al. were used as the benchmark. Simulated data were output from the model at the appropriate anatomical locations for kinematic comparison. Force data were output at the seat belts, seat pan, knee, and foot restraints.
Results: Objective comparisons from 53 time history data channels were compared to the experimental results. To compare the different methods, all objective comparison metrics were cross-plotted and linear regressions were calculated. The following ratings were found to be statistically significantly correlated (P < .01): SGM and CORrelation and Analysis (CORA) size, R 2 = 0.73; SGP and CORA shape, R 2 = 0.82; and CSD and CORA's corridor factor, R 2 = 0.59. Relative strengths of the correlated ratings were then investigated. For example, though correlated to CORA size, SGM carries a sign to indicate whether the simulated response is greater than or less than the benchmark signal. A further analysis of the advantages and drawbacks of each method is discussed.
Conclusions: The results demonstrate that a single metric is insufficient to provide a complete assessment of how well the simulated results match the experiments. The CORA method provided the most comprehensive evaluation of the signal. Regardless of the method selected, one primary recommendation of this work is that for any comparison, the results should be reported to provide separate assessments of a signal's match to experimental variance, magnitude, phase, and shape. Future work planned includes implementing any forthcoming International Organization for Standardization standards for objective evaluations.
Supplemental materials are available for this article. Go to the publisher's online edition of Traffic Injury Prevention to view the supplemental file.
Introduction
Finite element analysis (FEA) has become an important tool in the field of injury biomechanics. Mathematical models have been used in this field for decades (Yang et al. Citation2006), with finite element models (FEMs) of full human bodies becoming more widespread recently (Robin Citation2001; Ruan et al. Citation2003, Citation2005). Advances in computing power have allowed for more complex models and, as a result, modern full human body FEMs have been created with an increased focus on anatomical accuracy (Shigeta et al. Citation2009; Vavalle et al. Citation2012). Even with accurate anatomical representation, an FEM must be rigorously validated against experimental data before it can be used to study injury. To do this, models are often compared to mean responses and corridors created from postmortem human surrogate (PMHS) testing. Objective comparisons of models are critical when evaluating validation. A comparison made based on mathematics and not human judgment is the preferred method for determining model validity. This approach offers the most robust means of evaluating model performance during development (to quantitatively track improvements) or validation (to quantify the degree to which a model agrees with experimental data).
A new full-body FEM of the mid-sized male (M50) was recently developed by the Global Human Body Models Consortium (GHBMC). The GHBMC model is the result of a collaboration between the automotive industry, government agencies, and academia, with a goal to create a set of advanced full human body FEMs. The purpose of full-body models (FBMs) is to study blunt injury in motor vehicle crashes and to be used as a tool for the community to enhance the safety of vehicles. The development of the M50 model has been discussed in the literature (Gayzik et al. Citation2011; Gayzik, Moreno, Danielson, et al. 2012; Gayzik, Moreno, Vavalle, et al. 2012; Vavalle et al. Citation2012) and will be briefly reviewed here. To obtain accurate anatomical geometry for the model, a multimodality medical imaging approach was taken (Gayzik et al. Citation2009). Magnetic resonance imaging, upright magnetic resonance imaging, computed tomography, and laser surface scanning with bony landmark identification were used to obtain model geometries.
CAD (computer aided design) data were distributed to collaborating universities, whose goal was to create regional FEMs (Beillas and Berthet Citation2012; DeWit and Cronin Citation2012; Fice et al. Citation2011; Li, Kindig, Kerrigan, et al. Citation2010; Li, Kindig, Subit, and Kent Citation2010; Mao et al. Citation2012; Shin et al. Citation2012; Yue et al. Citation2011). The Global Human Body Models Consortium (GHBMC) seated male FBM was constructed by combining 5 regional models (head, neck, thorax, abdomen, and pelvis/lower extremity; Thompson et al. Citation2012). The version of the model used in this study (v. 3.5) contained a total of 1.3 million nodes, 1.95 million elements, and 961 parts and represents a weight of 75.5 kg. The focus of this article will be one validation case in which the experiments of Shaw et al. (Citation2009) were simulated. This particular case provided 53 channels of data for comparing objective evaluation (OE) methods.
OE methods to make comparisons between model response and experimental data are gaining favor. An International Organization for Standardization (ISO) workgroup (ISO/TC 22/SC 10/WG 4) is working to standardize their use for FEM simulations, indicating a broad need for nonsubjective means to calculate the level of correlation observed in the validation of FEMs. The ISO-TR9790 method for evaluating side impact ATDs has been used to evaluate the validation of an FEM in the past (Ruan et al. Citation2006), but this method has been noted to be too subjective (Hsu and Nusholtz Citation2005). The National Highway Traffic Safety Administration also formulated a method for creating cadaveric corridors to which lateral impact anthropomorphic test dummies (ATDs) can be compared (Maltese et al. Citation2002). This method is an improvement over ISO-TR9790 in that it is more automated and less subjective, but there are still shortcomings that have been outlined in the literature (Hsu and Nusholtz Citation2005; Irwin et al. Citation2005). A third method for comparing side impact ATD responses to experimental results was developed by Nusholtz et al. (Citation2007) using cross-correlation. Rather than creating corridors from PMHS tests, this method compares inter-PMHS cross-correlations to cross-correlations between the ATD and each PMHS.
The methods used in the comparison presented in this study were biased toward those that employed averages and standard deviations of PMHS data points. This decision was made because those data are typically presented in the literature and are commonly accessible for computational model comparison. Individual test curves are required for the ISO-TR9790, Maltese, and Nusholtz methods. Individual traces are often not published, creating a higher barrier for groups outside of the lab that conducted the experiment to employ them. Though these previous 3 methods are considered beyond the scope of this study, they are of great interest and reserved for future investigations. The overarching objective of the current work was to investigate several methods for OE. Because there are a number of methods available in the literature, the first aim was to select several commonly used methods and apply them to a robust data set to determine how the methods are correlated, if at all. Methods proposed by Sprague and Geers (Citation2004), Rhule et al. (Citation2002), and Gehre et al. (Citation2009) were implemented. Correlations were used to investigate the relationships between the different metrics and the relative strengths and weaknesses of each method. This work provides an assessment of the method that provided the most complete evaluation of the model with regards to the literature data.
Methods
The GHBMC average male seated model (M50) was used in this study. The subject used as the template for the model was a living male volunteer, 26 years old, 174.9 cm, and 78.6 ± 0.77 kg. The model has been validated in lateral (Vavalle et al. Citation2012) and frontal impacts (Gayzik, Moreno, Vavalle, et al. Citation2012). More details on the model and its development can be found in the GHMBC M50 manual (GHBMC Citation2011).
The model was simulated in a frontal sled test configuration presented by Shaw et al. (Citation2009). The simulation was conducted using MPP LS-Dyna R 4.2.1 running on a Red Hat Linux computational cluster using 48 cores (Livermore Software Technology Corporation [LSTC], Livermore, CA). The seat buck was modeled per the data in the above reference and adjusted from personal communications with the authors of that study (January 31, 2013, Damien Subit). The buck was modeled as a rigid body. Belt properties were made to match descriptions of the webbing of the belt reported by Shaw et al. (Citation2009; 26 kN of force for 7% strain). No pretensioners or load limiters were modeled. Friction was not included between the body and the buck. The model was gravity-settled for 90 ms prior to executing the sled pulse. Belts were fit and final adjustments were made for components of the buck (i.e., knee bolster) following the settling simulation. This particular buck was designed to focus loading on the thorax as the knees are in contact with rigid bolsters at the outset of the simulation. This limited forward excursion of the PMHS in the experiment. The 40 kph change in velocity pulse reported in the study was applied as a velocity history to the buck in the simulation.
All data were recorded in binary output files at a sample rate of 10 kHz. The SAE J1733 coordinate system was used for all kinematic output and seat pan reaction forces. The forces at the upper and lower shoulder belt and outer lap belt corresponded to forces along the belt. Reaction forces at the knee bolsters and foot rest were recorded in the global coordinate system and transformed to local coordinate system as reported in the literature (Ash et al. Citation2012). All kinematic data were reported in the global coordinate system with the exception of chest deflection data, which utilized a local coordinate system defined on T8 (Shaw et al. Citation2009). The time history of the model output is available in the literature (Gayzik, Moreno, Vavalle, et al. Citation2012). Data extracted from the model were compared against an average and standard deviation of n = 5 PMHS tests (Shaw et al. Citation2009). The subjects were all male and had an average mass and age of 75.5 kg and 54 years. Because the average mass of the subjects was equal to that of the model, mass scaling was not explicitly performed.
Table 1 Comparison of objective evaluation methods used in the study
The model and simulated case are critical background for this study. However, the focus was to compare and contrast various OE techniques using this simulation. To that end, it is necessary to briefly describe the 3 methods of quantitative comparison that were used. Equations are readily available in the literature for these methods, so a general overview of each method will be provided instead. A summary of the differences in these methods is found in and Table A1 (see online supplement). The first of these methods, introduced by Sprague and Geers (Citation2004), utilized 2 metrics; magnitude and phase error factors (Vavalle et al. Citation2012). The magnitude factor (SGM) is insensitive to phase shifts and the phase factor (SGP) is insensitive to magnitude. Both are functions of the definite integrals of time-valued functions for a given output. These factors only take into account information from the experimental mean (i.e., no information on the experimental variance is used).
The cumulative standard deviation, or CSD, introduced by Rhule et al. (Citation2002), is the second OE method used. Unlike the Sprague and Geers values, it does take into the account the spread of the experimental data by normalizing the sum of squared difference between a model and an experimental mean by the experimental variance. Because the CSD is the square root of that ratio, it can be interpreted as the number of standard deviations the simulated response is from the mean of the experimental data (Rhule et al. Citation2002).
The final method used in the comparisons of OE methods is a suite of comparison metrics known as CORA (Gehre et al. Citation2009). This is a set of algorithms that takes into account corridor fit, phase shift, and size and shape differences. Among these, only the corridor evaluation takes into account experimental variance. However, the method acknowledges that there are a number of different relevant criteria that are required to make a comprehensive assessment of the signal alignment. CORA v. 3.5 was used in this study. All defaults were used for parameters that control the evaluation except the phase range. The range for interpolation between a perfect (1) and a poor (0) phase match is suggested to be 3 to 12 percent of the time span of the signal. This was changed to 5 to 15 percent for this analysis (i.e., a time shift less than 5% of the signal scored a 1, whereas a time shift greater than 15% scored a 0). For the corridor analysis, the inner corridors were set at one standard deviation, and the outer corridors were set at 2 standard deviations.
The kinematic and kinetic results of the model simulation were run through each of the OE methods identified above (n = 7 metrics). A total of 53 time-valued signals were extracted from the simulation. For each of these signals, corresponding experimental data from the literature (Shaw et al. Citation2009) were available for comparison. The S and G magnitude and phase factors, as well as the CSD factor by Rhule et al. (Citation2002), were programmed in MATLAB v. 11 (The Mathworks, Natick, MA). The values from CORA were obtained from release 3.5 of the CORA software. The flowchart of this comparison study can be found in .
Fig. 1 Study flowchart. Results from 53 channels of data were applied to each of the methods above. Therefore, at the end of this part of the study there was a value of each of the 7 metrics listed above for 53 time-valued signals (color figure available online).
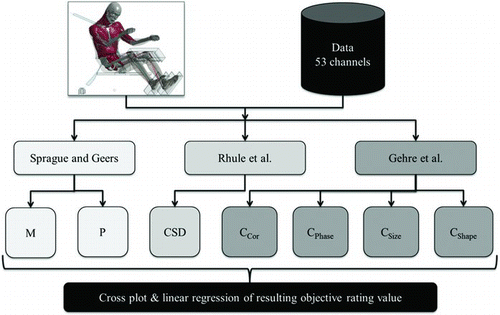
Fig. 2 Time lapse images showing the kinematics of the GHBMC M50 model. The top row indicates overall body motion and the second and third rows show spine kinematics from the side and back, respectively (color figure available online).
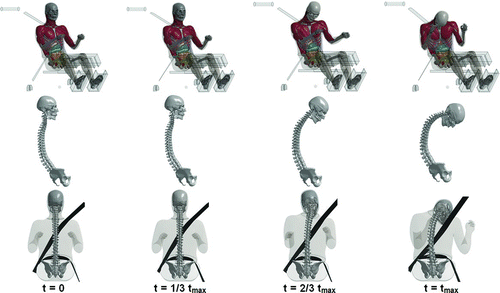
The results of this phase of the study were 7 53 × 1 vectors with the coefficients of the OE methods. Each of these was then cross-plotted to determine whether there was a linear correlation between the results.
Table 2 Cross-plot matrix for comparison study
provides a schematic of the cross-plots that were conducted. No comparisons along the diagonal of the test matrix were conducted because they would yield a perfect correlation, and only the upper off-diagonal terms were considered as the lower terms would be equivalent. Linear regressions were conducted in MATLAB v. 11. The coefficient of determination, R 2, was used to evaluate regressions. The P value for all regressions was also determined from the F-test and a significance value of α = .01 was used to assess significance due to the relatively large sample size.
Results
The frontal sled event was conducted in 2 separate simulations. A 90-ms settling event was simulated and required 16 h to run on 48 cores. The full-body sled test was then simulated using output from the settling run to determine initial nodal locations. The simulation of this 150-ms event required 29 h on 48 cores. A time sequence of the sled event modeled is shown in , with an oblique view of the model, a lateral view of the spine, and a posterior view of the spine. The model showed good robustness with hourglass energy remaining below 12.4 percent of the sum of kinetic and potential energy in the model (including the buck). The peak of this hourglass energy ratio occurred at 127 ms, which closely matched the maximum forward excursion of the model, which occurred at approximately 130 ms.
Following simulation of the event, 53 time history traces were extracted and compared to the experimental data. The results of these comparisons were OE metrics for each of the 3 methods tested. These values are found in the Appendix in Tables A1 and A2 (see online supplement). Cross-plots and linear regressions of the metrics resulted in the correlations summarized in . The 4 coefficients of determination shown with dark gray backgrounds were found to be statistically significant at the selected significance level of α = .01. To summarize, the study's findings indicate that the following metrics were correlated. SGM was correlated to CORA size (R 2 = 0.73), SGP was related to CORA shape (R 2 = 0.82), and CSD was correlated to CORA corridor (R 2 = 0.59). Additionally, statistically significant relationships, though with mild correlations, were found between SGP and CORA phase (R 2 = 0.31) and CORA shape and CORA phase (R 2 = 0.21). Coefficients in bold demonstrated R 2 values greater than 0.5, the linear regressions of which are plotted in .
Table 3 Results from the 21 regressions conducted with the statistically significant correlations highlighted
Discussion
Objective evaluation methods are becoming increasingly important in modeling. A comprehensive set of simulation results including seat belt loads, reaction forces, and kinematic data was used to evaluate 3 common OE techniques. The findings of this study show which of these methods are correlated. They are also of use to researchers familiar with one of these techniques, because these results enable users of one technique to more accurately evaluate results in the literature that employ one of the other techniques tested. There are other intrinsic values to using OE methods not just in validation but in the modeling process. These methods provide the most robust means of tracking model improvement during the model development process. A quantitative means of evaluating model performance also provides a means for comparing the results of 2 different FEA models that have been used to simulate the same event.
Three pairs of metrics were found to have statistically significant correlations and have R 2 values greater than 0.5: SGP and CORA shape, SGM and CORA size, and CSD and CORA corridor. These relationships are consistent with the relationships between the mathematical formulations of the metrics. The correlations between SGP and CORA phase and CORA shape and CORA phase were also found to be statistically significant, but with R 2 = 0.31 and R 2 = 0.21, respectively. It is clear from these coefficients of determination that no 2 metrics were identical. SGM and CORA size, though related, encompassed different ranges. SGM ranged from −1 to +∞, whereas CORA size ranged from 0 to 1. This difference is highlighted by , in which the bar chart shows that many of the SGM values were less than 0, whereas all of the CORA size values were greater than 0. To reiterate from , a negative SGM indicates that the model response is, on average, smaller than the experimental mean. This is an advantage of the SGM, because the user can quickly evaluate whether the model is underpredicting or overpredicting the experiment. Because it does not carry the sign, the CORA size metric does not offer this advantage. An additional difference between these metrics is that a perfect match between model and experiment would receive SGM = 0 and CORA size = 1. Again, this is shown in because many of the smaller SGM values correspond to larger CORA size values.
Fig. 4 Left: Bar chart showing that SGM can be less than zero while CORA size is always positive. Right: (A) a case where SGM is negative because, on average, the model response is smaller than the experimental mean; (B) a case where both SGM and CORA size are positive. Both the SGM and CORA size factor do not consider the phase shift that is apparent in either A or B.
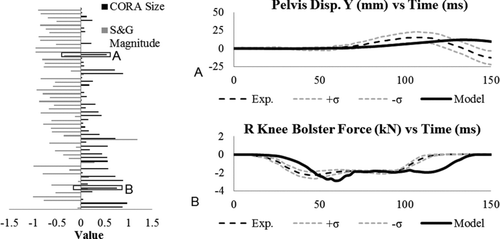
Other interesting differences exist in the correlated pairs of metrics, such as between CSD and CORA corridor. Both values measure how well a model response fits within the corridors of experimental data. However, similar to the case of SGM and CORA size, CSD can range from 0 to +∞, whereas CORA corridor can range from 0 to 1. This is illustrated in , which shows a histogram of the CSD and CORA corridor values obtained. Note that the range of the CSD value was much greater than the CORA value, because it was not normalized. The advantage to this range is that the value of CSD corresponds to the number of standard deviations the model results are (on average) from the mean experimental results and is therefore more easily interpreted by the engineer. Of course, it should be noted that the choice of corridors strongly influences the rating that a model response receives. With respect to CSD, if the standard deviation is narrow (i.e., the data have a low coefficient of variation), a model response that is a number of standard deviations from the mean may not amount to a large relative difference. Conversely, if the standard deviation is large, a better CSD value is to be expected. Though CSD always uses the experimental standard deviation, CORA uses an inner and outer corridor. It is common to use a constant corridor width for both the inner and outer corridors (Gehre et al. Citation2009); however, an alternative to this is to use plus or minus one standard deviation for the inner corridor and plus or minus 2 standard deviations for the outer corridor. The latter approach was used in this study because standard deviation curves were readily available for the experimental data. Of course, the same issues related to the effect of tight or narrow standard deviations also apply to the CORA output.
It was found that Sprague and Geers’ (Citation2004) phase was more highly correlated to CORA shape than to CORA phase. Though both correlations were found to be statistically significant, the correlation to CORA shape was more than twice that of the correlation to CORA phase. The small correlation between CORA shape and CORA phase combined with a strong correlation between SGP and CORA shape was the likely cause of the mild correlation between SGP and CORA phase. This stands to reason considering the method to calculate CORA phase. This metric reflects the amount of time shift needed to align the signal to the experiment. It also caps the value of the time shift by allowing the user to input a minimum and maximum shift. If the calculated shift is less than the minimum, the phase rating is automatically set to 1; if it is larger than the maximum, it is automatically set to 0. This artificial cap likely decreases the correlation with other techniques whose results are continuous functions of the time series data. SGP and CORA shape, on the other hand, both use more complex calculations involving a cross-correlation of the signals. As evidenced from this result, the names of these metrics can lead to incorrect assumptions and the mathematical formulae should be familiar to the user.
Relative strengths and weaknesses of each method were examined to determine the most comprehensive of the 3. The Sprague and Geers (Citation2004) method is able to distinguish between magnitude and phase contributions to the model's error from the experimentally determined responses. Separating these contributions can be helpful in determining how model response can be improved. However, this method does not consider experimental variance, which can play a substantial role in injury biomechanics data due to human variation. A main part of the Rhule et al. (Citation2002) BioRank method is calculating the CSD, which represents how many standard deviations the model is from the experimental data, on average. The variance in the experimental data is clearly taken into account using this calculation. However, if the model response is simply out of phase and would otherwise be a good match, it results in a poor CSD value because only a point-by-point comparison is made and no phase shift is incorporated. The methods of Gehre et al. (Citation2009), known as CORA, provide size, phase, shape, and corridor ratings. This method is the most comprehensive of the 3 examined in this study. Significant correlations were found between each of the metrics in the 2 other studies and the metrics of CORA. Furthermore, no strong correlations were found between the 4 metrics within the CORA approach, indicating that each metric provides independent data regarding the model to experiment comparison. Though the correlation between CORA shape and CORA phase was statistically significant, an R 2 = 0.21 does not indicate a strong correlation.
All 3 of these methods rely on average experimental data and 2 methods require data on the experimental variance. How the average and standard deviations from the experiments are determined is important and has obvious implications on the data reported in this study. Though this is a limitation of the analysis, it is considered beyond the scope of this study because the same data were used for all 3 comparison methods. The topic of averaging biomechanics data is an area of much research as well (Hsu and Nusholtz Citation2005; Irwin et al. Citation2005; Maltese et al. Citation2002; Nusholtz et al. Citation2009). Additionally, though only one study was used to conduct this comparison, it was chosen for a number of reasons. First, a large amount of data was collected (53 data channels used in the comparison); second, the breadth of the results cover kinematics and kinetics of the PMHS; and, finally, the subjects were reasonably close to the anthropometry of a 50th percentile male. A further limitation of the work is that standards from the ISO/TC 22/SC 10/WG 4 were not evaluated in this work. To the authors’ knowledge, these standards are forthcoming and will be evaluated once they become available.
One final limitation is whether the results from Shaw et al.'s (Citation2009) data can be generalized to other data sets. In an attempt to answer this, a brief study of 6 additional validation cases was conducted. These 6 cases (Table A4; see online supplement) resulted in 10 time-valued output traces that could be analyzed in a similar fashion as the primary analysis presented above. Table A5 (see online supplement) summarizes the results from this data set. In this test, CORA shape and SGP as well as CORA size and SGM were again highly correlated and significant. In a trend that mirrors the primary data set, CORA corridor and CSD approached significance in the second data set. These findings do suggest that the approach is generalizable to other data sets.
Unlike Shaw et al.'s (Citation2009) data, a statistical correlation between CORA phase and CSD was found in the second data set. However, the data suggest that this finding may be particular to the reduced data set for a number of reasons. First is the reduced sample size (n = 10 vs. n = 53) and reduced variety (all were force vs. time) in the secondary data set. Second, in the additional data set, there were few samples (3 of 10) in which the CORA phase scores were at the extremes (0 or 1), meaning that most of the phase shifts were between 5 and 15 percent of the total simulation time. The larger data set had a greater share of results at the extremes (24 of 53). These truncated scores generally weaken the correlation between metrics. For instance, when the signal agreement between experiment and model is poor, CSD can be several factors larger than 1, but CORA phase cannot be less than 0. Therefore, the more values at these caps that are included in the metric correlation, the weaker the result. The effects of the CORA normalization are also shown in .
Although one should be mindful of dependence on the data set used, this analysis suggests that the correlations in are reasonably repeatable across data sets because 3 of the same trends emerged in this reduced data set. Furthermore, the findings above indicate that such an analysis should include a variety of both force and displacement data and underscores the importance of standardizing parameters used in the individual correlation analysis, particularly in CORA.
Regardless of which method is chosen, this analysis shows that it is useful to keep metrics separate rather than combining them into a single biofidelity score. Though having one number associated with the validation of a model can be convenient, this can oversimplify the analysis. Each of the types of metrics that are calculated in the methods has different meanings. Much can be learned from investigating each metric separately, and this can guide further model improvements. For example, a reaction force that shows the proper magnitude but a poor phase may indicate that the interaction of the model with the buck is the principal source for the discrepancy between model and experiment. However, combining these scores could result in a loss of that insight and lead to counterproductive efforts to address problems that may not even exist in the model.
Future work in this area may include implementing and applying Nusholtz et al.'s (Citation2007) cross-correlation method to this set of data. An advantage of this method is that it does not use a mean or corridor of the experimental data. Rather, it compares the inter-PMHS cross-correlations to the cross-correlations of the model to the individual tests. Scores are then assigned to magnitude, shape, and phase for each body region. Finally, there is a need to establish standards for regional weighting of OE metrics in FBM analysis so that the most pertinent data are given the greatest consideration.
This study examined 3 methods commonly used to objectively compare model response to experimental data. A simulation of a full-body frontal sled was conducted, from which 53 outputs were compared to experimental data using 3 methods of objective evaluation. It was found that the following 3 methods were both statistically significant and had an R 2 of greater than 0.5: Sprague and Geers’ (Citation2004) phase and CORA shape; Sprague and Geers’ (Citation2004) magnitude and CORA size; and Rhule et al.'s (Citation2002) CSD and CORA corridor. Based on the findings in this study, it is recommended to use an approach similar to CORA for FBM evaluation because it provides the most comprehensive evaluation of the results tested. The 4 metrics provided as part of the CORA analysis package were found not found to be correlated, indicating that each one provides independent data. Advantages for the other methods tested were reviewed as well. This work will additionally serve to clarify how these methods are related and facilitate comparisons between their results.
Supplemental Materials
Download Zip (2 MB)Acknowledgments
Funding support for this work was provided by the Global Human Body Models Consortium. A preliminary version of the test buck used in the simulation was furnished by the National Highway Traffic Safety Administration. Published data in digital format were provided by the Center for Applied Biomechanics at the University of Virginia. All simulations were run on the DEAC cluster at Wake Forest University, with support provided by Drs. David Chin and Timothy Miller.
© Nicholas A. Vavalle, Benjamin C. Jelen, Daniel P. Moreno, Joel D. Stitzel, and F. Scott Gayzik
References
- Ash , J , Shaw , C G , Lessley , D and Crandall , J . PMHS restraint and support surface forces in simulated frontal crashes . Paper presented at: 2012 JSAE Annual Congress (Spring) , Detroit, MI April 24–26
- Beillas , P and Berthet , F . Performance of a 50th percentile abdominal model for impact: effect of size and mass . Paper presented at: European Society of Biomechanics Conference; July 1–4 , Lisbon, Portugal
- DeWit , J A and Cronin , D S . 2012 . Cervical spine segment finite element model for traumatic injury prediction . J Mech Behav Biomed Mater. , 10 : 138 – 150 .
- Fice , J B , Cronin , D S and Panzer , M B . 2011 . Cervical spine model to predict capsular ligament response in rear impact . Ann Biomed Eng. , 39 : 2152 – 2162 .
- Gayzik , F S , Hamilton , C A Tan , J C . 2010 . A Multi-Modality Image Data Collection Protocol for Full Body Finite Element Analysis Model Development Detroit, MI Presented at 2010 SAE World Congress, April 13 SAE Technical Paper 2009-01-2261
- Gayzik , F S , Moreno , D P , Danelson , K A , McNally , C , Klinich , K D and Stitzel , J D . 2012 . External landmark, body surface, and volume data of a mid-sized male in seated and standing postures . Ann Biomed Eng. , 40 : 2019 – 2032 .
- Gayzik , F S , Moreno , D M , Geer , C P , Wuertzer , S D , Martin , R S and Stitzel , J D . 2011 . Development of a full body CAD dataset for computational modeling: a multi-modality approach . Ann Biomed Eng. , 39 : 2568 – 2583 .
- Gayzik , F S , Moreno , D P , Vavalle , N A , Rhyne , A C and Stitzel , J D . Development and validation of a novel full human body FE model utilizing a multi-modality medical imaging protocol . Paper presented at: International Crashworthiness Conference; July 18–20 , Milan, Italy
- Gehre , C , Gades , H and Wernicke , P . Objective rating of signals using test and simulation responses . Paper presented at: 21st ESV Conference; June 15–18 , Stuttgart, Germany
- Global Human Body Models Consortium . 2011 . GHBMC M50 Users Manual Southfield, MI: GHBMC, LLC
- Hsu , T P and Nusholtz , G S . Considerations of Bio-fidelity Corridors for Lateral Impacts Presented at 2005 SAE World Congress, April 11, 2005, Detroit, MI. SAE Technical Paper 2005-01-0308
- Irwin , A L , Sutterfield , A Hsu , T P . 2005 . Side impact response corridors for the rigid flat-wall and offset-wall side impact tests of NHTSA using the ISO method of corridor development . Stapp Car Crash J. , 49 : 423 – 456 .
- Li , Z , Kindig , M W Kerrigan , J R . 2010 . Rib fractures under anterior–posterior dynamic loads: experimental and finite-element study . J Biomech. , 43 : 228 – 234 .
- Li , Z , Kindig , M W , Subit , D and Kent , R W . 2010 . Influence of mesh density, cortical thickness and material properties on human rib fracture prediction . Med Eng Phys. , 32 : 998 – 1008 .
- Maltese , M R , Eppinger , R H , Rhule , H H , Donnelly , B R , Pintar , F A and Yoganandan , N . 2002 . Response corridors of human surrogates in lateral impacts . Stapp Car Crash J. , 46 : 321 – 351 .
- Mao , H , Zhang , L Jiang , B . Recent advances in developing finite element head model . Paper presented at: International Crashworthiness Conference; July 18–20 , Milan, Italy
- Nusholtz , G S , Hsu , T P and Byers , L C . A proposed side impact ATD bio-fidelity evaluation scheme using cross-correlation approach . Paper presented at: 20th ESV Conference; June 18–21 , Lyon, France
- Nusholtz , G S , Hsu , T P , Shi , Y , Kochekseraii , S B and Luna , M AG . Creating representative curves from multiple time histories of vehicle, ATD, and biomechanics tests . Paper presented at: 21st ESV Conference; June 15–18 , Stuttgart, Germany
- Rhule , H H , Maltese , M R , Donnelly , B R , Eppinger , R H , Brunner , J K and Bolte , J H . 2002 . Development of a new biofidelity ranking system for anthropomorphic test devices . Stapp Car Crash J. , 46 : 477 – 512 .
- Robin , S . Human model for safety—a joint effort towards the development of redefined human-like car–occupant models . Paper presented at: 17th International Conference for the Enhanced Safety of Vehicles; June 4–7 , Amsterdam, The Netherlands
- Ruan , J S , El-Jawahri , R , Barbat , S and Prasad , P . 2005 . Biomechanical analysis of human abdominal impact responses and injuries through finite element simulations of a full human body model . Stapp Car Crash J. , 49 : 343 – 366 .
- Ruan , J , El-Jawahri , R , Chai , L , Barbat , S and Prasad , P . 2003 . Prediction and analysis of human thoracic impact responses and injuries in cadaver impacts using a full human body finite element model . Stapp Car Crash J. , 47 : 299 – 321 .
- Ruan , J S , El-Jawahri , R , Rouhana , S W , Barbat , S and Prasad , P . 2006 . Analysis and evaluation of the biofidelity of the human body finite element model according to ISO-TR9790 procedures . Stapp Car Crash J. , 50 : 491 – 507 .
- SAE , J1733 . Sign Convention for Vehicle Crash Testing. December .
- Shaw , G , Parent , D Purtsezov , S . 2009 . Impact response of restrained PMHS in frontal sled tests: skeletal deformation patterns under seat belt loading . Stapp Car Crash J. , 53 : 1 – 48 .
- Shigeta , K , Kitagawa , Y and Yasuki , T . Development of next generation human FE model capable of organ injury prediction . Paper presented at: 21st ESV Conference; June 15–18 , Stuttgart, Germany
- Shin , J , Yue , N and Untaroiu , C D . 2012 . A finite element model of the foot and ankle for automotive impact applications . Ann Biomed Eng. , 40 ( 12 ) : 2519 – 2531 .
- Sprague , M A and Geers , T L . 2004 . A spectral-element method for modelling cavitation in transient fluid–structure interaction . Int J Numer Methods Eng. , 60 : 2467 – 2499 .
- Thompson , A B , Gayzik , F S , Moreno , D P , Rhyne , A C , Vavalle , N A and Stitzel , J D . 2012 . A paradigm for human body finite element model integration from a set of regional models . Biomed Sci Instrum. , 48 : 423 – 430 .
- Vavalle , N A , Moreno , D P , Rhyne , A C , Stitzel , J D and Gayzik , F S . 2012 . Lateral impact validation of a geometrically accurate full body finite element model for blunt injury prediction . Ann Biomed Eng. , 41 ( 3 ) : 497 – 512 .
- Yang , K H , Hu , J , White , N A , King , A I , Chou , C C and Prasad , P . 2006 . Development of numerical models for injury biomechanics research: a review of 50 years of publications in the Stapp Car Crash Conference . Stapp Car Crash J. , 50 : 429 – 490 .
- Yue , N , Shin , J and Untaroiu , C D . Development and Validation of an Occupant Lower Limb Finite Element Model Presented at 2011 SAE World Congress, April 12, 2011, Detroit, MI. SAE Technical Paper 2011-01-1128