Abstract
Using clinical and claims records from the National Emphysema Treatment Trial, we sought to identify factors that accurately predicted COPD exacerbations. This prospective cohort study consisted of subjects with severe emphysema randomized to medical therapy. Exacerbations were defined as a hospitalization or emergency department visit for COPD. Patient characteristics obtained before randomization were entered as independent variables in multivariable logistic regression models to estimate the risk of exacerbation. Discrimination was determined using the area under the receiver operator characteristic curve (AUC). Baseline measures included demographics, body mass index, pulmonary function, arterial blood gases, radiology studies, dyspnea (Shortness of Breath Questionnaire—SOBQ), health-related quality of life (St. George's Respiratory Questionnaire—SGRQ), 6-minute walk, exercise capacity, medication use, prior exacerbations and co-morbidity. In 610 participants, 26.6% had a COPD exacerbation over 1-year follow-up. In a model incorporating spirometry, PaO2, dyspnea, prior exacerbations and co-morbidity, a 5-point decrement in percent predicted FEV1 (OR 1.16, 95% CI 1.00–1.34) and a 5-point worsening in SOBQ (OR 1.08, 1.02–1.14) independently predicted exacerbations (AUC for full model 0.68). Combining physiologic variables, dyspnea, prior exacerbations and co-morbidity may be useful in identifying patients at high risk for COPD exacerbations.
INTRODUCTION
Patients with chronic obstructive pulmonary disease (COPD) may experience exacerbations that vary in severity from mild exacerbations treated as outpatients to more severe exacerbations that necessitate treatment in the emergency department or result in hospitalizations (Citation[1]). More frequent exacerbations are associated with worse clinical outcomes including a reduction in health-related quality of life (Citation[2], Citation[3]), and may be related to a more rapid decline in lung function (Citation[4]). COPD exacerbations result in over 600,000 hospitalizations in the United States, accounting for more than 70% of all COPD-related health care costs (Citation[5]).
Interventions that may decrease exacerbations and associated hospitalizations include influenza vaccination (Citation[6]) and pharmacologic treatment with long-acting bronchodilators (Citation[7]) or inhaled corticosteroids (Citation[8], Citation[9]). Comprehensive disease management programs may also decrease hospitalizations for COPD (Citation[10], Citation[11]). Therefore, identifying patients at greatest risk for exacerbations may assist in targeting interventions known to prevent exacerbations.
The risk of exacerbations among COPD patients increases among those with a forced expiratory volume in 1 second (FEV1) < 50% (Citation[1]); however, the association with lung function is not consistent (Citation[12], Citation[13]). Other clinical factors associated with an increased risk of COPD hospitalizations include muscle weakness (Citation[12]), impaired gas exchange (Citation[13]), cardiac disease (Citation[14]), self-reported physical activity (Citation[15]), dyspnea (Citation[16]), and quality of life (Citation[17]). The multidimensional BODE index incorporates FEV1, dyspnea, body mass index and 6-minute walk to predict mortality in COPD (Citation[18]). The BODE index was recently shown to be associated with risk of COPD admissions (Citation[19]).
Factors that influence mortality, however, are not necessarily the same as those that increase the risk of hospitalizations. Identifying other variables that contribute to the risk of COPD exacerbations will improve the ability of prediction models. An elevated risk, however, does not necessarily translate into accurate individual-level prediction (Citation[20]), and few studies have assessed the predictive accuracy of risk factors to discriminate between those with and without severe exacerbations, or have been able to compare several different physiologic, functional status and utilization factors in the same population.
We therefore sought to determine which components of the BODE index correctly predict severe COPD exacerbations, and whether additional physiologic, radiologic, functional status and health care utilization characteristics improve the ability to predict these exacerbations in a large sample of well-characterized outpatients with severe emphysema for whom extensive baseline data are available.
METHODS
Setting and data collection
The data for this prospective cohort study were collected as part of the National Emphysema Treatment Trial (NETT), a randomized controlled trial of lung volume reduction surgery vs. continued medical treatment conducted at 17 clinics across the United States between January 1998 and July 2002 (Citation[21]). Details of the study have been published elsewhere (Citation[21], Citation[22], Citation[23]). Briefly, all subjects enrolled in the study had emphysema with severe airflow limitation (FEV1 ≤ 45% predicted) and completed 6 to 10 weeks of pulmonary rehabilitation prior to randomization. The primary outcome measures were mortality and maximal exercise capacity two years after randomization.
During the study, information on utilization of medical care for study participants based on Medicare claims was provided by the Centers for Medicare and Medicaid Services (Citation[24]), and included inpatient and outpatient care provided by physicians during hospitalizations, emergency department visits, and outpatient clinic visits. These Medicare data were then linked to the NETT clinical trial data. Of 1,218 patients enrolled in NETT, the 610 patients randomized to medical therapy comprise the subjects for the present set of analyses.
COPD exacerbations
The primary outcome for these analyses was a COPD exacerbation that resulted in a visit to the emergency department (ED) or in a hospitalization during the first year after randomization. From Medicare claims data, we identified patients with a COPD-related ED visit or hospitalization, defined as a primary discharge diagnosis code of ICD-9 CM 491, 492, 493, or 496. These diagnosis codes have been found to accurately identify patients with obstructive lung disease compared to chart data with agreement between computer and chart diagnoses in 94.2% of cases (Citation[25]).
Potential predictors of exacerbations
We considered demographic, physiologic, functional status, radiologic, medication use and prior health care utilization as potential markers of disease severity and predictors of COPD hospitalizations and ED visits. Baseline variables were obtained following pulmonary rehabilitation and prior to randomization.
Physiologic variables
We assessed baseline pulmonary function testing measures including spirometry, lung volumes, diffusion capacity, and arterial blood gas measurements as potential predictors of exacerbations. Subjects had maximal exercise capacity on cycle ergometry measured with an increment of 5 or 10 watts per minute while breathing 30 percent oxygen. Due to gender differences in exercise capacity, sex-specific cutoff points (25W for women and 40W for men) were found to be predictors of benefit from LVRS (Citation[21]), and were used for modeling of risk of exacerbation in this analysis.
Functional status variables
Several different measures of functional status were evaluated as potential predictors of exacerbations including the 6-minute walk test (6-MW) (Citation[26]), health-related quality of life (HRQoL), and dyspnea. The 6-MW measures the maximum distance walked during 6-minutes, with a minimal clinically significant distance of approximately 50 meters (180-feet) (Citation[26], Citation[27]). COPD-specific HRQoL was measured with the St. George's Respiratory Questionnaire (SGRQ), consisting of 76 questions summarized into a total score (Citation[28]) with a range from 0 (best) to 100 (worst). Dyspnea was measured with the UCSD Shortness of Breath Questionnaire (SOBQ), a 24-item questionnaire with a total score ranging from 0 (best) to 120 (worse) (29). The minimum clinically important difference for the SOBQ is estimated to be 5-points (Citation[30]), whereas for the SGRQ score it is 4-points (Citation[31]).
Radiologic variables
Radiographic severity of emphysema was assessed both by chest radiography (CXR) and high-resolution computed tomography (CT) scans, and assessed as a potential predictor of exacerbations. CXR appearance was classified into four groups with increasing severity of hyperinflation: normal, minimal, flattened, and inverted hemi-diaphragm. The distribution of emphysema was assess by the radiologist from CT scans of the chest obtained during full inspiration using two different methods: (1) homogeneity or heterogeneity of the distribution of emphysema (Citation[23]), and (2) presence or absence of upper-lobe predominance of emphysema (Citation[21]).
Prior utilization and co-morbidity
Medicare claims data from the year prior to randomization were used to determine baseline health care utilization. All hospitalizations were identified, and COPD-specific ER visits and hospitalizations were categorized using the same ICD-9 CM discharge diagnosis codes used to define the primary outcome. Baseline co-morbidity was determined using the Deyo adaptation (Citation[32]) of the Charlson co-morbidity index (Citation[33]). Co-morbid conditions were identified from ICD-9 CM diagnosis codes from both inpatient and outpatient visits in the 12-months preceding randomization.
Other potential predictors
In addition to baseline demographic characteristics, we considered patient body mass index (BMI), as this is predictive of mortality in COPD when incorporated into the BODE index (Citation[18]). The MRC dyspnea scale was not collected as part of the NETT trial. Therefore, we calculated a modified BODE index, replacing the MRC dyspnea scale with the SOBQ divided into quartiles (e.g., lowest quartile = 0 points, highest quartile = 3 points) (Citation[34]). Patients were asked whether they were prescribed COPD-related medications, which were analyzed by class (e.g., long-or short-acting sympathomimetics, anticholinergics, inhaled corticosteroids or theophylline). All patients were asked about influenza and pneumococcus vaccination, and offered immunization if they had not already received it.
Statistical analysis
We used t-tests for continuous variables, and the χ2 statistic for categorical variables in bivariate analysis. Multivariable logistic regression was used to predict the outcome of at least one COPD hospitalization or ED visit during the 1-year follow-up period. The index date was defined as the date of randomization for the clinical trial. Candidate variables were identified using univariate logistic regression, and considered for the predictive model if significant at the p < 0.1 level. To further develop a parsimonious model, variables were selected using forward and backward stepwise selection procedures, retaining variables that were significant at the p < 0.05 level and eliminating variables with p > 0.10 using both selection methods. We assessed model fit using the Hosmer–Lemeshow goodness-of-fit test statistic (Citation[35]).
Predictive validation
To determine the ability of the model to discriminate between those who had a COPD exacerbation, and those who did not, the area under the receiver operating characteristic curve (AUC) was calculated. The probability equation for COPD exacerbation is:where βi is the model coefficient for the variable and χi is the valued of the variable for an individual patient. The AUC corresponds to the c statistic, and whether a randomly selected subject with a COPD exacerbation within 1 year has a greater predicted probability than a randomly selected subject who did not have an exacerbation. The AUC ranges from 0 to 1, with 0.5 indicating chance prediction, and 1 perfect prediction. An AUC of 0.7–0.8 is considered acceptable, and values of 0.8–0.9 excellent (Citation[36]). To compare the predictive ability of the models, the AUC and associated standard error and 95% confidence intervals were compared, using the method of DeLong, DeLong, and Clarke-Pearson for correlated data (Citation[37]). STATA (Version 8) statistical software was used for all analyses.
The performance of the predictive model in the sample from which it was developed is known often to be too optimistic due to over-fitting, and we therefore assessed internal predictive validity using bootstrap methods which enables the use of all the data in the analysis compared to alternate strategies to avoid over-fitting such as splitting the sample into a development and validation set (Citation[38]). The predictive model was first developed using all the observations in the dataset. Random samples, with replacement, were then taken 200 times from the original data. With each bootstrap sample, the predictive model was re-estimated and the coefficients used to determine the AUC in the original dataset. As a result, a correction (shrinkage) factor for the AUC can be calculated to adjust for the over-optimism in the model calibration (Citation[39]).
All continuous variables except for FEV1 were found to be approximately linear terms and entered as linear terms. For FEV1, adding a quadratic term did not significantly improve the predictive ability of the model (AUC: 0.7045 vs. 0.6954, p = 0.38). Because there was little difference in the performance of the AUC whether or not the quadratic term was included in the model, only results for models using the linear term for FEV1 are presented. For each functional status measure, the estimated OR corresponding to the minimum clinical important difference is presented (i.e., 5-point change in SOBQ, 4-point change in SGRQ and a 180-ft change in 6-MW).
RESULTS
This analysis includes 610 patients randomized to medical therapy regardless of subsequent course of treatment. The mean age of patients was 66.5 (SD ± 5.9), and 64% were male (). Nearly all patients had received both the influenza and pneumococcal vaccinations at baseline (> 90% for either immunization). Based on claims data for the year preceding randomization, 34.6% of patients had a Deyo–Charlson co-morbidity score ≥ 1. During the 1-year baseline period, 24.4% of patients had been either hospitalized or seen in the ED for a COPD exacerbation, and 19.2% required hospitalization.
Table 1 Baseline characteristics
Patients' functional status was limited as measured by 6-minute walk (mean 6-MW, 1210 ± 316 ft), health-related quality-of-life (mean SGRQ total score, 53.6 ± 12.7) and dyspnea (mean SOBQ score 63.4 ± 18.5) (). Patients had severe impairment in pulmonary function with a mean FEV1 of 0.78 L (± 0.24), and by GOLD criteria 32.3% would be classified as severe and 67.7% very severe. Nearly 22% of patients had resting hypoxemia by arterial blood gas measurement, and based on criteria from the NETT trial 44.6% are classified as having low exercise capacity ().
Table 2 Baseline physiologic variables
During the follow-up period, 20.8% of patients were hospitalized at least once for COPD and 26.6% of patients had either a hospitalization or ED visit for COPD. During the same 1-year period, 25 patients are known to have undergone lung-volume reduction surgery (LVRS) outside of the NETT study (4 subsequently died the same year), 3 had a lung transplant, and 1-year mortality was 7.5%. One additional patient had non-NETT LVRS but it is unknown if this occurred during the 1-year follow-up period. Of the 70 patients who had LVRS, lung transplantation and/or died, 42% had a COPD exacerbation before surgery or death during the follow-up period.
Unadjusted analysis showed that FEV1 divided into tertiles was significantly associated with exacerbation-free survival (). Similarly, the UCSD Shortness of Breath Questionnaire (SOBQ) and the SGRQ divided into tertiles were also associated with exacerbations over the 1-year follow-up period (). The modified BODE score was associated with an increased risk of COPD exacerbations (OR for a 1-point increase in score 1.27, 95% CI 1.14, 1.42). However the ability of the BODE to discriminate between patients with and without a severe exacerbation was modest (AUC of 0.62).
Figure 1 Kaplan–Meier COPD exacerbation-free survival estimates, by baseline spirometry and functional status (in tertiles).
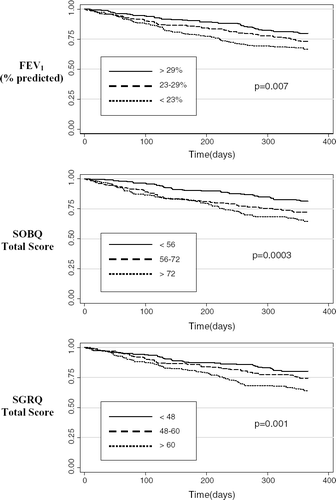
Development of the predictive model
The results of univariate logistic regression models revealed that 15 candidate variables were associated with ≥ 1 COPD hospitalization or ER visit during the 1-year follow-up period at the p < 0.1 level: female gender, COPD medication use, pulmonary function measures (FEV1, FVC and DLCO), resting hypoxemia, low exercise capacity, worse functional status (6-minute walk, SGRQ and SOBQ scores), prior utilization, and co-morbidity (). These variables were therefore evaluated as potential predictors in the multivariable predictive model.
Table 3 Candidate variables identified from univariate logistic regression to predict a COPD hospitalization or emergency department visit during the 1-year follow-up period
Both forward and backwards stepwise selection methods produced the same final model incorporating 6 independent variables: FEV1, PaO2, SOBQ score, COPD hospitalization or ER visit in the year prior to randomization, and co-morbidity (). In the multivariable model, spirometry and dyspnea were independent measures of disease severity to predict exacerbations. A 5-point change in predicted FEV1 (e.g., 30% to 25%) is associated with an increased risk of an exacerbation (OR 1.15, 95% CI 1.00–1.34). In the same model, a 5-point higher dyspnea score (indicating more severe dyspnea) measured with the SOBQ total score is also associated with exacerbations (OR 1.07, 1.02–1.14) ().
Table 4 Final multivariate logistic regression models comparing 3 different functional status measures to predict a COPD hospitalization or emergency department visit during the 1-year follow-up period
The discriminatory ability of the final model was determined using the area under the receiver operator characteristic (AUC) curves for COPD exacerbation during the 1-year follow-up period, with an AUC for the final model of 0.70. Using bootstrap methods to assess internal predictive validity, the AUC after correction for the optimism in the original dataset was 0.68 ().
Since the SOBQ may not be as widely used as other measures of functional status, we repeated the analysis substituting the SGRQ total score and the 6-minute walk instead of the SOBQ in the final model to determine whether these measures combined with the other variables similarly predicted exacerbations (). In both of these models, the estimated coefficient for FEV1 was similar whether the SGRQ or 6-MW was substituted for the SOBQ. In the model with SGRQ total score instead of SOBQ score, there was an increased risk of exacerbation with each 4-point increase (worsening) in HRQOL (OR 1.07, 1.01–1.14). Prediction of exacerbation using the SGRQ was similar to the SOBQ (AUC 0.69 vs. 0.70, p = 0.9), and after correction for optimism using bootstrap validation, the AUC was 0.67.
When the distance walked in 6 minutes was entered into the model instead of SOBQ score, a decrease in 6-MW of 180-feet was associated with an increased point-estimate for the risk of exacerbation (OR 1.08, 0.96–1.21), however this did not reach statistical significance (p = 0.19). Discrimination between those with and without exacerbations using the 6-MW was similar to a model with the SOBQ (AUC 0.69 vs. 0.70, p = 0.33), with a corrected AUC of 0.67 ().
The predictive ability of the final model using the SOBQ was significantly better than using FEV1 alone (AUC in original dataset: 0.70 vs. 0.59, p = 0.0001), and also better than prior COPD hospitalizations and/or ER visits alone (AUC 0.70 vs. 0.61, p < 0.02). When compared to the modified BODE score alone, the final model had better predictive ability (AUC 0.70 vs. 0.62, p = 0.002). Using only physiologic and functional status variables (FEV1, PaO2 and SOBQ scores) to predict COPD exacerbations had only modest predictive ability (validated AUC 0.63).
DISCUSSION
We found that in a well-characterized prospective cohort of patients with severe COPD, hypoxemia, prior utilization, and co-morbidity significantly improved the ability to predict exacerbations compared to the BODE index alone. Similar to the BODE index, we found that physiologic variables and functional status independently predict COPD exacerbations, however BMI and the 6-MW were not predictive of 1-year exacerbations in a model including FEV1 and dyspnea measured with the SOBQ.
Pulmonary function, generally measured with FEV1, and functional status are two measures of disease severity in COPD that are independently predictive of adverse outcomes such as mortality (Citation[40], Citation[41]) and exacerbations (Citation[15], Citation[16], Citation[42], Citation[43]). A recent study suggests that the multidimensional BODE index, originally developed to predict mortality (Citation[18]), also predicts COPD hospitalizations (Citation[19]). We similarly found that the BODE score predicted 1-year hospitalizations and ED visits for COPD, but the predictive accuracy (AUC 0.62) was lower than previously seen for mortality (AUC 0.74) (Citation[18]). Unlike the BODE index, we did not find that 6-MW or BMI independently predicted exacerbations in a model already including a measure of dyspnea. This may reflect the difference in COPD patients enrolled in the NETT study but suggests that predictors of exacerbations may differ from those of mortality.
We found that among functional status measures, dyspnea measured with the SOBQ was the most predictive of exacerbations. The SGRQ or the 6-MW are different measures of functional status used to assess patients with emphysema, and we were able to compare these measures of functional status to predict COPD exacerbations. Using either self-reported dyspnea measured with the SOBQ scale or disease-specific health-related quality of life with the SGRQ had a similar predictive ability to for COPD exacerbations with a validated AUC of 0.67–0.68. Using 6-minute walk instead of the SOBQ also had similar discrimination, although it did not reach statistical significance in the adjusted model.
In the unadjusted analysis, baseline use of oral corticosteroids and methylxanthines were associated with an increased risk of exacerbations, suggesting that these may be surrogate markers for patients with more severe or more symptomatic disease. In the final adjusted model that included physiologic and functional status measures, these medications were no longer associated with exacerbations, indicating that the other factors in the model adequately adjusted for disease severity in this population.
All patients in this study also all had extensive physiologic testing and radiographic examinations at baseline. Demographic factors such as age or gender, and radiographic measures of emphysema severity were not associated with the development of COPD exacerbations. Unlike previous studies we did not find that hypercapnea was associated with exacerbations (Citation[13]), although patients with severe hypercapnea (pCO2 > 60) were not included in this study. We did find that hypoxemia was predictive, consistent with a prior prospective study (Citation[15]). Similar to other studies, prior utilization (Citation[15]) and co-morbidity (Citation[14], Citation[16]) were associated with exacerbations.
A major strength of this study is the large sample of well-characterized patients with clearly defined emphysema followed prospectively and for whom nearly complete data on health care utilization was available. As a result, complete baseline data was available for all patients for spirometry, blood gases, exercise testing, radiology studies, functional status, and baseline health care utilization, providing a unique opportunity to compare these factors in the same population.
Importantly, we also were able to assess predictive validity by calculating the AUC, a measure that summarizes the ability of the model to discriminate between individuals with and without a COPD hospitalization or ED visit. Furthermore, to ensure that we did not overfit the model we performed prospective validation using bootstrap techniques, a strategy that has advantages over spitting the sample into development and validation sets and uses all the data in the sample (Citation[38]). In addition, because all patients were required to be non-smokers and were offered both pneumococcal and influenza vaccinations, there was less potential for confounding based on these factors.
A limitation of using data collected as part of a clinical trial is that the results may be less generalizeable to all patients with severe COPD. Patients in this analysis had severe or very severe airflow limitation by GOLD criteria, hyperinflation, emphysema on high resolution computed tomography and did not have significant co-morbidity. Patients with mild COPD at low risk for exacerbations were not included, and thus the model may have less ability to predict exacerbations in such patients. A benefit of this study population was to minimize misclassification of patients with asthma and co-morbid disease such as congestive heart failure, allowing us to focus primarily on predictors related to COPD.
COPD exacerbations were ascertained using administrative data, and although this is a commonly used approach (Citation[1]), it identifies more severe exacerbations than those based on symptoms or medication use. Also, criteria for hospitalization may vary depending on provider factors, and there may be misclassification of COPD hospitalizations. We addressed this issue by combining ER visits and hospitalizations due to COPD to focus on exacerbations severe enough to prompt the patient to present for emergency care.
In conclusion, in addition to the components of the BODE index, hypoxemia, co-morbidity, and prior COPD hospitalizations or emergency room visits are also independently associated with COPD exacerbations. In patients with severe COPD, a model incorporating physiologic variables, functional status, prior COPD utilization and co-morbidity predicts exacerbations, and may be useful in identifying patients at high risk for COPD exacerbations that result in emergency department visits or hospitalizations.
*Members of the NETT Research Group:
Office of the Chair of the Steering Committee, University of Pennsylvania, Philadelphia, PA: Alfred P. Fishman, MD (Chair); Betsy Ann Bozzarello; Ameena Al-Amin.
Clinical centers
Baylor College of Medicine, Houston, TX: Marcia Katz, MD (Principal Investigator); Carolyn Wheeler, RN, BSN (Principal Clinic Coordinator); Elaine Baker, RRT, RPFT; Peter Barnard, PhD, RPFT; Phil Cagle, MD; James Carter, MD; Sophia Chatziioannou, MD; Karla Conejo-Gonzales; Kimberly Dubose, RRT; John Haddad, MD; David Hicks, RRT, RPFT; Neal Kleiman, MD; Mary Milburn-Barnes, CRTT; Chinh Nguyen, RPFT; Michael Reardon, MD; Joseph Reeves-Viets, MD; Steven Sax, MD; Amir Sharafkhaneh, MD; Owen Wilson, PhD; Christine Young PT; Rafael Espada, MD (Principal Investigator 1996–2002); Rose Butanda (1999–2001); Minnie Ellisor (2002); Pamela Fox, MD (1999–2001); Katherine Hale, MD (1998–2000); Everett Hood, RPFT (1998–2000); Amy Jahn (1998–2000); Satish Jhingran, MD (1998–2001); Karen King, RPFT (1998–1999); Charles Miller III, PhD (1996–1999); Imran Nizami, MD (Co-Principal Investigator, 2000–2001); Todd Officer (1998–2000); Jeannie Ricketts (1998–2000); Joe Rodarte, MD (Co-Principal Investigator 1996–2000); Robert Teague, MD (Co-Principal Investigator 1999–2000); Kedren Williams (1998–1999).
Brigham and Women's Hospital, Boston, MA: John Reilly, MD (Principal Investigator); David Sugarbaker, MD (Co-Principal Investigator); Carol Fanning, RRT (Principal Clinic Coordinator); Simon Body, MD; Sabine Duffy, MD; Vladmir Formanek, MD; Anne Fuhlbrigge, MD; Philip Hartigan, MD; Sarah Hooper, EP; Andetta Hunsaker, MD; Francine Jacobson, MD; Marilyn Moy, MD; Susan Peterson, RRT; Roger Russell, MD; Diane Saunders; Scott Swanson, MD (Co-Principal Investigator, 1996–2001).
Cedars-Sinai Medical Center, Los Angeles, CA: Rob McKenna, MD (Principal Investigator); Zab Mohsenifar, MD (Co-Principal Investigator); Carol Geaga, RN (Principal Clinic Coordinator); Manmohan Biring, MD; Susan Clark, RN, MN; Jennifer Cutler, MD; Robert Frantz, MD; Peter Julien, MD; Michael Lewis, MD; Jennifer Minkoff-Rau, MSW; Valentina Yegyan, BS, CPFT; Milton Joyner, BA (1996–2002).
Cleveland Clinic Foundation, Cleveland, OH: Malcolm DeCamp, MD (Principal Investigator); James Stoller, MD (Co-Principal Investigator); Yvonne Meli, RNC (Principal Clinic Coordinator); John Apostolakis, MD; Darryl Atwell, MD; Jeffrey Chapman, MD; Pierre DeVilliers, MD; Raed Dweik, MD; Erik Kraenzler, MD; Rosemary Lann, LISW; Nancy Kurokawa, RRT, CPFT; Scott Marlow, RRT; Kevin McCarthy, RCPT; Pricilla McCreight, RRT, CPFT; Atul Mehta, MD; Moulay Meziane, MD; Omar Minai, MD; Mindi Steiger, RRT; Kenneth White, RPFT; Janet Maurer, MD (Principal Investigator, 1996–2001); Terri Durr, RN (2000–2001); Charles Hearn, DO (1998–2001); Susan Lubell, PA-C (1999–2000); Peter O'Donovan, MD (1998–2003); Robert Schilz, DO (1998–2002).
Columbia University, New York, NY in consortium with Long Island Jewish Medical Center, New Hyde Park, NY: Mark Ginsburg, MD (Principal Investigator); Byron Thomashow, MD (Co-Principal Investigator); Patricia Jellen, MSN, RN (Principal Clinic Coordinator); John Austin, MD; Matthew Bartels, MD; Yahya Berkmen, MD; Patricia Berkoski, MS, RRT (Site coordinator, LIJ); Frances Brogan, MSN, RN; Amy Chong, BS, CRT; Glenda DeMercado, BSN; Angela DiMango, MD; Sandy Do, MS, PT; Bessie Kachulis, MD; Arfa Khan, MD; Berend Mets, MD; Mitchell O'Shea, BS, RT, CPFT; Gregory Pearson, MD; Leonard Rossoff, MD; Steven Scharf, MD, PhD (Co-Principal Investigator, 1998–2002); Maria Shiau, MD; Paul Simonelli, MD; Kim Stavrolakes, MS, PT; Donna Tsang, BS; Denise Vilotijevic, MS, PT; Chun Yip, MD; Mike Mantinaos, MD (1998–2001); Kerri McKeon, BS, RRT, RN (1998–1999); Jacqueline Pfeffer, MPH, PT (1997–2002).
Duke University Medical Center, Durham, NC: Neil MacIntyre, MD (Principal Investigator); R. Duane Davis, MD (Co-Principal Investigator); John Howe, RN (Principal Clinic Coordinator); R. Edward Coleman, MD; Rebecca Crouch, RPT; Dora Greene; Katherine Grichnik, MD; David Harpole, Jr., MD; Abby Krichman, RRT; Brian Lawlor, RRT; Holman McAdams, MD; John Plankeel, MD; Susan Rinaldo-Gallo, MED; Sheila Shearer, RRT; Jeanne Smith, ACSW; Mark Stafford-Smith, MD; Victor Tapson, MD; Mark Steele, MD (1998–1999); Jennifer Norten, MD (1998–1999).
Mayo Foundation, Rochester, MN: James Utz, MD (Principal Investigator); Claude Deschamps, MD (Co-Principal Investigator); Kathy Mieras, CCRP (Principal Clinic Coordinator); Martin Abel, MD; Mark Allen, MD; Deb Andrist, RN; Gregory Aughenbaugh, MD; Sharon Bendel, RN; Eric Edell, MD; Marlene Edgar; Bonnie Edwards; Beth Elliot, MD; James Garrett, RRT; Delmar Gillespie, MD; Judd Gurney, MD; Boleyn Hammel; Karen Hanson, RRT; Lori Hanson, RRT; Gordon Harms, MD; June Hart; Thomas Hartman, MD; Robert Hyatt, MD; Eric Jensen, MD; Nicole Jenson, RRT; Sanjay Kalra, MD; Philip Karsell, MD; Jennifer Lamb; David Midthun, MD; Carl Mottram, RRT; Stephen Swensen, MD; Anne-Marie Sykes, MD; Karen Taylor; Norman Torres, MD; Rolf Hubmayr, MD (1998–2000); Daniel Miller, MD (1999–2002); Sara Bartling, RN (1998–2000); Kris Bradt (1998–2002).
National Jewish Medical and Research Center, Denver, CO: Barry Make, MD (Principal Investigator); Marvin Pomerantz, MD (Co-Principal Investigator); Mary Gilmartin, RN, RRT (Principal Clinic Coordinator); Joyce Canterbury; Martin Carlos; Phyllis Dibbern, PT; Enrique Fernandez, MD; Lisa Geyman, MSPT; Connie Hudson; David Lynch, MD; John Newell, MD; Robert Quaife, MD; Jennifer Propst, RN; Cynthia Raymond, MS; Jane Whalen-Price, PT; Kathy Winner, OTR; Martin Zamora, MD; Reuben Cherniack, MD (Principal Investigator, 1997–2000).
Ohio State University, Columbus, OH: Philip Diaz, MD (Principal Investigator); Patrick Ross, MD (Co-Principal Investigator); Tina Bees (Principal Clinic Coordinator); Jan Drake; Charles Emery, PhD; Mark Gerhardt, MD, PhD; Mark King, MD; David Rittinger; Mahasti Rittinger.
Saint Louis University, Saint Louis, MO: Keith Naunheim, MD (Principal Investigator); Robert Gerber, MD (Co-Principal Investigator); Joan Osterloh, RN, MSN (Principal Clinic Coordinator); Susan Borosh; Willard Chamberlain, DO; Sally Frese; Alan Hibbit; Mary Ellen Kleinhenz, MD; Gregg Ruppel; Cary Stolar, MD; Janice Willey; Francisco Alvarez, MD (Co-Principal Investigator, 1999–2002); Cesar Keller, MD (Co-Principal Investigator, 1996–2000).
Temple University, Philadelphia, PA: Gerard Criner, MD (Principal Investigator); Satoshi Furukawa, MD (Co-Principal Investigator); Anne Marie Kuzma, RN, MSN (Principal Clinic Coordinator); Roger Barnette, MD; Neil Brister, MD; Kevin Carney, RN, CCTC; Wissam Chatila, MD; Francis Cordova, MD; Gilbert D'Alonzo, DO; Michael Keresztury, MD; Karen Kirsch; Chul Kwak, MD; Kathy Lautensack, RN, BSN; Madelina Lorenzon, CPFT; Ubaldo Martin, MD; Peter Rising, MS; Scott Schartel, MD; John Travaline, MD; Gwendolyn Vance, RN, CCTC; Phillip Boiselle, MD (1997–2000); Gerald O'Brien, MD (1997–2000).
University of California, San Diego, San Diego, CA: Andrew Ries, MD, MPH (Principal Investigator); Robert Kaplan, PhD (Co-Principal Investigator); Catherine Ramirez, BS, RCP (Principal Clinic Coordinator); David Frankville, MD; Paul Friedman, MD; James Harrell, MD; Jeffery Johnson; David Kapelanski, MD; David Kupferberg, MD, MPH; Catherine Larsen, MPH; Trina Limberg, RRT; Michael Magliocca, RN, CNP; Frank J. Papatheofanis, MD, PhD; Dawn Sassi-Dambron, RN; Melissa Weeks.
University of Maryland at Baltimore, Baltimore, MD in consortium with Johns Hopkins Hospital, Baltimore, MD: Mark Krasna, MD (Principal Investigator); Henry Fessler, MD (Co-Principal Investigator); Iris Moskowitz (Principal Clinic Coordinator); Timothy Gilbert, MD; Jonathan Orens, MD; Steven Scharf, MD, PhD; David Shade; Stanley Siegelman, MD; Kenneth Silver, MD; Clarence Weir; Charles White, MD.
University of Michigan, Ann Arbor, MI: Fernando Martinez, MD (Principal Investigator); Mark Iannettoni, MD (Co-Principal Investigator); Catherine Meldrum, BSN, RN, CCRN (Principal Clinic Coordinator); William Bria, MD; Kelly Campbell; Paul Christensen, MD; Kevin Flaherty, MD; Steven Gay, MD; Paramjit Gill, RN; Paul Kazanjian, MD; Ella Kazerooni, MD; Vivian Knieper; Tammy Ojo, MD; Lewis Poole; Leslie Quint, MD; Paul Rysso; Thomas Sisson, MD; Mercedes True; Brian Woodcock, MD; Lori Zaremba, RN.
University of Pennsylvania, Philadelphia, PA: Larry Kaiser, MD (Principal Investigator); John Hansen-Flaschen, MD (Co-Principal Investigator); Mary Louise Dempsey, BSN, RN (Principal Clinic Coordinator); Abass Alavi, MD; Theresa Alcorn, Selim Arcasoy, MD; Judith Aronchick, MD; Stanley Aukberg, MD; Bryan Benedict, RRT; Susan Craemer, BS, RRT, CPFT; Ron Daniele, MD; Jeffrey Edelman, MD; Warren Gefter, MD; Laura Kotler-Klein, MSS; Robert Kotloff, MD; David Lipson, MD; Wallace Miller, Jr., MD; Richard O'Connell, RPFT; Staci Opelman, MSW; Harold Palevsky, MD; William Russell, RPFT; Heather Sheaffer, MSW; Rodney Simcox, BSRT, RRT; Susanne Snedeker, RRT, CPFT; Jennifer Stone-Wynne, MSW; Gregory Tino, MD; Peter Wahl; James Walter, RPFT; Patricia Ward; David Zisman, MD; James Mendez, MSN, CRNP (1997–2001); Angela Wurster, MSN, CRNP (1997–1999).
University of Pittsburgh, Pittsburgh, PA: Frank Sciurba, MD (Principal Investigator); James Luketich, MD (Co-Principal Investigator); Colleen Witt, MS (Principal Clinic Coordinator); Gerald Ayres; Michael Donahoe, MD; Carl Fuhrman, MD; Robert Hoffman, MD; Joan Lacomis, MD; Joan Sexton; William Slivka; Diane Strollo, MD; Erin Sullivan, MD; Tomeka Simon; Catherine Wrona, RN, BSN; Gerene Bauldoff, RN, MSN (1997–2000); Manuel Brown, MD (1997–2002); Elisabeth George, RN, MSN (Principal Clinic Coordinator 1997–2001); Robert Keenan, MD (Co-Principal Investigator 1997–2000); Theodore Kopp, MS (1997–1999); Laurie Silfies (1997–2001).
University of Washington, Seattle, WA: Joshua Benditt, MD (Principal Investigator), Douglas Wood, MD (Co-Principal Investigator); Margaret Snyder, MN (Principal Clinic Coordinator); Kymberley Anable; Nancy Battaglia; Louie Boitano; Andrew Bowdle, MD; Leighton Chan, MD; Cindy Chwalik; Bruce Culver, MD; Thurman Gillespy, MD; David Godwin, MD; Jeanne Hoffman; Andra Ibrahim, MD; Diane Lockhart; Stephen Marglin, MD; Kenneth Martay, MD; Patricia McDowell; Donald Oxorn, MD; Liz Roessler; Michelle Toshima; Susan Golden (1998–2000).
Other participants
Agency for Healthcare Research and Quality, Rockville, MD: Lynn Bosco, MD, MPH; Yen-Pin Chiang, PhD; Carolyn Clancy, MD; Harry Handelsman, DO.
Centers for Medicare and Medicaid Services, Baltimore, MD: Steven M Berkowitz, PhD; Tanisha Carino, PhD; Joe Chin, MD; JoAnna Baldwin; Karen McVearry; Anthony Norris; Sarah Shirey; Claudette Sikora; Steven Sheingold, PhD (1997–2004).
Coordinating Center, The Johns Hopkins University, Baltimore, MD: Steven Piantadosi, MD, PhD (Principal Investigator); James Tonascia, PhD (Co-Principal Investigator); Patricia Belt; Amanda Blackford, ScM; Karen Collins; Betty Collison; Ryan Colvin, MPH; John Dodge; Michele Donithan, MHS; Vera Edmonds; Gregory L. Foster, MA; Julie Fuller; Judith Harle; Rosetta Jackson; Shing Lee, ScM; Charlene Levine; Hope Livingston; Jill Meinert; Jennifer Meyers; Deborah Nowakowski; Kapreena Owens; Shangqian Qi, MD; Michael Smith; Brett Simon, MD; Paul Smith; Alice Sternberg, ScM; Mark Van Natta, MHS; Laura Wilson, ScM; Robert Wise, MD.
Cost Effectiveness Subcommittee: Robert M. Kaplan, PhD (Chair); J. Sanford Schwartz, MD (Co-Chair); Yen-Pin Chiang, PhD; Marianne C. Fahs, PhD; A. Mark Fendrick, MD; Alan J. Moskowitz, MD; Dev Pathak, PhD; Scott Ramsey, MD, PhD; Steven Sheingold, PhD; A. Laurie Shroyer, PhD; Judith Wagner, PhD; Roger Yusen, MD.
Cost Effectiveness Data Center, Fred Hutchinson Cancer Research Center, Seattle, WA: Scott Ramsey, MD, PhD (Principal Investigator); Ruth Etzioni, PhD; Sean Sullivan, PhD; Douglas Wood, MD; Thomas Schroeder, MA; Karma Kreizenbeck; Kristin Berry, MS; Nadia Howlader, MS.
CT Scan Image Storage and Analysis Center, University of Iowa, Iowa City, IA: Eric Hoffman, PhD (Principal Investigator); Janice Cook-Granroth, BS; Angela Delsing, RT; Junfeng Guo, PhD; Geoffrey McLennan, MD; Brian Mullan, MD; Chris Piker, BS; Joseph Reinhardt, PhD; Blake Robinswood; Jered Sieren, RTR; William Stanford, MD.
Data and Safety Monitoring Board: John A. Waldhausen, MD (Chair); Gordon Bernard, MD; David DeMets, PhD; Mark Ferguson, MD; Eddie Hoover, MD; Robert Levine, MD; Donald Mahler, MD; A. John McSweeny, PhD; Jeanine Wiener-Kronish, MD; O. Dale Williams, PhD; Magdy Younes, MD.
Marketing Center, Temple University, Philadelphia, PA: Gerard Criner, MD (Principal Investigator); Charles Soltoff, MBA.
Project Office, National Heart, Lung, and Blood Institute, Bethesda, MD: Gail Weinmann, MD (Project Officer); Joanne Deshler (Contracting Officer); Dean Follmann, PhD; James Kiley, PhD; Margaret Wu, PhD (1996-2001).
Other acknowledgments
Arthur Gelb, MD, Lakewood Regional Medical Center, Lakewood, CA.
The research reported here was supported by the Department of Veteran Affairs, Health Services Research and Development Service Grants RCD 02-170-2 and by the NETT Coordinating Center. The National Emphysema Treatment Trial (NETT) is supported by contracts with the National Heart, Lung, and Blood Institute (N01HR76101, N01HR76102, N01HR76103, N01HR76104, N01HR76105, N01HR76106, N01HR76107, N01HR76108, N01HR76109, N01HR76110, N01HR76111, N01HR76112, N01HR76113, N01HR76114, N01HR76115, N01HR76116, N01HR76118, and N01HR76119), the Centers for Medicare and Medicaid Services (CMS; formerly the Health Care Financing Administration), and the Agency for Healthcare Research and Quality (AHRQ).
Note: The views expressed in this article are those of the authors and do not necessarily represent the views of the Department of Veterans Affairs.
The authors would like to acknowledge the contribution of Janne Abullarade to the preparation of the data set for analysis.
REFERENCES
- Burge S, Wedzicha J A. COPD exacerbations: definitions and classifications. Eur Respir J Suppl 2003; 41: 46s–53s
- Seemungal T A, Donaldson G C, Paul E A, Bestall J C, Jeffries D J, Wedzicha J A. Effect of exacerbation on quality of life in patients with chronic obstructive pulmonary disease. Am J Respir Crit Care Med 1998; 157: 1418–1422, (5 Pt 1)
- Spencer S, Calverley P M, Burge P S, Jones P W. Impact of preventing exacerbations on deterioration of health status in COPD. Eur Respir J 2004; 23(5)698–702
- Donaldson G C, Seemungal T A, Bhowmik A, Wedzicha J A. Relationship between exacerbation frequency and lung function decline in chronic obstructive pulmonary disease. Thorax 2002; 57(10)847–852
- Sullivan S D, Ramsey S D, Lee T A. The economic burden of COPD. Chest 2000; 117: 5S–9S, (2 Suppl)
- Nichol K L, Baken L, Nelson A. Relation between influenza vaccination and outpatient visits, hospitalization, and mortality in elderly persons with chronic lung disease. Ann Intern Med 1999; 130(5)397–403
- Sin D D, McAlister F A, Man S F, Anthonisen N R. Contemporary management of chronic obstructive pulmonary disease: scientific review. JAMA 2003; 290(17)2301–2312
- Calverley P, Pauwels R, Vestbo J, Jones P, Pride N, Gulsvik A, et al. Combined salmeterol and fluticasone in the treatment of chronic obstructive pulmonary disease: a randomized controlled trial. Lancet 2003; 361(9356)449–456
- Szafranski W, Cukier A, Ramirez A, Menga G, Sansores R, Nahabedian S, et al. Efficacy and safety of budesonide/formoterol in the management of chronic obstructive pulmonary disease. Eur Respir J 2003; 21(1)74–81
- Bourbeau J, Julien M, Maltais F, Rouleau M, Beaupre A, Begin R, et al. Reduction of hospital utilization in patients with chronic obstructive pulmonary disease: a disease-specific self-management intervention. Arch Intern Med 2003; 163(5)585–591
- Casas A, Troosters T, Garcia-Aymerich J, Roca J, Hernandez C, Alonso A, et al. Integrated care prevents hospitalizations for exacerbations in COPD patients. Eur Respir J 2006
- Decramer M, Gosselink R, Troosters T, Verschueren M, Evers G. Muscle weakness is related to utilization of health care resources in COPD patients. Eur Respir J 1997; 10(2)417–423
- Kessler R, Faller M, Fourgaut G, Mennecier B, Weitzenblum E. Predictive factors of hospitalization for acute exacerbation in a series of 64 patients with chronic obstructive pulmonary disease. Am J Respir Crit Care Med 1999; 159(1)158–164
- Miravitlles M, Guerrero T, Mayordomo C, Sanchez-Agudo L, Nicolau F, Segu J L. Factors associated with increased risk of exacerbation and hospital admission in a cohort of ambulatory COPD patients: a multiple logistic regression analysis. The EOLO Study Group. Respiration 2000; 67(5)495–501
- Garcia-Aymerich J, Farrero E, Felez M A, Izquierdo J, Marrades R M, Anto J M. Risk factors of readmission to hospital for a COPD exacerbation: a prospective study. Thorax 2003; 58(2)100–105
- Oostenbrink J B, Rutten-van Molken M P. Resource use and risk factors in high-cost exacerbations of COPD. Respir Med 2004; 98(9)883–891
- Gudmundsson G, Gislason T, Janson C, Lindberg E, Hallin R, Ulrik C S, et al. Risk factors for rehospitalization in COPD: role of health status, anxiety and depression. Eur Respir J 2005; 26(3)414–419
- Celli B R, Cote C G, Marin J M, Casanova C, Montes de Oca M, Mendez R A, et al. The body-mass index, airflow obstruction, dyspnea, and exercise capacity index in chronic obstructive pulmonary disease. N Engl J Med 2004; 350(10)1005–1012
- Ong K C, Earnest A, Lu S J. A multidimensional grading system (BODE index) as predictor of hospitalization for COPD. Chest 2005; 128(6)3810–3806
- Pepe M S, Janes H, Longton G, Leisenring W, Newcomb P. Limitations of the odds ratio in gauging the performance of a diagnostic, prognostic, or screening marker. Am J Epidemiol 2004; 159(9)882–890
- Fishman A, Martinez F, Naunheim K, Piantadosi S, Wise R, Ries A, et al. A randomized trial comparing lung-volume-reduction surgery with medical therapy for severe emphysema. N Engl J Med 2003; 348(21)2059–2073
- Rationale and design of the National Emphysema Treatment Trial (NETT). A prospective randomized trial of lung volume reduction surgery. J Thorac Cardiovasc Surg 1999; 118(3)518–528
- National Emphysema Treatment Trial Research Group. Patients at high risk of death after lung-volume-reduction surgery. N Engl J Med 2001; 345(15)1075–1083
- Ramsey S D, Berry K, Etzioni R, Kaplan R M, Sullivan S D, Wood D E. Cost effectiveness of lung-volume-reduction surgery for patients with severe emphysema. N Engl J Med 2003; 348(21)2092–2102
- Rawson N S, Malcolm E. Validity of the recording of ischaemic heart disease and chronic obstructive pulmonary disease in the Saskatchewan health care datafiles. Stat Med 1995; 14(24)2627–2643
- Redelmeier D A, Bayoumi A M, Goldstein R S, Guyatt G H. Interpreting small differences in functional status: the Six Minute Walk test in chronic lung disease patients. Am J Respir Crit Care Med 1997; 155(4)1278–1282
- Sciurba F, Criner G J, Lee S M, Mohsenifar Z, Shade D, Slivka W, et al. Six-minute walk distance in chronic obstructive pulmonary disease: reproducibility and effect of walking course layout and length. Am J Respir Crit Care Med 2003; 167(11)1522–1527
- Jones P W. A self-complete measure of health status for chronic airflow limitation: The St. George's Respiratory Questionnaire. Am Rev Respir Dis 1992; 145: 1321–1327
- Eakin E G, Resnikoff P M, Prewitt L M, Ries A L, Kaplan R M. Validation of a new dyspnea measure: the UCSD Shortness of Breath Questionnaire. University of California, San Diego. Chest 1998; 113(3)619–624
- Ries A. Minimally clinically important difference for the UCSD Shortness of Breath Questionnaire, Borg Scale, and Visual Analog Scale. J Chron Obstructive Pulm Dis 2005; 2: 105–110
- Jones P W. Interpreting thresholds for a clinically significant change in health status in asthma and COPD. Eur Respir J 2002; 19(3)398–404
- Deyo R A, Cherkin D C, Ciol M A. Adapting a clinical co-morbidity index for use with ICD-9-CM administrative databases. J Clin Epidemiol 1992; 45(6)613–619
- Charlson M E, Pompei P, Ales K L, MacKenzie C R. A new method of classifying prognostic co-morbidity in longitudinal studies: development and validation. J Chronic Dis 1987; 40(5)373–383
- Martinez F J, Foster G, Curtis J L, et al. Predictors of mortality in patients with emphysema and severe airflow obstruction. Am J Respir Crit Care Med 2006; 173: 1326–1334
- Hosmer D, Lemeshow S. Applied Logistic Regression. Wiley-Interscience, New York 1989
- Hosmer D, Lemeshow S. Applied Logistic Regression, 2nd ed. John Wiley & Sons, New York 2000
- DeLong E R, DeLong D M, Clarke-Pearson D L. Comparing the areas under two or more correlated receiver operating characteristic curves: a nonparametric approach. Biometrics 1988; 44(3)837–845
- Harrell F E, Jr., Lee K L, Mark D B. Multivariable prognostic models: issues in developing models, evaluating assumptions and adequacy, and measuring and reducing errors. Stat Med 1996; 15(4)361–387
- Efron B, Tibshirani R J. An Introduction to the Bootstrap. Chapman & Hill, Boca Raton, FL 1998
- Nishimura K, Izumi T, Tsukino M, Oga T. Dyspnea is a better predictor of 5-year survival than airway obstruction in patients with COPD. Chest 2002; 121(5)1434–1440
- Domingo-Salvany A, Lamarca R, Ferrer M, Garcia-Aymerich J, Alonso J, Felez M, et al. Health-related quality of life and mortality in male patients with chronic obstructive pulmonary disease. Am J Respir Crit Care Med 2002; 166(5)680–685
- Miravitlles M, Murio C, Guerrero T. Factors associated with relapse after ambulatory treatment of acute exacerbations of chronic bronchitis. DAFNE Study Group. Eur Respir J 2001; 17(5)928–933
- Soler J J, Sanchez L, Roman P, Martinez M A, Perpina M. Risk factors of emergency care and admissions in COPD patients with high consumption of health resources. Respir Med 2004; 98(4)318–329