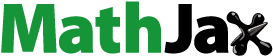
ABSTRACT
A tutorial introduction to the statistical mechanics of phase transitions and phase coexistence is presented, starting out from equilibrium systems and nonequilibrium steady-state situations in externally driven systems, such as unmixing of sheared binary fluid mixtures, the driven lattice gas model, and the onset of Rayleigh-Bénard convection. Then, some models for phase separation in models for active systems, where particles possess internal motility, are discussed, emphasizing what one can learn by extending analysis methods to study phase transitions in equilibrium systems by computer simulations to active systems. Specific examples will include colloid-polymer mixtures where the colloids are assumed to be active particles, and active Brownian particles. The extent to which concepts familiar from the study of equilibrium systems are still useful will be critically discussed.
Introduction: Phase Transition in Equilibrium
Active matter has become a hot topic of research in recent years, [Citation1] and many phenomena have been found which are reminiscent of phase transitions that are familiar from systems in thermal equilibrium (e.g. [Citation2–18]). Both experiment (e.g. [Citation6]) and simulation studies of various models (e.g. [Citation4,Citation7,Citation8,Citation10,Citation12,Citation13,Citation15]) have revealed cases of coexistence between different states of active matter, resembling coexistence between different phases in thermodynamic equilibrium. Is this an accidental analogy, or does there exist a deep connection? Similarly, cases occur where the difference in properties between coexisting states of active matter gradually vanishes, when some control parameter of the active system is varied. Such cases typically involve the observation of large (and slow) fluctuations (e.g. [Citation2,Citation10,Citation12]) reminiscent of critical fluctuations associated with continuous-phase transitions in thermal equilibrium. This analogy is particularly intriguing because it is known that such critical behavior is universal for broad classes of systems: this means that the ”critical exponents” [Citation19–25] and certain dimensionless combinations of prefactors in the power laws that describe the critical singularities (the “critical amplitudes”) are universal constants, for all systems belonging to such a “universality class.”[Citation23–26] E.g., the critical behavior of anisotropic magnets (and corresponding theoretical models, such as the Ising model), the vapor-liquid critical behavior and unmixing of binary fluids all belong (with respect to their static properties near criticality) to the same class, and details about the interactions between the Ising spins or the fluid molecules are irrelevant for the universal properties.[Citation19–26] E.g., experimental evidence for fluids near the vapor-liquid critical point and binary (AB) liquids near the critical mixing point is reviewed in .[Citation27]
Within the framework of equilibrium statistical mechanics, the renormalization group theory [Citation20,Citation22,Citation23] has provided general concepts to understand why universal behavior occurs, and to explain which universality classes exist [Citation20,Citation22,Citation23] and identify the parameters that distinguish them: dimensionality (d) of space the system is in; dimensionality (n) of the order parameter that characterizes spontaneous breaking of symmetry associated with the transition from the disordered phase (at temperatures higher than the critical temperature
, in case of a thermally driven phase transition) to the ordered phase (at
). Finally, also, the range of the interactions between the elementary degrees of freedom are also involved in the ordering matters: all systems with finite range define one class, while for systems with power-law decay of interactions, different classes may arise.[Citation24] (Effects due to quenched disorder [Citation28,Citation29,Citation30] are disregarded here and also quantum transitions are out of our focus).
This general theoretical framework does not provide information on the nonuniversal properties (such as the location of the critical temperature in relation to the detailed interactions between the elementary degrees of freedom, the values of the critical amplitudes, and the behavior away from the immediate vicinity of the critical point). However, equilibrium statistical mechanics also provides a sound basis for methodologies applied in the context of Monte Carlo and Molecular Dynamics simulations [Citation31–34]of model systems, which are suitable to obtain accurate information on all these nonuniversal properties associated with critical behavior and yield in favorable cases also very precise estimates of the universal properties as well.[Citation24,Citation25] Moreover, such computer simulations are also valuable tools to study coexistence between the different phases of a system, associated with first-order phase transitions, [Citation35] including the properties of interfaces separating such coexisting phases.[Citation36,Citation37]
While, so far, only static properties of systems in thermal equilibrium have been addressed here, it is important to recall that also the dynamics of the (small) thermal fluctuations that occur spontaneously in equilibrium can be addressed similarly. However, there is one important distinction: while with respect to equilibrium properties, in the thermodynamic limit when the number of the elementary degrees of freedom tends to infinity, the different ensembles of statistical mechanics are strictly equivalent, this is not the case with respect to the dynamics of long-wavelength fluctuations. For instance, in the microcanonical ensemble of a system with a considered volume
both internal energy
and particle number
are strictly constant, i.e. “conserved.” Such conservation laws imply continuity equations for the corresponding densities and gradients. In the canonical NVT ensemble, however, the internal energy is not conserved, and in the grand-canonical
ensemble where the chemical potential
is prescribed and the particle number
may fluctuate, no conservation laws apply. As we shall see later, for the study of static properties of equilibrium systems the freedom in the choice of statistical ensemble is very convenient and useful for the application of computer simulation tools to study phase transitions.[Citation32] No such freedom occurs for systems far from thermal equilibrium, since no analog of concepts such as thermodynamic potentials and Legendre transformations between them has yet been established.
An interesting aspect of the dynamics of fluctuations in equilibrium states near critical points is also that one static universality class splits into several universality classes with respect to dynamic critical behavior.[Citation38,Citation39] This is not only due to the conservation laws but also derives from the need to consider the appropriate couplings between the coarse-grained variables needed to describe the dynamics of fluctuations, namely the so-called “Poisson-bracket relations” between the order parameter and the conserved densities. For example, a binary (AB) fluid that undergoes an unmixing transition is considered. There are six conserved densities: the energy density, the mass densities for both components, and the three components of the momentum density vector. Small long-wavelength deviations of these quantities from equilibrium are described by linearized hydrodynamics.[Citation40] The solution of the corresponding hydrodynamic equations yields five modes (four diffusive modes with relaxation rates proportional to where
is the wavenumber of the mode, and a propagating sound wave (with complex frequency due to sound damping)). The Poisson bracket relation between the total momentum and other densities in the system then implies coupling of modes, which is then crucial for a correct description of the singular behavior of transport coefficients in the system.[Citation38,Citation39,Citation41] An important feature of fluctuations in equilibrium states is that the correlation function between a fluctuation
of a quantity
and another quantity
,
, does not depend on the two times
,
separately, but only on their difference
(this is called “time translation invariance”).
Kinetics of Phase Changes
One obvious extension of the consideration of the dynamics of fluctuations in equilibrium is the kinetics of phase transitions, where one changes a control parameter of the system (e.g. temperature , or magnetic field
, in the case of a ferromagnet) by a finite amount, such that in the phase diagram of the system, a phase boundary is crossed. For example, performing a (instantaneous) quench of temperature in a one-component fluid (or a binary fluid mixture, respectively) such that the final state is inside the two-phase coexistence region, while the initial state was in thermal equilibrium in the (macroscopically homogeneous) one-phase region, a transient non-equilibrium relaxation process occurs, where at intermediate stages of the process, the system is far from equilibrium.[Citation39,Citation42] When the final state point is only slightly inside the coexistence curve that describes the final macroscopic equilibrium phases, that coexist at this temperature in the thermodynamic limit, the transition starts by nucleation and growth of mesoscopic domains of the minority phase.[Citation35,Citation43–46] However, both a precise understanding of the lifetime of metastable states (that is limited by such nucleation processes) and of the nature of the saddle point in phase space that is crossed in a nucleation event are still incompletely understood. Further problems concern deeper quenches, when the nucleation barrier due to this saddle point is only of the order of a few times the thermal energy, and a gradual transition to spinodal decomposition (i.e., spontaneous growth of long wave density or concentration fluctuations[Citation39,Citation42,Citation47,Citation48]) takes place. While for systems with long-range forces that are described by mean field theory this transition occurs at a well-defined spinodal curve, [Citation49] for systems with short range forces the concept of a spinodal lacks precise meaning.[Citation35,Citation48,Citation50] However, it is worth remarking that often for the description of the late stages of such phase transition processes the concept of local equilibrium is useful. For example, when after the quench into the two-phase region of a mixture, many nuclei of the minority phase have been formed and grown, so that the supersaturation of the binary mixture has strongly decreased and no more new nuclei can be formed at an observable rate, there is a transient equilibrium between the population of (spherical) domains and the supersaturated background phase: the radius of a domain of the average size is related to the supersaturation of the background with a Kelvin-like relation. This is not a stable equilibrium, since domains larger than the average size will grow while domains smaller than the average size will shrink and ultimately disappear, and thus also the average droplet size grows and the associated supersaturation diminishes. As the time t passed after the quench tends to infinity, also the domain size according to this Lifshitz-Slyozov [Citation51] mechanism becomes macroscopic, and true thermal equilibrium is ultimately reached. Irrespective of whether phase separation starts via nucleation processes or spinodal decomposition, characteristic linear dimensions at late stages grow according to power laws with time.[Citation39,Citation42] Of course, depending on the system that is considered (and the dynamical laws that govern the time evolution of the degrees of freedom of interest), many different kinetic processes associated with phase changes from one equilibrium state to another can be considered;[Citation39,Citation42,Citation47] while analogous processes occur also in active systems (e.g. [Citation52]), we shall not consider such processes here further. Such transient processes are particularly difficult to describe exhaustively, since correlations between dynamical variables at time t and
do not only depend on the time difference
(as in full thermal equilibrium) but also on the time t passed after the instantaneous change of the considered control variable.
Phase Transitions in Externally Driven Systems
Here, we shall rather focus on systems that are far from equilibrium but in a steady state that does not change. Considering again fluid binary mixtures, a problem of great importance in materials sciences is phase separation under steady state shear flow.[Citation39] Is there an extension of the concepts from equilibrium statistical mechanics to such systems? The simplest case to consider is a flow profile of the form , i.e. the flow direction is taken to be along the x-axis,
denotes the unit vector along the x-axis. The velocity field varies in the y-direction (i.e., the direction of the shear gradient), while the z-axis denotes the so-called vorticity direction. The shear rate
characterizes the strength of the resulting deformation of the system. The concentration fluctuations, which in a system without shear would on average be isotropic in space, become now very anisotropic: this deformation is controlled by the so-called Deborah number
with
being the characteristic time of the critical fluctuations in equilibrium, when the correlation length is
, and
.[Citation38,Citation39] While in the absence of shear, the starting point for the description of critical dynamics is a Langevin equation known as “model
”, [Citation38]
where is the order parameter field,
the velocity field,
a kinetic coefficient, and
a random force (satisfying the fluctuation-dissipation theorem). In the presence of shear, a term
needs to be added. While in mean field theory (without shear) the static structure factor is (
is the wavenumber,
is the distance from criticality in the phase diagram, for a state point in the one-phase region)
in the presence of shear, the isotropy in reciprocal space, which applies to this well-known Ornstein-Zernike result, gets replaced by an anisotropic and singular expression [Citation53]
where is a constant. A consequence of this result is that the “upper critical dimensionality”
(for
mean field critical exponents apply) gets lowered from the standard result [Citation20–23]
to a value
. In addition, a nontrivial shift of the critical point due to the shear occurs.
EquationEquations (1(1)
(1) –Equation3
(3)
(3) ) already illustrate the generic features common to many nonequilibrium phase transitions: one must base the treatment on a description of the dynamics of the corresponding equilibrium system (EquationEquation 1
(1)
(1) ) even if one wishes just to calculate structural properties, such as
, for the nonequilibrium system, and typically the nonequilibrium perturbation (the term
in the present case) creates an essential anisotropy of the system. Note that this extra term cannot be incorporated into the functional derivative of the effective free energy of the system – there is no counterpart to “thermodynamic potentials” far from equilibrium, at least not in general. We mention, however, that for shear induced phase separation of polymer solutions and polymer blends one can obtain a quasi-thermodynamic theory, by adding a stored elastic energy contained in the sheared system to the standard (Flory-Huggins[Citation54]) free energy of the system, describing thus the shift of the critical point due to shear.[Citation55] However, the validity of this approach is doubtful.
There is a rich variety of nonequilibrium phase transitions occurring in systems that are externally driven. One of the simplest systems is the lattice gas model driven by an electric field (the particles carry an electrical charge, but as in the standard lattice gas model, that is equivalent to the Ising ferromagnet, the only interparticle interaction is the nearest-neighbor attraction between the particles).[Citation56,Citation57] The density of this lattice gas is conserved, and the dynamics is associated by allowing hopping of the particles from their sites to adjacent empty sites on the lattice. Due to the periodic boundary conditions, a steady-state situation (with a constant current of particles along the field direction) is established. While for the equilibrium lattice gas model, the rate for attempted jumps (in the case of infinite temperature, , when the attraction does not matter) is the same for all neighboring sites, say
, in the presence of the field the rates are largest in the direction of the field
, smallest against it
, and in between in transverse direction
. In the absence of the field, this model is the Kawasaki spin exchange kinetic Ising model [Citation58] suitable to describe (qualitatively) the dynamics of interstitial alloys (in
dimensions) or diffusion in physisorbed monolayers at crystal surfaces (in
dimensions). The extension including the electric field is sometimes used to model fast ionic conductors (also called superionic conductors).[Citation59] Often, this model is considered in the limit of a very strong electric field, where no hopping against the field is possible. The model is clearly formulated as appropriate for studies using Monte Carlo simulation methods;[Citation31,Citation32,Citation33] but while the latter have yielded very precise and reliable results for the lattice gas/Ising model in thermal equilibrium,[Citation24,Citation25] despite longstanding attempts [Citation57,Citation60] the description of the critical behavior of this model has met many difficulties. Note that due to the anisotropy created by the driving field one needs to distinguish two correlation lengths
parallel and perpendicular to the field direction (which also diverge with different exponents
). While for standard critical phenomena in isotropic systems finite size scaling methods [Citation61] are well developed and rather straightforward to apply [Citation31,Citation32] in systems with anisotropic critical behavior, the linear dimensions
have to be deliberately chosen consistent with this anisotropic critical behavior.[Citation62,Citation63] While in equilibrium systems, the principle that, in the thermodynamic limit, the different ensembles of statistical thermodynamics (that are related to each other, as is well known, by Legendre transformations) yield equivalent results allows the choice of the ensemble which for finite size scaling methods is most convenient, no such principle exists for systems far from equilibrium! In the equilibrium lattice gas, the choice of the grand canonical ensemble allows to study the phase transition from the disordered phase to the ordered phase without being hampered by interfacial effects associated with the two-phase coexistence: the latter cannot be avoided in nonequilibrium problems, such as the driven lattice gas.
While the driven lattice gas (as well as phase separation in a sheared binary mixture) starts with a system that already has a phase transition in equilibrium, and one then asks the question how is the transition changed when the external driving is present, there are also many cases of genuine non-equilibrium phenomena, not related to any phase transition in equilibrium. Examples include hydrodynamic instabilities such as the onset of the convective Rayleigh-Bénard instability,[Citation64–66] or “Absorbing Phase Transitions” in models for reaction-diffusion processes.[Citation67] Here we shall briefly describe the Rayleigh-Bénard problem only. Here, one considers a fluid held between parallel plates, where the upper plate is held at temperature while the lower plate is held at temperature
, the distance between the (infinitely large) plates is
. Due to this temperature gradient
, heat is transported from the lower plate to the upper one, maintained by a power density
of heating, and one is interested in the effective thermal conductivity
. If no convection is present, only standard heat conduction occurs,
, the standard thermal heat conductivity. The instability arises because, in this setup, the colder liquid on top is slightly heavier than the warmer liquid on the bottom, against the action of gravity. The characteristic control variable for this problem is the “Rayleigh number”
, defined as
with gravitational acceleration , isobaric thermal expansion coefficient
, thermal diffusivity
, and kinematic viscosity
. It turns out that convection in the form of a roll pattern sets in [Citation64–66] when
, while for
the “Nusselt number”
exceeds unity. Thus, the region
plays the role of the “disordered phase” of this nonequilibrium transition, while
is a kind of order parameter.
To estimate , one uses the standard hydrodynamic equations, but assumes that the temperature dependence of
, k,
can be neglected. Denoting by
and
the deviations of the velocity, temperature and pressure profiles across the layer from the profiles in pure heat conduction, these equations are
being a unit vector in the direction of the gradient. In EquationEquations (5)
(5)
(5) ,(Equation6
(6)
(6) ), lengths are measured in units of the layer thickness
, times in units of
, temperature in units of
, so we deal here with macroscopic variables, not a description on molecular scales. One can add random force terms [Citation66] to describe statistical fluctuations, but there are no fluctuation–dissipation relations linking them to transport coefficients of the fluid. The critical value
is then estimated applying the well-known concept of linear stability analysis [Citation68] of EquationEquations 5
(5)
(5) ,Equation6
(6)
(6) , using boundary conditions
,
,
at both the lower and upper plates.
By studying the decay rate of modes for various wavevectors , one finds that an instability sets in for
, and the convective roll pattern is characterized by a wavenumber
. The amplitude and shape of the pattern can only be obtained from a study of the full nonlinear problem.[Citation66] While the driven lattice gas model concerns a nonequilibrium phase transition where the elementary degrees of freedom (the hopping particles) represent certain atoms of a superionic conductor, for the Rayleigh-Bénard problem, one considers pattern formation on a macroscopic scale. The active systems, which we will consider next, study degrees of freedom also of macroscopic objects (e.g. flocks of birds) or at least mesoscale objects, such as active Brownian particles.
Selected Models for Active Matter Systems
While the constituent particles of standard condensed matter range in size from atoms or molecules to mesoscopic size of a few (e.g. the colloidal particles in colloid-polymer mixtures where liquid-gas type phase separation in a phase rich in polymers and a phase rich in colloids can be observed [Citation69–72]), the “particles” constituting “active matter” range in size from mesoscopic objects (colloids, [Citation9,Citation73–75] living bacteria, [Citation6,Citation76] etc.) to macroscopic living animals (flocks of birds [Citation77] etc.). The distinctive feature of these “particles” in active matter is that they are self-propelled, i.e. they convert free energy into directed motion. In all these systems, energy has to be supplied continuously to maintain a steady state: this can be due to chemical processes, e.g. when a spherical colloidal particle has two different hemispheres (“Janus particle”) so that the chemical reaction occurs on one hemisphere only.[Citation78] However, this energy can also be supplied by external fields (light, magnetic fields, etc.) or by mechanical shaking of granular particles.[Citation79] In all such active systems, there is no detailed balance, unlike systems in thermal equilibrium. In the present article, we shall not at all dwell on the mechanisms that create such a motility of particles, but shall only give a qualitative discussion of the consequences of this motility for the behavior of a few popular models.
The most well known of these models is the Vicsek model, [Citation2–5] which was originally intended to understand the collective behavior of flocks of birds or other animals. No realistic description at all was aimed at, and thus, particles moving in a two-dimensional plane with periodic boundary conditions were assumed. Each particle is characterized by its position and velocity
, pointing in a direction characterized by an angle
relative to the x-axis, at time
.
, and at each time step
the direction of the motion is updated according to [Citation2,Citation4]
where the noise is a random variable with a uniform distribution in the interval
and
denotes an average over all particles in the local neighborhood of particle
(i.e., all particles within a circle of radius
centered at the position of the i’th particle). The locations of the particles are updated as [Citation2,Citation4]
The motivation for the choice of interactions among particles implicit in EquationEq. 7(7)
(7) is the analogy with isotropic ferromagnets, where exchange interactions align the directions of neighboring spins, while here the directions of the neighboring particles are preferentially aligned, depending on the strength
of the noise and the density
of the particles. The “fluid” described by EquationEquations 7
(7)
(7) , Equation8
(8)
(8) is far from equilibrium also on small timescales, since only the density is conserved, while there is no momentum conservation nor Galilean invariance.[Citation80] One may introduce the analog of an order parameter by considering the magnitude of the average momentum of the N particles,
. Depending on the strength of the noise
, one finds (for
) that a steady state develops, with a disordered phase for
, and an ordered phase with
for
, even though one starts the system with an initially disordered configuration,
. Note that a system at initially uniform density and
develops into a state of non-uniform density, i.e. phase separation into an ordered cluster with large
and a low-density disordered phase occurs ().[Citation4] While for a XY ferromagnet in
dimensions, there is no long-range order,[Citation81] in this nonequilibrium phase transition, obviously there does occur a spontaneous breaking of a continuous symmetry in
. This destruction of the spontaneous magnetization in two-dimensional isotropic magnets is due to long wavelength spin wave excitations, which cost little energy
(
at wavevector
), and occur with a large statistical weight, proportional to the Boltzmann factor
, where
is absolute temperature and
Boltzmann’s constant). However, for nonequilibrium systems such as described by the Vicsek model, there is no quantity that would correspond to temperature, and the Boltzmann factor there has no meaning. While in equilibrium, critical phenomena are universal, and hence details on small scales are irrelevant and can be eliminated by a suitable coarse-graining, [Citation20–23] for active matter systems it is by no means a priori clear which features of a system are irrelevant details and which features are generic. Thus, one should not be misled by superficial analogies!
Figure 1. Snapshot pictures of the time development of a system with particles in
box with
and
at 50 (a) and 3000 (b) steps. Adapted from Czirok et al.[4] © IOP publishing. Reproduced with permission. All rights reserved
![Figure 1. Snapshot pictures of the time development of a system with N=4000 particles in L×L box with L=40 and v0=0.01 at 50 (a) and 3000 (b) steps. Adapted from Czirok et al.[4] © IOP publishing. Reproduced with permission. All rights reserved](/cms/asset/b272f225-59f5-424e-a21c-6094f98dc2d4/lsfm_a_1906703_f0001_b.gif)
Our second example are active Brownian particles (ABP).[Citation8,Citation82–86] Recall that passive Brownian particles are ubiquitous in colloidal suspensions, where we may have spherical silica particles with radius R much larger than the size of the fluid molecules in the suspension, and also their mass is orders of magnitude larger than the masses of the fluid molecules.[Citation71,Citation87] Due to this disparity of scales, the motions of the colloidal particles are orders of magnitude slower than the motions of the molecules: on the time scale of colloidal motion, interactions with molecules can simply be modeled as uncorrelated random collisions, and so one is led to the standard Langevin equation description for the diffusive motion of colloidal particles.[Citation87,Citation88] Both translational diffusion and rotational diffusion can be considered. Again, the statistical thermodynamics of equilibrium enters on a fundamental level, linking the noise and the friction constant via the fluctuation dissipation theorem.[Citation88] In the absence of any (effective) attractive interactions between such passive colloidal particles, no phase transitions whatsoever are expected in such a colloidal suspension in equilibrium, apart from entropy-driven crystallization [Citation87] at high densities of the colloids (when the typical distance between the particles is of the order of the colloid diameter) and potentially an intermediate hexatic phase in two-dimensional systems.[Citation89–91]
However, the situation changes fundamentally when we give the Brownian particles a velocity of fixed absolute value
, like in the Vicsek model. The direction of this speed decorrelates gradually via rotational diffusion, as in thermal equilibrium, with angular diffusion constant
. The equation of motion for an active Brownian particle is then postulated as a Langevin equation for the position
of particle
[Citation86]
where the first term on the right-hand side describes that the particle is propelled with constant velocity along the direction of its orientation vector
. The translational friction coefficient here is denoted by
,
is the force acting on the particle due to the total potential
acting on the particle, and
is a random force as in thermal equilibrium, with zero average
) and satisfying the usual fluctuation-dissipation theorem [Citation88]
with in
dimensions or
in
dimensions. Note that the translational diffusion constant (in equilibrium) then simply is
; henceforth we may use units such that
and then
is unity as well. A crucial point now is that the direction
undergoes rotational diffusion, with a diffusion coefficient
. The importance of noise for the motion of the active particle is measured by the Péclet number,
,
being the diameter of the (spherical) particles. In the context of simulation studies, it is often convenient to work with a strongly repulsive Weeks-Chandler-Andersen pair potential [Citation92] modeling the interaction between the particles and then
in
gets renormalized by an effective hard sphere or hard disk parameter, respectively.[Citation16]
Clearly, in the absence of self-propulsion, this model is essentially a fluid of hard disks or hard spheres
, and no vapor-liquid-type phase transition can occur. Thus, the occurrence of a vapor-liquid-type critical point and liquid-vapor phase separation in this model for strong self-propulsion has been a surprise () .[Citation16]
Figure 2. Simulation results for the phase diagram of active Brownian particles in dimensions, plotting the state of the system in the plane of variables packing fraction
and Péclet number
. Within the region confined by the binodal (dashed and solid line), two-phase coexistence occurs between a gas-like phase and a liquid like-phase, as indicated. Open circles show the coexisting packing fractions of the gas
and liquid
, respectively, and also the packing fractions at the rectilinear diameter
are indicated (red circles). The location of the critical point (diamond) was estimated by a subsystem finite size scaling analysis. Reprinted figure with permission from J.T. Siebert et al., physical review E 98, 030601 (2018) [Citation16] (https://doi.org/10.1103/PhysRevE.98.030601). Copyright 2018 by the American Physical Society
![Figure 2. Simulation results for the phase diagram of active Brownian particles in d=2 dimensions, plotting the state of the system in the plane of variables packing fraction ϕ and Péclet number Pe. Within the region confined by the binodal (dashed and solid line), two-phase coexistence occurs between a gas-like phase and a liquid like-phase, as indicated. Open circles show the coexisting packing fractions of the gas (ϕg) and liquid (ϕℓ), respectively, and also the packing fractions at the rectilinear diameter ϕd=(ϕg+ϕℓ)/2 are indicated (red circles). The location of the critical point (diamond) was estimated by a subsystem finite size scaling analysis. Reprinted figure with permission from J.T. Siebert et al., physical review E 98, 030601 (2018) [Citation16] (https://doi.org/10.1103/PhysRevE.98.030601). Copyright 2018 by the American Physical Society](/cms/asset/9c0ce576-87e2-4b54-b6ce-a36d92580e9a/lsfm_a_1906703_f0002_oc.jpg)
At first sight, looks like an equilibrium-phase diagram, taking the role that inverse temperature plays in equilibrium. However, this analogy is extremely superficial: in a thermal equilibrium system, in the thermodynamic limit, the properties of the system do not depend on the boundary conditions that are chosen, and all (large) subsystems of the system are at rest, there are no large-scale relative motions of subsystems, so choosing (in a theoretical or simulational study) periodic boundary conditions does not affect the findings on the “bulk” properties of the system. This is completely different here: When we choose periodic boundary conditions here, the system develops at high densities an alignment of the particle velocities
which differ from the orientations of the active forces,
. This phenomenon is shown in , and has no analog whatsoever in equilibrium.[Citation18] It is also noteworthy that the internal structure of the dense phase is rather complex consisting of patches with hexagonal order and bubbles with rather low densities.[Citation93] The special character of active fluids emerges already in the dilute limit, where pair correlations between active particles display a special anisotropic structure (“depletion wings” [Citation94]), unlike equilibrium fluids formed from point like particles, where pair correlations are isotropic, of course. Due to the active motion, a new characteristic length, called “persistence length”
, matters. Experiments on Janus particles have verified some of the theoretical consequences of this description.
Figure 3. (a) Snapshot configuration of the two-dimensional active Brownian particle model, for a large volume fraction , in a square
box with periodic boundary conditions and
,
. (b) Enlarged portion of the high density cluster. Colors encode the direction of the self-propulsion. (c,d) Windows from the bulk where the velocities of the particles are shown by blue arrows, showing aligned and vortex domains, respectively. So one can see that there is little correlation between these directions for neighboring particles, even if strong correlations between their velocities (shown by arrows) occur. Reprinted figure with permission from L. Caprini et al., physical review letters 124, 078001 (2020) [Citation18] (https://doi.org/10.1103/PhysRevLett.124.078001). Copyright 2020 by the American Physical Society
![Figure 3. (a) Snapshot configuration of the two-dimensional active Brownian particle model, for a large volume fraction η=0.64, in a square L×L box with periodic boundary conditions and Dr=0.2, v0=5.0. (b) Enlarged portion of the high density cluster. Colors encode the direction of the self-propulsion. (c,d) Windows from the bulk where the velocities of the particles are shown by blue arrows, showing aligned and vortex domains, respectively. So one can see that there is little correlation between these directions for neighboring particles, even if strong correlations between their velocities (shown by arrows) occur. Reprinted figure with permission from L. Caprini et al., physical review letters 124, 078001 (2020) [Citation18] (https://doi.org/10.1103/PhysRevLett.124.078001). Copyright 2020 by the American Physical Society](/cms/asset/a03b149b-69bf-4bad-974d-04b6b28d008c/lsfm_a_1906703_f0003_oc.jpg)
When, for a system in thermal equilibrium, one has a phase diagram of the type in , a state within the miscibility gap means two-phase coexistence, with both phases having then the same temperature and pressure
. It is already a nontrivial issue to give these quantities a precise and unique meaning (recall that also for the Rayleigh-Bénard problem), [Citation66] we had to deal with a space-and-time-dependent temperature field, but due to the macroscopic character of the problem, the formulation of EquationEquations 5
(5)
(5) ,Equation6
(6)
(6) rested on a local equilibrium assumption, so there was no problem to define what the temperature field
meant). For the mesoscopic active particles of interest in the present section, we have a well-defined temperature
for the background fluid of the colloid suspension, responsible for the noise terms in the Brownian motion (cf. EquationEquation 10
(10)
(10) ), but this temperature has nothing to do with the effective temperature related to the average translational kinetic energy of the active particles,
(
denotes the mass of the active particles). Now it turns out that for the model defined by EquationEquations 9
(9)
(9) 10
indeed is constant in the steady states of the system, but this is not the case for a slightly different model, [Citation17] where one considers underdamped rather than overdamped Brownian motion, where an acceleration term
is added to the friction term
in EquationEquation 9
(9)
(9) , and also the moment of inertia I needs to be accounted for orientational relaxation.[Citation17] In this case, particles in the gas phase are typically much faster than in the dense phase. It has been shown that the coexistence of phases is possible which have different kinetic temperatures!
Also, the meaning of the pressure needs to be carefully discussed.[Citation86] In equilibrium, for a system enclosed by hard walls, there is an obvious mechanical interpretation of the pressure in terms of the forces exerted by the particles on the walls. Being interested in the simulation of systems with periodic boundary conditions, the standard approach is to apply the Virial theorem, which can be extended to active Brownian particles as
density of the particles) [Citation86]
Here, the first term represents the ideal gas contribution, and the (standard) second term is due to the forces acting between particles
and
. The so-called “swim pressure” is due to the activity of the particles,
being the average velocity of the particles. As an example, the swim pressure is shown to be a function of volume fraction for various Péclet numbers in .[Citation86] A detailed analysis of the total pressure shows that the phase transition from vapor to liquid is accompanied by a pressure discontinuity, [Citation86] unlike what happens in equilibrium. Recall that in thermal equilibrium two bulk coexisting phases have the same pressure, and an isotherm of the density versus pressure has a horizontal part (a state in this horizontal part means that both phases are present in the system, their amount being given by the lever rule). Mean field theories in equilibrium rather yield a “van der Waals loop,” but the correct description of phase coexistence is then found by a Maxwell construction. The latter also applies to simulation results for finite systems (which are numerically exact, apart from statistical errors[Citation32,Citation33]), where a loop occurs as a finite size effect due to interfacial contributions (see, e.g., [Citation50]). However, there is no Maxwell construction for active systems, not even within a mean field framework (see, e.g., [Citation95] and references therein).
Figure 4. The swim pressure of a three-dimensional system of active Brownian particles plotted vs packing fraction , for various Péclet numbers: Pe = 9.8 (purple), 29.5 (olive), 44.3 (green), 59.0 (blue), and 295.0 (red). The values are normalized by the ideal (bulk) pressure of individual active Brownian particles as at the same Péclet number. From Winkler et al. [86] – Published by the royal society of chemistry
![Figure 4. The swim pressure of a three-dimensional system of active Brownian particles plotted vs packing fraction ϕ, for various Péclet numbers: Pe = 9.8 (purple), 29.5 (olive), 44.3 (green), 59.0 (blue), and 295.0 (red). The values are normalized by the ideal (bulk) pressure of individual active Brownian particles as at the same Péclet number. From Winkler et al. [86] – Published by the royal society of chemistry](/cms/asset/02b49707-9029-4279-96e1-60abbb44a7a7/lsfm_a_1906703_f0004_oc.jpg)
While neither the Vicsek model nor the model of active Brownian particles exhibits a liquid-gas phase separation when the motility is shut off, it is also possible to modify models, which show a transition already in equilibrium, by adding motility to them, in the desire to study how the properties of the transition then change. An example of this approach is colloid-polymer mixtures, where already in the passive limit, phase separation may occur in a polymer-rich phase and a colloid-rich phase.[Citation69–72] In thermal equilibrium, the generic model for such systems is the Asakura-Oosawa model,[Citation69,Citation71,Citation72] where the colloids are hard spheres of diameter and the polymers are soft spheres of diameter
. While polymers can overlap without energy cost, overlap of colloids and polymers is strictly forbidden. This choice of interactions creates the entropic “depletion attraction” [Citation71] among the colloids. One wants to understand for which choices of the polymer and colloid packing fractions
(with polymer density
(with
this depletion attraction leads to phase separation into a polymer-rich and a colloid-rich phase.
As is well known, equilibrium phase behavior is discussed most elegantly in terms of intensive rather than extensive thermodynamic variables (or the densities of the latter). Thus, for the standard liquid–vapor transition for a simple fluid, we use temperature and pressure rather than entropy (or enthalpy) and volume. For the colloid-polymer mixture, the intensive variables of interest are the chemical potentials of polymers and colloids
, respectively. It has become common practice [Citation72] to use the so-called polymer reservoir packing fraction
instead of
as a control variable. The phase transition then occurs at a transition line in the
plane, ending in a critical point. Of course, in equilibrium we can use Legendre transformations to map out the phase diagram also in the
-plane or
plane, respectively ( [Citation96]). The latter choice has the advantage that the tie lines between coexisting vapor-liquid and liquid-like phases must be strictly horizontal, and hence the critical point must be at the minimum of the miscibility gap in (insert). In contrast, the tie lines in the
representation have nontrivial slopes and finding the location of the critical point is a difficult task.
Figure 5. Phase diagram of the AO model for in the plane of variables
and
, as well as using
and
(insert). Continuous curves show the so-called free volume approximation (which implies a mean-field-type critical behavior) while symbols show Monte Carlo data (which imply a critical behavior compatible with the Ising model universality class). The square indicates the location of the critical point. Reprinted from R.L.C. Vink and J. Horbach, journal of chemical physics, 121, 3253, (2004), [Citation96] with the permission of AIP Publishing
![Figure 5. Phase diagram of the AO model for σp/σc=0.8 in the plane of variables ηc and ηp, as well as using ηc and ηpr (insert). Continuous curves show the so-called free volume approximation (which implies a mean-field-type critical behavior) while symbols show Monte Carlo data (which imply a critical behavior compatible with the Ising model universality class). The square indicates the location of the critical point. Reprinted from R.L.C. Vink and J. Horbach, journal of chemical physics, 121, 3253, (2004), [Citation96] with the permission of AIP Publishing](/cms/asset/35ec8037-ab2d-447b-b2b9-87c81db7c0d6/lsfm_a_1906703_f0005_b.gif)
In order to extend the AO model to the case of active colloids, a variant of the original model was used, where the hard-core potentials were mimicked by smooth potentials of the WCA type, [Citation97] and instead of a soft repulsion was chosen.[Citation97] The change of the phase diagram of this model in comparison with is almost negligibly small. For this model, it is then possible to use a Langevin-type equation of motion similar to EquationEquation 9
(9)
(9) [Citation10,Citation12]
but including an inertial term. Unlike the active Brownian particles, a force is chosen that aligns the velocity of the considered particle with the velocities of its neighbors, as in the Vicsek model [Citation10,Citation12]
where is an amplitude factor, which is a control variable (typically
), and
denotes an average over all colloids within a sphere of radius
(typically twice the colloid diameter). While a configuration snapshot of phase coexistence in the active system () looks like its counterpart of a passive system, and also the average density profiles in the direction perpendicular to the interfaces () resemble equilibrium systems, other properties are very different. Indeed, it was found that using EquationEquations 13
(13)
(13) , Equation14
(14)
(14) , the miscibility gap became enlarged, in comparison with the passive model.[Citation10,Citation12] In addition, the model exhibits very pronounced nonequilibrium effects, since the velocities of the particles exhibit huge fluctuations both in sign and in magnitude. In the regime where phase coexistence occurs, the clustered active particles travel mostly in the same direction, and the kinetic temperature in the colloid-rich phase deviates from the temperature chosen for the thermostat by more than an order of magnitude () .[Citation10]
Figure 6. (a) Snapshot picture of the active polymer-colloid mixture at the state point ,
with
, for a box with linear dimensions
,
. Colloids are shown in yellow, polymers in black. (b) Profiles of
and
in z-direction. (c) Corresponding profiles for the various components of T(z) as obtained from the equipartition theorem. Reprinted from B. Trefz et al., journal of chemical physics, 144, 144902, (2016), [Citation10] with the permission of AIP publishing
![Figure 6. (a) Snapshot picture of the active polymer-colloid mixture at the state point ηc=0.15, ηp=0.20 with fA=10, for a box with linear dimensions Lx×Ly=12, Lz=48. Colloids are shown in yellow, polymers in black. (b) Profiles of ηp and ηc in z-direction. (c) Corresponding profiles for the various components of T(z) as obtained from the equipartition theorem. Reprinted from B. Trefz et al., journal of chemical physics, 144, 144902, (2016), [Citation10] with the permission of AIP publishing](/cms/asset/a90963f5-8801-4290-a047-d0ab1b0b182e/lsfm_a_1906703_f0006_oc.jpg)
The AO model [Citation69] already in equilibrium is very useful to understand another important aspect of phase behavior, namely, the competition between the liquid–vapor transition and the liquid-solid transition of fluids.[Citation70–72] If the attractive forces (in equilibrium) between the particles are completely absent, we still have (for spherical particles of diameter ) a liquid – solid transition. This transition in
is of first order; we have a two-phase coexistence region from a packing fraction
to
, where a face-centered cubic solid takes over. Attractive interactions (due to flexible polymer coils) are characterized by two basic parameters, their strength and their range
. Only when
is large enough can we observe a vapor-liquid-like transition: for small
one rather observes that the boundary between the fluid and the two-phase region with increasing interaction strength bends over to rather small
. For intermediate attraction strength, a metastable vapor, liquid-like transition is found (in mean-field treatments) within the fluid-solid two-phase region. Interestingly, there is also evidence that the
ABP model belongs to this scenario, with a metastable phase boundary separating the vapor-like phase from the vapor-liquid two-phase region INSIDE of the vapor-crystal two-phase coexistence region .[Citation98] In
, however, for small Peclet numbers rather a coexistence region between an active fluid and a hexatic phase, followed by a pure hexatic phase, is found.[Citation99] Note, however, that the existence of hexatic phases is due primarily to entropic effects: there seems to exist evidence [Citation100] that in the presence of strong enough attractive forces the fluid-solid transition in
is of first order, as in
dimensions.
How Can We Learn from Simulation Studies about the Critical Behavior of Active Systems?
When strong phase separation occurs, as shown in extracting the densities in either of the coexisting phases can be done rather straightforwardly, directly from the average profiles (6b). However, near the critical point, this naive analysis breaks down, since the width of the interfaces increases and strong fluctuations in the remaining bulk phases make quantitatively reliable direct estimations almost impossible.
This problem would arise also if we study the equilibrium phase transitions by simulations and analyze the data in this straightforward way. However, for equilibrium phase transitions, it is well established that the most efficient approach to analyze simulations is finite size scaling.[Citation23,Citation31,Citation32,Citation61,Citation101] The simplest case is the Ising model of ferromagnets, where at zero magnetic field upon lowering the temperature
a phase transition occurs, in the thermodynamic limit, from the paramagnetic state at
to the ferromagnetic state at
, where states of nonzero spontaneous magnetization
; we normalize the magnetization per spin) occur. Of course, in a finite system there cannot be any spontaneous symmetry breaking: in equilibrium the distribution
of the magnetization per spin, for a d-dimensional lattice of linear dimension
, in thermal equilibrium is always symmetric with respect to the sign of
. To a good approximation,
for
is simply a Gaussian centered at
, as one can justify simply from the law of large numbers; for
one finds an appearance of two peaks, centered near
. As one approaches
from above, the width of the distribution broadens and its form becomes distinctly nongaussian, when the correlation length of spin correlations
(which diverges at
for
) becomes comparable to the chosen
. Near
a gradual change of the distribution from single peak form to double peak form occurs. Thus, as a function of
both
and all its moments
are nonsingular functions of
. Now, finite size scaling [Citation61,Citation101] states that near
and for large enough
,
depends on the three variables
and
not separately but only on two scaled variables,
and
. Since
,
, where
and
are the critical exponents of the correlation length and order parameter, one can choose also
instead of
as a scaling variable. Normalization of the probability then requires that
can be written as
here) [Citation31,Citation32,Citation61,Citation101]
where . Choosing the arguments of the scaling functions
and
in this particular way is advantageous because it brings out that
and
are nonsingular functions of their variables, since in a finite system
and
are not singular as function of
and hence singularities only arise when the limit
is taken before
.
From EquationEquation 15(15)
(15) the well-known cumulant intersection method [Citation101] emerges, i.e.,
is a function of
only and hence for
all cumulants for different choices of
must intersect at a common point
,
The location of this common intersection point hence yields , with no need to know anything else in beforehand. At
, the second moment varies as
, hence allows to estimate the ratio
of the critical exponents. Since
is a continuous function of
, we must have
near
, studying the L-dependence of the slope of
at
hence yields
. Since finite size scaling implies [Citation101] also the validity of the “hyperscaling” relation [Citation21–23]
, the susceptibility exponent
follows (as well as the other exponents via scaling laws[Citation19–21]).
This account of finite size scaling in a nutshell is not the whole story, of course: (i) EquationEquations 15(15)
(15) ,Equation16
(16)
(16) hold only strictly in the asymptotic limit
, for practically useful values of
corrections to finite size scaling need careful consideration.[Citation24,Citation25,Citation32] (ii) If one would apply a local Monte Carlo algorithm [Citation31,Citation32] e.g. single spin flips, the accuracy of the sampling is strongly hampered by critical slowing down,[Citation38,Citation39] i.e. the sequence of generated system configuration exhibits strong “dynamic” correlations with a relaxation time
, where (in the absence of any conservation laws) the dynamic exponent
is [Citation32,Citation38]
. Accurate results for large
could be obtained [Citation24,Citation25] thanks to the invention of cluster algorithms [Citation32] which strongly reduce critical slowing down.
However, obtaining accurate information on the critical behavior of models for fluids exhibiting a vapor-liquid transition has been much more difficult and possible only at the beginning of the present century, [Citation102] due to several reasons: (i) for off-lattice systems cluster algorithms are much less powerful than for the Ising model (ii) While in the Ising model there is the special symmetry that the internal energy is invariant against spin reversal while the order parameter changes its sign, these two quantities are in a sense orthogonal to each other, unlike fluids where no special symmetry exists, and energy density and particle number density are “coupled operators” (“field mixing” effect [Citation103]). Therefore, it is a nontrivial task not only to locate the critical temperature, but also to locate the critical density (which in the lattice gas fluid, that corresponds to the Ising model, by symmetry is ) and the chemical potential
and pressure
at criticality. A consistent analysis [Citation102] needs to consider the coupling between the three “scaling fields”
and
in order to correctly extract critical properties.
Still, the task is manageable since in equilibrium one can perform the simulation in the grand canonical ensemble where is used as a control variable, and moves involving the insertion and deletion of particles are carried out, and thus the density distribution
, which is the analog of EquationEquation 15
(15)
(15) , can be sampled. However, for systems of active particles, no analog of the grand-canonical ensemble exists; rather one is forced to work in the canonical ensemble where the average density of active particles in the system is strictly constant; and it is just a heuristic speculation to assume that the finite size scaling concept can be transferred to phase transitions in active matter!
Of course, also in equilibrium systems one can try an extension of the finite size scaling method to the canonical ensemble where . This can be done by considering sub-systems of the considered total system: exchange of particles through the subsystem boundary into the neighborhood is then possible, and for each subsystem, the rest of the system acts like a reservoir of particles.
This approach was tried by Siebert et al., [Citation16] using subsystems of a simulation box which has extensions
. When the system is in the two-phase coexistence region, the interfaces between the coexisting phases of low density and high density will be oriented perpendicular to the long direction (the x-direction). In each configuration that is analyzed one can then locate these interfaces (in the region where phase separation occurs) and record the properties of subsystems that are located in the “pure” phases, to avoid artifacts due to “contamination” of the bulk properties with interfacial properties (). If one includes subsystems that contain the interfaces, one does not obtain useful results as one can verify already for the Ising model.[Citation16,Citation104] With the present variant of the method, the critical temperature of the Ising model can be located with reasonable accuracy (), and also good estimates of the critical exponents could be extracted .[Citation16] Unfortunately, for the ABP system, the observed cumulant crossing is less impressive (). Of course, for the Ising system using a total magnetization of zero trivially ensures that at
one reaches critical conditions; the choice of density for the ABP model requires already some estimate for the critical density, which is unknown beforehand, and an inaccurate choice clearly may be the source of an uncontrolled error. Neither in this Ising model with conserved magnetization nor in the ABP model could the region very close to criticality be reliably probed (unlike studies of the Ising model in the grand canonical ensemble). This fact is due to the difficulty that in the Ising model with conserved order parameter the dynamic exponent
is much larger than in the non-conserved case,[Citation38] critical slowing down hence is a much more serious problem for simulations. So while Siebert et al.[Citation16] found that the exponents differ significantly from those of the Ising model, one must make the caveat that the range of
that could be explored is only
to
, i. e. it spans less than a factor 2, while for accurate estimates of equilibrium systems, one needs about a decade.[Citation24,Citation25,Citation32] Also, a direct study of the order parameter as a function of the distance
from criticality
here could only probe the range
, while in equilibrium the proper exponents are seen for
only!
Figure 7. (a) Schematic representation of the simulation box used for the cumulant analysis. Simulations are done at medium packing fraction of particles with an edge length ratio of 3:1 and periodic boundary conditions. This results in a two-phase coexistence with a slab geometry of the liquid domain. The slab is always aligned with the short axis. Two subboxes are then placed in the liquid domain, such that the x-coordinates of their center of mass coincides with the x-coordinate of the liquid domain. The other two subboxes are shifted by
along the x-axis, so they are centered in the vapor region. (b) Cumulant ratio
for several choices of
, namely
and 15 lattice spacings, for the Ising/lattice gas model in
dimensions, plotted vs. temperature
(in units of the exchange constant
). (c) Cumulants for the two-dimensional active Brownian particle model plotted vs. the Péclet numbers for four choices of
(in units of
) as indicated. A crossing of
can be seen for roughly
. The dashed lines are guides to the eye only. Reprinted figure with permission from J.T. Siebert et al., physical review E 98, 030601 (2018) [16] (https://doi.org/10.1103/PhysRevE.98.030601). Copyright 2018 by the American Physical Society
![Figure 7. (a) Schematic representation of the simulation box used for the cumulant analysis. Simulations are done at medium packing fraction of particles with an edge length ratio of 3:1 and periodic boundary conditions. This results in a two-phase coexistence with a slab geometry of the liquid domain. The slab is always aligned with the short axis. Two ℓ×ℓ subboxes are then placed in the liquid domain, such that the x-coordinates of their center of mass coincides with the x-coordinate of the liquid domain. The other two subboxes are shifted by 3ℓ along the x-axis, so they are centered in the vapor region. (b) Cumulant ratio Qℓ=⟨m2⟩ℓ2/⟨m4⟩ℓ for several choices of ℓ, namely ℓ=8,10,12 and 15 lattice spacings, for the Ising/lattice gas model in d=2 dimensions, plotted vs. temperature T (in units of the exchange constant J/kB). (c) Cumulants for the two-dimensional active Brownian particle model plotted vs. the Péclet numbers for four choices of ℓ (in units of σ) as indicated. A crossing of Qℓ(Pe) can be seen for roughly Pe=40±2. The dashed lines are guides to the eye only. Reprinted figure with permission from J.T. Siebert et al., physical review E 98, 030601 (2018) [16] (https://doi.org/10.1103/PhysRevE.98.030601). Copyright 2018 by the American Physical Society](/cms/asset/c4da6bdd-802d-4c83-89db-13ecdc43b7e0/lsfm_a_1906703_f0007_oc.jpg)
Thus, it is no surprise that doubts on the validity of the results of Siebert et al.[Citation16] have been raised.[Citation105] In particular, Partridge and Lee [Citation105] proposed a variant of APB on a triangular lattice, where particles with an orientation pointing in the lattice direction can either undergo rotational diffusion or jump (with preference in the direction of their orientation) to a neighboring site, provided this site is empty. Applying the same type of finite size scaling analysis as Siebert et al. [Citation16] for , evidence for Ising-like behavior was found, while indications are somewhat less clear for another active lattice model [Citation106] as investigated, recently, in Ref.[Citation107] 2d-Ising behavior has also been reported of late for off-lattice active Ornstein-Uhlenbeck particles.[Citation108]
In our view, these discrepancies between [Citation16] and [105] do not imply that the described finite size scaling analysis fails in principle; rather, the difficulties are reminiscent of the early days of Monte Carlo studies of equilibrium critical behavior, [Citation31,Citation32,Citation101] where due to the use of too small system sizes and too short simulation runs the desired accuracy of estimates could not be reached. Thus, it is hoped that future work can resolve these problems.
Also, in the active version of the AO model (polymers + active colloids) [Citation10,Citation12] the situation allows to draw rather tentative conclusions only: while again a subsystem finite size scaling approach is the method of choice, one needs to analyze the distribution of two densities, . Using subsystems in the disordered phase near criticality gave results [Citation12] compatible with the Ising universality class, but (in
dimensions) only rather small values of
were accessible
, and the location of the critical point could only be very roughly estimated[Citation12]). Thus, while in principle simulations should be able to clarify the critical behavior of phase transitions in active matter systems, clearly more work is still necessary.
Conclusions
Active matter systems undergoing phase transitions exhibit a wealth of interesting phenomena, with similarities to systems undergoing phase transitions in thermal equilibrium, or in systems under external drive creating a steady flow of heat or particles through the system, but there also occur very interesting differences. We have shown that phenomena such as coherent spontaneous large-scale spontaneous flow patterns of particles can emerge (e.g. ), the effective temperatures can differ between coexisting phases (), and so on. An interesting feature that we have not yet addressed is the fact that the interface tension between coexisting phases, when defined from the anisotropy of the pressure tensor, can become negative.[Citation14,Citation37,Citation109] In equilibrium fluid systems, the interfacial tension equals the interfacial stiffness, which controls the capillary wave broadening of the interface (and must be positive, while the interface would be unstable against roughening). Bialké et al.[109] did find a positive interfacial stiffness together with a negative interfacial tension: this result again shows that many relations one knows to hold in equilibrium do not carry over to nonequilibrium systems. Note that in thermal equilibrium the interfacial free energy can be defined mechanically (interfacial stress is defined as an excess term computed from the stress tensor[Citation110]) or thermodynamically (e.g. considering the variation of the free energy with interfacial area[Citation110]), and both definitions must yield the same result. This agreement is lost in active systems (see, e.g. [Citation95]), where the mechanical route (which is still well defined) yields a negative interfacial tension. Hence, alternative definitions of interfacial tension are still a matter of debate (e.g. [Citation93, Citation111, Citation112]), since a uniquely defined free energy does not exist for active systems.
A description related to these issues is the so-called “active model B+ “ and the “cluster phases” following from it.[Citation113] In the classification of dynamic critical phenomena, [Citation40] “model B” describes critical dynamics of binary mixtures where only the concentration is conserved. Ref.[Citation113] suggests that an active version of this model is obtained by adding suitable terms to the current that break the local time reversal symmetry. The strength of these terms is described by two coupling parameters and
, and in the space of these two parameters, regions can be observed where “reverse Ostwald ripening” for droplets or bubbles (but never for both of them) occurs, in addition to the standard (Lifshitz-Slyozov[Citation51]) Ostwald ripening (i.e., droplets coarsen with time), that is observed near equilibrium. Tjhung et al.[Citation113] suggest that in this way one can obtain a generic phase diagram for all variants of “motility induced phase separation (MIPS)” as a generalization of the standard theory of phase separation kinetics leading to thermal equilibrium.
There are very interesting attempts to develop a theoretical understanding of phase transition in active matter systems by formulating suitable analytical theories, extending (e.g. [Citation9,Citation14]) theories on the dynamics of critical phenomena [Citation38,Citation39] or of the kinetics of transitions caused by quenching experiments.[Citation35,Citation42,Citation48] In this tutorial perspective article, we have not attempted to describe them for several reasons: (i) lack of expertise of the authors; (ii) basic assumptions are sometimes not consistent with features of the specific models that we describe in Sec. II: e.g., in [Citation14] it is assured that the vectorial degrees of freedom corresponding to the orientations of the active particles are fast degrees of freedom and hence there is the dynamics sufficiently described by the continuity equation for the density , and the current vanishes in homogeneous phases.[Citation14] We feel, however, that in such a description interesting phenomena (such as seen in ) are disregarded from the outset. (iii) While the extensions of Cahn-Hilliard theory [Citation35,Citation48] to transitions in active matter clearly render a mathematically very elegant description, [Citation14] we recall that for equilibrium systems many predictions of Cahn-Hilliard theory (initial exponential growth of unstable fluctuations, critical-like singularities at the spinodal, etc.) are misleading [Citation35,Citation50] apart from fluids with extremely weak and extremely long range attractive forces.[Citation47,Citation49] We have also not described attempts to obtain an approximate mapping of active systems to passive ones [Citation10] where integral equation theories are used to derive effective forces between the active particles from the observed pair correlations; we again expect that such approaches are of limited usefulness. A very interesting subject that we could not cover here are phase transitions of active nematics [Citation114] and active polymer networks, [Citation115] which may play a role in biological contexts.
Thus, many open questions exist in the field; we hope that the present article helps to formulate such questions more precisely, and stimulates to develop further models and concepts for their analysis.
Acknowledgments
The authors had the privilege of a stimulating collaboration with S. K. Das, F. Dittrich, S. A. Egorov, F. Schmid, J. T. Siebert, T. Speck and B. Trefz in some preceding original work [10, 12, 16]. It is a pleasure to thank them for many insightful discussions, and for permitting them to reproduce some figures. PV gratefully acknowledges the financial support by the Deutsche Forschungsgemeinschaft within priority program SPP 1726 (Grant VI 237/5-2). The authors gratefully acknowledge the computing time granted on the supercomputer Mogon at Johannes Gutenberg University Mainz (hpc.uni-mainz.de).
Additional information
Funding
References
- Gompper, G.; Winkler, R. G.; Speck, T.; Solon, A.; Nardini, C.; Peruani, F.; Löwen, H.; Golestanian, R.; Kaupp, U. B.; Alvarez, L.;, et al. The 2020 motile active matter roadmap. J. Phys. Condens. Matter. 2020. 32(19). 193001. DOI: https://doi.org/10.1088/1361-648X/ab6348
- Vicsek, T.; Czirok, A.; Ben-Jacob, E.; Cohen, I.; Shochet, O. Novel Type of Phase Transition in a System of Self-Driven Particles. Phys. Rev. Lett. 1995, 75(6), 1226. DOI: https://doi.org/10.1103/PhysRevLett.75.1226.
- Toner, J.; Wu, Y. Long-Range Order in a Two-Dimensional Dynamical XY Model: How Birds Fly Together. Phys. Rev. Lett. 1995, 75(23), 4326. DOI: https://doi.org/10.1103/PhysRevLett.75.4326.
- Czirok, A.; Stanley, H. E.; Vicsek, T. Spontaneously Ordered Motion of Self-propelled Particles. J. Phys. A: Math. Gen. 1997, 30(5), 1375. DOI: https://doi.org/10.1088/0305-4470/30/5/009.
- Czirok, A.; Vicsek, T. Collective Behavior of Interacting Self-propelled Particles. Physica A: Statistical Mechanics and Its Applications. 2000, 281(1–4), 17. DOI: https://doi.org/10.1016/S0378-4371(00)00013-3.
- Peruani, F.; Starniss, J.; Jakovljevic, V.; Sogaard-Andersen, L.; Deutsch, A.; Bär, M. Collective Motion and Nonequilibrium Cluster Formation in Colonies of Gliding Bacteria. Phys. Rev. Lett. 2012, 108(9), 098102. DOI: https://doi.org/10.1103/PhysRevLett.108.098102.
- McCandlish, S. R.; Baskaran, A.; Hagan, M. F. Spontaneous Segregation of Self-propelled Particles with Different Motilities. Soft Matter. 2012, 8(8), 2524. DOI: https://doi.org/10.1039/c2sm06960a.
- Redner, G. S.; Baskaran, A.; Hagan, M. F. Reentrant Phase Behavior in Active Colloids with Attraction. Phys. Rev. E. 2012, 88(1), 012305. DOI: https://doi.org/10.1103/PhysRevE.88.012305.
- Cates, M. E.; Tailleur, J. Motility-Induced Phase Separation. Annual Revs. Condens. Matter Phys. 2015, 6(1), 219. DOI: https://doi.org/10.1146/annurev-conmatphys-031214-014710.
- Trefz, B.; Das, S. K.; Egorov, S. A.; Virnau, P.; Binder, K. Activity Mediated Phase Separation: Can We Understand Phase Behavior of the Nonequilibrium Problem from an Equilibrium Approach? J. Chem. Phys. 2016, 144(14), 144902. DOI: https://doi.org/10.1063/1.4945365.
- Das, S. K.;. Pattern, Growth, and Aging in Aggregation Kinetics of a Vicsek-like Active Matter Model. J. Chem. Phys. 2017, 146(4), 044902. DOI: https://doi.org/10.1063/1.4974256.
- Trefz, B.; Siebert, J. T.; Speck, T.; Virnau, P.; Binder, K. Estimation of the Critical Behavior in an Active Colloidal System with Vicsek-like Interactions. J. Chem Phys. 2016, 146(7), 074901. DOI: https://doi.org/10.1063/1.4975812.
- Levis, D.; Codina, J.; Pagonabarraga, I. Active Brownian Equation of State: Metastability and Phase Coexistence. Soft Matter. 2017, 13(44), 8113. DOI: https://doi.org/10.1039/C7SM01504F.
- Solon, A. P.; Stenhammar, J.; Cates, M. E.; Kafri, Y.; Tailleur, J. Generalized Thermodynamics of Motility-induced Phase Separation: Phase Equilibria, Laplace Pressure, and Change of Ensembles. New J. Phys. 2018, 20(7), 075001. DOI: https://doi.org/10.1088/1367-2630/aaccdd.
- Siebert, J. T.; Letz, J.; Speck, T.; Virnau, P. Phase Behavior of Active Brownian Disks, Spheres, and Dimers. Soft Matter. 2017, 13(5), 1020. DOI: https://doi.org/10.1039/C6SM02622B.
- Siebert, J. T.; Dittrich, F.; Schmid, F.; Binder, K.; Speck, T.; Virnau, P. Critical Behavior of Active Brownian Particles. Phys. Rev E. 2018, 98(3), 030601. DOI: https://doi.org/10.1103/PhysRevE.98.030601.
- Mandal, S.; Liebchen, B.; Löwen, H. Motility-Induced Temperature Difference in Coexisting Phases. Phys. Rev. Lett. 2019, 123(22), 228001. DOI: https://doi.org/10.1103/PhysRevLett.123.228001.
- Caprini, L.; Marconi, U. M. B.; Puglisi, A. Spontaneous Velocity Alignment in Motility-Induced Phase Separation. Phys. Rev. Lett. 2020, 124(7), 078001. DOI: https://doi.org/10.1103/PhysRevLett.124.078001.
- Stanley, H. E.;. An Introduction to Phase Transition and Critical Phenomena; Oxford University Press: Oxford, 1971.
- Fisher, M. E.;. The Renormalization Group in the Theory of Critical Behavior. Rev. Mod. Phys. 1974, 46(4), 597. DOI: https://doi.org/10.1103/RevModPhys.46.597.
- Yeomans, J.;. Statistical Mechanics of Phase Transitions; Oxford University Press: Oxford, 1992.
- Stanley, H. E.;. Scaling, Universality, and Renormalization: Three Pillars of Modern Critical Phenomena. Rev. Mod. Phys. 1999, 71(2), S385. DOI: https://doi.org/10.1103/RevModPhys.71.S358.
- Zinn-Justin, J.;. Precise Determination of Critical Exponents and Equation of State by Field Theory Methods. Physics Reports. 2001, 344(4–6), 179. DOI: https://doi.org/10.1016/S0370-1573(00)00126-5.
- Binder, K.; Luijten, E. Monte Carlo Tests of Renormalization-group Predictions for Critical Phenomena in Ising Models. Physics Reports. 2001, 344(4–6), 179. DOI: https://doi.org/10.1016/S0370-1573(00)00127-7.
- Pelisetto, E.; Vicari, E. Critical Phenomena and Renormalization-group Theory. Physics Reports. 2002, 368(6), 549. DOI: https://doi.org/10.1016/S0370-1573(02)00219-3.
- Privman, V.; Hohenberg, P. C.; Aharony, A. Phase Transitions in Critical Phenomena. eds. Domb, C., Lebowitz, J. L. Academic Press: New York, 1991, Vol. 14; pp 1.
- Sengers, J. V.;. Phase Transitions-Cargese 1980; eds., Levy, M., Le Guillou, J.-C., Zinn-Justin, J. Academic Press: New York, 1982 95.
- Stinchcombe, R. B.;. Phase Transitions and Critical Phenomena, Vol. 7; eds., Domb, C., Lebowitz, J. L. Academic Press: New York, 1983 150.
- Binder, K.; Young, A. P. Spin glasses - experimental facts, theoretical concepts, and open questions. Rev. Mod. Phys. 58, 1986, 801.
- Fytas, N. G.; Martin-Mayor, V. Universality in the Three-Dimensional Random-Field Ising Model. Phys. Rev. Lett. 2013, 110(22), 227201. DOI: https://doi.org/10.1103/PhysRevLett.110.227201.
- Binder, K.; Heermann, D. W. Monte Carlo Simulation in Statistical Physics: An Introduction. 6th Ed; Springer: Berlin, 2019.
- Landau, D. P.; Binder, K. A Guide to Monte Carlo Simulation in Statistical Physics, 4th ed.; Cambridge Univ. Press: Cambridge, 2015.
- Frenkel, D.; Smit, B. Understanding Molecular Simulation. From Algorithms to Applications, 2nd ed.; Academic Press: San Diego, 2002.
- Allen, M. P.; Tildesley, D. Computer Simulation of Liquids, 2nd ed.; Oxford: Oxford University Press, 2017.
- Binder, K.;. Theory of First-order Phase Transitions. Rep. Progr. Phys. 1987, 50(7), 783. DOI: https://doi.org/10.1088/0034-4885/50/7/001.
- Binder, K.; Block, B.; Das, S. K.; Virnau, P.; Winter, D. Monte Carlo Methods for Estimating Interfacial Free Energies and Line Tensions. J. Stat. Phys. 2011, 144(3), 690. DOI: https://doi.org/10.1007/s10955-011-0226-7.
- Wang, X.; Binder, K.; Chen, C.; Koop, T.; Pöschl, U.; Su, H.; Cheng, Y. Second Inflection Point of Water Surface Tension in the Deeply Supercooled Regime Revealed by Entropy Anomaly and Surface Structure Using Molecular Dynamics Simulations. Physical Chemistry Chemical Physics (PCCP). 2019, 21(6), 3360. DOI: https://doi.org/10.1039/C8CP05997G.
- Hohenberg, P. C.; Halperin, B. I. Theory of Dynamic Critical Phenomena. Rev. Mod. Phys. 1977, 49(3), 435. DOI: https://doi.org/10.1103/RevModPhys.49.435.
- Onuki, A.;. Phase Transition Dynamics; Cambridge Univ. Press.: Cambridge, 2002.
- Landau, L. D.; Lifshitz, E. M. Fluid Mechanics; Pergamon Press: London, 1959.
- Kawasaki, K.;. Phase Transitions and Critical Phenomena, Vo. 5a; Domb, C., Green, M. S. eds.; Academic Press: New York, 1976.
- Puri, S.; Wadhavan, V., eds. Kinetics of Phase Transitions; CRC Press: Boca Raton, 2009.
- Zettlemoyer, A. C.;, ed. Nucleation; Marcel Dekker: New York, 1969.
- Abraham, F. F.;. Homogeneous Nucleation Theory; Academic Press: New York, 1974.
- Binder, K.; Stauffer, D. Statistical Theory of Nucleation, Condensation and Coagulation. Adv. Phys. 1976, 25(4), 343. DOI: https://doi.org/10.1080/00018737600101402.
- Kashchiev, D.;. Nucleation: Basic Theory with Applications; Butterworth, Heinemann: Oxford, 2000.
- Gunton, J. D.; San Miguel, M.; Sahni, P. S. Phase Transitions and Critical Phenomena; Domb, C., Lebowitz, J. L., eds.; Academic Press: New York, Vol. 8, 1983.
- Binder, K.; Fratzl, P. Phase Transformations in Materials, chap. 6; Kostorz, G. Ed; Wiley-VCH: Weinheim and New York, 2001.
- Penrose, O.; Lebowitz, J. L. Rigorous Treatment of Metastable States in the Van Der Waals-Maxwell Theory. J. Stat. Phys. 1971, 3(2), 211. DOI: https://doi.org/10.1007/BF01019851.
- Binder, K.; Block, B. J.; Virnau, P.; Tröster, A. Beyond the Van Der Waals Loop: What Can Be Learned from Simulating Lennard-Jones Fluids inside the Region of Phase Coexistence. Am. J. Phys. 2012, 80(12), 1099. DOI: https://doi.org/10.1119/1.4754020.
- Lifshitz, I. M.; Slyozov, V. V. The Kinetics of Precipitation from Supersaturated Solid Solutions. J. Phys. Chem. Solids. 1961, 19(1–2), 35. DOI: https://doi.org/10.1016/0022-3697(61)90054-3.
- Chakraborty, S.; Das, S. K.; Binder, K. Relaxation in a Phase-separating Two-dimensional Active Matter System with Alignment Interaction. J. Chem. Phys. 2020, 153(4), 044905. DOI: https://doi.org/10.1063/5.0010043.
- Onuki, A.; Kawasaki, K. Nonequilibrium Steady State of Critical Fluids under Shear Flow: A Renormalization Group Approach. Ann. Phys NY. 1979, 121(1–2), 456. DOI: https://doi.org/10.1016/0003-4916(79)90105-2
- Flory, P. J.;. Principles of Polymer Chemistry; Cornell University Press: Ithaca, 1953.
- Rangel-Nafaile, C.; Metzner, A. B.; Wissburn, K. F. Analysis of Stress-induced Phase Separations in Polymer Solutions. Macromolecules. 1984, 17(6), 1187. DOI: https://doi.org/10.1021/ma00136a015.
- Katz, S.; Lebowitz, J. L.; Spohn, H. Nonequilibrium Steady States of Stochastic Lattice Gas Models of Fast Ionic Conductors. J. Stat. Phys. 1984, 34(3–4), 497. DOI: https://doi.org/10.1007/BF01018556.
- Marro, J.; Dickman, R. Nonequilibrium Phase Transitions in Lattice Models; Cambridge University Press: Cambridge, 1999.
- Kawasaki, K.;. Phase Transitions and Critical Phenomena, Vol 2; Domb, C., Green, M. S., eds.; New York: Academic Press, 1972; pp 2.
- Salomon, M. B.;. Physics of Superionic Conductors; Springer: Berlin, 1972.
- Caracciolo, S.; Gambassi, A.; Gubinelli, M.; Pelisetto, A. Finite-Size Scaling in the Driven Lattice Gas. J. Stat. Phys. 2004, 115(1/2), 281. DOI: https://doi.org/10.1023/B:JOSS.0000019824.34397.1d.
- Binder, K.;. Computational Methods in Field Theory; Gausterer, H., Lang, C. B. eds.; Springer: Berlin, 1992.
- Binder, K.; Wang, J. S. Finite-size Effects at Critical Points with Anisotropic Correlations: Phenomenological Scaling Theory and Monte Carlo Simulations. J. Stat. Phys. 1989, 55(1–2), 87. DOI: https://doi.org/10.1007/BF01042592.
- Leung, K.-T.;. Finite-size Scaling of Driven Diffusive Systems: Theory and Monte Carlo Studies. Phys. Rev. Lett. 1991, 66(4), 543. DOI: https://doi.org/10.1103/PhysRevLett.66.453.
- Whitehead, J. A., Jr.;. Fluctuations, Instabilities and Phase Transitions; ed, Riste, T. Plenum Press: New York, 1975 153.
- Ahlers, G.;. Fluctuations, Instabilities and Phase Transitions; ed., Riste, T. Plenum Press: New York, 1975 181.
- Graham, R.;. Fluctuations, Instabilities and Phase Transitions; ed., Riste, T. Plenum Press: New York, 1975 215.
- Lübeck, S.;. Universal Scaling Behavior of Non-equilibrium Phase Transitions Int. J. Mod. Phys. B. 2004, 18(31n32), 3977. DOI: https://doi.org/10.1142/S0217979204027748.
- Chandrasekhar, C.;. Hydrodynamics and Hydrodynamic Stability; Oxford: Clarendon Press, 1961.
- Asakura, S.; Osawa, F.On Interaction between Two Bodies Immersed in a Solution of Macromolecules. J. Chem. Phys. 1954, 22(7), 1255. DOI: https://doi.org/10.1063/1.1740347.
- Poon, W. C. K.;. The physics of a model colloid-polymer mixture. J. Phys.: Condens. Matter. 2002, 14, R859.
- Lekkerkerker, H. N. W.; Tuinier, R. Colloids and the Depletion Interaction; Springer: Dordrecht, 2011.
- Binder, K.; Virnau, P.; Statt, A. Perspective: The Asakura Oosawa model: A colloid prototype for bulk and interfacial phase behavior. J. Chem. Phys. 2014, 141,140901.
- Elgeti, J.; Winkler, R. G.; Gompper, G. Physics of Microswimmers—single Particle Motion and Collective Behavior: A Review. Rep. Progr. Phys. 2015, 78(5), 056601. DOI: https://doi.org/10.1088/0034-4885/78/5/056601.
- Bechinger, C.; DiLeonardo, R.; Löwen, H.; Reichhardt, C.; Volpe, G.; Volpe, G. Active Particles in Complex and Crowded Environments. Ref. Mod. Phys. 2016, 88(4), 045006. DOI: https://doi.org/10.1103/RevModPhys.88.045006.
- Kurzthaler, C.; Devailly, C.; Aolt, J.; Franosch, T.; Poon, W. C. K.; Martinez, V. A.; Brown, A. J. Probing the Spatiotemporal Dynamics of Catalytic Janus Particles with Single-Particle Tracking and Differential Dynamic Microscopy. Phys. Rev. Lett. 2018, 121(7), 078001. DOI: https://doi.org/10.1103/PhysRevLett.121.078001.
- Tailleur, J.; Cates, M. E. Statistical Mechanics of Interacting Run-and-Tumble Bacteria. Physical Review Letters. 2008, 100(21), 218103. DOI: https://doi.org/10.1103/PhysRevLett.100.218103.
- Perrish, J. K.; Leah, E.-K. Complexity, Pattern, and Evolutionary Trade-Offs in Animal Aggregation. Science. 1999, 284(5411), 99. DOI: https://doi.org/10.1126/science.284.5411.99.
- Buttinoni, I.; Bialké, J.; Kümmel, F.; Löwen, H.; Bechinger, C.; Speck, T. Dynamical Clustering and Phase Separation in Suspensions of Self-Propelled Colloidal Particles. Phys. Rev. Lett. 2013, 110(23), 238301. DOI: https://doi.org/10.1103/PhysRevLett.110.238301.
- Narayan, V.; Ramaswamy, S.; Menon, N. Long-Lived Giant Number Fluctuations in a Swarming Granular Nematic. Science. 2007, 317(5834), 105. DOI: https://doi.org/10.1126/science.1140414.
- Tu, Y.; Ulm, M.; Toner, J. Sound waves and the absence of Galilean invariance in flocks. Phys. Rev. Lett., 1998, 4819.
- Mermin, N. D.; Wagner, H. Absence of Ferromagnetism or Antiferromagnetism in One- or Two-Dimensional Isotropic Heisenberg Models. Phys. Rev. Lett. 1966, 17(22), 1133. DOI: https://doi.org/10.1103/PhysRevLett.17.1133.
- Bialké, J.; Speck, T.; Löwen, H. Crystallization in a Dense Suspension of Self-Propelled Particles. Phys. Rev. Lett. 2012, 19(16), 168301. DOI: https://doi.org/10.1103/PhysRevLett.108.168301.
- Fily, Y.; Marchetti, M. C. Athermal Phase Separation of Self-Propelled Particles with No Alignment. Phys. Rev. Lett. 2012, 108(23), 235702. DOI: https://doi.org/10.1103/PhysRevLett.108.235702.
- Wysocki, A.; Winkler, R. G.; Gompper, G. Cooperative Motion of Active Brownian Spheres in Three-dimensional Dense Suspensions. EPL (Europhysics Letters). 2014, 105(4), 48004. DOI: https://doi.org/10.1209/0295-5075/105/48004.
- Romanczuk, P.; Bär, M.; Ebeling, W.; Lindner, B.; Schimansky-Geier, L. Eur. Phys. J.: Spec. Top. 202, 2012, 1.
- Winkler, R. G.; Wysocki, A.; Gompper, G. Virial Pressure in Systems of Spherical Active Brownian Particles. Soft Matter. 2015, 11(33), 6680. DOI: https://doi.org/10.1039/C5SM01412C.
- Poon, W. C. K.; Pusey, P. N. Observation, Prediction and Simulation of Phase Transitions in Complex Fluids; edited by, Baus, M., Rull, L. F., Ryckaert, J.-P. Kluwer Akad. Publ.: Dordrecht, 1995 1.
- Snook, I. K.;. The Langevin and Generalized Langevin Approach to the Dynamics of Atomic, Polymeric and Colloidal Systems; Elsevier: Amsterdam, 2017.
- Halperin, B. I.; Nelson, D. R. Theory of Two-Dimensional Melting. Phys. Rev. Lett. 1978, 41(7), 519. DOI: https://doi.org/10.1103/PhysRevLett.41.519.
- Kapfer, S. C.; Krauth, W. Two-Dimensional Melting: From Liquid-Hexatic Coexistence to Continuous Transitions. Phys. Rev. Lett. 2015, 114(3), 035702. DOI: https://doi.org/10.1103/PhysRevLett.114.035702.
- Zázvorka, J.; Dittrich, F.; Ge, Y.; Kerber, N.; Raab, K.; Winkler, T.; Litzius, K.; Veis, M.; Virnau, P.; Kläui, M. Skyrmion Lattice Phases in Thin Film Multilayer. Adv. Funct. Mat. 2020, 30(46), 2004037. DOI: https://doi.org/10.1002/adfm.202004037.
- Weeks, J. D.; Chandler, D.; Andersen, H. C. Role of Repulsive Forces in Determining the Equilibrium Structure of Simple Liquids. J. Chem. Phys. 1971, 55(12), 5297. DOI: https://doi.org/10.1063/1.1674820.
- Caporusso, C. B.; Digregorio, P.; Levis, D.; Cugliandolo, L. F.; Gonnella, G. Motility-Induced Microphase and Macrophase Separation in a Two-Dimensional Active Brownian Particle System. Phys. Rev. Lett. 2020, 125(17), 178004. DOI: https://doi.org/10.1103/PhysRevLett.125.178004.
- Poncet, A.; Bénichou, O.; Démery, V.; Nishiguchi, D. Pair Correlation of Dilute Active Brownian Particles: From Low-activity Dipolar Correction to High-activity Algebraic Depletion Wings. Phys. Rev. E. 2021, 103(1), 012605. DOI: https://doi.org/10.1103/PhysRevE.103.012605.
- Speck, T.;. Coexistence of Active Brownian Disks: Van Der Waals Theory and Analytical Results. Phys. Rev.E. 2021, 103(1), 012607. DOI: https://doi.org/10.1103/PhysRevE.103.012607.
- Vink, R. L. C.; Horbach, J. Grand Canonical Monte Carlo Simulation of a Model Colloid–polymer Mixture: Coexistence Line, Critical Behavior, and Interfacial Tension. J. Chem. Phys. 2004, 121(7), 3253. DOI: https://doi.org/10.1063/1.1773771.
- Zausch, J.; Virnau, P.; Binder, K.; Horbach, J.; Vink, R. L. C. Statics and Dynamics of Colloid-polymer Mixtures near Their Critical Point of Phase Separation: A Computer Simulation Study of A Continuous Asakura–Oosawa Model. J. Chem. Phys. 2009, 130(6), 064906. DOI: https://doi.org/10.1063/1.3071197.
- Turci, F.; Wilding, N. B. Phase Separation and Multibody Effects in Three-Dimensional Active Brownian Particles. Phys.Rev.Lett. 2021, 126(3), 038002. DOI: https://doi.org/10.1103/PhysRevLett.126.038002.
- Digregorio, P.; Levis, D.; Suma, A.; Cugliandolo, L. F.; Gonella, G.; Pagonabarraga, I. Full Phase Diagram of Active Brownian Disks: From Melting to Motility-Induced Phase Separation. Phys.Rev. Lett. 2018, 121(9), 098003. DOI: https://doi.org/10.1103/PhysRevLett.121.098003.
- Li, X.-W.; Ciamarrro, M. P. Attraction Tames Two-Dimensional Melting: From Continuous to Discontinuous Transitions. Phys. Rev. Lett. 2020, 124(21), 218002. DOI: https://doi.org/10.1103/PhysRevLett.124.218002.
- Binder, K.;. Finite Size Scaling Analysis of Ising Model Block Distribution Functions. Zeitschrift F�r Physik B Condensed Matter. 1981, 43(2), 119. DOI: https://doi.org/10.1007/BF01293604.
- Kim, Y. C.; Fisher, M. E.; Luijten, E. Precise Simulation of Near-Critical Fluid Coexistence. Phys. Rev. Lett. 2003, 91(6), 065701. DOI: https://doi.org/10.1103/PhysRevLett.91.065701.
- Wilding, N. B.;. Simulation studies of fluid critical behaviour. J. Phys.: Condens. Matter. 1997, 9, 585.
- Rovere, M.; Nielaba, P.; Binder, K. Simulation Studies of Gas-liquid Transitions in Two Dimensions via a Subsystem-block-density Distribution Analysis. Z. Phys. B. 1993, 90(2), 215. DOI: https://doi.org/10.1007/BF02198158.
- Partridge, B.; Lee, C. F. Critical Motility-Induced Phase Separation Belongs to the Ising Universality Class. Phys. Rev. Lett. 2019, 123(6), 068002. DOI: https://doi.org/10.1103/PhysRevLett.123.068002.
- Whitelam, S.; Klymko, K.; Mandal, D. Phase Separation and Large Deviations of Lattice Active Matter. J. Chem. Phys. 2018, 148(15), 154902. DOI: https://doi.org/10.1063/1.5023403.
- Dittrich, F.; Speck, T.; Virnau, P., arXiv:2010.08387, 2020.
- Maggio, C.; Paoluzzi, M.; Crisanti, A.; Zaccarelli, E.; Gnan, N., arXiv:2007.12660, 2020.
- Bialké, J.; Siebert, J. T.; Löwen, H.; Speck, T. Negative Interfacial Tension in Phase-Separated Active Brownian Particles. Phys. Rev. Lett. 2015, 115(9), 098301. DOI: https://doi.org/10.1103/PhysRevLett.115.098301.
- Rowlinson, J. S.; Widom, B. Molecular Theory of Capillarity; Clarendon Press: Oxford, 1982.
- Hermann, S.; De Las Heras, D.; Schmidt, M. D. De Las Heras, and M. Schmidt. Phys. Rev. Lett. 2019, 123(26), 268002. DOI: https://doi.org/10.1103/PhysRevLett.123.268002.
- Osnar, A. K.;. Z. -G. Wang, and J. F. Brady. Phys. Rev. E. 2020, 101, 012604.
- Tjhung, E.; Nardini, C.; Cates, M. E. Cluster Phases and Bubbly Phase Separation in Active Fluids: Reversal of the Ostwald Process. Phys. Rev. X. 2018, 8, 031080.
- Norton, M. N.; Grover, P.; Hagan, M. F.; Fraden, S. Optimal Control of Active Nematics. Phys. Rev. Lett. 2020, 125(17), 178005. DOI: https://doi.org/10.1103/PhysRevLett.125.178005.
- Gagnon, D. A.; Dessi, C.; Berezney, J. P.; Chen, D. T.-N.; L. Blair, D.; Blair, D. L.; Blair, D. L. Shear-Induced Gelation of Self-Yielding Active Networks. Phys. Rev. Lett. 2020, 125(17), 178003. DOI: https://doi.org/10.1103/PhysRevLett.125.178003.