Abstract
Acute exacerbation (AE) is the main cause of increased disability and mortality for patients with Chronic Obstructive Pulmonary Disease (COPD). Short-term re-exacerbation after discharge is common for in-hospital patients with AECOPD. Thus, we aimed to design a scoring system to effectively predict the 30-day re-exacerbation using simple and easily accessible variables. We retrospectively enrolled 686 cases hospitalized for AECOPD in two Chinese hospitals from 2005 to 2017. A variety of parameters were collected like demographics, clinical manifestations and treatments in stable and AE period. The optimal subset of covariates in the multivariate logistic analysis was identified by the smallest Akaike Information Criterion (AIC) and was further used to develop a practical and reliable nomogram to predict the 30-day re-exacerbation. The efficacy of the nomogram was internally validated by concordance index (C-index) and a calibration plot. The incidence of 30-day re-exacerbation was 15.8%. Based on the smallest AIC, eight easily-accessible parameters were included in the nomogram, including sex, COPD assessment test (CAT) scores, AE with respiratory failure in the previous year, new purulent sputum, new cardiovascular events, combined antibiotic therapy, theophylline therapy for AE and ICU admission. Our nomogram revealed good discriminative ability with the C-index of 0.702. The calibration curve showed good agreement between nomogram-predicted probability and actual observation. Incorporating eight common variables, a nomogram for 30-day re-exacerbation after discharge with high predictive performance was constructed for patients with AECOPD, which was helpful in predicting individualized risk of re-exacerbation and offering individualized post-discharge support.
Introduction
Chronic Obstructive Pulmonary Disease (COPD) is a leading cause of mortality and disability worldwide and brings heavy burden to patients (Citation1,Citation2). As a main source of burden, acute exacerbation (AE) results in accelerated decline of lung function, poorer health status and increased mortality (Citation3–5). A previous clinical audit demonstrated that the rate of unscheduled re-admission within 90 days after discharge for AECOPD was as high as nearly 30%, and higher medical staff ratio and intensified management could improve prognosis (Citation6). Thus, it is necessary to identify patients susceptible to re-admission or re-exacerbation and provide them with additional medical resources.
Various predictive factors in stable and exacerbation period are reported to be associated with AE, such as age, sex, smoking status, lung function, COPD assessment test (CAT) scores, dyspnea, AE frequency in the previous year, stable maintenance medications, radiologic phenotypes, chronic comorbidities, abnormality of blood gas analysis as well as some clinical manifestations and treatments in exacerbation period (Citation5,Citation7–13). Although several scoring systems have been developed to evaluate the risk of re-exacerbation (Citation7,Citation12,Citation14), most of them aim to predict re-exacerbation risks within 3–6 months. What’s more, some variables in these systems are inaccessible and complex for many AE cases, such as lung function, limiting their applications in clinical settings more or less.
As a user-friendly graphical reflection of multivariable analysis, nomogram can provide individualized and visualized prediction of outcomes based on a combination of variables (Citation15). It can be used to calculate approximate probabilities for a specific patient, instead of assigning prognosis based on risk groups, which helps clinicians to provide specific clinical information to patients and develop individualized therapeutic and follow-up strategies. With regard to COPD, nomograms for predicting in-hospital mortality and 6-month re-exacerbation of patients with AECOPD have been recently published (Citation16,Citation17). However, the nomogram for evaluating risks of short-term AE recurrence has not been constructed yet.
Therefore, we used a retrospective cohort to construct a nomogram, aiming to provide a simple, reliable and lung function-independent tool to predict 30-day re-exacerbation for in-hospital patients with AECOPD and provided them with individualized post-discharge support.
Methods
Patient selection
We established a retrospective database for in-hospital patients with AECOPD in Shanghai Zhongshan Hospital and Shanghai Putuo District People's Hospital between 2005 and 2017.
The cases with the discharged diagnosis of AECOPD were firstly screened. Then those with recorded diagnosis of COPD and acute deterioration of respiratory symptoms were further reviewed. If deterioration of respiratory symptoms, as assessed by two separate pulmonologists, were caused by the following comorbidities, the case would be excluded: asthma, bronchiectasis, restrictive respiratory disease, pneumothorax, pleuritis, pulmonary embolism or congestive heart failure. Patients whose records of CAT scores, modified Medical Research Council (mMRC) scales and 30-day follow-up data were missing were also excluded.
The retrospective database was approved by Institutional Review Board (IRB) of Shanghai Zhongshan Hospital (Certificate No. B2015-119R) and registered on the Chinese Clinical Trial Registry website (Registry No. ChiCTR-POC-15007304). Specific informed consent related to this study was waived because of the retrospective and observational nature of the study.
Data collection
Our dataset was composed of demographic characteristics, stable COPD status at baseline, manifestations and therapies in AE period as well as follow-up data after discharge.
Age, sex and smoking history were collected as demographic characteristics.
Baseline variables prior to AE were collected by retrieving medical records and previous questionnaires, including post-bronchodilator forced expiratory volume in 1 second percent of predicted (FEV1%), mMRC questionnaire for dyspnea (Citation18) CAT score for general health status (Citation19) AE frequency and severity in the previous year. Moreover, comorbidities and stable maintenance medications were also collected.
Some parameters of exacerbation status were recorded including clinical manifestations, laboratory tests, arterial blood gas analysis, pathogen tests, chest imaging and emerging complications. Treatment options consisted of respiratory therapies and drug therapies, including antibiotics, steroids, bronchodilators, theophylline, etc.
After discharge, the re-exacerbation events and survival status were followed up at the 30th day by telephone call or outpatient department visit.
Outcome
In this study, we regarded re-exacerbation events within 30 days as the outcome of interest and the period at risks began at discharge. The definition of re-exacerbation was the emerging worsening of respiratory symptoms for no less than 2 consecutive days (Citation20), which required the intervention of medication changes, after the sustained relief of respiratory symptom deterioration of the last AE for more than 14 days (Citation21). In addition, 12 patients who died due to AE within 30 days after discharge from hospital were also included into the re-exacerbation group.
Definition
The diagnostic definition of COPD was determined as follows: (1) presentations with chronic cough and chronic sputum production, with or without dyspnea; (2) a recorded spirometry showing post-bronchodilator FEV1/FVC ratio < 0.70. The spirometric grades in were according to the Global Initiative for Chronic Obstructive Lung Disease (GOLD) report (Citation20).
Table 1. Baseline characteristics of study population.
Of the recorded variables, new cardiovascular events were defined as the emerging or worsening left cardiac insufficiency or arrhythmia, and combined antibiotic therapy was defined as simultaneous usage of no less than two kinds of antibiotics at initial treating period. Theophylline therapy for AE was defined as usage of any one of aminophylline, doxophylline and diprophylline in the AE period.
Statistical analysis and nomogram construction
The continuous variables were expressed as mean ± standard deviation and the categorical variables were described by constituent ratio. SPSS 23.0 (SPSS Inc, Chicago, IL) was used to perform the multivariate logistic analysis. Due to the interactions between variables, the variable which does not have statistically significant difference in univariate analysis may regain statistical significance in the multivariate model. Furthermore, whether every variable included in the predictive model has independently statistical differences (p < 0.05) does not directly determine the predictive performance of the model. Therefore, the smallest Akaike Information Criterion (AIC), (Citation22,Citation23) which indicated the minimal loss of prognostic information of predictive models, was applied to identify the optimal subset of parameters in the multivariate logistic analysis by a manual backward stepwise method.
The rms package (Citation24) and R version 3.1.3 (www.r-project.org) were used to develop a nomogram including the best subset of parameters to predict risks of 30-day re-exacerbation. The performance of the nomogram was evaluated by the concordance index (C-index) and the calibration plot. C-index is similar to area under receiver operating characteristic curve (AUC) and a C-index >0.7 generally reflects a well-fitted feature of the predictive model. Calibration plots were performed with 1000 bootstrapped resamples for internal validation. For the purpose of a sensitivity analysis, we also performed a calibration plot in the subpopulation with the exact record of FEV1/FVC < 0.7 during this hospitalization. The little deviation between the apparent line, the bias-corrected line and the diagonal line at 45° in a calibration plot could reflect the optimal agreement of nomogram-predicted and observed probability.
Results
Baseline characteristics
A total of 885 cases of AECOPD were enrolled in our database. After excluding 199 cases lack of records of CAT scores, mMRC scales and 30-day follow-up data, the remaining 686 cases were used for subsequent analysis (). The incidence of 30-day re-exacerbation was 15.8%. The average length of hospital stay was 12 days (interquartile range: 9–15 days).
Figure 1. Flowchart of case selection. AE: acute exacerbation; COPD: chronic obstructive pulmonary disease; CAT: COPD assessment test; mMRC: modified Medical Research Council.
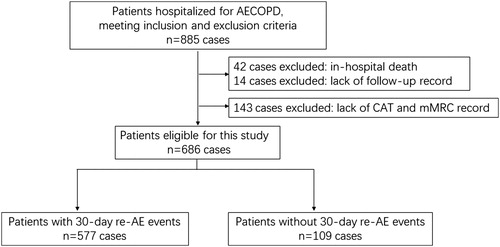
As shows, a majority of our population were classified as severe to very severe COPD grade and high-risk group (Citation20). The missing rate of FEV1% (within half a year) during the stable period was 49.5%, implying its poor accessibility in clinical settings. In the stable period, 21.4% of patients took oral theophylline drugs and 10.9% of patients had self-reported sputum overproduction (presenting with regular mucolytics intake). Moreover, 40.7, 12.8 and 17.1% of patients had self-reported hypertension, diabetes and coronary heart disease, respectively. In the exacerbation period, nearly 97% of patients were prescribed antibiotics in our cohort.
Predictive factors in the nomogram
To ensure its clinical practicability, we excluded some inaccessible predictors like recent FEV1%, possibly sacrificing precision to some extent. The smallest AIC of 577.31 occurred when we included sex, CAT scores, AE with respiratory failure in the previous year, new purulent sputum, new cardiovascular events, combined antibiotic therapy, theophylline therapy for AE, and ICU admission into the multivariate logistic models. These eight parameters were identified as the best subsets of covariates and were used to construct the nomogram ().
Table 2. Identification of predicted variables for nomogram.
In the multivariate logistic analyses, male patients [Odds Ratio (OR), 2.14; 95% Confidence Interval (CI), 1.039–4.400; p < 0.039), AE with respiratory failure in the previous year (OR, 2.259; 95%CI, 1.334–3.827; p < 0.002), new purulent sputum (OR, 2.256; 95%CI, 1.391–3.657; p < 0.001) and ICU admission (OR, 1.931; 95%CI, 1.199–3.111; p < 0.001) were independently associated with 30-day re-exacerbation. Combined antibiotic therapy (OR, 0.626; 95%CI, 0.375–1.044; p < 0.073) had preventive effects on short-term re-exacerbation with insufficient statistical power ().
Development and performance of the nomogram
The nomogram is shown in . To show how to use the nomogram for calculating an individualized risk, we take a case as an example. A 78-year-old male hospitalized for AECOPD was preparing for pre-discharge assessment. He had a CAT score of 15 and did not have a previous history of AE with respiratory failure. The patient presented with new purulent sputum at this onset of exacerbation, and received combined antibiotic therapy and theophylline therapy in the general ward. During the course of hospital stay, he did not have any emerging or worsening complications, including cardiovascular events. To estimate his 30-day probabilities of re-exacerbation, we could obtain the risk score of each parameter by directly retrieving or vertically mapping the score point on the variable line to the upper point line in . After summing up all the points, the patient got 193 total points in the nomogram. We then draw a vertical line connecting the Total Point scale to the lower Risk scale in and obtained the 30-day re-AE risk rate of 15% for this patient.
Figure 2. The nomogram for predicting 30-day re-AE events in patients hospitalized for AECOPD. To use the nomogram for predicting an individual patient’s risk, we firstly locate the status of each variable on the horizontal scale, and draw a line vertically to the upward point line to determine the corresponding points. Then, we sum up the points of all variables and locate the total points on the total point scale. Finally, we draw a vertical line from the dot of the total point scale to the bottom risk scale and calculate the risk of 30-day re-AE. AECOPD: acute exacerbation of chronic obstructive pulmonary disease; CAT: COPD assessment test; ICU: intensive care unit; pre-AE: acute exacerbation in the previous year.
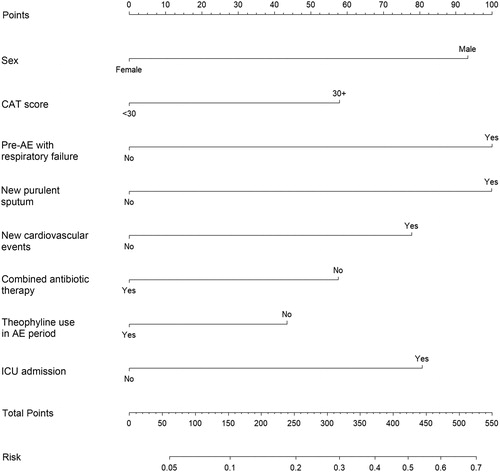
Table 3. Predictive risk points of each variable in the nomogram.
The C-index of the nomogram for 30-day re-AE prediction was 0.702 (95% confidence interval: 0.648–0.756). As shown in , the internal calibration plot in the total population showed close distances between the fit line and the diagonal line, implying optimal consistency between the nomogram-predicted probability and actual observation. Besides, the sensitivity analysis showed the good compatibility of our nomogram in the subpopulation without the lack of lung function data.
Figure 3. Calibration plot of the nomogram in total and subgroup population. For an individual patient, nomogram-predicted probability of 30-day re-exacerbation is plotted on the x-axis; observed probability is plotted on the y-axis. The apparent line represents the actual outcome of patients from the dataset. The bias-corrected line is calculated by the bootstrap algorithm with 1000 resamples. The lower deviation between the apparent line, the bias-corrected line and the diagonal line at 45° indicates the higher predictive accuracy of this nomogram.
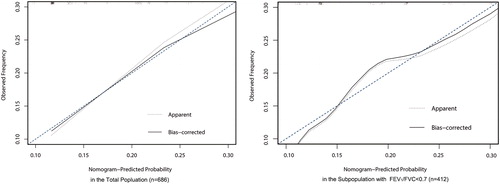
Discussion
Based on the optimal subset of eight variables with the smallest AIC, a practical and highly predictive nomogram to predict 30-day re-exacerbation after discharge for patients hospitalized with AECOPD was established.
In general, re-admission within 30 days after discharge is common for patients with AECOPD and accounts for nearly 1/3 of COPD healthcare expenditure (Citation25) and the corresponding program has been developed aiming at reducing the re-admission rate (Citation26). Some researches on COPD showed that the 30-day re-admission rate was 6–19% with great variation (Citation10,Citation11,Citation25,Citation27). We set the 30-day re-exacerbation as the primary outcome, which could cover the patients who had the symptom deterioration but were not re-admitted to hospitals. Our 30-day re-exacerbation rate of 15.8% was similar to the corresponding re-admission rate of 19.1% in an UK cohort, most of which had severe airflow limitation like our population (Citation27).
To our knowledge, previous reported assessment tools were all developed for predicting 3-month or 6-month re-exacerbation (Citation7,Citation12,Citation17), and we designed the first nomogram to evaluate the risks of 30-day re-exacerbation for in-hospital patients with AECOPD. Moreover, our predictive model had several advantages. First, excluding spirometric indices and laboratory markers, our nomogram was composed of easily accessible markers. The high missing rate of FEV1% (49.5%) during the recent stable period in our total population and a previous clinical audit (Citation28) indicated that lung function indices had poor clinical applicability, especially during exacerbation period and for patients with severe diseases. Furthermore, patients without recent FEV1% records had higher risks of 30-day re-exacerbation in our cohort (OR = 2.011, 95%CI = 1.387–2.916, p < 0.001), supporting that those had more severe COPD were less likely to perform spirometry follow-up. Thus, it is necessary to develop a high-predictive prognostic assessment tool without lung function for re-exacerbation. In the multivariate model, several variables have interaction and overlapping effects, so that other variables in our model could make up for the vacancy of spirometric indices. Second, our nomogram presented the calculation process in the visualized form and was thus easy for physicians to use.
Of the identified predictors, we found that male patients had significantly increased risks of 30-day re-exacerbation after discharge in our population, which was consistent with a previous Asian study for predicting in-hospital mortality (Citation16). We speculated that it was due to the distinct distribution of smoking history between male and female group (male, 85.6% versus female, 30%, p < 0.001). As described in some previous studies, higher CAT scores were significantly associated with shorter time to first exacerbation and higher exacerbation risks (Citation9,Citation29,Citation30). Our study also demonstrated that higher CAT scores were associated with 30-day re-exacerbation events. Our study demonstrated that patients discharged from ICU were predisposed to re-exacerbation within 30 days compared with those in the general ward. According to the GOLD report, patients with life-threatening exacerbation of COPD should be admitted into ICU (Citation20). In other words, ICU admission could be regarded as a comprehensive indicator for evaluating the severity of this exacerbation.
As a sufficient indicator of bacterial pneumonia, emerging purulent sputum was identified as a risk factor of re-exacerbation in our study. As proved in previous studies, pneumonic exacerbation of COPD indicated increased risks of in-hospital mortality and early re-admission compared with nonpneumonic AECOPD (Citation16,Citation27). Furthermore, our study showed patients with new cardiovascular events also had a trend towards re-exacerbation within 30 days with insufficient statistical power. Previous studies suggested atrial fibrillation and newly detected heart failure in AE period were independent risk factors of prognosis for patients with COPD (Citation31–33). In addition, we found that combined antibiotic therapy had a beneficial effect on reducing risks of 30-day re-exacerbation. Limited FEV1% data showed that nearly 80% of our subjects were classified as severe to very severe grade of COPD, implying the structural alteration of lung and high risks of infection or colonization of drug-resistant bacteria and Pseudomonas aeruginosa (Citation34). Thus, we postulated that combined antibiotic therapy might play an important role, not only in effectively killing drug-resistant bacteria but also in reducing the intrinsic bacterial load, especially for patients with severe COPD.
We did not incorporate some well-accepted predictors of COPD outcomes into our nomogram, such as AE numbers in the previous year and mMRC scale. First, we added the parameter of previous AE with respiratory failure instead of previous AE numbers, because we argued previous AE numbers might bring recalling biases and the severity of previous AE was also a strong predictor of re-exacerbation (Citation20). Owing to the inherent interaction of many variables and complexity of COPD, mMRC scale lost its prognostic value in our multivariate model as well as in another UK study (Citation27) and were excluded. As promising factors in many studies, blood eosinophil level and CRP level showed poor statistical power to predict re-exacerbation events by multivariate analysis in our population.
Our study had some limitations. First, limited spirometric data showed that most of our cohort were patients with severe to very severe COPD, implying our nomogram was unsuitable to be generalized to the mild COPD population or primary care settings. Second, with the aim of simplifying our assessment tool, we did not consider the drug category, dosage and duration of combined antibiotic therapy and theophylline therapy, which may be confounding factors of re-exacerbation. Third, we did not validate our results in broad external population. Fourth, we did not take the treatments administered for COPD at discharge into consideration, because we similarly prescribed bronchodilators and inhaled corticosteroids for all the hospitalized patients at discharge according to the GOLD report. Whereas, in the period of nomogram development, the stable medications prior to AE were all ruled out after multivariate logistic analysis, implying their poor abilities to prevent exacerbation in our population.
Conclusion
We integrate eight clinical variables to construct a practical and high-predictive nomogram, with the aim of predicting 30-day re-exacerbation events after discharge for patients hospitalized with AECOPD. This nomogram could help pulmonologists to assess risks of 30-day re-exacerbation for patients and offer individualized post-discharge support.
Disclosure
The authors report no conflicts of interest in this work.
Author contributions
Study design: Wei-ping Hu, Tsokyi Lhamo, Dong Liu, Jing Zhang; Data Collection: Tsokyi Lhamo, Dong Liu, Jing-qing Hang, Feng-ying Zhang, Yi-hui Zuo, Ying-ying Zeng; Statistical analysis: Wei-ping Hu, Tsokyi Lhamo; Manuscript writing: Wei-ping Hu, Tsokyi Lhamo, Dong Liu, Yi-hui Zuo, Ying-ying Zeng; Critical manuscript revision: Wei-ping Hu, Jing-qing Hang, Feng-ying Zhang, Jing Zhang. All authors read and approved the final manuscript.
Acknowledgments
The authors would like to acknowledge Dr Shi Xiao who critically reviewed the article for English writing.
Additional information
Funding
References
- Global Health Estimates. 2016: Disease burden by cause, age, sex, by country and by region, 2000–2016. Geneva: World Health Organization; 2018.
- Global Health Estimates. 2016: Deaths by cause, age, sex, by country and by region, 2000–2016. Geneva: World Health Organization; 2018.
- Donaldson GC, Seemungal TA, Bhowmik A, Wedzicha JA. Relationship between exacerbation frequency and lung function decline in chronic obstructive pulmonary disease. Thorax. 2002; 57(10): 847–852. doi: 10.1136/thorax.57.10.847.
- Seemungal TA, Donaldson GC, Paul EA, Bestall JC, Jeffries DJ, Wedzicha JA. Effect of exacerbation on quality of life in patients with chronic obstructive pulmonary disease. Am J Respir Crit Care Med. 1998; 157(5 Pt 1): 1418–1422. doi: 10.1164/ajrccm.157.5.9709032.
- Mullerova H, Maselli DJ, Locantore N, Vestbo J, Hurst JR, Wedzicha JA, Bakke P, Agusti A, Anzueto A. Hospitalized exacerbations of COPD: risk factors and outcomes in the ECLIPSE cohort. Chest. 2015; 147(4): 999–1007. doi: 10.1378/chest.14-0655.
- Price LC, Lowe D, Hosker HS, Anstey K, Pearson MG, Roberts CM, British Thoracic S, the Royal College of Physicians Clinical Effectiveness Evaluation U. UK National COPD Audit 2003: Impact of hospital resources and organisation of care on patient outcome following admission for acute COPD exacerbation. Thorax. 2006; 61(10): 837–842. doi: 10.1136/thx.2005.049940.
- Make BJ, Eriksson G, Calverley PM, Jenkins CR, Postma DS, Peterson S, Ostlund O, Anzueto A. A score to predict short-term risk of COPD exacerbations (SCOPEX). Int J Chron Obstruct Pulmon Dis. 2015; 10: 201–209. doi: 10.2147/COPD.S69589.
- Garcia-Aymerich J, Farrero E, Felez MA, Izquierdo J, Marrades RM, Anto JM, Estudi del Factors de Risc d'Aguditzacio de la Mi. Risk factors of readmission to hospital for a COPD exacerbation: a prospective study. Thorax. 2003; 58(2): 100–105. doi: 10.1136/thorax.58.2.100.
- Lee SD, Huang MS, Kang J, Lin CH, Park MJ, Oh YM, Kwon N, Jones PW, Sajkov D, Investigators of the Predictive Ability of CATiAEoCS. The COPD assessment test (CAT) assists prediction of COPD exacerbations in high-risk patients. Respir Med. 2014; 108(4): 600–608. doi: 10.1016/j.rmed.2013.12.014.
- Baker CL, Zou KH, Su J. Risk assessment of readmissions following an initial COPD-related hospitalization. Int J Chron Obstruct Pulmon Dis. 2013;8:551–559.
- Lin J, Xu Y, Wu X, Chen M, Lin L, Gong L, Feng J. Risk factors associated with chronic obstructive pulmonary disease early readmission. Curr Med Res Opin. 2014; 30(2): 315–320. doi: 10.1185/03007995.2013.858623.
- Liu D, Peng SH, Zhang J, Bai SH, Liu HX, Qu JM. Prediction of short term re-exacerbation in patients with acute exacerbation of chronic obstructive pulmonary disease. Int J Chron Obstruct Pulmon Dis. 2015; 10: 1265–1273. doi: 10.2147/COPD.S83378.
- Han MK, Kazerooni EA, Lynch DA, Liu LX, Murray S, Curtis JL, Criner GJ, Kim V, Bowler RP, Hanania NA, et al. Chronic obstructive pulmonary disease exacerbations in the COPDGene study: associated radiologic phenotypes. Radiology. 2011; 261(1): 274–282. doi: 10.1148/radiol.11110173.
- Almagro P, Soriano JB, Cabrera FJ, Boixeda R, Alonso-Ortiz MB, Barreiro B, Diez-Manglano J, Murio C, Heredia JL, Working Group on Copd SoIM. Short- and medium-term prognosis in patients hospitalized for COPD exacerbation: the CODEX index. Chest. 2014; 145(5): 972–980. doi: 10.1378/chest.13-1328.
- Wang Y, Li J, Xia Y, Gong R, Wang K, Yan Z, Wan X, Liu G, Wu D, Shi L, et al. Prognostic nomogram for intrahepatic cholangiocarcinoma after partial hepatectomy. J Clin Oncol. 2013; 31(9): 1188–1195. doi: 10.1200/JCO.2012.41.5984.
- Sakamoto Y, Yamauchi Y, Yasunaga H, Takeshima H, Hasegawa W, Jo T, Sasabuchi Y, Matsui H, Fushimi K, Nagase T. Development of a nomogram for predicting in-hospital mortality of patients with exacerbation of chronic obstructive pulmonary disease. Int J Chron Obstruct Pulmon Dis. 2017; 12: 1605–1611. doi: 10.2147/COPD.S129714.
- Niewoehner DE, Lokhnygina Y, Rice K, Kuschner WG, Sharafkhaneh A, Sarosi GA, Krumpe P, Pieper K, Kesten S. Risk indexes for exacerbations and hospitalizations due to COPD. Chest. 2007; 131(1): 20–28. doi: 10.1378/chest.06-1316.
- Mahler DA, Harver A. A factor analysis of dyspnea ratings, respiratory muscle strength, and lung function in patients with chronic obstructive pulmonary disease. Am Rev Respir Dis. 1992;145(2 Pt 1):467–470. doi: 10.1164/ajrccm/145.2_Pt_1.467.
- Jones PW, Harding G, Berry P, Wiklund I, Chen WH, Kline Leidy N. Development and first validation of the COPD Assessment Test. Eur Respir J. 2009; 34(3): 648–654. doi: 10.1183/09031936.00102509.
- Global Strategy for the Diagnosis, Management and Prevention of COPD-2017 [Internet]. Global Initiative for Chronic Obstructive Pulmonary Disease; [cited 2018 Aug]. Available from: https://goldcopd.org/gold-2017-global-strategy-diagnosis-management-prevention-copd/.
- Seemungal TA, Donaldson GC, Bhowmik A, Jeffries DJ, Wedzicha JA. Time course and recovery of exacerbations in patients with chronic obstructive pulmonary disease. Am J Respir Crit Care Med. 2000; 161(5): 1608–1613. doi: 10.1164/ajrccm.161.5.9908022.
- Posada D, Buckley TR. Model selection and model averaging in phylogenetics: advantages of akaike information criterion and bayesian approaches over likelihood ratio tests. Syst Biol. 2004;53(5):793–808. doi: 10.1080/10635150490522304.
- Wagenmakers EJ, Farrell S. AIC model selection using Akaike weights. Psychon Bull Rev. 2004;11:192–196.
- Rms: Regression modeling strategies. R package version 5.0–0. 2016 [Internet]. Harrell Jr FE; [cited 2018 Aug]. Available from: http://CRAN.R-project.org/ package = rms.
- Jacobs DM, Noyes K, Zhao J, Gibson W, Murphy TF, Sethi S, Ochs-Balcom HM. Early Hospital Readmissions after an Acute Exacerbation of Chronic Obstructive Pulmonary Disease in the Nationwide Readmissions Database. Ann Am Thorac Soc. 2018; 15(7): 837–845. doi: 10.1513/AnnalsATS.201712-913OC.
- Kocher RP, Adashi EY. Hospital readmissions and the Affordable Care Act: paying for coordinated quality care. JAMA. 2011;306(16):1794–1795. doi: 10.1001/jama.2011.1561.
- Steer J, Norman EM, Afolabi OA, Gibson GJ, Bourke SC. Dyspnoea severity and pneumonia as predictors of in-hospital mortality and early readmission in acute exacerbations of COPD. Thorax. 2012; 67(2): 117–121. doi: 10.1136/thoraxjnl-2011-200332.
- Hartl S, Lopez-Campos JL, Pozo-Rodriguez F, Castro-Acosta A, Studnicka M, Kaiser B, Roberts CM. Risk of death and readmission of hospital-admitted COPD exacerbations: European COPD Audit. Eur Respir J. 2016; 47(1): 113–121. doi: 10.1183/13993003.01391-2014.
- García-Rivero JL, Esquinas C, Barrecheguren M, Bonnin-Vilaplana M, García-Sidro P, Herrejón A, Martinez-Rivera C, Molina RM, Marcos PJ, Mayoralas S, et al. Risk factors of poor outcomes after admission for a COPD exacerbation: multivariate logistic predictive models. COPD. 2017;14(2): 164–169. doi: 10.1080/15412555.2016.1260538.
- García-Sidro P, Naval E, Rivera CM, Bonnin-Vilaplana M, Garcia-Rivero JL, Herrejon A, Molina RM, Marcos PJ, Mayoralas-Alises S, Ros JA, et al. The CAT (COPD Assessment Test) questionnaire as a predictor of the evolution of severe COPD exacerbations. Respir Med. 2015;109(12): 1546–1552. doi: 10.1016/j.rmed.2015.10.011.
- Steer J, Gibson J, Bourke SC. The DECAF Score: predicting hospital mortality in exacerbations of chronic obstructive pulmonary disease. Thorax. 2012;67(11):970–976. doi: 10.1136/thoraxjnl-2012-202103.
- Boudestein LC, Rutten FH, Cramer MJ, Lammers JW, Hoes AW. The impact of concurrent heart failure on prognosis in patients with chronic obstructive pulmonary disease. Eur J Heart Fail. 2009; 11(12): 1182–1188. doi: 10.1093/eurjhf/hfp148.
- Echevarria C, Steer J, Heslop-Marshall K, Stenton SC, Hickey PM, Hughes R, Wijesinghe M, Harrison RN, Steen N, Simpson AJ, et al. The PEARL score predicts 90-day readmission or death after hospitalisation for acute exacerbation of COPD. Thorax. 2017; 72(8): 686–693. doi: 10.1136/thoraxjnl-2016-209298.
- Mandell LA, Wunderink RG, Anzueto A, Bartlett JG, Campbell GD, Dean NC, Dowell SF, File TM, Jr., Musher DM, Niederman MS, et al. Infectious Diseases Society of America/American Thoracic Society consensus guidelines on the management of community-acquired pneumonia in adults. Clin Infect Dis. 2007; 44 Suppl 2: S27–S72. doi: 10.1086/511159.