ABSTRACT
Objectives: There is no consensus yet on how to determine which patients with cognitive impairment are able to drive a car safely and which are not. Recently, a strategy was composed for the assessment of fitness to drive, consisting of clinical interviews, a neuropsychological assessment, and driving simulator rides, which was compared with the outcome of an expert evaluation of an on-road driving assessment. A selection of tests and parameters of the new approach revealed a predictive accuracy of 97.4% for the prediction of practical fitness to drive on an initial sample of patients with Alzheimer's dementia. The aim of the present study was to explore whether the selected variables would be equally predictive (i.e., valid) for a closely related group of patients; that is, patients with mild cognitive impairment (MCI).
Methods: Eighteen patients with mild cognitive impairment completed the proposed approach to the measurement of fitness to drive, including clinical interviews, a neuropsychological assessment, and driving simulator rides. The criterion fitness to drive was again assessed by means of an on-road driving evaluation. The predictive validity of the fitness to drive assessment strategy was evaluated by receiver operating characteristic (ROC) analyses.
Results: Twelve patients with MCI (66.7%) passed and 6 patients (33.3%) failed the on-road driving assessment. The previously proposed approach to the measurement of fitness to drive achieved an overall predictive accuracy of 94.4% in these patients. The application of an optimal cutoff resulted in a diagnostic accuracy of 100% sensitivity toward unfit to drive and 83.3% specificity toward fit to drive. Further analyses revealed that the neuropsychological assessment and the driving simulator rides produced rather stable prediction rates, whereas clinical interviews were not significantly predictive for practical fitness to drive in the MCI patient sample.
Conclusions: The selected measures of the previously proposed approach revealed adequate accuracy in identifying fitness to drive in patients with MCI. Furthermore, a combination of neuropsychological test performance and simulated driving behavior proved to be the most valid predictor of practical fitness to drive.
Introduction
Cognitive impairment is a risk factor for unsafe driving (Devlin et al. Citation2012; Dubinsky et al. Citation2000; Frittelli et al. Citation2009; Wadley et al. Citation2009), but advising patients with cognitive impairment on fitness to drive is difficult due to the many factors that influence driving safely (Bacon et al. Citation2007). Several clinical assessment tools have been composed to evaluate fitness to drive in patients with cognitive impairment, but there is no consensus yet on how fitness to drive should be investigated in clinical practice (Carr and Ott Citation2010). It has been shown that predictive accuracies of the available methods for the assessment of fitness to drive differ greatly when compared to pass–fail decisions of on-road evaluators and often fail a successful replication on independent samples (Gifford Citation2013; Hoggarth et al. Citation2013; Innes et al. Citation2011; Mathias and Lucas Citation2009; Vrkljan et al. Citation2011). Several possible explanations for this variation in predictive accuracy of such approaches can be identified. First, a great heterogeneity of patients exists both within and between studies that becomes particularly obvious with respect to clinical characteristics such as etiologies, symptoms, and impairments. It can be argued that patients suffering from different types of impairments may require a different set of measures for the prediction of fitness to drive (Piersma, de Waard et al. Citation2016). Second, many studies that explored predictors of fitness to drive employed comprehensive assessment tools and test batteries, including numerous tests and test variables. For these reasons, many studies introduced a large number of candidate variables and explored the validity of these measures for the prediction of fitness to drive on a rather small, heterogeneous clinical sample. The identification of significant predictors among a large number of variables on small samples may result in a problem that is referred to as capitalization on chance (MacCallum et al. Citation1992), which describes the risk of a large part of the associations found between predictor variables and the outcome fitness to drive having occurred due to random error. Capitalization on chance is a relevant issue for the identification of predictors for fitness to drive, because prediction models tend to perform better on the data set on which the model was estimated (derivation set) than on a new data set (validation set). External validation of a prediction model on an independent data set is therefore a crucial step before clinical application can be suggested (Bleeker et al. Citation2003; Toll et al. Citation2008).
In a recent study, an assessment strategy was composed for the prediction of practical fitness to drive in patients with Alzheimer's dementia (AD), consisting of clinical interviews, a neuropsychological assessment, and driving simulator rides (Piersma, Fuermaier et al. Citation2016). The predictive accuracy of this approach was found to be 97.4% on a sample of 55 patients with AD. Before application in clinical practice can be recommended, the validity of such an assessment strategy needs to be evaluated on an independent sample of patients. The aim of this study was therefore to explore the validity of the assessment approach and to extent this approach to a closely related group of patients with cognitive impairment; that is, mild cognitive impairment (MCI).
Methods
Patients with mild cognitive impairment
Recruitment and assessment of patients with MCI was performed as part of the original study and followed the same study protocol as described by Piersma, Fuermaier and colleagues (Citation2016). MCI was diagnosed by a neurologist, geriatrician, psychiatrist, or general practitioner. The diagnosis was established by following the criteria as described by Petersen (Citation2004) and Albert et al. (Citation2011), which includes (1) cognitive complaints of the patient indicating cognitive decline, usually corroborated by an informant; (2) objective evidence of cognitive impairment that cannot be explained by normal aging; (3) essentially preserved functional abilities; and (4) the absence of a diagnosis of dementia. There was no predefined set of measures that was consistently used in the diagnostic process of all patients in the present study. However, the diagnostic process of all patients was supported by the use of various diagnostic instruments that were mainly used in a qualitative fashion for diagnostic purposes. Data on formal neuropsychological testing were not available for all patients; therefore, a distinction between amnestic and nonamnestic MCI, as well as single or multiple domain impairment, cannot be made for all patients in the present sample. Recruitment and assessment were conducted at 5 locations in The Netherlands, resulting in the inclusion of 18 patients diagnosed with MCI who performed the complete fitness to drive assessment method as described in the previous study on patients with AD. Participants’ ages ranged from 49 to 79 years (mean = 67.5 years; SD = 8.6 years), including 2 females and 16 males. Inclusion criteria were a valid driver's licence, a diagnosis of MCI, a binocular visual acuity of at least 0.5, and a horizontal visual field of at least 120°. Exclusion criteria were neurological conditions unrelated to any etiology of dementia and usage of medications with known severe influence on driving. The study was approved by the Medical Ethical Committee of the University Medical Center Groningen, The Netherlands.
Fitness to drive assessment
The approach to the assessment of fitness to drive recently described (Piersma, Fuermaier et al. Citation2016), including clinical interviews, a neuropsychological assessment, and driving simulator rides, was administered to all patients. For the purpose of this study, only those measures entered analysis that included the final prediction equations as derived from the original study on the assessment of fitness to drive. Measures of clinical interviews included 2 subscores of the Clinical Dementia Rating (CDR; Morris Citation1993)—that is, Orientation and Judgment and Problem Solving—as well as additional information about the patients’ judgments about their own driving safety and recent driving experience. The neuropsychological assessment included the Mini-Mental State Examination (MMSE; Folstein et al. Citation1975; Kok and Verhey Citation2002), the Reaction Time S2 (Prieler Citation2008; Schuhfried Citation2013), the Hazard Perception Test (Vlakveld Citation2011), and a traffic theory test (for details, see Piersma, Fuermaier et al. Citation2016). For the driving simulation, fixed-based Jentig50 driving simulators of ST Software (Groningen, the Netherlands) were used. Driving simulator measures included the minimal speed when approaching an intersection with traffic lights, the number of collisions in a ride with intersections, and 2 measures regarding a merging maneuver; that is, the deceleration of the car behind right after merging and the time headway to the car in front directly after merging (for details of simulator rides, see Piersma, Fuermaier et al. Citation2016).
On-road driving assessment
The on-road driving assessments were performed by approved experts on practical fitness to drive of the CBR Dutch driving test organization. Experts were blind to the patients’ diagnoses and fitness to drive assessment results. The experts made use of the Test Ride Investigating Practical fitness to drive (Tant et al. Citation2002; Withaar Citation2000). Finally, one overall score was determined by the expert, resulting in a pass, doubtful, or fail outcome. The overall score was recoded into a dichotomous item; that is, pass outcomes indicating fitness to drive and doubtful or fail outcomes indicating unfit to drive (criterion).
Statistical analysis
Receiver operating characteristic (ROC) analyses were performed to evaluate the predictive accuracies of the obtained measures in determining individuals’ practical fitness to drive. ROC analyses were performed on the basis of clinical interviews, neuropsychological test performances, driving simulator rides, as well as the complete assessment approach including all 3 types of predictors. The area under the curve (AUC) was used as a classification measure with larger areas indicating better predictive accuracy. The predictive accuracy of the complete approach was further evaluated by calculating classification statistics; that is, sensitivity, specificity, positive predictive power, and negative predictive power.
Results
Of the 18 patients with MCI, 12 patients (66.7%) passed the on-road assessment and were regarded fit to drive, and 6 patients (33.3%) with doubtful or fail outcomes were regarded unfit to drive. Of the 12 patients who were regarded fit to drive, 5 patients had no restrictions placed on them, 2 patients were restricted to driving with automatic transmission, and 5 patients had a restriction on the duration that the driver's license was valid, ranging between 1 and 5 years (depending on the severity of impairments found in neuropsychological tests). Descriptive results of patients with MCI who passed and failed the on-road assessment are presented in .
Table 1. Descriptive results of patients with MCI who pass and patients with MCI who fail the on-road assessment on selected measures of clinical interviews, neuropsychological assessment, and driving simulator rides (M ± SD).
Prediction equations of fitness to drive as described in the previous study on patients with AD were calculated for all types of assessments applied (Piersma, Fuermaier et al. Citation2016). The coefficients that determine the prediction equations are presented in the footnote in . Based on these prediction equations, ROC analysis revealed that the clinical interview was not useful for the prediction of practical fitness to drive in the present patient sample, because it was shown by a nonsignificant AUC value close to chance level (AUC = 0.528, SE = 0.151, P =.851). In contrast, ROC analyses demonstrated good predictive accuracies of results from the neuropsychological assessment (AUC = 0.819, SE = 0.102, P =.031) and driving simulator rides (AUC = 0.861, SE = 0.089, P =.015), with significant predictive accuracies of greater than 80%. ROC curves depicting graphical plots of sensitivity versus 1 − specificity of results derived from all 3 types of assessments are presented in .
Figure 1. ROC curves presenting diagnostic accuracies of clinical interviews, neuropsychological assessment, and driving simulator rides for the prediction of fitness to drive.
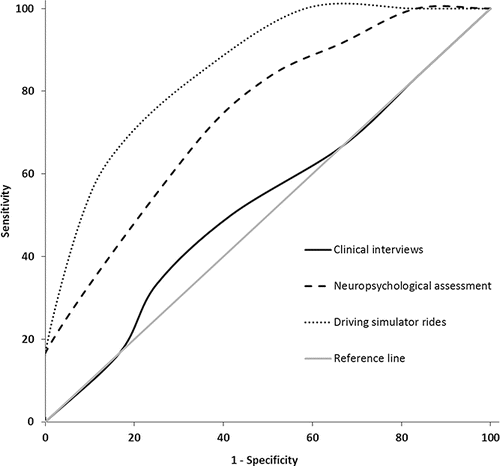
The complete approach combining all 3 types of information (interviews, neuropsychological assessments, and driving simulator rides) achieved a high predictive accuracy of detecting patients who were unfit to drive (AUC = 0.944, SE = 0.052, P =.003), which was close to the predictive accuracy of the approach as derived from the data set of patients with AD (97.4%; Piersma, Fuermaier et al. Citation2016). Applying previously proposed cutoffs of the final predictor variable (−0.6 and −0.8) on the present sample of patients with MCI, 14 out of 18 patients were classified correctly, with 2 patients incorrectly classified as failing (false negatives) and 2 patients incorrectly classified as passing (false positives). When adapting the cutoff to −1.2, 16 out of 18 patients were classified correctly, with 2 false negatives but no false positives ().
Table 2. Classification accuracy of the final predictor variable (including clinical interviews, neuropsychological assessment, and driving simulator rides) in detecting patients being unfit to drive (n = 6) relative to patients being fit to drive (n = 12).
Discussion
Of the 18 patients with MCI who participated in this study, 12 patients passed and 6 patients failed the on-road assessment. This rate of one third of the patients failing the on-road assessment supports the notion that MCI can be a threat to safe driving (Devlin et al. Citation2012; Frittelli et al. Citation2009; Wadley et al. Citation2009). At the same time, results show that for two third of the patients there is no need to stop driving, and they can retain mobility.
Due to the risk of overestimating predictive validity when interpreting classification statistics on the derivation set (also referred to as capitalization on chance; Bleeker et al. Citation2003; MacCallum et al. Citation1992; Toll et al. Citation2008), the purpose of the present study was to evaluate predictive accuracy of the recently proposed approach to the assessment of practical fitness to drive (Piersma, Fuermaier et al. Citation2016) on a closely related group of patients with cognitive impairment; that is, MCI. High overall predictive accuracy of the proposed approach combining clinical interviews, a neuropsychological assessment, and driving simulator rides was achieved on the present sample of patients with MCI (AUC = 94.4%), which is almost similar to what has been found in the original study on patients with AD (AUC = 97.4%). When applying cutoff values of the final predictor variable as proposed in the study on patients with AD, only mediocre classification accuracies for identifying practical fitness to drive in patients with MCI were reached; that is, 67.7% sensitivity and 83.3% specificity. More adequate diagnostic accuracies were achieved when applying a stricter cutoff (−1.2), yielding 100% sensitivity and 83.3% specificity.
In correspondence with findings in patients with AD (Piersma, Fuermaier et al. Citation2016), results of the neuropsychological assessment and driving simulator rides were also found to be valid predictors for practical fitness to drive in patients with MCI. However, contrary to the findings in patients with AD, results of the clinical interview were not found to significantly predict practical fitness to drive in patients with MCI. This lack of predictive validity on the validation set in contrast to predictive validity that has been found on the derivation set might be explained by capitalization on chance (MacCallum et al. Citation1992), indicating that a considerable proportion of shared variance between predictors and the criterion practical fitness to drive in the derivation set may have occurred by random error. Another explanation might be that the patients with MCI might not be so much aware of their actual deficits because their deficits are mild in nature, potentially leading them to under- or overestimate their deficits. Thus, one may conclude that clinical interviews do not represent valid measures for the prediction of fitness to drive in patients with MCI. However, as an alternative explanation, one may argue that the selected measures of clinical interviews in fact present valid predictors for fitness to drive in AD but not in other types of dementia or milder cognitive impairments. Patients with MCI may or may not develop dementia and, if so, they may develop AD or another type of dementia. This usually remains uncertain until a later stage of their disease (Jungwirth et al. Citation2012). Patients with MCI therefore represent a more heterogeneous group than patients with AD. For example, problems with orientation (as reflected by CDR Orientation) are very common in AD (Tu et al. Citation2015) and may therefore be predictive for fitness to drive in samples with AD, whereas these problems may be rare in patients with MCI, resulting in a lack of predictive accuracy in patients with MCI.
As a limitation of this study, it must be noted that some but not all patients with MCI in the present sample may have a subclinical AD-like pathology. Thus, the majority of patients used for the validation of the prediction strategy (patients with MCI) might be characterized by a different etiology than the sample of patients used for the derivation (patients with AD). Before clinical application can be recommended, further validation on another sample of patients with AD is advisable to determine the predictive accuracy of the different types of assessments, in particular measures of clinical interviews that have not been shown to be useful in the present study. The methodology applied in the current study should also be employed in studies on fitness to drive in patients with other types of dementia than AD, because other predictor variables may play a role in the prediction of fitness to drive in patients with other types of dementia (Piersma, de Waard et al. Citation2016).
It is important to note that validation of the prediction strategy and the extension to patients with MCI as described in the present study is based on a relatively small sample of 18 patients with MCI. A comparison of the different types of measures between patients passing and failing the on-road test as presented in must therefore be interpreted with caution. Thus, further validation studies on patients with AD and other types of dementia should preferably be based on larger samples, in order to derive more valid conclusions on the utility of the proposed approach to the practical assessment of fitness to drive. Further studies on patients with MCI should also consider distinguishing between different subtypes of MCI, including amnestic and nonamnestic MCI, as well as single or multiple domain impairment, in order to explore their differential effects on fitness to drive.
In conclusion, the proposed approach to the assessment of fitness to drive revealed adequate accuracy in predicting practical fitness to drive of a group of patients closely related to the original group of patients with AD; that is, patients with MCI. Though a combination of a neuropsychological assessment and driving simulator rides may yield most valid prediction of fitness to drive in patients with cognitive impairment, it cannot be determined on the basis of the present data whether the selected measures of clinical interviews are valid predictors for fitness to drive.
Funding
This work was funded by the Ministry of Infrastructure and the Environment (NL). The funders had no role in study design, data collection and analysis, decision to publish, or preparation of the article.
References
- Albert MS, DeKosky ST, Dickson D, et al. The diagnosis of mild cognitive impairment due to Alzheimer's disease: recommendations from the National Institute on Aging–Alzheimer's Association workgroups on diagnostic guidelines for Alzheimer's disease. Alzheimers Dement. 2011;7:270–279.
- Bacon D, Fisher S, Morris JC, Rizzo M, Spanaki MV. American Academy of Neurology position statement on physician reporting of medical conditions that may affect driving competence. Neurology. 2007;68:1174–1177.
- Bleeker SE, Moll HA, Steyerberg EW, et al. External validation is necessary in, prediction research: a clinical example. J Clin Epidemiol. 2003;56:826–832.
- Carr DB, Ott BR. The older adult driver with cognitive impairment: “it's a very frustrating life.” J Am Med Assoc. 2010;303:1632–1641.
- Devlin A, McGillivray J, Charlton J, Lowndes G, Etienne V. Investigating driving behaviour of older drivers with mild cognitive impairment using a portable driving simulator. Accid Anal Prev. 2012;49:300–307.
- Dubinsky R, Stein A, Lyons K. Practice parameter: risk of driving and Alzheimer's disease (an evidence-based review)—report of the Quality Standards Subcommittee of the American Academy of Neurology. Neurology. 2000;54:2205–2211.
- Folstein MF, Folstein SE, McHugh PR. A practical method for grading the cognitive state of patients for the clinician. Psychiatry Res. 1975;12:189–198.
- Frittelli C, Borghetti D, Iudice G, et al. Effects of Alzheimer's disease and mild cognitive impairment on driving ability: a controlled clinical study by simulated driving test. Int J Geriatr Psychiatry. 2009;24:232–238.
- Gifford R. Flawed conclusion. Can Fam Physician. 2013;59:726–727.
- Hoggarth PA, Innes CRH, Dalrymple-Alford JC, Jones RD. Predicting on-road assessment pass and fail outcomes in older drivers with cognitive impairment using a battery of computerized sensory-motor and cognitive tests. J Am Geriatr Soc. 2013;61:2192–2198.
- Innes CRH, Lee D, Chen C, Ponder-Sutton AM, Melzer TR, Jones RD. Do complex models increase prediction of complex behaviours? Predicting driving ability in people with brain disorders. Q J Exp Psychol. 2011;64:1714–1725.
- Jungwirth S, Zehetmayer S, Hinterberger M, Tragl KH, Fischer P. The validity of amnestic MCI and non-amnestic MCI at age 75 in the prediction of Alzheimer's dementia and vascular dementia. Int Psychogeriatr. 2012;24:959–966.
- Kok R, Verhey F. Dutch Translation of the Mini Mental State Examination (Folstein et al., 1975). Unpublished manuscript. 2002.
- MacCallum RC, Roznowski M, Necowitz LB. Model modifications in covariance structure analysis—the problem of capitalization on chance. Psychol Bull. 1992;111:490–504.
- Mathias JL, Lucas LK. Cognitive predictors of unsafe driving in older drivers: a meta-analysis. Int Psychogeriatr. 2009;21:637–653.
- Morris J. The Clinical Dementia Rating (CDR)—current version and scoring rules. Neurology. 1993;43:2412–2414.
- Petersen RC. Mild cognitive impairment as a diagnostic entity. J Intern Med. 2004;256:183–194.
- Piersma D, de Waard D, Davidse R, Tucha O, Brouwer WH. Car drivers with dementia: different complications due to different etiologies? Traffic Inj Prev. 2016;17:9–23.
- Piersma D, Fuermaier ABM, de Waard D, et al. Prediction of fitness to drive in patients with Alzheimer's dementia. PLoS One. 2016;11:e0149566.
- Prieler J. RT: Reaction Test (Manual)—Vienna Test System (VTS). Vienna, Austria: Schuhfried; 2008.
- Schuhfried G. Vienna Test System (VTS) 8. Ver 8.2.00 [computer software]. Vienna, Austria: Schuhfried; 2013.
- Tant M, Brouwer WH, Cornelissen F, Kooijman A. Driving and visuospatial performance in people with hemianopia. Neuropsychol Rehabil. 2002;12:419–437.
- Toll DB, Janssen KJM, Vergouwe Y, Moons KGM. Validation, updating and impact of clinical prediction rules: a review. J Clin Epidemiol. 2008;61:1085–1094.
- Tu S, Wong S, Hodges JR, Irish M, Piguet O, Hornberger M. Lost in spatial translation—a novel tool to objectively assess spatial disorientation in Alzheimer's disease and frontotemporal dementia. Cortex. 2015;67:83–94.
- Vlakveld W. Hazard Anticipation of Young Novice Drivers; Assessing and Enhancing the Capabilities of Young Novice Drivers to Anticipate Latent Hazards in Road and Traffic Situations [dissertation]. Groningen, The Netherlands: University of Groningen; 2011.
- Vrkljan BH, McGrath CE, Letts LJ. Assessment tools for evaluating fitness to drive: a critical appraisal of evidence. Can J Occup Ther. 2011;78:80–96.
- Wadley VG, Okonkwo O, Crowe M, et al. Mild cognitive impairment and everyday function: an investigation of driving performance. J Geriatr Psychiatry Neurol. 2009;22:87–94.
- Withaar F. Divided Attention and Driving. The Effects of Aging and Brain Injury [dissertation]. Groningen, The Netherlands: University of Groningen; 2000.