Abstract
The CODEX index was developed and validated in patients hospitalized for COPD exacerbation to predict the risk of death and readmission within one year after discharge. Our study aimed to validate the CODEX index in a large external population of COPD patients with variable durations of follow-up. Additionally, we aimed to recalculate the thresholds of the CODEX index using the cutoffs of variables previously suggested in the 3CIA study (mCODEX).
Individual data on 2,755 patients included in the COPD Cohorts Collaborative International Assessment Plus (3CIA+) were explored. A further two cohorts (ESMI AND EGARPOC-2) were added. To validate the CODEX index, the relationship between mortality and the CODEX index was assessed using cumulative/dynamic ROC curves at different follow-up periods, ranging from 3 months up to 10 years. Calibration was performed using univariate and multivariate Cox proportional hazard models and Hosmer-Lemeshow test.
A total of 3,321 (87.8% males) patients were included with a mean ± SD age of 66.9 ± 10.5 years, and a median follow-up of 1,064 days (IQR 25–75% 426–1643), totaling 11,190 person-years. The CODEX index was statistically associated with mortality in the short- (≤3 months), medium- (≤1 year) and long-term (10 years), with an area under the curve of 0.72, 0.70 and 0.76, respectively. The mCODEX index performed better in the medium-term (<1 year) than the original CODEX, and similarly in the long-term.
In conclusion, CODEX and mCODEX index are good predictors of mortality in patients with COPD, regardless of disease severity or duration of follow-up.
Abbreviations | ||
COPD | = | Chronic Obstructive Pulmonary Disease |
FEV1 | = | Forced Expiratory Volume in the first second |
mMRC | = | modified dyspnea scale of the Medical Research Council |
3CIA | = | COPD Cohorts Collaborative International Assessment |
Post-BD | = | post bronchodilator |
STROBE | = | STrengthening the Reporting of OBservational studies in Epidemiology |
25–75% IQR | = | 25–75% interquartile range |
ROC | = | receiver operating characteristic curve |
AUC | = | area under the curve |
NNE | = | nearest-neighbor estimator |
ESMI | = | COPD in internal medical services |
HR | = | Hazard Ratio |
95% C.I. | = | 95% Confidence Interval |
CODEX index | = | Comorbidity, Obstruction, Dyspnea, Exacerbations |
mCODEX index | = | modified CODEX index |
BODE | = | Body mass index, Obstruction, Dyspnea, Exercise |
BODEX | = | Body mass index, Obstruction, Dyspnea, Exacerbations |
ADO | = | Age, Dyspnea, Obstruction |
HADO | = | Health, Activity, Dyspnea, Obstruction |
DOSE | = | Dyspnea, Obstruction, Smoking, Obstruction |
PEARL | = | Previous admissions, eMRCD score, Age, Right-sided heart failure, Left-sided heart failure |
Introduction
The study of prognosis has been inseparable from medical practice for centuries (Citation1). Some prognostic scores have been widely validated, such as the Karnofsky, Charlson, APACHE and other indices, while others have never been externally validated and their usefulness is debatable (Citation2–4).
The most commonly used variable for evaluating the severity and mortality risk in COPD is postbronchodilator FEV1, expressed as a percentage of predicted value according to ethnicity, age, sex and height (FEV1%). Indeed, FEV1% predicts survival, not only in respiratory patients, but also in cardiovascular disorders and even in the general population (Citation5–8). In COPD, severity of airflow limitation has been classified according to different thresholds, which have changed over time and which have been endorsed by different scientific societies (Citation9). To date, the most widely accepted classification, for the sake of simplicity and its broad implementation, is the staging proposed by the Global Obstructive Lung Disease Initiative (GOLD) to evaluate with the degree of postbronchodilator FEV1% expressed as percentage of their predicted value (≥80%; 50–79%; 30–49%; ≤ 29% for mild, moderate, severe and very severe airflow limitation, respectively), although these suggested cutoffs are slightly different from those validated for mortality in prospective cohort studies (namely ≥85%; 55–84%; 35–54%; ≤ 34%) (Citation7,Citation8). The second variable in importance for staging COPD is dyspnea, often measured with the modified scale of the Medical Research Council (mMRC), which in patients with more severe obstruction is an even better predictor of mortality than FEV1% alone (Citation10,Citation11). These two variables were historically the first prognostic variables recognized in COPD (Citation12,Citation13). Additionally, the combination of these two variables – airflow limitation and dyspnea – is the cornerstone of most of the multicomponent indices developed for COPD prognosis including BODE, BODEx, ADO, DOSE and HADO (Citation14–18). There are other important variables to evaluate prognosis in COPD, such as sub-phenotypes and the risk of exacerbations or comorbidities, among others (Citation15,Citation19).
The CODEX index was developed and validated in patients hospitalized for an acute exacerbation of COPD with the objective of evaluating the prognosis in the short- (3 months) and medium-term (1 year) for mortality, hospital readmission or their combination (Citation19). Later, it was revalidated in a small cohort of outpatients with severe COPD for mortality and exacerbations, and more recently exclusively for mortality in another retrospective study performed in a cohort of ambulatory patients (Citation20,Citation21). Finally, CODEX index was compared with other index for the combination of 90-day mortality and readmissions after a hospitalization for COPD exacerbation (Citation22). However, to date formal validation of its accuracy across a variety of COPD patient cohorts, and different follow-up periods has not been done.
Our main objective was the validation and recalculation of the CODEX index for mortality, in a broader cohort of COPD patients, recruited either at the general population, outpatient or hospital levels, with different stages of severity and with varying periods of follow-up ranging from 3 months up to 10 years.
Methods
We obtained individual pooled data from 26 cohort studies from 8 countries, all previously published, and from the COPD Cohorts Collaborative International Assessment (3CIA) consortium database, later expanded to 3CIA+ (Citation7). Briefly, the 3CIA database contains individual data from 16,332 COPD patients, at the outpatient and hospital levels, spirometrically confirmed by a post-BD ratio FEV1/FVC <0.7, according to the GOLD criteria (Citation7). The 3CIA + cohort has follow-up data and information on age, sex, pre-and post-BD FEV1, mMRC dyspnea scale and mortality, among others. Only in a number of 3CIA + cohorts were data of comorbidity measured with Charlson index and number of hospitalizations in the previous year available. For the current study, we selected exclusively those cohorts in which Charlson index and number of severe exacerbations in the previous year were available in the database, since both are required to calculate the CODEX index. CODEX index is composed of the combination of FEV1%, dyspnea and number of severe COPD exacerbations in the previous year, stratified according to the BODE and BODEX thresholds, but replacing body mass index with the original age-adjusted Charlson index, the most widely recognized prognostic index of comorbidity (Citation3). Severe exacerbations were defined as those that required hospitalization or emergency room visits (Citation6,Citation15). ()
Table 1. Variables and thresholds to estimate the CODEX and mCODEX indices
The original, age-adjusted Charlson index is a standard scale with 15 chronic diseases graded for severity, including COPD, in which one point is added to the total score of comorbidity for each decade of life over the age of 50 years (Citation3). To calculate the CODEX index, age-adjusted Charlson was stratified in tertiles, while the stratification of %predicted FEV1 and dyspnea was the same as is used in both the BODE and BODEX indices and the thresholds for exacerbations were those used in the BODEX index. In the present study, we attempted to recalculate the CODEX index (mCODEX) by replacing the original CODEX thresholds for FEV1% and dyspnea (mMRC) with the previously suggested cutoffs based on survival prediction analysis in 3CIA and ADO, which are ≥85%; 55–84%; 35–54%; <34% for FEV1% and 0-1; 2; 3 and 4 for dyspnea (Citation7,Citation8,Citation16). Thus, possible scores for the CODEX and mCODEX indices range from 0 to 10 points (Citation19) ().
Two cohorts not previously included in 3CIA+, namely ESMI and EGARPOC-2, were added. Since the CODEX index was developed using the data of the ESMI study, and in order to rule out a possible bias, a previous subanalysis was carried out to assess the AUCs of the ESMI study vs. the rest of the cohorts. ESMI and EGARPOC-2 contain all the variables included in 3CIA+, plus the Charlson index and follow-up for mortality (Citation20,Citation23). All the original cohort studies were approved by the respective ethics committees and all patients gave their informed consent. For the development of the present study, the STROBE recommendations for observational cohort studies were followed (Citation24).
Statistical analysis
Categorical variables were expressed as absolute frequencies and percentages, and continuous variables were summarized as mean and standard deviation, or median and 25–75% interquartile range (25–75% IQR), wherever appropriate. Comparisons among means were made using the Student t-test or Mann–Whitney test according to normality assumptions. For validation purposes, we used the cumulative/dynamic area under the receiver operating characteristic curve (ROC) to express the ability of both CODEX and mCODEX indices to predict all-cause mortality for short-term (0–3 months), medium-term (3–12 months) and long-term follow-up (1–10 years). Dynamic cumulative ROC curves were selected as they are considered the most appropriate method when the considered outcome (in our case mortality) is a time-dependent variable. We used the nearest-neighbor estimator (NNE) proposed by Heagerty, Lumley and Pepe to estimate the AUC, and the naïve bootstrap procedure to estimate 95% confidence intervals (95% CI) (Citation25). Detailed methodology is available elsewhere (Citation26). Calibration was performed with univariate and multivariate Cox proportional hazard models and Hosmer–Lemeshow test. Mortality curves were calculated using the Kaplan–Meier estimator. Univariate Cox proportional hazard models were used to study the crude effect of the CODEX and mCODEX tertiles on survival. A random-effect multivariable Cox proportional hazard model including sex and age was used to study the adjusted effect of the CODEX and mCODEX tertiles on survival. In order to deal with the sample heterogeneity, a Gaussian frailty term was added to both models, which were stratified by cohort (Citation25,Citation27). Finally, we explored the reliability of CODEX and mCODEX in different subgroups stratified by sex, age, FEV1(%) and dyspnea. For all analyses, we used free software R (www.r-project.org). In particular, package survival ROC and survival were used to compute the AUC indices and develop the time-dependent analysis. A two-sided p-value below 0.05 was considered statistically significant.
Results
Twelve of the 26 cohorts included in the 3CIA contain in their protocol the data necessary to calculate the CODEX index, specifically Charlson index and the number of exacerbations in the previous year, totalling 3,142 patients. Of these 3,142 patients initially included, 363 were excluded due to a lack of individual data to calculate CODEX index and 24 for missing follow-up.
These excluded patients had better lung function (mean FEV1%: 56.2% vs. 51.6%; p < 0.001) and were more often male [394/2,785 (14.3%) vs. 32/387 (8.3%); p = 0.002), with no differences on the dyspnea scale (median 2.72 vs 2.40; p = 0.381) or age (mean 66.8 vs. 67.2 years; p = 0.415). A total of 566 patients from the ESMI and EGARPOC cohorts were added to the study. () In all included patients, data to calculate the CODEX index were available, and hence we did not impute missing data.
Figure 1. Strengthening the reporting of observational studies in epidemiology (STROBE) flowchart of participants and causes of exclusion.
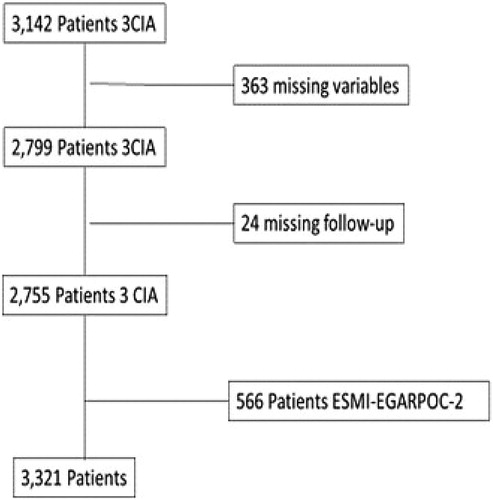
The AUC for ESMI study compared with the global cohort was nearly identical, and therefore we decided to maintain it in both the validation and recalibration cohort. (Figure S1, Supplementary material)
In sum, a total of 3,321 patients were included in the study, with a mean age of 66.9 (SD 10.5) years, and 87.8% were males. The median follow-up was 1,064 days with an interquartile range (IQR) 25–75% of 426–1,643 days, totalling an experience of 11,990 person-years. The main characteristics of the studied population are presented in , while the distribution of CODEX and mCODEX indices is detailed in . A total of 1,175 (35.4%) patients were included after a hospitalization for exacerbation of COPD, while 2,146 (64.6%) were selected in ambulatory settings. Hospitalized patients were older [72 (9.4) vs. 64.1 (10) years; p < .001], with higher scores in the Charlson index [6.8 (2.6) vs. 4.3 (2.2); p < 0.001], lower values of FEV1% [46 (17.1) vs. 53.9 (19.8); p < 0.001], higher scores in the mMRC dyspnea scale [median 3 (IQR 75%:3–4) vs. 2 (IQR 75%: 2–3); p < 0.001], and without differences for gender and severe exacerbations in the previous year. Both CODEX [5.4 (2) vs. 3.8 (2.2); p < 0.001] and mCODEX [5.7 (1.7) vs. 4.3 (1.9); p < 0.001] showed higher scores in hospitalized patients.
Figure 2. Distribution of CODEX and mCODEX in the study population. N = number of subjects for each point of CODEX and mCODEX.
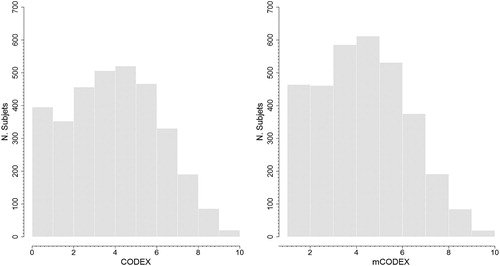
Table 2. Demographic and clinical characteristics of the study participants
The overall observed mortality ranged from 6.3% (1 year) to 20% (3 years) to 58% at 10 years. The AUC for CODEX and mortality ranged from 0.72 (95% CI: 0.60–0.77) at 3 months to 0.76 (95% CI:0.70–0.79) at 10 years, and between 0.73 (95% C.I:0.67–0.78) at 3 months and 0.75 (95% C.I.: 0.69–0.79) at 10 years in the mCODEX. The mCODEX performed slightly better, although without statistically significant differences, in the short (3 months) and medium term (1 year), and similarly in the rest of the follow-up (). Both models were well calibrated according to the Hosmer–Lemeshow test. (Table S1, Supplementary material)
Table 3. Cumulative/dynamic area under the ROC curves, and 95% confidence interval at different periods of follow-up.
shows the hazard ratios (HRs) and their respective 95% confidence intervals for crude and adjusted survival, one in which just the covariate of interest was included, and another random-effect one adjusted for age, sex and cohort. Other covariables were not included since the CODEX and mCODEX indices already contained comorbidity, obstruction, dyspnea and previous exacerbations. The HR of the highest and lowest tertiles was 4.59 (95% C.I.:3.93–4.74) and 5.02 (95% C.I.:4.17–6.05) for CODEX and mCODEX in the unadjusted model and 3.93 (95% C.I.:3.27–4.44) and 4.31 (95% C.I.: 5.54–5.26), respectively, in the adjusted model. shows the Kaplan–Meier estimates for the survival curves stratified by tertiles of CODEX and mCODEX.
Figure 3. Kaplan-Meier curves for mortality stratified in tertiles for CODEX and mCODEX. The gray shading represents the 95% confidence intervals.
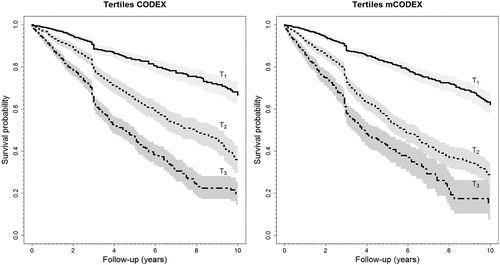
Table 4. HRs and their respective 95% confidence intervals for crude and adjusted survival.
presents the sensitivity analysis for subgroups stratified by age, gender, FEV1% and dyspnea at clinically relevant cutoffs and different follow-up periods, graphically displayed in . In these analyses, CODEX and mCODEX performed well, confirming that both indices are useful in the different population subgroups, and highlighting the high AUC in the younger patients in the short term (0.95 and 0.84 at 3 and 6 months, respectively) for CODEX and mCODEX. Inversely, the utility of both indices in women in the short and medium term (<3-years) was lower for CODEX than for mCODEX (0.66 and 0.64 vs. 0.7 and 0.71) at 3 and 12 months, respectively. Of note, the predictive capacity of CODEX and mCODEX for mortality in the short and medium term was higher in outpatients (. ).
Figure 4. (a and b). AUCs and 95% confidence intervals for mortality, stratified by relevant subgroups for (a) CODEX, and (b) mCODEX, and different periods of follow-up.
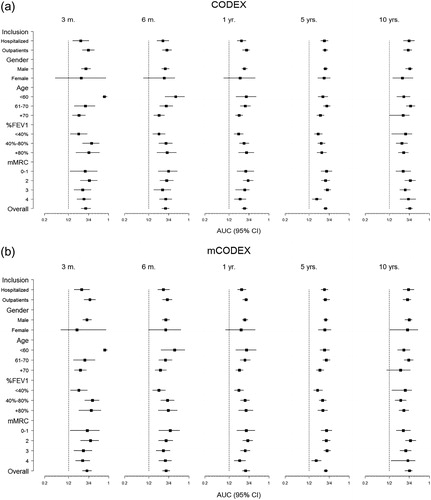
Table 5. Sensitivity analysis for subgroups and mortality, at different follow-up periods.
Discussion
Our study confirms the utility of the CODEX index to predict mortality in a large set of COPD patients. The study design – a pooled-analysis of individual patient-data from several cohorts – sample size and the different degrees of severity of the patients in the different cohorts maximize its high external validity. Importantly, these replication results were consistent in sensitivity analyses and across different COPD sub-populations.
Additionally, we recalculated the original CODEX index with different thresholds for FEV1% and dyspnea, previously obtained from the 3CIA cohort for survival prediction. Of note, these new cutoffs were similar to those found in a large-scale international validation study conducted in 10 cohorts including 13,914 patients in the validation of the ADO index (Citation7,Citation8,Citation16).
In the past few years, a number of multicomponent prognostic indices have been developed to predict progression and outcomes in COPD patients (Citation28). These scores were created by the combination of different variables with diverse thresholds, but their usefulness and reproducibility are highly variable. Some of them were created basically with statistical criteria, for others calculation is complex, some were based on literature reviews and most have never been externally validated (Citation4,Citation29,Citation30).
To date, the most frequently referenced multicomponent prognostic scale in COPD is the BODE index, originally developed in ambulatory patients with a low burden of comorbidity, and subsequently validated in other populations (Citation14,Citation31). The BODE index has also shown good sensitivity in detecting changes in the progression and outcomes of COPD, such as exacerbations, pulmonary rehabilitation, lung volume reduction techniques and lung transplantation, among others (Citation32–35). Following BODE, several other multicomponent indices have been developed and validated in different populations and with diverse objectives. The DECAF score was developed and later validated to predict in-hospital mortality in patients with COPD exacerbations, while the DOSE index was developed in primary care to evaluate the risk of exacerbations; it was later related with mortality (Citation17,Citation36–39). The ADO index has been shown to have a high discriminatory power (AUC 0.85 and 0.73 for the updated cohort and derivation cohort, respectively) and calibration for 3-year mortality, although some authors feel that the weight of age in ADO may be excessive (Citation16,Citation40). A modification of BODE is the BODEx index, which replaces the 6-minute walking test with severe exacerbations in the previous year, and which has a similar 3-year predictive value (Citation15). More recently, a new tool (PEARL score) has been developed to predict the risk of death or readmission at 90 days after hospital discharge. This study was performed in 2,417 patients included in the DECAF study who survived to discharge. PEARL score was superior to ADO, BODEX and DOSE in all three cohorts, and to CODEX within the internal and external validation cohorts, but similar in the internal validation cohort (AUC for CODEX =0.66 vs PEARL 0.68) (Citation22).
All these indices have been externally validated and even directly compared in others cohorts (Citation41,Citation42). External validation is essential to determine the reproducibility of prediction models and to explore whether predictions obtained by the model are valid in other populations (Citation43,Citation44).
CODEX index was originally developed in a multicenter cohort of patients hospitalized for COPD exacerbation, and externally validated in the original publication in three other similar cohorts (Citation19). Later, it was revalidated in two cohorts of ambulatory patients with good discrimination (AUC: 0.80) (Citation20,Citation21). This is in accordance with the data of the present study that show a higher predictive capacity in outpatients, retaining similar values of AUC to those observed in the original study for hospitalized patients.
CODEX has several strengths and some weaknesses. Among the strengths are that its variables are easy to collect, and all closely and clinically related to the prognosis of these patients, especially the impact of comorbidity measured with the original age-adapted Charlson index (Citation45). Additionally, CODEX was superior to BODEX, DOSE and updated ADO in patients hospitalized for COPD in the short and medium term (Citation19). However, to date few external validations are available, and its performance in the longer term and in other populations has not been studied. Our results confirm the ability of the CODEX index to predict mortality in a large sample of patients and across diverse COPD populations, with different degrees of severity. In COPD multicomponent indices are useful to compare the severity of the disease among different populations, and to enhance informed decision-making with the patient. However, the individual prognosis in COPD is highly variable and these models can assist clinicians but do not replace clinical judgment (Citation46).
Additionally, we attempted to improve CODEX by modifying the cutoffs for FEV1% and dyspnea with those suggested previously in 3CIA, which are very similar to those proposed by Puhan et al. in the updated ADO (Citation7,Citation8,Citation16). Updating a predictive model is often desirable, especially when the model is applied in settings that differ from that of the development sample or when investigating new thresholds of included variables if there are new data that suggest an improvement of its predictive capacity (Citation43,Citation44). This new mCODEX performed slightly better, in the short term (3-months) and medium term (1 year), and similarly in longer follow-up times. The most plausible explanation for the small differences found between CODEX and mCODEX is the small differences in the thresholds selected, confirming the reliability of the cutoffs previously selected in the BODE and BODEx index. Although these differences could have been maximized with statistical criteria their clinical applicability would be more doubtful.
Our study has several limitations. First, mortality was the only outcome assessed, while in the original publication CODEX index was related to three outcomes, namely risk of mortality, hospital admissions and their combination. Regrettably, we do not have sufficient, consistent data on 3CIA + in hospital admissions after inclusion of patients. In this sense, the present study is similar to the previous publications of prognostic indices in COPD (BODE, BODEX, ADO, etc.) that have mortality as the exclusive outcome (Citation14–16). Second, our study had a clear predominance of men. Whereas in the 3CIA + study the percentage of women was 31%, in our study after the exclusion of patients with missing data for Charlson index and previous severe exacerbations, this percentage dropped to 12%. Nevertheless, the number of women (404) was sufficient to detect differences between gender groups above or equal to 0.15 standard deviation at the standard statistical power of 80%. The rest of the differences between included and excluded cohorts are small; patients without Charlson index were slightly older with a similar number of severe exacerbations in the previous year and similar level of dyspnea. Third, there was great variability in the severity and outcomes across the individual studies included. However, this might also be considered a strength because it enabled inclusion of patients with a full range of COPD disease severity.
To conclude, our study confirms the utility of the CODEX index for mortality prediction in a large cohort of COPD patients. Its reliability was demonstrated across diverse COPD populations, in all subgroups analysed and in different periods of follow-up.
Declaration of interest
Marc Miravitlles has received speaker or consulting fees from AstraZeneca, Bial, Boehringer Ingelheim, Chiesi, Cipla, CSL Behring, Laboratorios Esteve, Gebro Pharma, GlaxoSmithKline, Grifols, Menarini, Mereo Biopharma, Novartis, pH Pharma, Rovi, TEVA, Verona Pharma and Zambon, and research grants from GlaxoSmithKline and Grifols unrelated to this manuscript Pere Almagro has received speaker or consulting fees from Chiesi, AstraZeneca, Boehringer-Ingelheim, GlaxoSmithKline, Laboratorios Esteve, Rovi, Menarini y Novartis unrelated to this manuscript.
Supplemental Material
Download PDF (219.2 KB)Acknowledgments
The COCOMICS study was funded in part by a grant from the Spanish Society of Pneumology and Thoracic surgery (SEPAR) coded with the number 057/12.SEPAR 2013. We thank Tom Yohannan (professional medical copy-editor) for his editorial assistance.
References
- Charles Joseph Singer EAU. A short history of medicine. Second ed. Oxford University Press; 1962.
- Evans CMM. Prognostic uncertainty in terminal care: can the Karnofsky index help? Lancet. 1985;1:1204–6.
- Charlson ME, Pompei P, Ales KL MC. A new method of classifying prognostic comorbidity in longitudinal studies: development and validation. J Chronic Dis. 1987;40(5):373–83.
- Salpeter SR, Luo EJ, Malter DS, Stuart B. Systematic review of noncancer presentations with a median survival of 6 months or less. Am J Med. 2012;125:512.e1–16.
- Schünemann HJ, Dorn J, Grant BJ, Winkelstein WTM Jr. Pulmonary function is a long-term predictor of mortality in the general population: 29-year follow-up of the Buffalo Health Study. Chest. 2000;118(3):656–64.
- Vogelmeier CF, Criner GJ, Martinez FJ, Anzueto A, Barnes PJ, Bourbeau J, Celli BR, Chen R, Decramer M, Fabbri LM, et al. Global Strategy for the diagnosis, management, and prevention of chronic obstructive lung disease 2017 Report. GOLD Executive Summary. Am J Respir Crit Care Med. 2017;195 (5):557–82.
- Soriano JB, Lamprecht B, Ramírez AS, Martinez-Camblor P, Kaiser B, Alfageme I, Almagro P, Casanova C, Esteban C, Soler-Cataluña JJ, et al. Mortality prediction in chronic obstructive pulmonary disease comparing the GOLD 2007 and 2011 staging systems: A pooled analysis of individual patient data. Lancet Respir Med. 2015;3(6):443–50.
- Almagro P, Martinez-Camblor P, Soriano JB, Marin JM, Alfageme I, Casanova C, Esteban C, Soler-Cataluña JJ, de-Torres JP, Celli BR, et al. Finding the best thresholds of FEV1and dyspnea to predict 5-year survival in COPD patients: The COCOMICS study. PLoS One. 2014;9(2):1–7.
- Atsou K, Chouaid C, Hejblum G. Variability of the chronic obstructive pulmonary disease key epidemiological data in Europe: systematic review. BMC Med. 2011;9:7.
- Bestall JC, Paul EA, Garrod R, Garnham R, Jones PW, Wedzicha JA. Usefulness of the Medical Research Council (MRC) dyspnoea scale as a measure of disability in patients with chronic obstructive pulmonary disease. Thorax. 1999;54(7):581–6.
- Nishimura K, Izumi T, Tsukino M, Oga T. Dyspnea is a better predictor of 5-year survival than airway obstruction in patients with COPD. Chest. 2002;121(5):1434–40.
- Jones NL, Burrows B, Fletcher CM. Serial studies of 100 patients with chronic airway obstruction in London and Chicago. Thorax. 1967; 22(4):327–35.
- Renzetti AD Jr. Prognosis in chronic obstructive pulmonary disease. Med Clin North Am. 1967;51:363–71.
- Celli BR, Cote CG, Marin JM, Montes de Oca M, Mendez RA, Pinto Plata V, Cabral HJ. The body-mass index, airflow obstruction, dyspnea, and exercise capacity index in chronic obstructive pulmonary disease. N Engl J Med. 2004;350(10):1005–12.
- Soler-Cataluña JJ, Martínez-García MÁ, Sánchez LS, Tordera MP, Sánchez PR. Severe exacerbations and BODE index: Two independent risk factors for death in male COPD patients. Respir Med. 2009;103(5):692–9.
- Puhan MA, Hansel NN, Sobradillo P, Enright P, Lange P, Hickson D, Menezes AM, Riet GT, Held U, Domingo-Salvany A, et al. Large-scale international validation of the ADO index in subjects with COPD: An individual subject data analysis of 10 cohorts. BMJ Open. 2012;2:2012–152.
- Jones RC, Donaldson GC, Chavannes NH, Kida K, Dickson-Spillmann M, Harding S, Wedzicha JA, Price D, Hyland ME. Derivation and validation of a composite index of severity in chronic obstructive pulmonary disease: the DOSE index. Am J Respir Crit Care Med. 2009;180(12):1189–95.
- Esteban C, Quintana JM, Moraza J, Aburto M, Aguirre U, Aguirregomoscorta JI, Aizpiri S, Basualdo LV, Capelastegui A. BODE-Index vs HADO-score in chronic obstructive pulmonary disease: which one to use in general practice? BMC Med. 2010;8:28.
- Almagro P, Soriano JB, Cabrera FJ, Boixeda R, Alonso-Ortiz MB, Barreiro B, Diez-Manglano J, Murio C, Heredia JL; Working Group on COPD, SpanishSociety of Internal Medicine. Short- and medium-term prognosis in patients hospitalized for COPD exacerbation: the CODEX index. Chest. 2014;145(5):972–80.
- Navarro A, Costa R, Rodriguez-Carballeira M, Yun S, Lapuente A, Barrera A, Acosta E, Viñas C, Heredia JL, Almagro P. Prognostic assessment of mortality and hospitalizations of outpatients with advanced chronic obstructive pulmonary disease. Usefulness of the CODEX index. Rev Clin Esp . 2015;215(8):431–8.
- Golpe R, Suárez-Valor M, Veres-Racamonde A, Cano-Jiménez E, Martín-Robles I, Sanjuán-López P, Pérez-de-Llano L. Octogenarian patients with chronic obstructive pulmonary disease: Characteristics and usefulness of prognostic indexes. Med Clin (Barc). 2017. [Epub ahead of print]
- Echevarria C, Steer J, Heslop-Marshall K, Stenton SC, Hickey PM, Hughes R, Wijesinghe M, Harrison RN, Steen N, Simpson AJ, et al. The PEARL score predicts 90-day readmission or death after hospitalisation for acute exacerbation of COPD. Thorax. 2017;72(8):686–93.
- Almagro P, Cabrera FJ, Diez J, Boixeda R, Alonso Ortiz MB, Murio C, Soriano JB; Working Group on, COPD, Spanish Society of Internal Medicine. Comorbidities and short-term prognosis in patients hospitalized for acute exacerbation of COPD: the EPOC en Servicios de medicina interna (ESMI) study. Chest. 2012;142(5):1126–33.
- von Elm E, Altman DG, Egger M, Pocock SJ, Gøtzsche PC, Vandenbroucke JP; STROBE Initiative. The strengthening the reporting of observational studies in epidemiology (STROBE) statement: Guidelines for reporting observational studies. Lancet. 2007;370(9596):1453–7.
- Heagerty PJ, Lumley T, Pepe MS. Time-dependent ROC curves for censored survival data and a diagnostic marker. Biometrics. 2000;56(2):337–44.
- Martínez-Camblor P, Bayón GF, Pérez-Fernández S. Cumulative/dynamic ROC curve estimation. J Stat Comput Simulat. 2016;86: 3582–94.
- O'Quigley J, Stare J. Proportional hazards models with frailties and random effects. Stat Med. 2002;21(21):3219–33.
- Dijk WD, Bemt Lv, Haak-Rongen Sv, Bischoff E, Weel Cv, Veen JC, Schermer TR. Multidimensional prognostic indices for use in COPD patient care. A systematic review. Respir Res. 2011;12:151.
- Connors AF Jr, Dawson NV, Thomas C, Harrell FE Jr, Desbiens N, Fulkerson WJ, Kussin P, Bellamy P, Goldman L, Knaus WA. Outcomes following acute exacerbation of severe chronic obstructive lung disease. The SUPPORT investigators (study to understand prognoses and preferences for outcomes and risks of treatments). Am J Respir Crit Care Med. 1996;154(4):959–67.
- Mannino D, Sin DD. Development and validation of a prognostic index for health outcomes in chronic obstructive pulmonary disease. Arch Intern Med. 2008;168(1):71–9.
- Sanjaume M, Almagro P, Rodríguez-Carballeira M, Barreiro B, Heredia JL, Garau J. Post-hospital mortality in patients re-admitted due to COPD. Utility of BODE index. Rev Clin Esp. 2009;209(8):364–70.
- Cote CG, Dordelly LJ, Celli BR. Impact of COPD exacerbations on patient-centered outcomes. Chest. 2007;131:696–704.
- Güell MR, Cejudo P, Ortega F, Puy MC, Rodríguez-Trigo G, Pijoan JI, Martinez-Indart L, Gorostiza A, Bdeir K, Celli B, Galdiz JB. Benefits of long-term pulmonary rehabilitation maintenance program in severe COPD Patients: 3 year follow-up. Am J Respir Crit Care Med. 2017;195(5):622–29.
- Imfeld S, Bloch KE, Weder W, Russi EW. The BODE index after lung volume reduction surgery correlates with survival. Chest. 2006;129(4):873–8.
- Lahzami S, Bridevaux PO, Soccal PM, Wellinger J, Robert JH, Ris HB, Aubert JD. Survival impact of lung transplantation for COPD. Eur Respir J. 2010; 36(1):74–80.
- Steer J, Gibson J, Bourke SC. The DECAF Score: predicting hospital mortality in exacerbations of chronic obstructive pulmonary disease. Thorax. 2012;67(11):970–6.
- Echevarria C, Steer J, Heslop-Marshall K, Stenton SC, Hickey PM, Hughes R, Wijesinghe M, Harrison RN, Steen N, Simpson AJ, et al. Validation of the DECAF score to predict hospital mortality in acute exacerbations of COPD. Thorax. 2016;71(2):133–40.
- Jones RC, Price D, Chavannes NH, Lee AJ, Hyland ME, Ställberg B, Lisspers K, Sundh J, van der Molen T, Tsiligianni I, et al. Multi-component assessment of chronic obstructive pulmonary disease: an evaluation of the ADO and DOSE indices and the global obstructive lung disease categories in international primary care data sets. NPJ Prim Care Respir Med. 2016;26:16010.
- Sundh J, Janson C, Lisspers K, Ställberg B, Montgomery S. The Dyspnea, Obstruction, Smoking, Exacerbation (DOSE) index is predictive of mortality in COPD. Prim Care Respir J. 2012;21(3):295–301.
- Celli BR, Marin JM, Cote CG, Aguirre A, Macario CC. Prognostic assessment of patients with COPD. Lancet. 2009;374(9705):1885.
- Marin JM, Alfageme I, Almagro P, Casanova C, Esteban C, Soler-Cataluña JJ, de Torres JP, Martínez-Camblor P, Miravitlles M, Celli BR, Soriano JB. Multicomponent indices to predict survival in COPD: the COCOMICS study. Eur Respir J. 2013;42(2):323–32.
- Ou CY, Chen CZ, Yu CH, Shiu CH, Hsiue TR. Discriminative and predictive properties of multidimensional prognostic indices of chronic obstructive pulmonary disease: a validation study in Taiwanese patients. Respirology. 2014; 19(5):581–9.
- Collins GS, de Groot JA, Dutton S, Omar O, Shanyinde M, Tajar A, Voysey M, Wharton R, Yu LM, Moons KG, Altman DG. External validation of multivariable prediction models: a systematic review of methodological conduct and reporting. BMC Med Res Methodol. 2014;14:40.
- Nieboer D, van der Ploeg T, Steyerberg EW. Assessing discriminative performance at external validation of clinical prediction models. PLoS One. 2016;11(2):e0148820.
- Donaldson GC, Wedzicha JA. The CODEX index: a collection or digest of laws: a code. Chest. 2014;145 (5):934–5.
- Almagro P, Yun S, Sangil A, Rodríguez-Carballeira M, Marine M, Landete P, Soler-Cataluña JJ, Soriano JB, Miravitlles M. Palliative care and prognosis in COPD: a systematic review with a validation cohort.Int J Chron Obstruct Pulmon Dis. 2017; 12:1005–729.
- Steyerberg EW. Prognosis Research Strategy (PROGRESS) 3: prognostic model research. PLoS Med. 2013;10(2):e1001381.