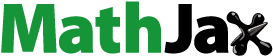
ABSTRACT
Objective: The research objective of the present investigation is to demonstrate the present status of passive in-vehicle driver breath alcohol detection and highlight the necessary conditions for large-scale implementation of such a system. Completely passive detection has remained a challenge mainly because of the requirements on signal resolution combined with the constraints of vehicle integration. The work is part of the Driver Alcohol Detection System for Safety (DADSS) program aiming at massive deployment of alcohol sensing systems that could potentially save thousands of American lives annually.
Method: The work reported here builds on earlier investigations, in which it has been shown that detection of alcohol vapor in the proximity of a human subject may be traced to that subject by means of simultaneous recording of carbon dioxide (CO2) at the same location. Sensors based on infrared spectroscopy were developed to detect and quantify low concentrations of alcohol and CO2. In the present investigation, alcohol and CO2 were recorded at various locations in a vehicle cabin while human subjects were performing normal in-step procedures and driving preparations. A video camera directed to the driver position was recording images of the driver's upper body parts, including the face, and the images were analyzed with respect to features of significance to the breathing behavior and breath detection, such as mouth opening and head direction.
Results: Improvement of the sensor system with respect to signal resolution including algorithm and software development, and fusion of the sensor and camera signals was successfully implemented and tested before starting the human study. In addition, experimental tests and simulations were performed with the purpose of connecting human subject data with repeatable experimental conditions. The results include occurrence statistics of detected breaths by signal peaks of CO2 and alcohol. From the statistical data, the accuracy of breath alcohol estimation and timing related to initial driver routines (door opening, taking a seat, door closure, buckling up, etc.) can be estimated.
The investigation confirmed the feasibility of passive driver breath alcohol detection using our present system. Trade-offs between timing and sensor signal resolution requirements will become critical. Further improvement of sensor resolution and system ruggedness is required before the results can be industrialized.
Conclusions: It is concluded that a further important step toward completely passive detection of driver breath alcohol has been taken. If required, the sniffer function with alcohol detection capability can be combined with a subsequent highly accurate breath test to confirm the driver's legal status using the same sensor device. The study is relevant to crash avoidance, in particular driver monitoring systems and driver–vehicle interface design.
Introduction
The number of fatalities and serious injuries related to drunk driving is high beyond proportion (NHTSA Citation2014), in view of the fact that a vast majority of vehicle drivers are sober. According to the NHTSA (Citation2015), the estimated societal cost for alcohol related accidents in the United States corresponds to a total amount of $34 billion annually. According to investigations on the risk of being involved in an accident, there is a sharp increase at high alcohol concentrations (Blomberg et al. Citation2005). Preventing highly intoxicated people from driving would potentially save many lives.
In this article, results are reported from a study of passive breath alcohol estimation in vehicles. The work is part of the Driver Alcohol Detection System for Safety (DADSS) program (Zaouk Citation2011) and related to other initiatives aiming at the prevention of drunk driving. The possibility of breath alcohol estimation in diluted breath samples in a vehicle cabin by using carbon dioxide as a tracer gas to compensate for the dilution has been demonstrated and evaluated elsewhere (Hök et al. 2006, Citation2010, Citation2014, 2015; Kaisdotter Andersson Citation2010; Kaisdotter Andersson et al. Citation2011, 2013; Ljungblad et al. 2014; Ljungblad, Hök, and Ekström Citation2016; Ljungblad, Hök, and Pettersson 2016). The results are building on the subsequent development of infrared sensing technology (Hummelgård et al. Citation2015) led by SenseAir, Sweden.
A basic requirement set forth in the DADSS program (Zaouk 2011) is that the sober driver should not be inconvenienced by the detection system. The system should be completely passive, requiring no active cooperation from the driver. Normally, the driver should be able to unlock and enter the vehicle, start the car, and drive away. The alcohol sensor is expected to respond almost instantaneously.
Obviously, breath alcohol detection assumes the occurrence of exhalations. Monitoring of driver behavior during in-step procedures is thus crucial to the detection system. In this investigation, the use of real-time image analysis of camera signals is introduced to supply complementary information to the CO2 and alcohol sensors used in the passive detection system.
Completely passive breath alcohol detection requiring no cooperation from the driver has remained a major technological challenge. The objective of the present investigation was to quantify the detailed requirements for in-vehicle passive detection using real-time image processing of camera signals to obtain improved performance. Simulations and experiments were performed in a realistic setting. Important aspects to consider are time to classification and reliability of measurement. The aim of the article is to report progress toward the goal of a completely passive in-vehicle breath alcohol sensor system.
Methods and technology
Sensor system
The sensor system used in the present investigation is based on infrared spectroscopy operating at the wavelength band at 9.5 µm for alcohol detection and 4.26 µm for CO2 detection. The optical cavity includes a set of high-precision mirrors to allow multiple reflections within the cavity, according to a method proposed by White (Citation1942). More details of the sensor system and its performance have been published elsewhere (Hök et al. Citation2014, 2015; Ljungblad et al. 2014).
The sensor system may be integrated into suitable parts of the vehicle cabin inventory as shown in . The left picture shows an integration within the casing above the steering column, and the picture at right shows a sensor positioned at the side door. In the current investigation, steering column implementation was evaluated. Cabin air is continuously drawn from the sensor inlet through the optical cavity. The sensor signals allow continuous monitoring of the CO2 and alcohol concentrations with a repetition rate of 5 Hz.
Figure 1. Sensors integrated into the casing of the steering column of a vehicle (left) and in the side door (right).
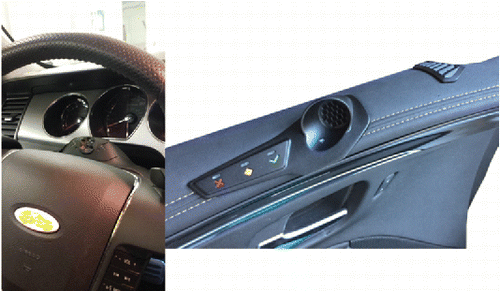
The distance between the sensor at the steering column and the driver's head is typically 40–70 cm. The exhaled breath will thus be highly diluted when entering the sensor, calling for exceptional demands on signal resolution.
Bench testing of diluted gas pulses
An experimental setup was designed and constructed to test the degree of dilution obtained at different distances from the source. Repetitive gas pulses were generated from gas bottles with specified concentration and humidified by passing through a temperature-controlled water bath. The gas pulse volume, flow, and repetition rate were controlled by valves connected to a desk-top computer.
A photograph of the experimental setup for testing of diluted gas pulses is shown in . The gas pulses are emitted vertically from the metal casing at the bottom, and the sensor attachment is movable on rails, at different distances above the gas pulse generator. The measurements were performed at room temperature in a laboratory environment. A reference instrument (Evidenzer, Nanoplus AB, Uppsala, Sweden) was used to verify the actual gas pulse concentrations. The number of tests at each concentration and distance is given in .
Vehicle simulations
Using the simulated breaths from a driver and the cabin enclosure as boundary conditions, it is possible to implement a total cabin air flow simulation by solving the adequate differential equations numerically for plume dispersion (Tang et al. Citation2006).
In-vehicle testing
Tests were performed in a vehicle setting, using both human test subjects and a test gas generator, similar to the one used for bench tests. In this case, the gas generators and valve control equipment were located in the back seat of the car. shows a photograph of the equipment.
The equipment shown in allows gas pulses to be emitted from both the driver's seat and the passenger's seat. Nasal or oral gas flow can be simulated by separate orifices. Correct gas temperature and elimination of water condensation are achieved by means of preheated tubing all the way from the gas pulse generator to the orifice.
More information on the human subject side of the study has previously been published elsewhere (Ljungblad, Hök, and Pettersson Citation2016). Ten subjects were included in the first human subjects experiment, 9 males and 1 female. Seven subjects stayed sober for the duration of the test, and 3 subjects were given 0.8 g of alcohol per kilogram of body mass. For intoxicated subjects, alcohol was consumed in less than 15 min. The study included a sequence where subjects entered a vehicle, sat down in the driver's seat, and performed a simulated driving task for approximately 10 min. During this time period, sensor signals and video recordings were continuously logged. Sober subjects performed the task 5 times. Intoxicated subjects performed one measurement before drinking and continued with a new measurement every 30 min until their breath alcohol level was recorded below 0.03 mg/L. Throughout the tests, an evidential breath analyzer (Nanopuls AB) was used as a reference. A new reference sample was taken before each measurement sequence. All participating subjects were between 22 and 70 years old. One recording from the human subjects experiment is presented in the present report, showing data from an intoxicated subject. More results from the study can be found in Ljungblad, Hök, and Pettersson (Citation2016). The camera used for video recordings operated at 60 Hz. A passive system requires knowledge about the origin of the exhaled gas; that is, there is a need to know whether the detected gas comes from the driver. A camera solution identifying exhalation triggers performed by the driver is expected to increase the probability of determining the driver as the source of the exhalation. As a first step toward aligning camera data to driver behavior, vital breath-related facial features—for example, open/closed mouth—were extracted.
A similar study design was also used to further investigate the in-vehicle exhalation dynamics. This was done by placing the inlet of a high-resolution CO2 sensor system at the steering column of the vehicle. Sensor signals were again recorded during the previously stated sequence. During both studies, the sensor inlet was placed on top of the vehicle's steering column. The setup is given in . Signal recording commenced before the subjects entered the vehicle. Fifteen entries were made using the high-resolution CO2 sensor setup. The entries were divided among three subjects, all of whom were sober, male, and between 25 and 50 years old.
Results
This section summarizes the experimental results of the present investigation, including the measurements of gas pulses at various distances, use of image analysis for real-time behavioral monitoring, simulation of in-vehicle breath flow patterns, simulated and measured breath-by-breath signals, and human subjects test results.
The results of experiments performed in the setup shown in are summarized below and illustrated in the graphs in .
Figure 6. Measured alcohol concentration as a function of distance with gas pulses of 0 (blue circles) and 0.3 (red circles) mg/L.
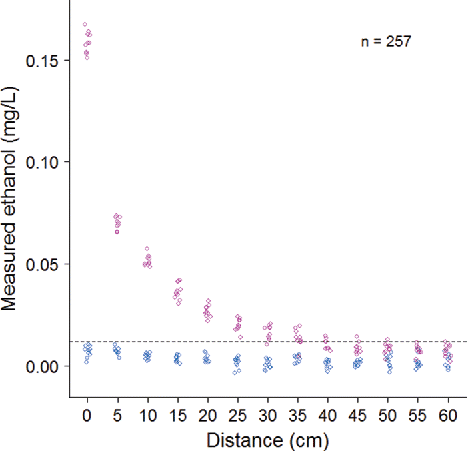
Figure 7. Measured dilution factor DF (given in Equation Equation1(1)
(1) ) as a function of distance.
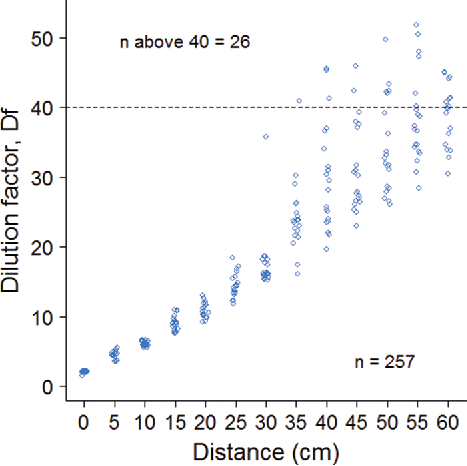
Figure 8. Estimation of breath alcohol concentration (BrAC = DF * EtOHmeas) for 131 recorded gas pulses.
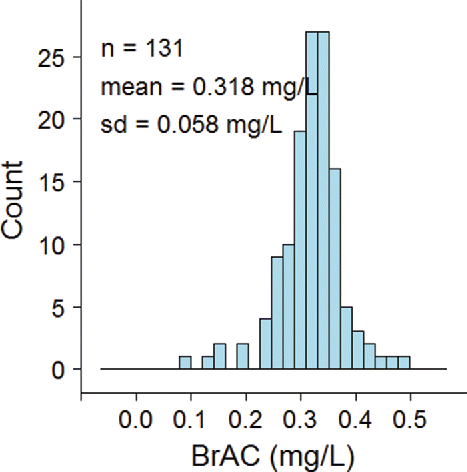
In , measured alcohol concentration in milligrams per liter is plotted against measurement distance in centimeters. The blue circles correspond to nominal zero concentration of the gas pulses and the red circles correspond to a nominal concentration of 0.3 mg/L. Two hundred fifty-seven tests were recorded. The observed variation in the output corresponds to a small offset and the signal noise, with magnitudes of approximately 0.01 mg/L and 0.002 mg/LRMS, respectively.
The red circles correspond to the signal output from gas pulses with a nominal concentration of 0.3 mg/L and a gas volume of approximately 1.5 L. At zero distance, the output is still significantly lower than the nominal value, and the signal declines with increasing distances, as expected from the higher degree of dilution.
From the measured CO2 concentrations, it is possible to determine the dilution factor DF according to the formula
(1)
(1)
CO2exp and CO2meas are the nominal and measured CO2 concentration of the gas pulses, respectively. CO2bgr is the background concentration.
The dilution factor is plotted against measurement distance in . As expected, DF increases with increasing distance. Furthermore, starting from a distance of approximately 30 cm, the variability increases dramatically.
Using the dilution factor, it is possible to calculate breath alcohol concentration (BrAC) according to the formula
The distribution shown in indicatesa small systematic overestimation and a standard deviation of 0.058 mg/L.
The image in includes landmarks indicating facial features that are useful for estimating the head distance and direction. Detection and tracking of facial landmarks are done with the CLM-Framework (Cambridge Face Tracker Citation2016). With the frame rate of 60 Hz it was possible to keep track of the facial landmarks with normal up/down, left/right head movements. Mouth-specific features enabled distinction between open and closed mouth; see .
Figure 9. Image of test subject including facial landmarks to define distance, direction, and mouth opening.
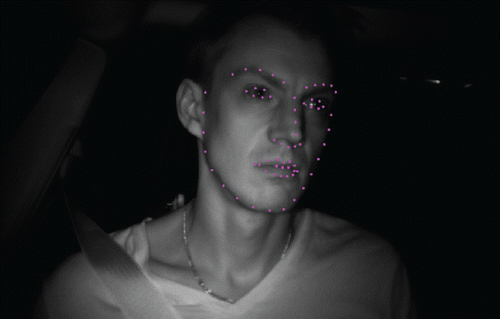
The simulation of in-vehicle breath flow patterns is shown in . Along with the actual scenario, the color-coded alcohol concentration (mass fraction) from breath is shown. The expanding plume corresponding to a mouth exhalation reaches the sensor area at a fairly high concentration. The input concentration at the mouth/nose region in the simulation was 5% and 1 mg/L.
The flow simulations allow sensor signals to be predicted at various locations. shows a breath-by-breath recording of signals at the steering column position in terms of simulated concentrations of CO2 and alcohol. Compared to the input concentrations, the observed dilution, according to Equation Equation1(1)
(1) , at the point of measurement was roughly DF = 30–40.
The graphs in show recorded CO2 and alcohol signals using the in-vehicle gas generator setup depicted in . The undiluted CO2 and alcohol concentrations were 5.0 vol% and 1 mg/L, respectively. From the peak magnitudes, the corresponding dilution factors of DF = 25–40 were calculated. The undiluted CO2 and alcohol concentrations correspond to the input values for the simulation.
Figure 13. Experimental recording of in-vehicle sensor signals using gas pulses from the setup depicted in : CO2 (top graph) and alcohol (bottom graph).
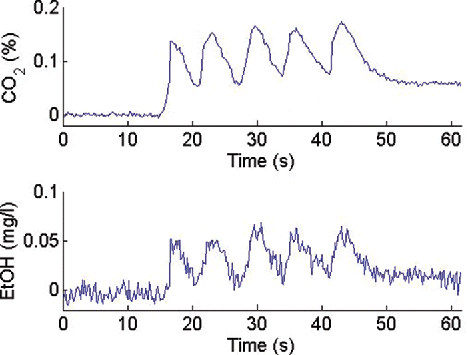
From the lower graph of it is evident that the noise of the alcohol of approximately 0.002 mg/LRMS sets an upper limit to the useful degree of dilution.
Tests with human subjects were performed in which the subjects performed a simulated driving task while the sensor signals were continuously monitored. shows a recording of CO2 and alcohol signals from an intoxicated subject. According to the reference, the true BrAC was 0.40 mg/L. During the recording lasting approximately 10 min, 5 major peaks are observed. The coincidence of CO2 and alcohol peaks allows for the estimation of breath alcohol concentration.
Fifteen signal recordings from a high-resolution CO2 sensor of subject entries into the research vehicle are summarized in the graph in . Note that the sensor saturates at slightly above 0.1 vol%. This sets a lower limit to the quantifiable dilution factor at 40. The recording clearly shows much more details than the recordings with a “standard” gen3 device.
Discussion
The results of the experiments performed in the laboratory and in-vehicle setups provide a more detailed understanding of the sensor signal behavior under conditions similar to a vehicle cabin. They confirm earlier results that in a passive detection mode, DF levels on the order of 100 or even higher can be expected. Consequently, further improvement in alcohol resolution is required.
Simulations of the air flow using an advanced model of a vehicle cabin provide a view of the propagation of gas pulses from the driver's mouth or nose to the sensor, in qualitative agreement with observed behavior. Reasonable quantitative agreement between experiments and simulations was also noted, when comparing observed and simulated signal dilution. The presented results concern the steady-state situation; that is, with no external interfering air flows. In the real world, environmental disturbances possibly caused by the vehicle's HVAC system will be present. Future investigations should include such effects.
The results from tests performed on human subjects were in good agreement with results from the test setups using artificial gas pulse generators and simulations. The measured alcohol concentrations were obviously lower, because the undiluted BrAC was 40% of the wet gas setup and simulation. These are important steps toward standardized test methods for passive in-vehicle breath alcohol detection. The availability of adequate objective test tools can be expected to improve the future development by allowing very time-consuming human subjects testing to be replaced by more efficient test methods. Standardizing in-vehicle test methods can never fully replace human subject tests, but before such trials take place vital knowledge can be gathered and will improve the outcome in future human subject tests.
The use of real-time image analysis of camera signals was tested with promising results. It was found that the head position and direction could be tracked with acceptable performance and the mouth opening could be quantified. Other important information can also be obtained using image analysis; for example, driver recognition may prove an important tool for identifying circumvention attempts by illicit subjects. The possibility of fusing these data with sensor signals will open new possibilities for improved breath detection reliability and to disclose driver behavior outside the norm.
Significant progress has been demonstrated toward the realization of passive breath alcohol detection in a standard vehicle cabin setting. Further work on the system design and sensor performance is expected to generate the necessary improvement. New tools for development and testing are expected to increase progress in the near future.
Acknowledgments
The authors express their thanks to all members of the project team at Autoliv, SenseAir, and Hök Instrument for their excellent contributions.
Funding
Financial contributions from the Automotive Coalition for Traffic Safety (ACTS), the National Highway Traffic Safety Administration (NHTSA), the Swedish Knowledge Foundation, and Vinnova, The Swedish Innovation Agency, are gratefully acknowledged.
References
- Blomberg RD, Peck RC, Burns M, Fiorentino D. Crash Risk of Alcohol Involved Driving: A Case–Control Study. Stamford, CT: Dunlap & Associates; 2005.
- Cambridge Face Tracker. Constrained local model based face tracking and landmark detection algorithms and their extensions/applications. 2016. Available at: https://github.com/TadasBaltrusaitis/CLM-framework. Accessed December 20, 2016.
- Hök B, Ljungblad J, Kaisdotter Andersson A, Ekström M, Enlund M. Unobtrusive and highly accurate breath alcohol determination enabled by improved methodology and technology. Journal of Forensic Investigation. 2014;2(4):1–8.
- Hök B, Pettersson H, Andersson G. Contactless measurement of breath alcohol. Paper presented at: Micro Structure Workshop; May 9–10, 2006; Västerås, Sweden.
- Hök B, Pettersson H, Kaisdotter Andersson A, Haasl S, Åkerlund P. Breath analyzer for Alcolocks and screening devices. IEEE Sens J. 2010;10:10–15.
- Hök B, Pettersson H, Ljungblad J. Unobtrusive breath alcohol sensing system. Paper presented at: 24th International Conference on the Enhanced Safety of Vehicles; June 8–11, 2015; Gothenburg, Sweden.
- Hummelgård C, Bryntse I, Bryzgalov M, et al. Low-cost NDIR based sensor platform for sub-ppm gas detection. Urban Climate. 2015;14:342–350.
- Kaisdotter Andersson A. Improved Breath Alcohol Analysis with Use of Carbon Dioxide as the Tracer Gas [doctoral thesis]. Västerås, Sweden: Mälardalen University; 2010.
- Kaisdotter Andersson A, Hök B, Karlsson A, Pettersson H. Unobtrusive breath alcohol testing. Paper presented at: International Conference on Alcohol, Drugs and Traffic Safety; August 25–28, 2013; Brisbane, Australia.
- Kaisdotter Andersson A, Hök B, Rentsch D, Rücker G, Ekström M. Improved breath alcohol analysis in patients with depressed consciousness. Med Biol Eng Comput. 2011;48:1099–1105.
- Ljungblad J, Hök B, Ekström M. Critical performance of a new breath analyzer for screening applications. Paper presented at: Intelligent Sensors, Sensor Networks and Information Processing; April 21–24, 2014; Singapore.
- Ljungblad J, Hök B, Ekström M. Development and evaluation of algorithms for breath alcohol screening. Sensors. 2016;16:1–7.
- Ljungblad J, Hök B, Pettersson H. Experimental proof-of-principle of in-vehicle passive breath alcohol estimation. Paper presented at: International Conference on Alcohol, Drugs and Traffic Safety; October 16–19, 2016; Gramado, Brazil.
- NHTSA. The Economic and Societal Impact of Motor Vehicle Crashes 2010. Washington, DC: Author;2014. DOT HS 812013.
- NHTSA. The Economic and Societal Impact of Motor Vehicle Crashes 2010 (Revised). Washington, DC: Author;2015. DOT HS 812013.
- Tang W, Huber A, Bell B, Schwarz W. Application of CFD simulations for short-range atmospheric dispersion over open fields and within arrays of buildings. Paper presented at: Joint Conference on the Applications of Air Pollution Meteorology with the A&WMA; January 30–February 2, 2006; Atlanta, GA.
- White JU. Long optical paths of large aperture. J Opt Soc Am. 1942;32:285–288.
- Zaouk A. Driver alcohol detection system for safety. Paper presented at: Transportation Research Board 90th Annual Meeting; January 25, 2011; Washington, DC.