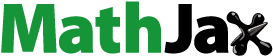
Abstract
Objectives
Vulnerable road users are globally overrepresented as victims of road traffic injuries. Developing biofidelic male and female pedestrian human body models (HBMs) that represent diverse anthropometries is essential to enhance road safety and propose intervention strategies.
Methods
In this study, 50th percentile male and female pedestrians of the SAFER HBM were developed via a newly developed image registration–based mesh morphing framework. The performance of the HBMs was evaluated by means of a set of cadaver experiments, involving subjects struck laterally by a generic sedan buck.
Results
In simulated whole-body pedestrian collisions, the personalized HBMs effectively replicate trajectories of the head and lower body regions, as well as head kinematics, in lateral impacts. The results also demonstrate the personalization framework’s capacity to generate personalized HBMs with reliable mesh quality, ensuring robust simulations.
Conclusions
The presented pedestrian HBMs and personalization framework provide robust means to reconstruct and evaluate head impacts in pedestrian-to-vehicle collisions thoroughly and accurately.
Introduction
With nonproportionally high fatality and injury rates (World Health Organization [WHO] Citation2018), the safety of vulnerable road users remains a leading public health concern worldwide. Vulnerable road users—that is, pedestrians, cyclists, and motorcyclists (Organisation for Economic Co-operation and Development Citation1998)—represent almost half of all traffic-related deaths (WHO Citation2018). With no indicators of progress in downward trends in the health burden (Ameratunga et al. Citation2006; WHO Citation2018; NHTSA Citation2021), there is an evident need for injury prevention measures.
Investigations of traffic accident situations have traditionally been carried out using full-scale anthropomorphic test devices (ATDs; i.e., crash test dummies). The widely used ATDs have been developed to mimic the anthropometry, articulation, and kinematic response of the human body in impacts, serving as a tool to predict potential human injuries in accidents (Crandall et al. Citation2011). However, ATD crash testing is a very time-consuming and expensive process, and full-scale pedestrian dummy tests are rarely imposed in current pedestrian safety test procedures. Most safety standards that encompass the pedestrian safety field have been concentrated on subsystem tests using impactors representing the most critical human body regions, such as the head or leg, even though it is debated to what extent these procedures represent real-world pedestrian accidents (Matsui et al. Citation2002; Ptak et al. Citation2012).
In the past decades, virtual human surrogates have been gaining more ground among researchers and in the automotive industry. Computational reconstructions of destructive crash scenarios are often carried out using state-of-the-art finite element (FE) human body models (HBMs). Because HBMs often are anatomically detailed, they can provide insights into the injury mechanisms of specific tissues during dynamic loading scenarios. As opposed to physical dummies, HBMs also enable measurements of tissue-based metrics for injury assessments (Crandall et al. Citation2011). A wide selection of HBMs are available, such as the Total HUman Model for Safety (THUMS; Iwamoto et al. Citation2002), the Global Human Body Models Consortium (Decker et al. Citation2019), and the VIVA + HBM (John et al. Citation2022). Another popular HBM is the SAFER HBM (Östh et al. Citation2021; Pipkorn et al. Citation2021), which was originally based on the THUMS v3. The SAFER HBM represents a 50th percentile male occupant and is provided with lumped internal organs and an active muscle package that enables human postural control (Östh et al. Citation2015). Because the head is one of the most commonly injured body part among road users, and head injuries are a major cause of death and hospitalization (Organisation for Economic Co-operation and Development Citation1998), the SAFER HBM also includes an extensively validated head model (Kleiven Citation2007).
Traditionally, ATDs and HBMs have been developed to mirror the anatomy of an average-sized U.S. male (Schneider et al. Citation1983). Recognition of the importance of including females and wider size ranges in traffic safety assessments is nevertheless increasing (Davis et al. Citation2016; Larsson et al. Citation2022). An advantage of HBMs is that their geometry can be modified to represent a broader anthropometric spectrum compared to full-scale ATDs. Multifold methods for geometric subject personalization have been used in association with the utilization of HBMs, with the most common approach being different forms of volumetric HBM scaling (Danelson and Stitzel Citation2015; Wu et al. Citation2017). For instance, scaling processes can introduce a length scaling factor to match the subject’s stature and a second scaling factor in the transverse plane to match the total body mass. An alternative approach to personalization involves parametric morphing of an HBM to match a target geometry (Piqueras-Lorente et al. Citation2018; Costa et al. Citation2020; Larsson et al. Citation2022). This option can often be more resource intensive due to its requirement for landmark and control point identification, followed by computationally intensive mesh transformations. Image registration–based morphing techniques that enable mesh morphing in a matter of minutes have been promoted in other fields of application (Li et al. Citation2021). This effective morphing strategy has also been shown to be effective for HBM personalization (Li et al. Citation2023). Correcting HBM geometries using morphing techniques has been shown to be the prevailing option for personalization in regard to maintaining biofidelity (Poulard et al. Citation2016).
Currently, the SAFER HBM is only available in a seated occupant posture. Despite the fact that vulnerable road users are globally overrepresented as victims of road traffic injuries, most proposed intervention strategies have been centered on the protection of vehicle occupants and less on vulnerable road users (Crandall et al. Citation2002; Ameratunga et al. Citation2006). Vulnerable road users should be of substantial focus in current prevention strategies, and developing biofidelic pedestrian HBMs is imperative to follow through with these efforts. Including female versions and supplying personalization pipelines along with the HBMs are equally important because the anthropometry has shown to have a nonnegligible influence on simulation accuracy (Poulard et al. Citation2016; Larsson et al. Citation2019, Citation2022). Evidence suggesting that females have a higher risk of sustaining injuries during motor vehicle crashes compared to males (Ulfarsson and Mannering Citation2004; Evans and Gerrish Citation2001; Evans Citation2001) provides an emphasis on the necessity of further developments.
The aim of this study is thus to develop baseline male and female pedestrian versions of the SAFER HBM, along with a robust framework for subject personalization. The study presents the application of a previously developed landmark-free image registration–based morphing approach that allows rapid generation of subject-specific HBMs (Li et al. Citation2023). The HBMs’ ability to capture head impact responses will be evaluated in whole-body impacts using a generic sedan buck. The HBMs are targeted for vehicle-to-pedestrian impact simulations, with an emphasis on head kinematics.
Methods
All simulations referred to in this study were preprocessed using LS-PrePost v4.8 (Citation2020) and PRIMER v18.1 (Citation2021). Simulations were performed using LS-DYNA v13 (Citation2021) with multiple CPUs, and postprocessing was carried out using MATLAB v2021a (Citation2021). An overview of the study is provided in .
Figure 1. Study overview and the generation of baseline male and female SAFER pedestrian HBMs: The occupant HBM was computationally positioned to an upright standing position by using pulling cables. The upright HBM was subsequently morphed to obtain the body shape and skeleton geometry representative of a 50th percentile male and female, constituting the newly established baseline pedestrian versions of the SAFER HBM. To evaluate the pedestrian HBMs and personalization technique, the male pedestrian HBM was morphed according to personalized anthropometries of 3 male PMHSs struck laterally using a generic buck model.
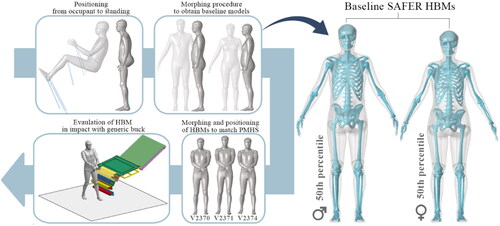
Development of male and female pedestrian HBMs
To obtain 2 baseline pedestrian HBMs, the SAFER occupant HBM was morphed to have the shape and inner skeleton geometry representative of a standing 50th percentile male and female. This was done in 4 processing steps: (1) preparation of target geometries, (2) preprocessing of the occupant HBM to obtain a template geometry, (3) the image registration–based morphing procedure, and (4) evaluation of the accuracy of personalization.
Target geometries
Target geometries were generated using 3 main tools: Skinned Multi-Person Linear Models (SMPLs), SMPL eXpressive (SMPLX), and the Obtaining Skeletal Shape from Outside (OSSO) system. SMPLs are skinned vertex-based models of the human body that represent a wide range of body shapes in natural human poses. The body models were originally generated based on input from the CEASAR data set, which compiles body scans of over 4,000 human subjects. Unlike SMPLs, SMPLX also enables the generation of per joint pose corrective blendshapes that depend on body pose, height, and weight. OSSO maps an internal skeleton to a 3D body surface. The OSSO-generated skeletons are based on 2,000 processed medical images of human subjects from the UK Biobank. References for these tools and data sets are provided in the Appendix (see online supplement).
An outer skin surface model was generated using SMPLX using height, weight, and gender as inputs. The 50th percentile male and female have been reported to have a height and weight of 175 cm and 77 kg and 162 cm and 62 kg, respectively (Schneider et al. Citation1983). The SMPLX models were positioned in an upright standing position using Blender v3.4 (Citation2022). The SMPLX was converted to SMPL format and a skeleton was inferred from the SMPL body shape using the OSSO system.
The primary advantage of using SMPLX is its ability to accept height/weight body mass index regression parameters for desired statures, a function that SMPL lacks. SMPL is needed because it is the only allowed input format in the OSSO system. The body and skeleton models were saved to 3-dimensional objects (STL format) and rigidly aligned with the head of the SAFER HBM. The obtained model geometries will be referred to as the target HBMs.
Preprocessing of the template HBM
To facilitate the impending morphing procedure, the SAFER HBM was roughly positioned from an occupant seated position to an upright standing position using an in-house-developed positioning script for PRIMER. The positioning follows the marionette method, partly described by Poulard et al. (Citation2015). In short, the model was forced into position in a series of simulations using pulling cables. The cables (formulated as beam elements) were defined with an initial tensile force that forced the movement, and displacement dampers were used to help the model settle during simulation. The cables were applied to prescribe the position and orientation of the head, shoulders, elbows, hands, knees, and feet. The technique allows smooth motion of the body parts from the start location to a target location, while maintaining the physiological constraints of the joints. The positioning was done stepwise with a series of simulations to avoid mesh distortion due to large element deformation. At the end of each simulation step, the nodal coordinates of the model were exported for the subsequent step. Manual adjustment of distorted elements and unwanted part intersections and penetrations was needed after positioning. The final positioned HBM had a minimum Jacobian of 0.12, which is comparable to the original occupant SAFER HBM, which had a minimum Jacobian of 0.14. Note how the body shape has been distorted, in particular the rear end of the HBM, as seen in .
Two separate surface models were created: One model containing the outer surface of the skeleton and one containing the outer skin surface. Both generated surface models were enclosed volumes and were converted to STL file format. The obtained upright SAFER HBM will be referred to as the template HBM.
Morphing pipeline
Based on the obtained target and template HBMs, 50th percentile male and female pedestrian HBMs were established following a newly developed image registration–based mesh morphing framework (Li et al. Citation2023), outlined in . The approach is based on the previous work of Li (Citation2021), who proposed an image registration–based morphing framework for head models that was later adapted for the application on HBMs (Li et al. Citation2021, Citation2023). Personalization of the SAFER pedestrian presented in this study was entirely automated using a MATLAB script. The required input is the rigidly aligned target geometry (STL format), and the morphed and ready-to-position SAFER pedestrian HBM along with its calculated Dice and Hausdorff distance (HD95) values were generated within a couple of minutes without any manual intervention.
Figure 3. Basic morphing framework using a target geometry and template HBM. (i) The target and template outer skin and skeleton are voxelized to segmented images and rigidly aligned by the head. (ii) Demons registration using the template image set as the fixed image and the target image set as moving image to generate a displacement field defined on the fixed image space. (iii) The displacement field is applied to the template HBM, morphing the HBM to the desired personalized HBM. (iv) The image of the template HBM is hardened by the inverse of the generated displacement field and is subsequently compared with the target image set to quantify personalization accuracy using Dice and HD95 metrics.
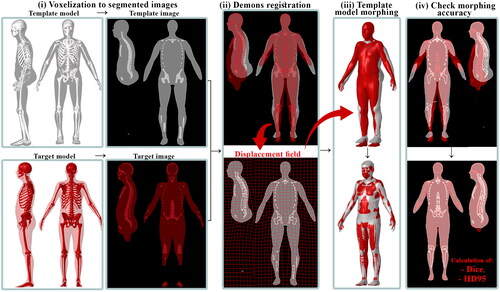
The morphing framework incorporates an initial preprocessing to voxelize the HBM shape to segmented images with 3 labels. The target and template models (i.e., the input skin and skeleton closed surface geometries) were voxelized using an open-source voxelization algorithm (Adam Citation2023). The voxel values were as follows: 0 for the background and 2 for the space enclosed within the skeleton surfaces; the remaining space between the skin surface and the skeleton surface had a value of 1 and represented the flesh, soft tissue, organs, and other matter inside the body.
Next, a displacement field was obtained using demons registration on the 3D image volumes. The displacement, which was later applied to the template HBM, defines a transformation of the template model mapped onto the target model. Here, a nonlinear registration method implemented in 3D Slicer (Citation2021) was used (Johnson and Zhao Citation2009). Deformable or nonrigid image registration algorithms are types of transformations that map one image to another image by finding a displacement field that optimally links or aligns the 2 images (Schwarz Citation2007). The software application used in the present study (Johnson and Zhao Citation2009) uses diffeomorphic demons algorithms to register a template image onto a target image and provides the resultant displacement fields in written file format. The resulting displacement field represents the distance between a geometric point in the target image and a point in the template image. The image registration was processed within 2 min for each case.
In the current application, the template images constitute the skeleton and outer skin surface of the SAFER HBM that was roughly positioned in a seated position. The target images were the 50th percentile skeleton and skin surfaces described in the preceding sections. Demons registration was performed on the segmented images containing both skin and skeleton.
The obtained displacement field was defined in the so-called fixed image space. Once the displacement field was generated in the above image registration, it was applied to the template HBM, leading to a geometrically corrected, or personalized, HBM. With this final step, the HBM assumed the geometry of the desired 50th percentile geometry, without compromising the mesh quality. Morphing was performed excluding the head and feet of the template SAFER HBM. This approach was used to maintain the integrity of the KTH head model and to isolate the geometrical correction to the area of interest. The resulting baseline male and female HBMs are illustrated in .
Evaluating morphing accuracy
To evaluate the accuracy of the registration, the voxelized image of the template HBM was warped by the inverse of the generated displacement fields. By comparing the warped image with the target image, the morphing accuracy can be quantified.
To measure the spatial overlap between the morphed HBMs and the target geometry, Dice scores (Ou et al. Citation2014) were calculated. The Dice coefficient can be used to compare the agreement between the morphed images and their corresponding ground truth. Defined as 2 times the element overlap divided by the total number of elements in both sets, a Dice value of 0 implies no overlap of the postmortem human subject (PMHS) surface and the morphed surface and 1 translates to a perfect overlap:
(1)
(1)
Here, A and B are the binary images of the target and morphed image sets, and and
are their corresponding number of voxels. The number of shared voxels by the 2 binary image sets is described by
.
As an additional accuracy surrogate, HD95 (Ou et al. Citation2014) was calculated. HD95 is a metric describing the maximum distance between any point on the morphed image and its nearest point on the target image and vice versa:
(2)
(2)
Here, d(m, t) is the Euclidean distance between the spatial locations of 2 points in the target point set T and morphed point set M, and m and t represent points in the respective sets. To avoid the influence of outliers, HD95 was calculated instead of the maximum HD. A small HD95 value indicate a better alignment of the skin and skeleton boundaries between the target and morphed image sets.
Any part intersections or negative Jacobians that might have been introduced after the morphing and/or positioning procedure were postprocessed and repaired if required.
Personalized validation of pedestrian HBMs
To evaluate the kinematic biofidelity of the SAFER HBM pedestrian, along with the presented personalization pipeline, personalized SAFER HBMs were evaluated by means of experiments using human cadavers conducted by Forman et al. (Forman, Joodaki, Forghani, Riley, Bollapragada, Lessley, Overby, Heltzel, and Crandall Citation2015; Forman, Joodaki, Forghani, Riley, Bollapragada, Lessley, Overby, Heltzel, Kerrigan et al. Citation2015). Forman et al. performed full-scale pedestrian impact tests on PMHS struck laterally in a mid-gait stance by a generic vehicle buck. Three tests were documented, involving 3 different PMHS denoted as V2370, V2371, and V2374. The trajectories of the head center of gravity (CoG), T1 and T8 vertebrae, pelvis, femurs, and tibias were tracked via video and a 3D motion tracking system and kinematic time histories from the head were gathered from accelerometers and angular rate sensors at selected several anatomical locations. For a comprehensive description of the conducted experiments, the reader is referred to the original publications (Forman, Joodaki, Forghani, Riley, Bollapragada, Lessley, Overby, Heltzel, and Crandall 2015; Forman, Joodaki, Forghani, Riley, Bollapragada, Lessley, Overby, Heltzel, Kerrigan et al. Citation2015).
Three SAFER HBMs were processed to match the anthropometries and stances of the PMHS. The presented morphing framework was followed to obtain representative body shapes, sizes, and skeletons of the PMHS. The generated baseline male 50th model was used as the template geometry and a SMPL model representative of each PMHS subject’s height and weight was obtained following the same approach as described in preceding sections of this article. The personalized HBMs were subsequently positioned to a mid-gait stance with the left foot forward and right foot behind the body. Positioning was done using the marionette method. The arms were positioned in front of the body and the hands were coupled using rigid-body constraints. The external measurements of the personalized HBMs matched well with the PMHS measurements, and a selection of representative dimensions are shown in . Note that subject V2374 is the same height and weight as the baseline 50th percentile male HBM and hence needed no morphing. The density of the HBM fat tissues was scaled to obtain the total body mass of the PMHS. The Jacobians of the positioned and morphed PMHS were all above 0.10.
The HBMs were struck laterally using a publicly available FE model of a generic sedan buck model developed by Pipkorn et al. (Citation2014). The friction coefficient between the HBM and the buck was set to 0.30. The dimensions of the vehicle were in agreement with the physical buck model used in the experiments; see further details in Pipkorn et al. (Citation2014). The impact velocities are presented together with details on the PMHS and HBM in .
Table 1. Anthropometric details and measurements of the positioned HBMs.
The HBMs were modeled with accelerometers at the same anatomical locations as the PMHS. Reaction forces were measured at joints on the buck model, placed at the same locations as the load cells between the load frame and frontal components of the buck in the experiments. The same coordinate system of the head and buck model as that in the experimental study was used (Forman, Joodaki, Forghani, Riley, Bollapragada, Lessley, Overby, Heltzel, and Crandall 2015; Forman, Joodaki, Forghani, Riley, Bollapragada, Lessley, Overby, Heltzel, Kerrigan et al. Citation2015). Trajectories and time histories are all truncated from the time of initial leg impact.
The capability of the SAFER HBM pedestrian to reproduce the trajectories of the anatomical locations (T1 and T8 vertebrae, pelvis, right and left femurs and tibias), along with the head linear and angular velocities and accelerations, was subsequently evaluated, as well as the produced reaction forces of the buck model. The linear acceleration and angular velocity signals were filtered to CFC 1000 and CFC 60, respectively, and all force signals were filtered to CFC 60, in accordance with the experiments.
To objectively evaluate the correlation of the simulation kinematic time histories of the head CoG with the PMHS head accelerometer, the CORrelation Analysis (CORA) rating method was used. The CORA score indicates how well 2 time histories correlate and ranges between 0 and 1, where 1 indicates a perfect correlation and 0 a poor correlation. In general, a CORA score above 0.44 indicates “fair” biofidelity, and a score greater than 0.68 is considered “good” biofidelity. The default CORA parameters were used. The presented scores are calculated as the average of each X-, Y-, and Z-component score.
Results
Generated HBMs
Personalization accuracy in terms of Dice scores and HD95 distances are presented in for baseline male and female pedestrian SAFER HBMs and the 3 personalized HBMs. The average Dice score was 0.93 and the average HD95 distance was 16.0 mm. To provide an indication of the element quality after morphing, the element minimum Jacobian for each generated model is included, showing that the minimum Jacobian was greater than 0.10 for all HBMs.
Table 2. Minimum Jacobians, Dice values, and HD95 distances for the baseline HBMs and personalized HBMs (PMHSs).
For further illustration of the morphing accuracy, the model-to-model distance of the generated baseline models is presented in . The model-to-model distance is the computed absolute HD between the target models and morphed models at each point.
Trajectories and kinematics
The trajectories of the simulated impact and the corresponding experimental impacts are presented in . The 2-dimensional trajectories are presented relative to the buck model. The component-wise trajectories of the head, vertebrae, pelvis, femurs, and tibias are presented in the Appendix (Figures A5–A13, see online supplement). The average CORA ratings for these trajectories were above 0.78 for the 3 subjects, indicating a good correspondence with PMHS experiments. One key difference between the trajectories of the PMHS and the personalized HBMs was found in the pelvis impact sequence. For the HBMs in the simulation, the pelvis went over the hood and lost contact with it, but for the PMHS models, the contact between the pelvis and hood was continuous. This is seen in , where the impact sequence is shown in a posterior view of the HBM and PMHS in a series of time steps. The pelvis movement is also highlighted by the corresponding trajectory (see , pelvis around X = 500 mm). Snapshots of pedestrian impact sequences for subjects V2370 and V2371 are available in the Appendix (see Figures A1–A3, online supplement).
Figure 5. Trajectories of the personalized HBMs in lateral impacts: Subject V2370 (top), subject V2371 (middle), and subject V2374 (bottom). Markers are displayed for every 30 ms from the point of leg contact (t = 0).
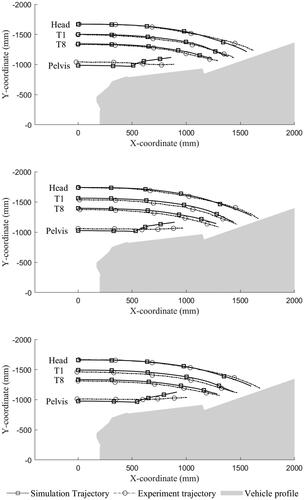
Figure 6. Posterior view of V2374 in selected time steps. Images of PMHS experiments are taken from a previous publication by Wu et al. (Citation2017).
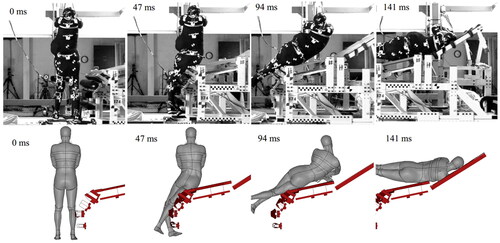
In all 3 impact tests, the subject-specific HBMs showed a delay in the impact sequence, with the final head impact occurring later compared to the experiments. The head contact occurred between 3 and 10 ms later compared to the experiments. The wrap-around-distance (WAD) was not less than 23 mm away from the experimentally derived WAD in all 3 impacts. The simulated head contact time and WAD for each impact test are presented in .
Table 3. Head contact times, WADs, and simulated strain in the skull bone.
The maximum principal strains measured in the skull bone are presented in . A threshold of 1.50% strain has been suggested for cortical bone fracture (Yasuki and Yamamae Citation2010; Golman et al. Citation2014). All 3 head impact simulations resulted in maximum strains below the proposed threshold, meaning that no skull fractures were predicted from the simulation, consistent with the 3 experimental PMHS tests that showed no skull fractures of the PMHS post impact.
The head CoG angular velocity and linear acceleration time histories are presented jointly with corresponding CORA ratings in . The average CORA ratings for the angular velocity and linear acceleration were 0.70 and 0.63, respectively. Resultant forces from the lower and upper bumper, grille, and hood of the buck model showed good correlation with the PMHS experiments. Force–time histories are included in the Appendix (see Figure A4, online supplement).
Discussion
In this study, 50th percentile male and female pedestrian SAFER HBMs were established using state-of-the-art image registration–based morphing techniques and positioning tools. In conjunction with the established baseline HBMs, an HBM personalization framework was introduced. The presented framework allows for rapid and landmark-free generation of subject-specific HBMs and accounts for the body size and shape as well as the skeletal morphology. The baseline HBMs together with the personalization framework were evaluated in simulated PMHS impact tests, where the model’s ability to capture impact responses in pedestrian-to-vehicle collisions was demonstrated. The baseline HBMs and personalization framework can be used to study head injury mechanisms in reconstructed pedestrian-to-vehicle collisions, among many other applications.
Validity of generated pedestrian HBM mesh
Two baseline 50th percentile HBMs were established by morphing a template HBM to a target geometry, without accounting for any other biological differences apart from the human anthropometry. Yet, human attributes have been argued to have significant effects on the material properties (Hwang et al. Citation2016). Such variations among populations were neglected in this study, yet judging by the CORA ratings, the HBMs seem to exhibit satisfactory biofidelity in the lateral impacts investigated in this study. This is in line with the findings of Hwang et al. (Citation2016), who found that the geometry and posture of the HBM have larger effects than the material properties of the human bone and soft tissues when analyzing occupant impact responses. The morphing pipeline was able to ensure the skin and skeleton surface geometries of the personalized HBM, and the mass distribution was accounted for via tuning the fat density, which overall contributed to the good prediction on overall trajectory and head kinematics.
The 2 baseline models are based on a seated occupant HBM that was positioned into an upright stance using FE simulation. The subsequent morphing procedure only takes the body shape and skeletal morphology into account. During the process of establishing the baseline pedestrian HBMs, deformations of the internal parts of the HBM were introduced conjointly with the positioning and applied displacement fields. Previous studies have found significant differences in abdominal organ position and shape between supine and seated postures (Beillas et al. Citation2009). With this in mind, the positioning from a seated to an upright stance might introduce unintended errors from the displaced positions, morphed volumes, and changed mass distributions of the inner organs. A qualitative check was performed after morphing and the inner organs remained intact in mesh quality, and the shape was not heavily distorted compared to the original occupant HBM. New intersections in the contact definitions were not detected. Most important, the validation presented in this study show that the biofidelity was preserved after the positioning and morphing procedures. Though further validation might be needed, the results of this study indicate good preservation of the biofidelity of the HBM, despite any possible mesh changes of the internal organs.
As indicated by the Dice and HD95 values, the female baseline HBM was not as close to its target shape as the male baseline HBM. The Dice value was lower and HD95 was higher for the female baseline model compared to the male HBM. This is explained by the obvious similarities between the 50th percentile male template and the target 50th percentile male. Very little displacement of the mesh was needed to morph the male template HBM to match the target geometry. The female target image, on the other hand, differs significantly in both size and shape compared to the template 50th percentile male. Thus, more distortion of the mesh is needed, resulting in less morphing accuracy. As seen by , the absolute error of the female baseline model is concentrated at the soft tissues that differ the most from the male HBM; that is, chest region, lower abdomen, and rear end.
Personalization framework
The SAFER HBM sustained significant deformation during positioning due to the leg rotations around the pelvis when transforming a sitting occupant model to a standing upright posture. This resulted in some mesh distortions that were manually repaired. Any part intersections or negative Jacobians introduced by the positioning were manually postprocessed and mesh quality was restored. The morphing procedure introduced no new intersections or negative Jacobians, which indicates the robustness of the proposed image registration–based personalization tool. Once the baseline male and female HBMs were established, subject-specific HBMs were generated with the baseline HBMs as template models. No postprocessing was necessary after the personalization procedure. This means that generating subject-specific HBMs based on the established baseline HBMs requires minimal effort and little pre- and postprocessing time.
The HBM personalization procedure described in this study differs from previously proposed personalization techniques. Many studies prior to this have introduced nonrigid deformation techniques to adapt the geometry of HBMs (Hwang et al. Citation2016; Poulard et al. Citation2016; Chen et al. Citation2018; Larsson et al. Citation2022). The presented personalization by morphing approaches have often been limited to isolated body parts; for example, the femur (Klein et al. Citation2015), pelvis (Salo et al. Citation2015), or head (Li Citation2021). Proposed morphing techniques for HBMs seldom consider both skeletal and outer shape morphology simultaneously. For instance, Chen et al. (Citation2018) used a morphing method using the THUMS HBM that did not account for the external surface of the HBM. They developed subject-specific HBMs by morphing an existing HBM to computed tomography (CT) and anthropometric measurements, following a so-called dual kriging interpolation process (Poulard et al. Citation2016) using 144 control points constructed from CT scans and anthropometric measurements. In contrast to the personalization framework presented in this study, this kriging interpolation process is very reliant on geometry data input (CT or anthropometric measurements of subject). The personalization method presented in this study merely requires height, weight, and gender as inputs. The current proposed morphing framework requires minimal information about the subject anthropometry apart from the subject’s height and weight. Any additional information, such as the subject’s age or additional CT scans, can, however, be used to increase the HBM personalization accuracy even further.
In this study, a method of mesh morphing is proposed that introduces very few practical limitations and has been shown to be useful for many applications. For instance, the method works well for generating subject-specific anatomically detailed head models (Li Citation2021). The same image registration–based morphing procedure can be used to morph other existing FE HBMs as well, as demonstrated in previous publications (Li et al. Citation2023).
Validation
This study focused on the development of baseline SAFER HBM pedestrians empowered by the accompanied personalization framework. To show the robustness of the morphed models in crash simulations, we reconstructed 3 PMHS experimental impact tests. To demonstrate the personalization framework, the trajectories of the HBMs were evaluated by means of individual responses instead of the proposed normalized PMHS responses. Three specific experiment scenarios were reconstructed, and the simulated trajectories of the HBMs exhibited very good agreement with PMHSs, with nearly identical trajectories for each location in the Y–Z plane. The CORA scores of the kinematic time histories indicate fair agreement between experimental and simulated time histories, demonstrating that the HBM was capable of mimicking the response of a human head in lateral impacts. However, there is room for improvement, as discussed below.
First, the accuracy of the reconstructed PMHS experiments could be further improved by using more representative template body models. In this study, a morphing template image was chosen based on the height, weight, age, and sex of each PMHS. The generated template image is thus a generic representative of a human body with the wanted height, weight, age, and sex. Of course, this will not be an exact representation of the PMHS, due to the many possible subject-to-subject variations. With knowledge of the PMHS measurements, even better target geometry can be generated. With a perfect target model, the distance between the head, T1 vertebra, T8 vertebra, and pelvis could be much more accurate. As seen in the simulated trajectories, these landmarks, especially the pelvis, do not perfectly coincide with the PMHS. Achieving better accuracy is feasible using the presented image registration–based morphing framework.
Secondly, the accuracy of the reconstructed PMHS experiments can be further improved by more accurate positioning of the HBMs. Under the assumption that the arms have little effect on the impact kinematics, the arms of the 3 subject-specific PMHSs were uniformly placed in front of the body. The arms and shoulders could have been positioned with better accuracy, and the shrugged posture seen in the PMHS due to the support of the cadavers in the experiments could have been captured. Furthermore, one PMHS (V2374) was missing an arm, which was not accounted for by the subject-specific HBM. Improving the HBM-to-PMHS similarity would most likely increase the agreement between the simulations and experiments. It should nonetheless be mentioned that positioning of the HBMs using the simulation-based marionette method is a time-consuming process, especially regarding fine-tuning of landmark positions.
Lastly, there might be inherent errors from the FE buck model used. The buck FE model has previously been validated in regard to car–pedestrian interactions (Takahashi et al. Citation2014). In general, the buck FE model has shown good correspondence with the mechanical buck. However, some discrepancies have been found in the hood edge reaction force (Takahashi et al. Citation2014). Nevertheless, the extracted forces from the buck joints exhibited good agreement with PMHS experiments. Evaluating the validity of the buck FE model is, however, beyond the scope of this study.
The simulated trajectory of the pelvis showed less similarity to the PMHS experiment. This might be explained by the extra weight of the experimental equipment, which was accounted for in the simulations by distributing the extra weight (7.4 kg) equally over the HBM body parts. In the PMHS experiments, cables and equipment were put in a bag attached to the lower back of the PMHS. The mass distribution of the equipment will influence the kinematics of the PMHS and, therefore, in the HBM validation, concentrating the mass of the cables to the lower back/buttocks can improve the agreement between model predictions and test results, especially for the pelvis.
A related limitation of the mass tuning is that the displacement field generated by the demons registration in this study was essentially dependent on the outer skin shape and skeleton outer surface shape. Because the adjacent flesh, soft tissues, and organs were assigned the same voxel values, this means that they were all treated as homogenous. This can pose an issue because the method does not consider variations in relative thickness between the fat layer and muscle layer. Tuning the fat density to obtain target body mass can thus lead to unrealistic fat properties. Yet, the current personalized models have a fat density on the same order as that of the originally set density. The original fat density of the SAFER HBM was set to 1,120 kg/m3. Subject V2374 needed no density scaling, and for V2370 and V2372, the density was scaled down to 870 and 1,080 kg/m3, respectively. The scaled densities were in fact even closer to the reported density of adipose tissue (920 kg/m3; Farvid et al. Citation2005).
The impact response of the HBMs was delayed compared to the PMHS experiments. Wu et al. (Citation2017), who performed the same validation simulations using scaled THUMS HBMs, observed the same phenomenon. The SAFER HBM is originally based on the THUMS v3, which could be a reason for the similar impact response discrepancy. Wu et al. (Citation2017) hypothesized that the delay could be due to a softer neck in terms of lateral bending stiffness of the THUMS model compared to the PMHS. This could be the case for the SAFER HBM as well because the response of the thoracic spine appears to be more similar in respect of timing to the PMHS experiments for subjects V2371 and V2374.
Because this study focused on head kinematics, future regional validation of the lower body and extremities is necessary. This would involve following validation protocols as demonstrated by Grindle et al. (Citation2021) and Untaroiu et al. (Citation2015), who validated pedestrian HBMs in lower extremity, shoulder, and upper body bending and blunt impacts and in shearing and bending impacts for pedestrian knee joints.
The importance of personalization
In this study, we did not explicitly evaluate whether subject-specific morphing increases the biofidelity of pedestrian impact kinematics. However, a very important question is: is geometrical personalization really necessary? In 2016, Poulard et al. attempted to answer this question by performing PMHS simulations using one generic HBM, one globally scaled HBM, and one personalized (morphed) HBM. In the study, the generic HBM was represented by a 50th percentile male THUMS HBM. Globally scaled HBMs were obtained by applying a global scaling factor to match the height of the PMHS and a second factor to scale the HBM in the transverse plane to match the PMHS’s weight. The morphed HBM was obtained using the dual kriging interpolation process (Trochu Citation1993). The simulations were set up to correspond to PMHS experiments, where 2 obese male PMHS were struck laterally by a mid-sized sedan traveling at 40 km/h, which is very similar to the validation experiments used in the present study. When comparing the simulations against the cadaver experiments, Poulard et al. (Citation2016) could clearly demonstrate how the subject-specific HBMs obtained by morphing produced the best correlation to the cadaver experiments. The morphed HBMs resulted in better prediction of the WAD, impact locations, and timing. The CORA scores for the generic, globally scaled, and morphed models were 0.85, 0.92, and 0.95, respectively, serving as a clear indicator of an increase in biofidelity when using subject-specific HBMs. Similar conclusions were drawn in previous studies by Piqueras-Lorente et al. (Citation2018), Larsson et al. (Citation2019, Citation2022), and Hwang et al. (Citation2016) using occupant HBMs.
Supplemental Material
Download Zip (2.2 MB)Disclosure statement
No potential conflict of interest was reported by the authors.
Data availability statement
All data relevant to the study are included in the article or uploaded as supplementary information.
Additional information
Funding
References
- Adam A. 2023. Mesh voxelisation. https://www.mathworks.com/matlabcentral/fileexchange/27390-mesh-voxelisation
- Ameratunga S, Hijar M, Norton R. 2006. Road-traffic injuries: confronting disparities to address a global-health problem. Lancet. 367(9521):1533–1540. doi:10.1016/S0140-6736(06)68654-6.
- Beillas P, Lafon Y, Smith FW. 2009. The effects of posture and subject-to-subject variations on the position, shape and volume of abdominal and thoracic organs. Stapp Car Crash J. 53:127–154. doi:10.4271/2009-22-0005.
- Blender LTS. 2022. Version 3.4 [Computer software]. Amsterdam (Netherlands): The Blender Foundation.
- Chen H, Poulard D, Forman J, Crandall J, Panzer MB. 2018. Evaluation of geometrically personalized THUMS pedestrian model response against sedan–pedestrian PMHS impact test data. Traffic Inj Prev. 19(5):542–548. doi:10.1080/15389588.2018.1450979.
- Costa C, Aira J, Koya B, Decker W, Sink J, Withers S, Beal R, Schieffer S, Gayzik S, Stitzel J, et al. 2020. Finite element reconstruction of a vehicle-to-pedestrian impact. Traffic Inj Prev. 21(sup1):S145–S147. doi:10.1080/15389588.2020.1829911.
- Crandall JR, Bhalla KS, Madeley NJ. 2002. Designing road vehicles for pedestrian protection. BMJ. 324(7346):1145–1148. doi:10.1136/bmj.324.7346.1145.
- Crandall JR, Bose D, Forman J, Untaroiu CD, Arregui-Dalmases C, Shaw CG, Kerrigan JR. 2011. Human surrogates for injury biomechanics research. Clin Anat. 24(3):362–371. doi:10.1002/ca.21152.
- 3D Slicer. 2021. Version 4.10.2 [Computer software]. Slicer Community.
- Danelson KA, Stitzel JD. 2015. Finite element model prediction of pulmonary contusion in vehicle-to-vehicle simulations of real-world crashes. Traffic Inj Prev. 16(6):627–636. doi:10.1080/15389588.2014.995266.
- Davis ML, Koya B, Schap JM, Gayzik FS. 2016. Development and full body validation of a 5th percentile female finite element model. Stapp Car Crash J. 60:509–544. doi:10.1080/15389588.2014.995266.
- Decker W, Koya B, Pak W, Untaroiu CD, Gayzik FS. 2019. Evaluation of finite element human body models for use in a standardized protocol for pedestrian safety assessment. Traffic Inj Prev. 20(sup2):S32–S36. doi:10.1080/15389588.2019.1637518.
- Evans L. 2001. Female compared with male fatality risk from similar physical impacts. J Trauma. 50(2):281–288. doi:10.1097/00005373-200102000-00014.
- Evans L, Gerrish PH. 2001. Gender and age influence on fatality risk from the same physical impact determined using two-car crashes. Paper presented at: Proceedings of SAE 2001 World Congress Detroit, Michigan. doi:10.4271/2001-01-1174.
- Farvid MS, Ng TWK, Chan DC, Barrett PHR, Watts GF. 2005. Association between adipose tissue density and cardiovascular risk factors in the rancho bernardo study. Diabetes Obes Metab. 7(4):406–413. doi:10.1111/j.1463-1326.2004.00410.x.
- Forman JL, Joodaki H, Forghani A, Riley P, Bollapragada V, Lessley D, Overby B, Heltzel S, Crandall J. 2015. Biofidelity corridors for whole-body pedestrian impact with a generic buck. 2015. Paper presented at: Proceedings of the 2015 IRCOBI Conference, Malaga, Spain.
- Forman JL, Joodaki H, Forghani A, Riley PO, Bollapragada V, Lessley DJ, Overby B, Heltzel S, Kerrigan JR, Crandall JR, et al. 2015. Whole-body response for pedestrian impact with a generic sedan buck. Stapp Car Crash J. 59:401–444. doi:10.4271/2015-22-0016.
- Golman AJ, Danelson KA, Miller LE, Stitzel JD. 2014. Injury prediction in a side impact crash using human body model simulation. Accid Anal Prev. 64:1–8. doi:10.1016/j.aap.2013.10.026.
- Grindle D, Pak W, Guleyupoglu B, Koya B, Gayzik FS, Song E, Untaroiu C. 2021. A detailed finite element model of a mid-sized male for the investigation of traffic pedestrian accidents. Proc Inst Mech Eng H. 235(3):300–313. doi:10.1177/0954411920976223.
- Hwang E, Hu J, Chen C, Klein KF, Miller CS, Reed MP, Rupp JD, Hallman JJ. 2016. Development, evaluation, and sensitivity analysis of parametric finite element whole-body human models in side impacts. Stapp Car Crash J. 60:473–508. doi:10.4271/2016-22-0014.
- Iwamoto M, Kisanuki Y, Watanabe I, Furusu K, Miki K. 2002. Development of a finite element model of the total human model for safety (THUMS) and application to injury reconstruction. Paper presented at: Proceedings of the 2002 IRCOBI Conference, Münich, Germany.
- John J, Klug C, Kranjec M, Svenning E, Iraeus J. 2022. Hello, world! VIVA+: a human body model lineup to evaluate sex-differences in crash protection. Front Bioeng Biotechnol. 10:918904. doi:10.3389/fbioe.2022.918904.
- Johnson H, Zhao Y. 2009. BRAINSDemonWarp: an applicaton to perform demons registration. The Insight J. 2009 January. doi:10.54294/ib7or9.
- Klein KF, Hu J, Reed MP, Hoff CN, Rupp JD. 2015. Development and validation of statistical models of femur geometry for use with parametric finite element models. Ann Biomed Eng. 43(10):2503–2514. doi:10.1007/s10439-015-1307-6.
- Kleiven S. 2007. Predictors for traumatic brain injuries evaluated through accident reconstructions. Stapp Car Crash J. 51:81–114. doi:10.4271/2007-22-0003.
- Larsson KJ, Pipkorn B, Iraeus J, Bolte JH, Agnew AM, Hu J, Reed MP, Sunnevång C. 2019. Evaluation of the benefits of parametric human body model morphing for prediction of injury to elderly occupants in side impact. Paper presented at: Proceedings of the 2019 IRCOBI Conference, Florence, Italy.
- Larsson KJ, Pipkorn B, Iraeus J, Forman J, Hu J. 2022. Evaluation of a diverse population of morphed human body models for prediction of vehicle occupant crash kinematics. Comput Methods Biomech Biomed Engin. 25(10):1125–1155. doi:10.1080/10255842.2021.2003790.
- Li X. 2021. Subject-specific head model generation by mesh morphing: a personalization framework and its applications. Front Bioeng Biotechnol. 9:706566. doi:10.3389/fbioe.2021.706566.
- Li X, Yuan Q, Lindgren N, Huang Q, Fahlstedt M, Östh J, Pipkorn B, Jakobsson L, Kleiven S. 2023. Personalization of human body models and beyond via image registration. Front Bioeng Biotechnol. 11:1169365. doi:10.3389/fbioe.2023.1169365.
- Li X, Zhou Z, Kleiven S. 2021. An anatomically detailed and personalizable head injury model: significance of brain and white matter tract morphological variability on strain. Biomech Model Mechanobiol. 20(2):403–431. doi:10.1007/s10237-020-01391-8.
- LS-DYNA. 2021. Version 13.0.0 [Computer software]. Canonsburg (PA): ANSYS, Inc.
- LS-PrePost. 2020. Version 4.8.24 [Computer software]. Canonsburg (PA): ANSYS, Inc.
- Matlab. 2021. Version R2021b [Computer software]. Natick (MA): The MathWorks, Inc.
- Matsui Y, Wittek A, Konosu A. 2002. Comparison of pedestrian subsystem safety tests using impactors and full-scale dummy tests. Paper presented at: Proceedings of SAE 2002 World Congress Detroit, Michigan. doi:10.1145/2816795.2818013.
- NHTSA. 2021. Traffic safety facts, research note, comparing demographic trends in vulnerable road user fatalities and the U.S. population, 1980–2019.
- [OECD] Organisation for Economic Co-operation and Development. 1998. Scientific expert group on the safety of vulnerable road users (RS7).
- Östh J, Brolin K, Bråse D. 2015. A human body model with active muscles for simulation of pretensioned restraints in autonomous braking interventions. Traffic Inj Prev. 16(3):304–313. doi:10.1080/15389588.2014.931949.
- Östh J, Pipkorn B, Forsberg J, Iraeus J. 2021. Numerical reproducibility of human body model crash simulations. Proc 2021 IRCOBI Conf Online Event. 2021:431–443. doi:10.1080/15389588.2014.931949.
- Ou Y, Akbari H, Bilello M, Da X, Davatzikos C. 2014. Comparative evaluation of registration algorithms in different brain databases with varying difficulty: results and insights. IEEE Trans Med Imaging. 33(10):2039–2065. doi:10.1109/TMI.2014.2330355.
- Pipkorn B, Forsberg C, Takahashi Y, Ikeda M, Fredriksson R, Svensson C, Thesleff A. 2014. Development and component validation of a generic vehicle front buck for pedestrian impact evaluation. Paper presented at: Proceedings of the 2014 IRCOBI Conference, Berlin, Germany.
- Pipkorn B, Östh J, Brynskog E, Larsson E, Rydqvist L, Iraeus J, Perez-Rapela D, Jakobsson L. 2021. Validation of the SAFER human body model kinematics in far-side impacts. Paper presented at: Proceedings of the 2021 IRCOBI conference, Online event.
- Piqueras-Lorente A, Iraeus J, Lorente AI, López-Valdés FJ, Juste-Lorente Ó, Maza-Frechín M, Pipkorn B. 2018. Kinematic assessment of subject personification of human body models (THUMS). Paper presented at: Proceedings of the 2018 IRCOBI Conference, Athens, Greece.
- Poulard D, Chen H, Panzer M. 2016. Geometrical personalization of pedestrian finite element models using morphing increases the biofidelity of their impact kinematics. SAE Technical Paper. doi:10.4271/2016-01-1506.
- Poulard D, Subit D, Donlon JP, Kent RW. 2015. Development of a computational framework to adjust the pre-impact spine posture of a whole-body model based on cadaver tests data. J Biomech. 48(4):636–643. doi:10.1016/j.jbiomech.2014.12.050.
- PRIMER. 2021. Version 18.1 [Computer software]. San Francisco (CA): Oasys Ltd.
- Ptak M, Rusiński E, Karliński J, Dragan S. 2012. Evaluation of kinematics of SUV to pedestrian impact-lower leg impactor and dummy approach. Arch Civ Mech Eng. 12(1):68–73. doi:10.1016/j.acme.2012.03.016.
- Salo Z, Beek M, Wright D, Marisa Whyne C. 2015. Computed tomography landmark-based semi-automated mesh morphing and mapping techniques: generation of patient specific models of the human pelvis without segmentation. J Biomech. 48(6):1125–1132. doi:10.1016/j.jbiomech.2015.01.013.
- Schneider L, Robbins D, Pflüg M, Snyder R. 1983. Development of anthropometrically based design specification for an advanced adult anthropomorphic dummy family. U.S. Department of Transportation, National Highway Traffic Safety Administration NHTSA, Washington, D.C.
- Schwarz LA. 2007. Non-rigid Registration Using Free-form Deformations. Diploma Thesis in computer Science, Technische Universität München Fakultät für Informatik.
- Takahashi Y, Ikeda M, Asanuma H, Svensson C, Pipkorn B, Forsberg C, Fredriksson R. 2014. Full-scale validation of a generic buck for pedestrian impact. Paper presented at: Proceedings of the 2014 IRCOBI Conference, Berlin, Germany.
- Trochu F. 1993. A contouring program based on dual kriging interpolation. Eng Comput. 9(3):160–177. doi:10.1007/BF01206346.
- Ulfarsson GF, Mannering FL. 2004. Differences in male and female injury severities in sport-utility vehicle, minivan, pickup and passenger car accidents. Accid Anal Prev. 36(2):135–147. doi:10.1016/S0001-4575(02)00135-5.
- Untaroiu CD, Putnam JB, Schap J, Davis ML, Gayzik FS. 2015. Development and preliminary validation of a 50th percentile pedestrian finite element model. Paper presented at: Proceedings of the ASME 2015 International Design Engineering Technical Conferences & Computers and Information in Engineering Conference, Boston, Massachusetts, USA. doi:10.1115/DETC2015-47781.
- [WHO] World Health Organization. 2018. Global Status Report on Road Safety 2018: summary. World Health Organization, Geneva.
- Wu T, Kim T, Bollapragada V, Poulard D, Chen H, Panzer MB, Forman JL, Crandall JR, Pipkorn B. 2017. Evaluation of biofidelity of THUMS pedestrian model under a whole-body impact conditions with a generic sedan buck. Traffic Inj Prev. 18(sup1):S148–S154. doi:10.1080/15389588.2017.1318435.
- Yasuki T, Yamamae Y. 2010. Validation of kinematics and lower extremity injuries estimated by Total Human Model for Safety in SUV to pedestrian impact test. JBSE. 5(4):340–356. doi:10.1299/jbse.5.340.