ABSTRACT
Objective: Accounting for one fifth of all crashes and one sixth of all fatal crashes in the United States, intersection crashes are among the most frequent and fatal crash modes. Intersection advanced driver assistance systems (I-ADAS) are emerging vehicle-based active safety systems that aim to help drivers safely navigate intersections. The objective of this study was to estimate the number of crashes and number of vehicles with a seriously injured driver (Maximum Abbreviated Injury Scale [MAIS] 3+) that could be prevented or reduced if, for every straight crossing path (SCP) intersection crash, one of the vehicles had been equipped with an I-ADAS.
Methods: This study retrospectively simulated 448 U.S. SCP crashes as if one of the vehicles had been equipped with I-ADAS. Crashes were reconstructed to determine the path and speeds traveled by the vehicles. Cases were then simulated with I-ADAS. A total of 30 variations of I-ADAS were considered in this study. These variations consisted of 5 separate activation timing thresholds, 3 separate computational latency times, and 2 different I-ADAS response modalities (i.e., a warning or autonomous braking). The likelihood of a serious driver injury was computed for every vehicle in every crash using impact delta-V. The results were then compiled across all crashes in order to estimate system effectiveness.
Results: The model predicted that an I-ADAS that delivers an alert to the driver has the potential to prevent 0–23% of SCP crashes and 0–25% of vehicles with a seriously injured driver. Conversely, an I-ADAS that autonomously brakes was found to have the potential to prevent 25–59% of crashes and 38–79% of vehicles with a seriously injured driver. I-ADAS effectiveness is a strong function of design. Increasing computational latency time from 0 to 0.5 s was found to reduce crash and injury prevention estimates by approximately one third. For an I-ADAS that delivers an alert, crash/injury prevention effectiveness was found to be very sensitive to changes in activation timing (warning delivered 1.0 to 3.0 s prior to impact). If autonomous braking was used, system effectiveness was found to largely plateau for activation timings greater than 1.5 s prior to impact. In general, the results of this study suggest that I-ADAS will be 2–3 times more effective if an autonomous braking system is utilized over a warning-based system.
Conclusions: This study highlights the potential effectiveness of I-ADAS in the U.S. vehicle fleet, while also indicating the sensitivity of system effectiveness to design specifications. The results of this study should be considered by designers of I-ADAS and evaluators of this technology considering a future I-ADAS safety test.
Introduction
In the United States, intersection crashes are among the most frequent crash modes and are often fatal. These crashes account for approximately one fifth of all police-reported crashes and one sixth of all fatal crashes each year (Kusano and Gabler Citation2013). In 2015 alone, there were 1.26 million police-reported intersection crashes and 5,251 fatal intersection crashes (NHTSA Citation2016a, Citation2016c).
This study focuses on the straight crossing path (SCP) scenario, which is the most frequent and most frequently fatal intersection crash mode in the United States. The SCP mode occurs when 2 nonturning vehicles collide. This scenario accounts for about one third of police-reported and one half of fatal intersection crashes (Kusano and Gabler Citation2015b). Nearly all SCP intersection crashes occur at signal-controlled (52%) or stop sign–controlled (46%) intersections. About one half of these crashes involve a vehicle that slowed or stopped prior to entering the intersection (Scanlon, Kusano, Sherony, and Gabler Citation2015). There are, of course, other intersection crash modes that should be of high priority for crash avoidance technology designers but that were not the focus of this study. With each different crash mode come differences in driver behavior, which will inherently change the design needs of crash avoidance technologies. Other crash modes include left turn across the path/opposite direction crash mode, which is the second most frequent (26% of police-reported intersection crashes; NHTSA Citation2016c) and lethal (32% of fatal intersection crashes; NHTSA Citation2016a) intersection crash mode in the United States. In this scenario, a left-turning driver attempted to turn left in front of a vehicle traveling from the opposite direction. A second example is the left turn across path/lateral direction scenario, where a vehicle attempts to turn left across the path of a straight-crossing vehicle approaching from the left. This is the third most frequent and lethal scenario in the United States, accounting for 16% of fatal and 17% of police-reported intersection crashes (NHTSA Citation2016a, Citation2016c).
Preventing and/or mitigating crashes and injuries that occur in intersection crashes are among the highest priority for designers, evaluators, and regulatory agencies (Mercedes-Benz USA Citation2015; NHTSA Citation2015; Volvo Car USA Citation2014). Crash avoidance systems aimed at preventing intersection crashes are currently being developed and deployed throughout the vehicle fleet. One promising method is to use an intersection advanced driver assistance system, or I-ADAS. This vehicle-based technology might use sensors, such as radar or lidar, to detect oncoming vehicles. The onboard system must then evaluate (a) whether there is a potential collision partner and (b) whether it is necessary to take crash avoidance action. If the system does detect an imminent threat, I-ADAS can respond by either (a) warning the driver and/or (b) autonomously preventing the crash (e.g., using automated emergency braking, or AEB). This system is likely to be influenced by the available time from earliest vehicle detection until impact. Accordingly, the effectiveness of this technology is expected to be a strong function of computational latency time (Kaempchen and Dietmayer Citation2003), sightline restrictions (Scanlon, Page, et al. Citation2016), driver perception reaction time (H. Chen et al. Citation2011), sensor specifications (Scanlon, Page, et al. Citation2016), and triggering algorithms (Kusano and Gabler Citation2015a).
There are several additional methods being explored for preventing intersection crashes. These other technologies can be divided into two basic groups: (1) vehicle-to-infrastructure (V2I) and (2) vehicle-to-vehicle (V2V). V2I uses infrastructure-based communication systems to relay information, such as upcoming traffic control devices, the location of other roadway users, and changes in speed limit, to surrounding vehicles (Samad and Annaswamy Citation2011; IEEE Connected Vehicles Citation2015; Papadimitratos et al. Citation2009). V2V is similar to V2I but relies on information relayed between vehicles (Samad and Annaswamy Citation2011). Both technologies have the potential to use data collected by either (a) vehicle-based sensors (Fuerstenberg et al. Citation2002; Li et al. Citation2009) or (b) roadside sensors (Cho and Rice Citation2006; Papadimitratos et al. Citation2009). Like I-ADAS, these technologies will require vehicle path prediction and crash threat detection algorithms and will need to make an appropriate intervention decision. One major advantage of V2V and V2I for intersection crashes is that by communicating the position of other neighboring vehicles, sight obstruction limitations can be overcome. This limitation, as demonstrated in the current study, is expected to play a major restrictive role in I-ADAS effectiveness. Although V2I and V2V are promising crash avoidance solutions, fleet-wide implementation will be gradual. Specifically, these technologies (a) are still being developed and tested, (b) may be prohibitively expensive to implement in some locations (e.g., rural, less frequently traversed roads; Cho and Rice Citation2006), and (c) will take time to be deployed throughout the vehicle fleet. In fact, in December 2015, the NHTSA set forth a proposed framework of testing procedures for a future intersection collision avoidance system that uses V2V communications (NHTSA Citation2015). Accordingly, I-ADAS serves as a promising, near-term alternative that is already present within the vehicle fleet (Audi Citation2015; Mercedes-Benz USA Citation2015; Volvo Car USA Citation2014).
There is a need to forecast the ability of I-ADAS technology to prevent and mitigate real-world intersection crashes. Using a benefits study, designers, evaluators, and regulators of this technology can quantify how effective the technology may be and identify design requirements and limitations. In a preliminary study, we examined the proportion of SCP crashes that could be prevented if I-ADAS were on both vehicles and delivered a warning to the driver (Scanlon, Sherony, and Gabler. Citation2016d). This study revealed that up to one third of drivers would have been able to respond to an I-ADAS warning but would not have been unable to successfully avoid the crash. During this scenario, crash conditions, such as impact speeds and impact location configurations, would have been modified. We hypothesized that, even in cases where the crash would not have been fully avoided, there was the potential for occupant injury mitigation.
The current study tested this hypothesis by retrospectively simulating SCP intersection crashes as if one of the vehicles had been equipped with an I-ADAS. The objective of this study was to estimate the proportion of vehicles with seriously injured drivers that could be reduced if, for every SCP intersection crash in the United States, one of the vehicles had been equipped with I-ADAS. Additionally, we analyzed the influence of I-ADAS design on system effectiveness. This included (1) examining both a system that either delivers a warning or autonomously brakes, (2) investigating the influence of computational latency time, and (3) investigating the influence of activation timing thresholds.
Methods
Overall strategy
There were 4 main steps in this study. First, a set of crashes were extracted from a nationally representative U.S. database. Second, each crash was reconstructed from the intersection approach through impact using information collected by the crash investigator. Third, each scenario was simulated as if one of the involved vehicles had been equipped with an I-ADAS. Fourth, the crash outcomes with and without I-ADAS were used to estimate the proportion of seriously injured drivers that could have potentially been prevented.
Simulation case set
Data source
This study used a database of crashes investigated as a part of the National Motor Vehicle Crash Causation Survey (NMVCCS; Bellis and Page Citation2008). The NMVCCS study was specifically designed in response to the emergence of crash avoidance systems in the United States (NHTSA Citation2008). This special study was conducted by the NHTSA from 2005 to 2007 over a 2.5-year period and contains in-depth crash records. Crash investigators must have been on scene prior to the crash being cleared by emergency responders in order to be included in the database. This allowed for a more extensive collection of information from the crash scene, such as by conducting interviews and taking detailed measurements of the crash scene. NMVCCS assigns each crash a national weighting factor, which allows for nationally representative estimates to be made.
Crash inclusion criteria
There were several criteria for a crash to be included in this study. First, the crash must have occurred at an intersection, defined here as the connection of 2 roadways, between 2 passenger vehicles. Crashes involving parking lots, driveways, alleys, or interchanges were excluded from further analysis. The NMVCCS “RELJNCT” variable was used to identify intersection and intersection-related crashes. Each crash was then manually reviewed to confirm its inclusion. Second, the crash must have been between 2 nonturning drivers. SCP crashes were identified using the “ACCTYPE” variable and confirmed through manual review. Third, crashes were excluded if the case was missing crucial data elements. This included cases (a) with at least one of the vehicles having an unknown precrash movement, (b) with missing photographs of the crash scene, (c) where it is unknown which vehicle had the right of way, (d) with an unknown speed limit, (e) with an unknown traffic control device, or (f) with missing driver age or gender. Fourth, several criteria were required for performing impact simulations. Crashes were excluded if (a) a rollover occurred, (b) more than 2 moving vehicles were involved, (c) a heavy truck was involved, or (d) the scene diagram was either scaled incorrectly or was missing vehicle rest positions.
Crash scenario replications
To account for the inherent uncertainty in driver behavior, each crash was simulated under several conditions. First, there was uncertainty in whether a driver took evasive action prior to impact. As described in detail in the Crash Reconstruction section, if a vehicle did not perform a complete or rolling stop prior to entering the intersection, the crash was simulated with and without evasive braking action, and each scenario was weighted based on how likely it was to have occurred. Second, an effective I-ADAS seeks to avoid a crash in a scenario where only one of the vehicles was equipped. Accordingly, every crash was simulated twice: once with the right-of-way vehicle being equipped and again with the violating vehicle being equipped. Each scenario was equally weighted. Third, there is also considerable variability in how drivers respond to an I-ADAS warning (H. Chen et al. Citation2011). Three equally probable reaction times were considered for how drivers would respond to I-ADAS.
As an example of how cases were replicated, consider a crash where neither of the vehicles stopped prior to entering the intersection. If this crash was simulated as if one of the vehicles had been equipped with I-ADAS, 24 scenarios would have been modeled (4 evasive braking scenarios [both vehicles with and without braking] × 2 possibly equipped vehicles × 3 reaction times).
Crash reconstructions
Crash reconstructions were performed in 2 parts. First, the positions of the vehicles prior to impact were reconstructed using the scene diagram prepared by the crash investigator. Second, a series of models was used to determine impact and precrash vehicle speeds.
Path reconstruction
The procedure used for determining precrash vehicle paths has been described in several prior studies (Scanlon, Page, et al. Citation2016; Scanlon, Sherony, and Gabler Citation2016d). The location where each driver entered the intersection was first determined for every crash. If a stop bar was present, it was used as the intersection entry location. If a stop bar was not present but a crosswalk was present, the location where the vehicle first crossed the crosswalk was taken as the intersection entry location. If neither a stop bar nor crosswalk was present, the intersection boundary line was used as the intersection entry location. The intersection boundary line was drawn by extrapolating the road edges through the intersection.
Each vehicle was assumed to have taken the path indicated by the crash investigator in the scene diagram. Measurements were taken from the NMVCCS scene diagram in order to draw the vehicles path leading up to impact. A full summary of these measurements can be found in the Appendix (see online supplement). Vehicle dimensions were obtained from the Canadian Vehicle Specifications database (Canadian Association of Road Safety Professionals Citation2015).
Speed reconstruction
Each driver's speed profile leading up to impact were determined using (1) the precrash movement of the driver and (2) the reconstructed impact speed. The precrash movement of each driver was placed into one of 3 categories. Drivers who proceeded into the intersection from a full stop were labeled “completely stopped.” Drivers who accelerated into the intersection after slowing, but not coming to rest, were labeled “rolling stopped.” Drivers who did not slow or stop prior to entering the intersection were labeled “traveling through.” The precrash movement of each driver was determined by examining the coded “PREMOVE” variable and the crash narrative prepared by the investigator. The “PREMOVE” variable was occasionally found to be inconsistent with the crash narrative in coding for drivers who stopped. Additionally, the variable lacks details regarding whether the driver performed a complete or rolling stop. The scene narrative was used in conjunction with the “PREMOVE” variable in order to determine each vehicle's most likely movements.
The impact speeds of vehicles were reconstructed using PC-Crash simulation software (Steffan Citation2011b; Steffan Citation2011a). This software simulates impacts using an impulse restitution model, which models compressive and restitution forces as occurring about a single point in equal and opposite directions. This modeling software accounts for each vehicle's weight, center of gravity location, stiffness, additional cargo and occupant weight, and friction between the road–tire interfaces. The software's internal genetic optimization algorithm was used to determine the impact speeds that best replicated the intermediate and rest positions depicted in the scene diagram. Previous studies have used staged crash tests to validate the PC-Crash impact model (Cliff and Montgomery Citation1996) and the impact speed optimizer tool (Cliff and Moser Citation2001).
Completely and rolling stopped
The speed profiles of completely and rolling stopped drivers were reconstructed using similar methods. First, the deceleration and acceleration phases of the vehicles were estimated and “stitched” together. Second, the impact speed was used to determine whether the driver was likely to have taken evasive braking action.
The deceleration phase of the driver until the point of minimum speed was modeled using a developed kinematics model of vehicle speed versus distance. Similar to the work by Noble et al. (Citation2016), regression models were generated using multivariate adaptive regression splines. This model was developed using the 100-Car Naturalistic Driving Study data collected by the Virginia Tech Transportation Institute (Dingus et al. Citation2006). To develop this model, approximately 12,000 previously identified (Sudweeks et al. Citation2007) speed traces of intersection approaches for complete and rolling stopped drivers were extracted from the 100-Car Naturalistic Driving Study data set. Model development was performed in MATLAB (The Mathworks Inc., Natlick, MA, USA) using the ARESLab toolbox (Jekabsons Citation2015). A complete summary of this model can be found in the Appendix.
The acceleration profiles of the drivers were estimated using a previously developed intersection traversal model (Scanlon, Sherony, and Gabler Citation2016b). This acceleration profile model represents vehicle acceleration as a function of velocity and was generated using a data set of event data recorder (EDR) records collected from vehicles involved in real-world, SCP intersection crashes.
The reconstructed impact speed was used to determine if the driver did or did not take evasive action (Scanlon, Sherony, and Gabler Citation2016b). The driver was initially assumed to have started accelerating into the intersection at the intersection entry location (i.e., the stop bar, crosswalk, or intersection boundary line). In the event that the reconstructed impact speed was less than the simulated speed at the point of impact, the driver was assumed to have evasively braked prior to impact, which is frequently observed during real-world intersection crashes (Scanlon, Kusano, and Gabler Citation2015). If the reconstructed impact speed was greater than the simulated speed, the driver was assumed to have not taken evasive braking action. In this scenario without evasive braking, the beginning of the acceleration was moved away from the intersection until the simulated impact speed matched the reconstructed impact speed.
Traveling through
Traveling-through vehicle speeds were reconstructed in 2 phases. First, a previously developed logistic regression model was used to estimate the likelihood that crash avoidance braking occurred given the reconstructed impact speed (Scanlon, Sherony, and Gabler Citation2016a). This model was developed using data collected from EDRs that had been taken from vehicles involved in real-world intersection crashes. Second, each speed profile was then reconstructed with and without evasive braking. The probability of an evasive braking action having occurred was used to weight each scenario. The most likely traversal speed for the trajectory with evasive braking was estimated using a linear regression model developed from the same data set of EDRs.
Only one simulation was modeled if the impact speed was excessively high or low. Specifically, if the probability of an evasive braking having or having not occurred was above 99%, only the more likely scenario was simulated. Additionally, if the impact speed was below 15 mph for traveling-through, straight-crossing drivers, only a scenario where the driver evasive braked was simulated.
Evasive braking
Evasive braking was modeled by assuming an initial jerk of −11.0 m/s3 down to some maximum deceleration value. This jerk magnitude is within the range of values typically observed by drivers during conflict situations (Nygård Citation1999). In general, maximum vehicle deceleration capacity depends on a number of factors, including vehicle characteristics, surface conditions, vehicle speed, and surface type (Baker Citation1975; Blau Citation2008; Franck and Franck Citation2009). This study used maximum deceleration values of 0.3, 0.4, or 0.8 g based on whether the road conditions were icy, wet, or dry, respectively.
I-ADAS simulations
An overview of the approach of the hypothetical I-ADAS modeled is shown in . Onboard sensors were assumed to be continuously scanning for oncoming vehicles. At the instant the first vehicle entered the intersection, a clear line of sight was modeled between the vehicles. After the oncoming vehicle was first within sensor range, some computational time would be required for the sensor to detect the approaching vehicle. I-ADAS would then either autonomously brake or deliver a warning to the driver if the crash was deemed imminent (i.e., if the crash was estimated to occur within a predefined time window). For a system that delivers a warning, driver reaction would have occurred only after some perception–reaction time.
A total of 30 variations of I-ADAS were considered in this study, which consisted of 5 separate time-to-collision (TTC) thresholds, 3 separate computational latencies, and 2 different I-ADAS response modalities (i.e., a warning or autonomous emergency braking, AEB). Every crash was simulated as if one of the vehicles had been equipped with an I-ADAS. To model I-ADAS, this study assumed the equipped vehicle had 2 frontal-side vehicle detection sensors. Each sensor was modeled as being oriented at 45° away from the vehicle heading, with a maximum detection range of 120 m and a field of view ±60°. These specifications are within the capacity of several commercially available, vehicle-based radars, such as Delphi Corporation's Electronically Scanning Radar, Smartmicro's UMRR Automotive Radar Sensors, and Eaton's VORAD® VS-400. Additionally, our preliminary analysis of these specifications indicated that 98% of oncoming vehicles could have been detected at the instant the first vehicle entered the intersection.
At a given time point, a warning to the driver or autonomous braking was only assumed to occur (a) if the oncoming vehicle could have been detected by the onboard sensors, (b) if the TTC fell below a predefined threshold, and (c) after some computational latency time. The earliest vehicle detection opportunity was assumed to be at the time point when the first vehicle entered the intersection (e.g., crossed over the stop bar). Because sightline restrictions are expected to limit sensor detection capacity during the intersection approach, this assumption implies that a clear line of sight is only possible after one of the vehicles had crossed into the intersection.
There is some inherent computational latency time while I-ADAS is processing incoming sensor data. Latency time can depend on a variety of factors, such as the number of vehicles being detected by the sensor (Kaempchen and Dietmayer Citation2003). Three separate latency times were considered in this study: 0-, 0.25-, and 0.5-s delays.
A crash was deemed imminent if the TTC fell below a predefined threshold. TTC was calculated by assuming that the I-ADAS equipped vehicle would maintain its current acceleration until the point of impact. The distance until the 2 vehicles would impact was calculated by extrapolating forward each vehicle's positions until they intersected. Like other active safety systems (Kusano and Gabler Citation2015a), the timing thresholds used for these system are automaker and model specific. Accordingly, 5 seperate TTC values were evaluated in this study, including 1.0-, 1.5-, 2.0-, 2.5-, and 3.0-s thresholds.
Two types of I-ADAS were evaluated in this study: one that delivers a warning and a second that autonomously brakes. For the system that delivers a warning, the driver was assumed to take crash avoidance braking action after some perception–reaction time. A previous simulator study analyzing driver reaction times in response to a warning in cross-traffic intersection crash scenarios was used to determine an appropriate reaction time between warning delivery and driver response (H. Chen et al. Citation2011). There is a distribution of times for how long it would take a driver to respond to an I-ADAS warning. Accordingly, every reconstructed crash was simulated with 3 equally probable reaction time values, 0.70, 0.99, and 1.89 s, which represent the 17th, 50th, and 83rd percentiles of reaction times, accordingly. Autonomous braking was assumed to begin without the delay of reaction time.
Drivers were assumed to brake with a jerk value of −11m/s3 (Nygård Citation1999), whereas autonomous braking was modeled using a substantially higher value of −35 m/s3 (Grover et al. Citation2013). Following the approach of our crash reconstructions, maximum evasive braking deceleration magnitudes were assumed to be dependent on the road surface conditions.
Injury benefits estimates
A key objective of this study was to project the potential reduction of injuries with and without I-ADAS in a future fleet. Because NMVCCS was compiled from 2005 to 2007, the proportion of crashed vehicles equipped with side airbags was expected to be substantially lower in the current fleet. Likewise, not all NMVCCS vehicles were equipped with frontal airbags. Because the intent of this study was to project I-ADAS effectiveness in a future fleet, we modeled all vehicles in the study as though they were equipped with both frontal and side airbags.
Injury risk was modeled as a function of total delta-V and other covariates. In order to determine delta-V, impacts with and without I-ADAS were simulated using PC-Crash simulation software (Steffen Citation2011a; Steffan Citation2011b). Simulations were performed by placing each vehicle in their respective impact position and orientation. Each vehicle was then assigned their respective impact speeds and the impact simulation was run. Total delta-V was computed for each impact from the total change in vehicle velocity over the first 300 ms of impact.
This study generated several injury risk models to estimate likelihood of a seriously injured driver (Maximum Abbreviated Injury Scale score of 3 or greater, MAIS 3+; Association for the Advancement of Automotive Medicine Citation1998) given the impact conditions. Details of these models can be found in the Appendix. A data set of 19,386 crashed vehicles from the NASS-CDS (NHTSA Citation2016b) database were used to generate 3 injury risk models (frontal, near-side, and far-side) for this study. Logistic regression modeling was used to compute the likelihood of an injury versus impact severity. Several predictor variables that have been identified as influencing injury risk were included, such as total delta-V (Kononen et al. Citation2011; Kusano and Gabler Citation2012), seat belt use (Durbin et al. Citation2003, Citation2005), driver age (Langford and Koppel Citation2006; McCoy et al. Citation1989), driver gender (Beck et al. Citation2007; Mock et al. Citation2002; Yau Citation2004), and whether or not the vehicle was equipped with side airbags (Arbelaez et al. Citation2002; Kuppa et al. Citation2003). The computation for calculating system effectiveness (i.e., the proportion of intersection crashes/injuries that could have potentially been prevented) can be found in the Appendix.
Results
Data set composition
The data set from the analysis was composed of 448 SCP crashes. The original data set extracted from NMVCCS consisted of 770 crashes. The online Appendix contains a breakdown of excluded cases. Using NMVCCS case weights, the data set was representative of 150,159 crashes that occurred in the United States. If the vehicles in these crashes had been equipped with both frontal and side airbags but without I-ADAS, the models estimate that the crashes would have resulted in 12,449 drivers with MAIS 3+ injuries.
Summary of benefits estimates
Potential crash prevention estimates for all 30 variants of I-ADAS that were evaluated in this study can be found in . The model predicted that 25–59% of SCP crashes are potentially preventable by an I-ADAS that autonomously brakes via an AEB system. Conversely, for an I-ADAS that delivers an alert to the driver, the potential crash prevention effectiveness drops to merely 0–23% of SCP crashes. For crashes that were found to be unavoidable but where the driver/AEB was able to begin evasive braking earlier, modified impact conditions were observed. The proportion of modified impacts depended on the design of the I-ADAS and was as high as 46% of all crashes.
Figure 2. Proportion of crashes within the simulation case set that (a) were avoided, (b) had modified impact conditions, or (c) had unchanged impact conditions. All 30 variations of I-ADAS considered in this study are shown.
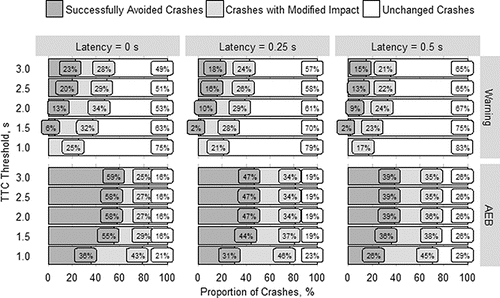
Even if a crash cannot be succesfully avoided, by reducing impact speeds there is the potential for injury mitigation/prevention. shows the proportion of vehicles with seriously injured drivers that could be potentially prevented if one of the involved vehicles had been equipped with an I-ADAS. The model predicts that up to 79% of vehicles with a seriously injured driver are potentially preventable with an I-ADAS that uses AEB. For an I-ADAS that uses a warning to the driver, up to only 23% of seriously injured drivers were estimated to be potentially preventable.
Effect of precrash behavior
Approximately one half (56%) of the crashes in the simulation case set involved at least one of the vehicles coming to a complete or rolling stop before entering the intersection. shows the proportion of crashes potentially prevantable by whether at least one of the involved drivers slowed or stopped prior to entering the intersection. For crashes where at least one vehicle stopped, the maximum computed potential benefits was 34% for a warning-based I-ADAS and 74% for an I-ADAS that utilized AEB. For crashes where neither vehicle stopped, the maximum potential benefits dropped dramatically to 10% of crashes if a warning-based I-ADAS was used and 41% for an I-ADAS with AEB.
Discussion
Summary of benefits estimates
The objective of this article was to estimate the proportion of vehicles with a seriously injured driver that could be potentially prevented if, for all U.S. SCP intersection crashes, one of the vehicles had been equipped with I-ADAS. The results of this article suggest that a substantial proportion of drivers with serious injuries would come from crashes that were not successfully avoided but where impact speeds were reduced. However, system effectiveness would be greatly dependent on design specifications.
This study has shown the importance of I-ADAS design on system effectiveness. A number of I-ADAS variants were considered in this study. First, any computational latency time from initial sensor detection to I-ADAS warning/AEB will limit system effectiveness (Kaempchen and Dietmayer Citation2003). The results suggest that increasing computational latency time from 0 to 0.5 s would reduce the proportion of preventable crashes/injuries by approximately one third. Without any latency, up to 23% of crashes were potentially preventable by an I-ADAS that uses a warning, and 59% of crashes were potentially preventable if AEB was used. If latency time was 0.5 s, the maximum proportion of crashes potentially preventable dropped to 15% with a warning-based system and 39% with a system that used AEB.
Second, the TTC threshold for determining when to deliver a warning or begin automated evasive action may influence the potential effectiveness of I-ADAS. For an I-ADAS that delivers an alert, crash/injury prevention effectiveness steadily increased from a TTC threshold of 1.0 s (no crashes or injuries prevented) to 3.0 s (15–23% of crashes, 18–25% of MAIS 3+ drivers). For an I-ADAS that autonomously brakes, a substantial increase in the proportion of crashes/injuries that could be prevented was observed from 1.0 s (25–36% of crashes, 50–72% of MAIS 3+ drivers) to 1.5 s (39–59% of crashes, 54–80% of MAIS 3+ drivers). Beyond 1.5 s, the predicted effectiveness estimates largely plateaued. This leveling-off time point for AEB is notable and can be interpreted as the amount of time required to ensure that a potentially avoidable crash is, in fact, avoided. The remaining crashes beyond this time point represent those with too short of an available time window for crash avoidance (i.e., elapsed time from earliest detection until impact).
The effect of TTC threshold is important to consider given the need for I-ADAS to give both a “timely” response and ensure driver acceptance of the technology. A sufficiently early I-ADAS response is essential for ensuring that crash prevention/injury mitigation is possible. Responses too late run the risk of limiting system effectiveness, whereas responses too early could lead to a higher proportion of false alarms, driver annoyance, less reactive drivers, and/or drivers turning off the systems altogether (Abe and Richardson Citation2006a, Citation2006b; Bliss and Acton Citation2003; Dingus et al. Citation1998; Neale and Dingus Citation2006; Scanlon, Sherony, and Gabler Citation2016c).
It is also important to consider these TTC values in the context of currently available ADAS technologies. Frontal crash avoidance systems (primarily intended for rear-end impacts), in general, deliver warnings at TTC values of around 2–3 s (Kusano and Gabler Citation2015a). For vehicles equipped with AEB, automated braking begins shortly after in the event that sufficient crash avoidance action has not been taken (Audi Citation2015; Lopez et al. Citation2015; Mercedes-Benz USA Citation2015; Toyota Motor Corporation Citation2016; Volvo Car Corporation Citation2015). It is likely unrealistic for implemented I-ADAS technology to initiate AEB at such high deceleration rates earlier than 2 s prior to a potential impact, especially given anticipated false-positive activations. The results from this study suggest that it may be appropriate for I-ADAS to first deliver a warning and, if necessary, apply emergency braking to help avoid a potential collision. Specifically, the model results suggest that, if the driver fails to adequately respond by 1.5 s prior to the crash, AEB activation would ensure near-maximum potential benefits.
Third, we were interested in comparing effectiveness estimates for an I-ADAS that utilizes an alert to the driver with an I-ADAS that autonomously brakes via AEB. The advantages of an AEB system are that it has the capacity to (a) respond sooner than a driver and (b) ramp up vehicle acceleration at a higher rate (i.e., higher jerk; Grover et al. Citation2013; Nygård Citation1999). In general, the results of this study suggest that I-ADAS will be 2–3 times more effective if an autonomous braking system is utilized over a warning-based system.
Effect of precrash behavior
Precrash stopping behavior was found to be an important governing factor influencing the effectiveness of I-ADAS. For drivers who slow or stop prior to accelerating into the intersection, I-ADAS is afforded additional time to detect and respond to an imminent crash. For a warning-based I-ADAS, the model predicted that the system has the potential to be over 3 times more effective in scenarios where one of the involved vehicles stopped. Given no computational latency and the most conservative TTC threshold (i.e., earliest TTC time of 3.0 s), only one out of every 10 crashes was estimated to be potentially preventable for crashes where neither vehicle stopped. Conversely, as many as 41% of crashes in which one vehicle stopped were potentially preventable if AEB were utilized. These results suggest that, if I-ADAS for SCP crashes is introduced with a warning-based technology, these systems would have greater success in crashes where at least one of the vehicles stopped are targeted.
Limitations
This study has a number of limitations. First, variability in certain aspects of how drivers approach and traverse intersections was not considered in this study. Factors such as acceleration rates, deceleration rates, and rolling stop speeds will influence the available time for an I-ADAS to deliver a warning or begin autonomous braking. This study took the approach of using models taken from the 50th percentile of driver behavior. Other factors, such as evasive braking action and reaction time, were simulated under several possible scenarios and weighted based on their likelihood of having occurred. Not accounting for variability in driver behavior could limit the generalizability of the results to various driving “styles” (e.g., accounting for aggressive driving styles). Expansions to the current study should focus on examining and accounting for the variability that exists in how drivers navigate intersections.
Second, our study makes several assumptions about obstructions. In general, sightline restrictions at intersections can be divided into 2 main categories: fixed and moving. This study's assumptions handle the bulk of fixed obstructions. Our study assumes that sightlines are completely obstructed until the first vehicle enters the intersection. Vehicles in our model cannot see around corners. This assumption corresponds to vehicles operating in an “urban canyon.” However, this assumption would also subsume cases in which approaches to intersections were obstructed by trees, shrubs, and signs. There were a few cases where objects/trees in the median could lead to the effectiveness being overestimated in some cases. Likewise, in many crashes in more open environments, vehicles could have a clear sightline earlier than was modeled, which could lead to an underestimation of effectiveness. Moving obstructions, such as roadway traffic or parked vehicles, were not accounted for in this study. By not accounting for these moving obstructions, effectiveness estimates are likely to be overestimated for some crashes.
Third, our study used a hypothetical I-ADAS algorithm. The exact specifications and algorithms developed by automakers are highly proprietary. In an effort to examine sensitivity to TTC threshold and computational latency time, our study considered several potential algorithms. Although this approach allows for a general analysis of I-ADAS, examining algorithms actually utilized by designers would allow for a general comparison of design. Fourth, drivers and autonomous crash avoidance systems could use steering to avoid a crash, but this countermeasure was not considered in this study's simulations. Higher benefits estimates would be expected if this extra degree of freedom were considered in the model. It should be noted, however, that automated steering technology is still being developed and is not currently implemented in existing I-ADAS technology. Fifth, in some cases, although the simulated first collision may have been avoided, there is always the potential for a separate vehicle to have been impacted. This assumption could lead to overestimates of system effectiveness in some crashes.
gcpi_a_1300257_sm3004.docx
Download MS Word (261.9 KB)Acknowledgments
Our special thanks to Katsuhiko Iwazaki of Toyota for sharing his technical insights and expertise throughout the project. The authors also acknowledge the undergraduate and graduate research assistants at Virginia Tech for their assistance in building the data sets used in this study: David Holmes, Stephen Hunter, Daniel Gutierrez, Kaitlyn Wheeler, Stephani Martinelli, Kristin Dunford, Kay Battogtokh, Elizabeth Mack, Robert Vasinko, Daniel Surinach, Dong Gyu Lee, Dillon Richardson, Kevin Ota, and Arnab Gupta.
Funding
The authors acknowledge the Toyota Collaborative Safety Research Center (CSRC) and Toyota Motor Corporation for funding this study.
References
- Abe G, Richardson J. Alarm timing, trust and driver expectation for forward collision warning systems. Appl Ergon. 2006a;37:577–586.
- Abe G, Richardson J. The influence of alarm timing on driver response to collision warning systems following system failure. Behav Inf Technol. 2006b;25:443–452.
- Arbelaez RA, Nolan JM, Dakin GJ, Lund AK. Comparison of EuroSID-2 and SID-IIs in vehicle side impact tests with the IIHS barrier. Stapp Car Crash J. 2002;46:397–415.
- Association for the Advancement of Automotive Medicine. The Abbreviated Injury Scale–1990, Update 1998. Des Plaines, IL: Author; 1998.
- Audi. 2016 Audi Q7 Owner's Manual. Germany: Audi AG; 2015.
- Baker JS. Traffic Accident Investigation Manual. 1st ed. Evanston, IL: Northwestern University Traffic Institute; 1975.
- Beck LF, Dellinger AM, O'Neil ME. Motor vehicle crash injury rates by mode of travel, United States: using exposure-based methods to quantify differences. Am J Epidemiol. 2007;166:212–218.
- Bellis E, Page J. National Motor Vehicle Crash Causation Survey SAS Analytical Users Manual. Washington, DC: National Highway Traffic Safety Administration; 2008.
- Blau PJ. Friction Science and Technology: From Concepts to Applications. Boca Raton, FL: CRC Press; 2008.
- Bliss JP, Acton SA. Alarm mistrust in automobiles: how collision alarm reliability affects driving. Appl Ergon. 2003;34:499–509.
- Braver ER, Ferguson SA, Greene MA, Lund AK. Reductions in deaths in frontal crashes among right front passengers in vehicles equipped with passenger air bags. JAMA, 1997;278:1437–1439.
- Canadian Association of Road Safety Professionals. Canadian Vehicle Specifications. 2015. Available at: http://www.carsp.ca/research/resources/safety-sources/canadian-vehicle-specifications/. Accessed: August 26, 2015.
- Chen H, Cao L, Logan DB. Investigation into the effect of an intersection crash warning system on driving performance in a simulator. Traffic Inj Prev. 2011;12:529–537.
- Chen R, Gabler HC. Risk of thoracic injury from direct steering wheel impact in frontal crashes. J Trauma Acute Care Surg. 2014;76:1441–1446.
- Cho Y, Rice J. Estimating velocity fields on a freeway from low-resolution videos. IEEE Trans Intell Transp Syst. 2006;7:463–469.
- Cliff WE, Montgomery DT. Validation of PC-Crash—A Momentum-Based Accident Reconstruction Program. Warrendale, PA: SAE International; 1996.
- Cliff WE, Moser A. Reconstruction of Twenty Staged Collisions with PC-Crash's Optimizer. Warrendale, PA: SAE International; 2001.
- Dingus TA, Jahns SK, Horowitz AD, Knipling R. Human Factors Design Issues for Crash Avoidance Systems. New York, NY: Psychology Press; 1998.
- Dingus TA, Klauer S, Neale V, et al. The 100-Car Naturalistic Driving Study, Phase II—Results of the 100-Car Field Experiment. Washington, DC: US Department of Transportation; 2006.
- Doerzaph Z, Neale V, Bowman J, Viita D, Maile M. Cooperative Intersection Collision Avoidance System Limited to Stop Sign and Traffic Signal Violations (CICAS-V) Subtask 3.2 Interim Report: Naturalistic Infrastructure-Based Driving Data Collection and Intersection Collision Avoidance Algorithm Development. NHTSA; 2008. Tech. Rep.
- Dr. Steffan Datentechnik GmbH. PC-Crash 9.1. Linz, Austria; 2011.
- Durbin DR, Chen I, Smith R, Elliott MR, Winston FK. Effects of seating position and appropriate restraint use on the risk of injury to children in motor vehicle crashes. Pediatrics. 2005;115:e305–e309.
- Durbin DR, Elliott MR, Winston FK. Belt-positioning booster seats and reduction in risk of injury among children in vehicle crashes. JAMA. 2003;289:2835–2840.
- Federal Highway Administration. Manual on Uniform Traffic Control Devices. Washington, DC: Author; 2009.
- Franck H, Franck D. Mathematical Methods for Accident Reconstruction: A Forensic Engineering Perspective. Boca Raton, FL: CRC Press; 2009.
- Fuerstenberg KC, Dietmayer KC, Willhoeft V. Pedestrian recognition in urban traffic using a vehicle based multilayer laserscanner. Paper presented at: IEEE Intelligent Vehicle Symposium, Versailles, France, June 17–21, 2002.
- Gabauer DJ, Gabler HC. The effects of airbags and seatbelts on occupant injury in longitudinal barrier crashes. J Safety Res. 2010;41:9–15.
- Grover C, Knight I, Okoro F, et al. Automated emergency brake systems: technical requirements, costs and benefits. 2013. Available at: http://europa.eu/enterprise/sectors/automotive/files/projects/report_aebs_en.pdf2008.1908855681.
- Hollowell WT, Gabler HC, Stucki SL, Summers S, Hackney JR. Updated Review of Potential Test Procedures for FMVSS No. 208. 1999. NHTSA Docket No. 6407-6406.
- IEEE Connected Vehicles. First Toyota Cars to Include V2V and V2I Communication by the End of 2015. IEEE Vehicular Technology Society 2015. Available at: http://sites.ieee.org/connected-vehicles/2015/09/30/first-toyota-cars-to-include-v2v-and-v2i-communication-by-the-end-of-2015/.
- Jekabsons G. ARESLab: adaptive regression splines toolbox for Matlab/Octave. 2015. Available at: http://www.cs.rtu.lv/jekabsons/
- Kaempchen N, Dietmayer K. Data synchronization strategies for multi-sensor fusion. Paper presented at: IEEE Conference on Intelligent Transportation Systems; Shanghai, China, October 12–15, 2003.
- Kononen DW, Flannagan CA, Wang SC. Identification and validation of a logistic regression model for predicting serious injuries associated with motor vehicle crashes. Accid Anal Prev. 2011;43:112–122.
- Kuppa S, Eppinger RH, McKoy F, Nguyen T. Development of side impact thoracic injury criteria and their application to the modified ES-2 dummy with rib extensions (ES-2re). Stapp Car Crash J. 2003;47:189–210.
- Kusano KD, Gabler HC. Safety benefits of forward collision warning, brake assist, and autonomous braking systems in rear-end collisions. IEEE Trans Intell Transp Syst. 2012;13:1546–1555.
- Kusano KD, Gabler HC. Comprehensive target populations for current active safety systems using national crash databases. Traffic Inj Prev. 2013;15:753–761.
- Kusano KD, Gabler HC. Comparison of expected crash and injury reduction from production forward collision and lane departure warning systems. Traffic Inj Prev. 2015a;16(Suppl 2):S109–S114.
- Kusano KD, Gabler HC. Target population for intersection advanced driver assistance systems in the US. SAE Int J Transp Saf. 2015b;3:1–16.
- Langford J, Koppel S. Epidemiology of older driver crashes—identifying older driver risk factors and exposure patterns. Transp Res Part F Traffic Psychol Behav. 2006;9:309–321.
- Li X, Shu W, Li M, Huang H-Y, Luo P-E, Wu M-Y. Performance evaluation of vehicle-based mobile sensor networks for traffic monitoring. IEEE Transactions on Vehicular Technology. 2009;58:1647–1653.
- Lopez A, Sherony R, Chien S, Li L, Qiang Y, Chen Y. Analysis of the braking behaviour in pedestrian automatic emergency braking. Paper presented at: IEEE 18th International Conference on Intelligent Transportation Systems; Canary Islands, Spain, September 15–18, 2015.
- McCoy G, Johnstone R, Duthie R. Injury to the elderly in road traffic accidents. J Trauma Acute Care Surg. 1989;29:494–497.
- Mercedes-Benz USA. 2016 S-Class Operator's Manual. Stuttgart, Germany: Daimler AG; 2015.
- Mock CN, Grossman DC, Kaufman RP, Mack CD, Rivara FP. The relationship between body weight and risk of death and serious injury in motor vehicle crashes. Accid Anal Prev. 2002;34:221–228.
- Moorcroft DM, Stitzel JD, Duma GG, Duma SM. Computational model of the pregnant occupant: predicting the risk of injury in automobile crashes. Am J Obstet Gynecol. 2003;189:540–544.
- Neale V, Dingus T. Motor vehicle warnings. In: Wogalter EMS, ed. Handbook of warnings. Mahwah, NJ: Lawrence Erlbaum Associates; 2006:687–700.
- NHTSA. National Motor Vehicle Crash Causation Survey: Report to Congress. Washington, DC: US Department of Transportation; 2008.
- NHTSA. Characterization Test Procedures for Intersection Collision Avoidance Systems Based on Vehicle-to-Vehicle Communications. Washington, DC: US Department of Transportation; 2015.
- NHTSA. Fatality Analysis Reporting System (FARS): Analytical User's Manual 1975–2015. Washington, DC: US Department of Transportation; 2016a.
- NHTSA. National Automotive Sampling System–Crashworthiness Data System: 2015 Analytical User's Manual. Washington, DC: US Department of Transportation; 2016b.
- NHTSA. National Automotive Sampling System (NASS) General Estimates System (GES): Analytical User's Manual 1988–2015. Washington, DC: US Department of Transportation; 2016c.
- Noble AM, Kusano KD, Scanlon JM, Doerzaph Z, Gabler HC. Driver approach and traversal trajectories for signalized intersections using naturalistic data. Paper presented at: 95th Annual Meeting of the Transportation Research Board; Washington, DC, January 10–14, 2016.
- Nygård M. A method for analysing traffic safety with help of speed profiles. Paper presented at: FERSI Workshop for young researchers; Prague, Czech Republic, October 8–9, 1998.
- Papadimitratos P, La Fortelle A, Evenssen K, Brignolo R, Cosenza S. Vehicular communication systems: enabling technologies, applications, and future outlook on intelligent transportation. Commun Mag. 2009;47(11):84–95.
- Peng Y, Deck C, Yang J, Otte D, Willinger R. A study of adult pedestrian head impact conditions and injury risks in passenger car collisions based on real-world accident data. Traffic Inj Prev. 2013;14:639–646.
- Samad T, Annaswamy A. The impact of control technology: Overview, success stories, and research challenges. Available at: www.ieeecss.org2011
- SAS Institute Inc. SAS/STAT 9.3 User's Guide. Cary, NC: Author; 2011.
- Scanlon JM, Kusano KD, Gabler HC. Analysis of driver evasive maneuvering prior to intersection crashes using event data recorders. Traffic Inj Prev. 2015;16(Suppl 2):S182–S189.
- Scanlon JM, Kusano KD, Gabler HC. Lane departure warning and prevention systems in the US vehicle fleet: influence of roadway characteristics on potential safety benefits. Transp Res Rec. 2016;2559:17–23.
- Scanlon JM, Kusano KD, Sherony R, Gabler HC. Potential of intersection driver assistance systems to mitigate straight crossing path crashes using US nationally representative crash data. Paper presented at: 2015 IEEE Intelligent Vehicles Symposium; Seoul, Korea, June 28–July 1, 2015.
- Scanlon JM, Page K, Sherony R, Gabler HC. Using Event Data Recorders from Real-World Crashes to Investigate the Earliest Detection Opportunity for an Intersection Advanced Driver Assistance System. Warrendale, PA: SAE International; 2016. SAE Technical Paper No. 2016-01-1457.
- Scanlon JM, Sherony R, Gabler HC. Estimating pre-crash driver actions when you don't have an EDR. Accid Anal Prev. (in review); 2016a.
- Scanlon JM, Sherony R, Gabler HC. Models of driver acceleration behavior prior to real-world intersection crashes. IEEE Trans Intell Transp Syst. (in review); 2016b.
- Scanlon JM, Sherony R, Gabler HC. Predicting crash-relevant violations at stop sign-controlled intersections for the development of an intersection driver assistance system. Traffic Inj Prev. 2016c;17(Suppl 1):59–65.
- Scanlon JM, Sherony R, Gabler HC. Preliminary potential crash prevention estimates for an intersection advanced driver assistance system in straight crossing path crashes. Paper presented at: Intelligent Vehicles Symposium (IV); 2016d; Gothenburg, Sweden, June 19–22, 2016.
- Sherwood CP, Shaw CG, Van Rooij L, et al. Prediction of cervical spine injury risk for the 6-year-old child in frontal crashes. Traffic Inj Prev. 2003;4:206–213.
- Snedeker JG, Muser MH, Walz FH. Assessment of pelvis and upper leg injury risk in car–pedestrian collisions: comparison of accident statistics, impactor tests and a human body finite element model. Stapp Car Crash Journal. 2003;47:437–457.
- Steffan, H. PC-Crash 9.1 [computer program]. Linz, Austria: The Dr. Steffan Datentechnik GmbH; 2011a.
- Steffan H. PC-Crash: A Simulation Program for Vehicle Accidents - Technical Manual. Linz, Austria: The Dr. Steffan Datentechnik GmbH; 2011b.
- Sudweeks J, Neale V, Wiegand K, Bowman J, Perez M, Maile M. Cooperative Intersection Collision Avoidance Systems Limited to Stop Sign and Traffic Signal Violations (CICAS-V) Subtask 3.1 Report: Mining of the 100-Car Naturalistic Database to Determine Factors Related to Intersection Violations and Near Violations. Washington, DC: NHTSA; 2007.
- Toyota Motor Corporation. Lexus 2016 NX300H Owners Manual. Aichi Prefecture, Japan: Author; 2016.
- Van Rooij L, Sherwood C, Crandall J, Orzechowski K, Eichelberger M. The effects of vehicle seat belt parameters on the injury risk for children in booster seats. SAE Trans. 2003;112:470–482.
- Volvo Car Corporation. 2016 Volvo XC90 Owner's Manual. Gothenburg, Sweden: Author; 2015.
- Volvo Car USA. City Safety by Volvo Cars—outstanding crash prevention that is standard in the all-new XC90 [press release]. Gothenburg, Sweden: Author; 2014.
- Weaver AA, Loftis KL, Duma SM, Stitzel JD. Biomechanical modeling of eye trauma for different orbit anthropometries. J Biomech. 2011;44:1296–1303.
- Yau KK. Risk factors affecting the severity of single vehicle traffic accidents in Hong Kong. Accid Anal Prev. 2004;36:333–340.
- Zhang P, Parenteau C, Wang L, et al. Prediction of thoracic injury severity in frontal impacts by selected anatomical morphomic variables through model-averaged logistic regression approach. Accid Anal Prev. 2013;60:172–180.