Abstract
Almost half of chronic obstructive pulmonary disease (COPD) exacerbations are estimated to be inaccurately reported by patients, inconsistently recorded in medical records, or not measured due to coding errors inherent to administrative claims. This retrospective observational study aimed to develop an algorithm capable of detecting acute COPD exacerbations (AECOPD) in healthcare claims and estimate costs associated with AECOPD over a 12-month period. Commercial and Medicare Advantage healthcare plan members (≥40 years old) with evidence of COPD were identified from US healthcare-claims database. To refine the algorithm detecting AECOPD in claims data, sensitivity and positive-predictive value calculations were performed to compare AECOPD identification in healthcare claims versus medical charts. Analyses were also performed to examine total exacerbation-related costs for events identified with the new claims algorithm plus events missed. The final algorithm had a sensitivity of 84.9%, with a positive-predictive value of 67.5%. Medical records were abstracted for 402 patients. In the overall sample of healthcare claims (n = 243,998), the algorithm detected ≥1 AECOPD event in 61.3% of patients. The mean cost per patient during an AECOPD episode, identified by the final algorithm, was USD 6,760 (n = 301), with an incremental average cost of USD 607 (n = 122) to ‘unobserved’ episodes (not reported in claims data) among the chart sample. After multivariate modeling, predicted yearly exacerbation costs translated to USD 1.12 billion per 100,000 patients (USD 12,000 per patient), with 35.76 million associated with unobserved exacerbations. While the final algorithm warrants further validation and study, these findings highlight unobserved AECOPD and their economic burden.
Introduction
Chronic obstructive pulmonary disease (COPD), the third leading cause of death worldwide in 2016 [Citation1], is associated with significant morbidity and a substantial and increasing economic and social burden [Citation2,Citation3].
There are varying definitions for COPD exacerbations, generally described as acute episodes of worsening COPD symptoms. The Global Initiative for Chronic Obstructive Lung Disease (GOLD) defines exacerbations as ‘an acute worsening of respiratory symptoms that result in additional therapy’ [Citation2], while other clinical definitions are more symptom-based, for example characterizing acute COPD exacerbation (AECOPD) by changes in the production and/or color of sputum and increased dyspnea, without requiring changes in medical treatment [Citation4–7]. Mild exacerbations are not of major interest in economic analyses as they are self-managed without the need of systemic corticosteroids or antibiotics [Citation8].
COPD exacerbations are associated with disease progression and a negative effect on both quality of life and healthcare outcomes (including patients' lung function and exercise performance) [Citation9]. Accounting for the largest proportion of the total COPD-related burden on healthcare systems, COPD exacerbations are a key driver of healthcare costs [Citation2–5]. However, it is estimated that up to 50% of exacerbations are inaccurately reported by patients, inconsistently recorded in medical records, or not measured due to coding errors inherent to administrative claims [Citation10,Citation11]. Coding errors may be introduced by administrative mistakes, such as the use of a wrong code based on the established guidelines [Citation12].
Because of their comprehensive coverage, detailed information, and ready availability, administrative claims data are an important source of information for managing population health and quantifying the disease burden and outcomes for patients with COPD and other chronic diseases. Healthcare claims provide broad information on COPD care and generally define the disease using the International Classification of Diseases (ICD) Clinical Modification (CM) codes. Algorithms to identify COPD exacerbations in healthcare claims typically focus on inpatient or emergency room (ER) events linked to COPD-related diagnoses, typically recorded through use of these ICD codes [Citation13,Citation14]. Medical records contain more detailed information and different data types than healthcare claims, thus may provide more accurate identification of medical conditions and events such as COPD exacerbations. In light of these differences, standard algorithms used in healthcare claims-based studies for identifying AECOPD may not include some of the exacerbation-related symptoms or exacerbation events; this may lead to possible underreporting exacerbations when assessing a population using claims data. These differences in identifying AECOPD may contribute to the broad variation in cost estimates associated with these events [Citation13].
Under-reporting or the inability or failure to record AECOPD events can result in unrecognized or under-recognized patient risk, suboptimal treatment, missed opportunities to reduce future exacerbations and poor health outcomes. Estimating the magnitude of unrecorded healthcare claims for AECOPD events and their associated costs will help to quantify potential gaps in care. Furthermore, in addition to the assessment of healthcare resource use, claims data are used for payment; by calculating the costs of unrecorded AECOPD events it is possible to estimate the economic burden this may represent across the COPD population. In this study, we aimed to define a more sensitive and specific algorithm for detecting AECOPD in healthcare claims (within a US insured population), to provide a more accurate understanding of the disease burden of COPD and an estimate of its associated costs in the hope of ultimately improving patient care.
The primary objective of our study was to create an algorithm to better identify cases of unobserved AECOPD in healthcare claims data and estimate the number of patients with at least one AECOPD event (including both unobserved and observed exacerbations in the claims data). The study also aimed to estimate the direct healthcare costs associated with unobserved and observed exacerbations.
Methods
Study design, patients and outcomes
This retrospective observational study in a US insured population (GlaxoSmithKline plc. HO-14-14650) used algorithms to identify AECOPD events reported within administrative healthcare claims data (hereafter referred to as ‘observed’ AECOPD), and compared with data from abstracted medical records to assess the performance of the algorithms and identify additional AECOPD not observed in claims data using the initial algorithm (hereafter referred to as ‘unobserved’ AECOPD).
Commercial and Medicare Advantage health plan members with evidence of COPD were identified from the Optum Research Database. Patients included in the analysis were ≥40 years of age, continuously enrolled in the health plan for ≥24 months and had evidence of COPD (≥2 claims on different dates with ICD-9-CM codes in any position for COPD: 491.XX, 492.X, 496) identified from 01 January 2009–31 December 2013.
The date of the first identification of COPD in the claims data was defined as the index date. Patient characteristics were assessed for a 12-month pre-index baseline period.
Outcomes were assessed over a 12-month follow-up period following and including index date; specifically, the presence of observed exacerbations (observed within the claims data using ICD-9-CM codes describing COPD exacerbations), unobserved exacerbations (COPD exacerbations that were identified in the abstracted medical records data but not observed in the claims data), and healthcare usage. Healthcare usage included both medical (ambulatory, ER, and inpatient) and pharmacy services, associated events.
Healthcare costs were calculated from the combined health-plan-paid and patient-paid amounts for medical and pharmacy services. Costs were adjusted using the annual medical-care component of the Consumer Price Index to reflect inflation between 2009 and 2017 [Citation15]. Multivariable analyses were performed to compare total exacerbation-related costs between patients with reported and unrecorded claims based moderate/severe COPD exacerbations. Cost outcomes were analyzed using generalized linear model with a gamma distribution and log link. In addition to multivariable analysis of total exacerbation-related costs, event-level multivariable analysis (average cost per day) were performed for reported and unrecorded exacerbations.
An independent review board and affiliated privacy board approved the study protocol and documents relating to medical chart review prior to fielding the data collection for the medical chart review. The review boards granted a waiver of authorization and informed consent in order to conduct the study as designed.
The primary data source was the Optum Research Database, consisting of administrative medical claims (for hospitalization events, ER or office visits), enrollment data and pharmacy claims. This database included information relating to approximately 12.6 million individuals per calendar year. Administrative claims included information on diagnoses and symptoms based on ICD-9-CM diagnosis codes. The data were geographically diverse and representative of the US commercially insured and Medicare-insured populations.
An initial claims-based algorithm, based on the literature [Citation16,Citation17], previous feasibility analysis and consensus of a research committee was selected, evaluated and refined by comparing the claims data with medical record data for a subset of the patients identified in the claims data. The algorithm started with the assumption that that it would be applied to a population of patients who were already identified as having COPD. The initial algorithm aimed to identify COPD exacerbations in both the inpatient and ambulatory setting and included as potential algorithm elements: diagnosis recorded with the ICD-9-CM diagnosis codes; procedures recorded with ICD-9-CM procedure codes; Current Procedural Terminology, or Healthcare Common Procedure Coding System codes; site of service codes; provider specialty codes; revenue codes (for facilities); and other information. Specific ICD-9-CM codes used to identify AECOPD events in this initial algorithm are available in Supplementary Appendix A. The algorithm defined acute COPD exacerbations in two ways. Events included hospitalizations with COPD-related diagnoses, or ER or ambulatory visits with COPD-related diagnoses and antibiotic or systemic corticosteroid prescriptions within 5 days. The inclusion of antibiotics and systemic corticosteroid prescriptions meet the standards required for a moderate exacerbation whereas hospitalization meets the requirement for a severe exacerbation as defined by the Global Initiative for Chronic Obstructive Lung Disease [Citation2]. Episodes consisted of single or repeated exacerbations falling within a 14 day period from the prior qualifying event (counted from the start date or date of hospital discharge, whichever was later). A full description of the initial algorithm is included in Supplementary Appendix B.
Medical record data were used to confirm algorithm-identified exacerbations that were observed in healthcare claims data, and to collect information on unobserved exacerbations. Medical record data were targeted to be collected from a convenience sample of 400 patients identified in the claims data with evidence of at least one reported or unrecorded AECOPD, as calculated via the claims algorithms. The aim here was 300 patients with AECOPD and 100 without AECOPD based on the original algorithm. The sample size calculation for the primary objective used a binomial distribution for a sample of 400 patients. This showed the expected size of 200 patients with unrecorded exacerbations to be adequate to estimate with a confidence interval for sensitivity/specificity of +5 percentage points for a proportion of 70%, +4 percentage points for a proportion of 80%, and +3 percentage points for a proportion of 90%. Medical record data for a random subset of patients with evidence in the claims data of at least one observed or no (but potentially unobserved) episode of AECOPD based upon the initial algorithm were requested from a sample of 1800 physicians invited to participate in the study. Medical records were obtained from three sources where possible depending on the setting for the AECOPD episode identified from the claims data (primary care providers, and ER and inpatient hospital admissions) and abstracted to collect information on exacerbations. Records from the patient’s primary care physician were obtained first; secondary records (ER or inpatient visit relating to the claims-identified exacerbation) were only obtained if the primary care chart did not contain sufficient information to confirm the existence of the ER or inpatient exacerbation that the claims-algorithm identified. Data collected included verifying the dates and characteristics of the observed exacerbations (location of treatment, treatment with corticosteroids or antibiotics), details regarding false positives (true diagnosis for that visit) and unobserved exacerbations or other lung-related symptoms (specifics regarding respiratory symptoms or conditions, notes regarding exacerbations, prescriptions for corticosteroids or antibiotics). Where possible, information collected from medical charts included confirmation of the dates and types of exacerbations identified in the claims data (inpatient, ER, outpatient), characteristics of the visit if not found to be an exacerbation and recording of COPD/lung-related symptoms and COPD exacerbations for visits that were not identified in the claims data as exacerbations. In addition to confirming exacerbations that were identified in the claims data, the chart abstraction collected information on lung-related signs and symptoms and diagnoses that had not been identified in the claims data; where these were found in the chart, the related note was recorded for further context. For exacerbations identified in the claims but not confirmed in the chart (i.e. non-exacerbation diagnosis or event), the note describing the event was recorded.
Algorithm development
Using a number of clinical variables, the initial algorithm was modified to generate new algorithms after reviewing the medical record data for characteristics of the false positive and the false negative exacerbations based on the original algorithm. The codes 493.12, 493.92, 494.1, 466.0 were used in addition to the original algorithm in order to help identify exacerbation-related visits. For example, as the algorithm was constructed to identify exacerbations among patients already identified as having COPD, there was a possibility of miscoding of a COPD exacerbation for patients with co-existing conditions; while the listed codes were used to identify exacerbation-related visits, note that these codes were not used to identify whether the patient had COPD. Codes for both primary and secondary diagnoses were included in the algorithm and both could be used to identify moderate and severe exacerbations; the diagnosis codes could be used in any position, which did not differ for moderate or severe exacerbations. Additional ICD-9-CM codes identifying lung infections (acute nasopharyngitis, croup, acute upper respiratory infection, acute bronchiolitis, bronchiectasis without acute exacerbation, pneumonia and influenza) and/or signs or symptoms, as identified in the medical charts (such as cyanosis, unspecified respiratory abnormality, orthopnea, apnea, Cheyn-Stoke respiration, shortness of breath, wheezing, tachypnea, stridor, cough, hemotopsis, abnormal sputum, asphyxia, hypoxemia and other, including respiratory distress or insufficiency), during the non-episode periods were also included as variables (further information is available in Supplementary appendix B).
Using the medical records as the ‘gold standard’ for exacerbation history, within the claims-based algorithm we identified; 1) true-positives (claims-based exacerbations positively confirmed by medical records), 2) false-positives (exacerbations identified in healthcare claims that were not positively confirmed in medical records) and 3) unrecorded (false-negatives) AECOPD (exacerbations in the medical records that were not identified by the claims-based algorithm) at the exacerbation level. The characteristics of false-positive and false-negative exacerbations were assessed to define potential codes for exclusion or addition to the revised algorithm.
Data analyses
All analyses were conducted using SAS 9.4 (SAS Institute Inc., Cary, NC, USA). Study variables were analyzed descriptively and stratified by the presence or absence of a claims-based AECOPD, according to the final updated algorithm definition. General comorbid conditions were defined using the Clinical Classifications Software managed by the Agency for Healthcare Research and Quality (AHRQ), which generated indicator variables for specific disease conditions based on ICD-9-CM diagnoses. A COPD severity score [Citation18] was created based on the sum of 12 weighted claims-based components. The 12 components (and their associated weights) were as follows: hospitalizations due to acute exacerbation of chronic bronchitis (AECB)—number of days (0.34); oxygen therapy (0.57); AECB—number of episodes (0.40); emphysema (0.46); count of spirometry tests (0.27); count of pulmonologist visits (0.38); count of anticholinergic claims (0.73); count of oral corticosteroids claims (0.58); count of inhaled corticosteroid claims (0.63); count of SABA (short-acting β2-agonist; including SABA/anticholinergics combination) claims (0.81); count of LABA (long-acting β2-agonist) claims (0.76); and age (0.30). Baseline medication variables were not captured.
To assess the performance of the claims-based algorithm, it was compared with the 'gold standard measure of all exacerbations found in the medical record data as described above. We did not test each element of the algorithm independently for contribution to accuracy of identification of the exacerbations; we assessed whether altering specific elements (e.g. adding specific diagnosis codes, disregarding antibiotic prescriptions filled close to surgery) improved accuracy. Specifically, the ability of the claims-based algorithm to identify all of the medical chart exacerbations was measured by calculating; sensitivity (proportion of exacerbations correctly captured by the algorithm) and positive-predictive value (PPV; proportion of the algorithm-defined exacerbations that were verified in the chart data). Negative-predictive value and specificity were not calculated due to the difficulty in appropriately determining (and validating) the denominator i.e. all healthcare visits that were not for COPD exacerbations. Descriptive analyses included total counts of patients with claims-based AECOPD and all-cause, COPD-specific and observed AECOPD costs, overall and stratified for patients with and without unobserved claims-based AECOPD events.
Multivariable analyses were also performed to compare total exacerbation-related costs between patients with observed and unobserved claims-based moderate/severe COPD exacerbations. Cost outcomes were analyzed using a generalized linear model with a gamma distribution and log link.
Results
Observed and unobserved AECOPD
Approximately 28.6 million commercial/Medicare enrollees with medical and pharmacy benefits from 01 January 2009–31 December 2013 were included in the analysis. Patients with COPD were identified from claims data; in total, 655,762 subjects (2.3%) had qualifying COPD diagnosis codes and 251,639 were continuously enrolled. Of these, 243,998 patients met full eligibility requirements and comprised the claims-based population on whom the starting algorithm was applied to identify AECOPD (). This population served as the starting point for the random sample of patients selected for chart review. Development of the final algorithm is described in Supplementary appendix D.
Table 1. Demographic and disease characteristics of patients in the claims sample.
Medical record enquiries for patients randomly selected for chart review were sent to each patient’s physician, with a target of acquiring a minimum sample of 400 patients, with a target of 300 with AECOPD and 100 without AECOPD based on the original algorithm. Overall, 1,587 physicians contributed 541 primary and secondary medical charts (one of which detailed two COPD exacerbations) that were subsequently validated as containing sufficient data for inclusion in the study. The medical chart-based data were collected for a final sample of 402 patients, who had 542 AECOPD events ().
Figure 1. Patient disposition flow chart.
Data from inpatient/ER (secondary care) charts, were requested only if the primary care chart had insufficient data.
Abbreviations: ER = emergency room.
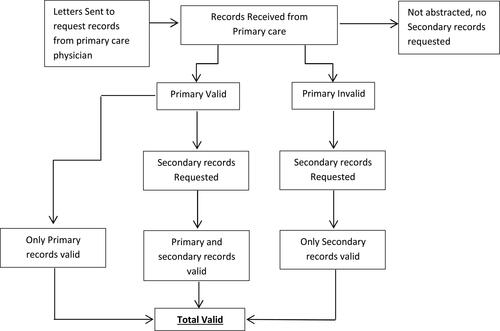
The primary care charts of 100% of patients who had an ER or inpatient exacerbation were found to lack sufficiently detailed information about the ER or inpatient exacerbation, thus secondary care (ER/inpatient) charts were requested for all of these patients; the secondary charts were successfully obtained in 93% of cases ().
Overall, 65.9% of claims-identified exacerbations had data in the charts to confirm true-positive exacerbations. For example, the initial claims data algorithm suggested that the patient visited their physician with a diagnosis of an exacerbation that had corresponding documentation in the physician notes. The remaining 34.1% of exacerbations identified in the claims data were false-positives (for example, the patient receiving an antibiotic in preparation for a surgery or experiencing a respiratory complaint that did not meet the level of an exacerbation). Among the 100 patients without claims-based exacerbations, there were 20 patients who had COPD ‘unidentified’ events found in the charts; (mean number of events 0.23 (SD 0.49), with 17 only having 1 event. None of these events were in the inpatient setting (none were severe, all were moderate).
In the overall claims sample of patients with documented COPD who met all eligibility criteria (n = 243,998), 61.3% of patients had at least one AECOPD event during the 12-month follow-up period according to the final updated algorithm; approximately 30% had at least one severe exacerbation and approximately 45% had at least one moderate exacerbation. In this population, the mean number of exacerbation events per patient was 1.48 (0.44 severe, 1.04 moderate) and the mean number of episodes was 1.13. Additional patient characteristics for this sample are shown in , presented according to whether the final algorithm detected any recorded exacerbations or not.
Healthcare costs
For the chart population (n = 402), the mean costs per patient across all AECOPD episodes during the 12-month follow-up period were lower for the final updated algorithm (US dollars [USD] 9,623; standard deviation [SD] 24,216) than for the initial algorithm (USD 9,911; SD 24,508; ).
Table 2. Healthcare costs for the chart population predicted by the initial and final algorithms.
These lower costs were attributed to the exclusion from the final algorithm of additional false positive events. The mean cost of unobserved episodes was lower for the final updated algorithm (USD 252; SD 976) versus the initial algorithm (USD 287; SD 1,136).
The final updated algorithm identified the mean cost per patient during an AECOPD episode as USD 6,760 (n = 301; SD 18,158), with an average cost for unobserved episodes of USD 607 (n = 122; SD 1,261) that was incremental to the cost of the observed episodes.
Multivariable modeling of patient- and treatment-level risk factors for costs
The regression model identified a number of predictors significantly associated with having any unobserved exacerbation costs. Factors associated with a higher odds of unobserved exacerbation costs included presence of fluid/electrolyte disorder at baseline, prescription fill for a short-acting β2-agonist (SABA) and the presence of ≥2 spirometry tests; whereas patients in the higher quartile of COPD severity or with baseline urinary diseases had a lower odds of having unobserved exacerbation costs (). In patients with at least some unobserved exacerbations costs, the costs of these events were found to be higher for patients with: at least one severe (observed) exacerbation, baseline urinary system disorders, emphysema in the follow-up period, administration or prescription of corticosteroids in the follow-up period or baseline ear conditions. Unobserved exacerbation costs were decreased with an increase in the count of observed exacerbation episodes identified via the revised algorithm.
Patients were less likely to have any costs for false-positive exacerbations if they were aged 65 or older (vs. patients aged 40–54; there was no difference between patients aged 55–64 and those aged 40–54), and had a filled prescription for SABA medications. Characteristics significantly associated with higher odds of false-positive exacerbation costs included having a higher count of exacerbations episodes identified using the final algorithm and a diagnosis of emphysema or baseline skin cancer ().
Table 3. Exacerbation costs for the final algorithm from the multivariate analysis two-part model, including predicted costs and bootstrapped 95% confidence intervals.
Taking into account true-positive, false-positive and unobserved (false-negative) exacerbations, increasing number of corticosteroid prescription fills and administrations was associated with higher odds of exacerbation costs, while receiving immunizations or infectious disease screening during the baseline period was associated with lower odds of exacerbation costs (Supplementary table C4). In patients who did have exacerbation costs, costs were higher for patients with COPD-related inpatient visits and were positively associated with the count of exacerbation episodes identified by the initial algorithm.
The models estimated using the medical records population were used to predict exacerbation costs for both the chart population and the total claims-based population ().
The predicted mean 1-year total costs per patient for identified exacerbations (true- and false-positives) as well as for unobserved exacerbations were USD 10,139 for the chart population and USD 11,191 for the claims population. For unobserved exacerbations, the predicted average costs per patient were USD 273 for the chart population and USD 358 for the claims population. For a population of 100,000 patients with COPD, this represents total yearly exacerbation costs of USD 1.12 billion, with USD 35.76 million in unobserved exacerbation costs and USD 673 million associated with false-positive exacerbations per year using health administration claims.
Discussion
This study refined an algorithm for identifying AECOPD from administrative healthcare claims, using medical charts as a reference standard. The initial algorithm aimed to identify COPD exacerbations in both the inpatient and ambulatory setting, and was based on a previous algorithm that was limited to hospitalized patients based on the literature [Citation17]. This initial algorithm was modified with the addition of further ICD codes and rules, and a final algorithm was selected with the best balance between increased ability to detect AECOPD and reduced risk of including false-positives. The final algorithm had a high sensitivity of 84.9% and PPV of 67.5%: an improvement over the initial algorithm, which had a sensitivity of 84.2% and PPV of 65.9%. In addition, our data provide information to help further the understanding of the direct healthcare costs associated with unobserved and observed exacerbations across the COPD population. These costs represent potential areas for improving care for patients in addition to the aim of reducing the rate of exacerbations.
Few studies have evaluated the sensitivity and PPV of methods for identifying exacerbations from patient surveys, medical records or healthcare claims data. One recent study found a sensitivity of 84% for patient self-reporting and 89–96% for single-physician chart reviews compared with rates from an adjudication committee, used as the reference standard [Citation10]. The sensitivity of the algorithm in the current study was comparable to this. Another recent study has compared AECOPD rates in patients based on their rates of healthcare utilization and using a symptom-based definition of AECOPD, finding a 59–78% higher risk of AECOPD in the hospital-based sample compared with the population sample [Citation19]. This increased incidence of AECOPD in the hospital sample is not surprising as it correlated with increased COPD severity according to the GOLD classifications, which may also be the case for our claims sample, given that a large proportion of the patients in our analysis had hospital claims data.
In the claims sample of the current study (n = 243,998), the final algorithm identified 61.3% of patients as having at least 1 AECOPD event or episode, with 30.5% of patients having an exacerbation classified as severe, which is a relatively high proportion compared to other COPD cohorts [Citation20–22]. Healthcare claims for the subset of patients with medical chart information available showed a mean cost during AECOPD episodes per patient of USD 6,760 and a total of USD 9,623 per patient observed across all episodes. The average cost of an unobserved AECOPD episode was USD 607. A previous analysis of healthcare claims data from 2007–2009 reported COPD-related mean annual costs of USD 4,069 [Citation23]. While these data cannot be directly compared with ours due to the differing time periods and differences in cost calculation, it is worth noting that costs in the current study do appear to be much higher. These are quite different perhaps, because our estimates are for the episode, not just individual events, so give a more complete picture of the impact of the whole sequence of events associated with exacerbations. However, the difference may also reflect a higher severity of COPD in the population we have studied, due to our stringent requirements for identifying true COPD from the claims database (two ICD codes diagnosing COPD were required at two different dates).
Using multivariable modeling on the current study population, projections to 100,000 patients with COPD suggest that yearly exacerbation costs totaling more than USD 1.12 billion may be observed using the final algorithm. These include USD 35.76 million associated with unobserved exacerbations and USD 673 million associated with ‘false-positive’ exacerbations. These are representative of true existing costs for the patient and also reflect actual health service use and accrued costs, often as a result of comorbid conditions, yet may be inaccurately recorded as COPD-associated costs in the claims data. Therefore, these false-positive costs, which account for nearly two-thirds of the total projections, do not represent potential cost-savings per se but rather areas in which the cost designation of treatment for other conditions may be complicated by the presence of underlying COPD, exaggerating the costs that are attributed to COPD treatment. It may be difficult to differentiate between these false-positive events and true exacerbations using a claims algorithm alone, particularly as these events may reflect service use that is specific to COPD patients, such as the ‘preventative’ receipt of antibiotics. The burden associated with these costs is an issue that likely extends to countries outside of US-setting described here – warranting further analysis specific to these countries.
Similarly, exacerbations unobserved in claims data may not simply reflect under-reporting, but coding under different selections due to the presence of comorbidities. Since large US health plans use their administrative claims data to assess the need for patient support programs or interventions, the failure of the data to accurately reflect patient needs results in the inability of plans to aid their patient population.
Predictors of higher costs for unobserved exacerbations identified in two-part modeling that included baseline presence of fluid/electrolyte disorder, prescription fill for a SABA, and the presence of ≥2 spirometry tests. This latter finding may reflect additional spirometry testing following readmission to hospital with a COPD exacerbation, as previously reported [Citation24]. Unobserved exacerbation costs were lower for patients having more exacerbations identified by the claims algorithm. Conversely, characteristics associated with higher odds of false-positive exacerbation costs included having more previously reported exacerbations identified by the algorithm, and diagnoses of emphysema or baseline skin cancer. The costs related to false-positive exacerbations were higher than those for true-positive or unobserved exacerbations, and were associated with more COPD-related inpatient hospitalization days.
In some cases, the presence of observed exacerbations may exclude the opportunity for unobserved exacerbations; if more of the follow-up period is attributed to confirmed exacerbations, less time (opportunity) is left for unobserved exacerbations to occur. It is unclear exactly why some of these factors influenced costs associated with incorrectly identified exacerbations. Further examination of algorithm results and comparison to chart reviews is warranted in a larger chart review sample.
Comorbid disease occurs frequently in patients with COPD and may confound the identification of AECOPD. Comorbidities, including acute myocardial infarction, congestive heart failure, cerebrovascular disease and lung cancer have previously been associated with high costs in patients with COPD [Citation25]. Hospitalizations with secondary COPD diagnoses were reported to rise over time from 2002 to 2010 [Citation26]. Furthermore, it is possible that newly emerging asthma may have impacted on some AECOPD episodes, as two codes that were included in the final algorithm to enhance specificity and PPV related to asthma (extrinsic asthma, unspecified, ICD-9-CM code 493.00, and asthma, unspecified, with unspecified status, ICD-9-CM code 493.90). Overlap between asthma and COPD is an increasingly recognized phenomenon, with the first specific guidance for treatment and management included in global asthma and COPD strategic documents in 2015 [Citation27]. Asthma-COPD overlap syndrome affects approximately a fifth of individuals with COPD in the US [Citation28], which may explain why inclusion of these asthma codes improved the precision of our algorithm as individuals with asthma were not excluded.
This study had several limitations due to the nature of the data sources [Citation29], meaning that it was not possible to infer indirect healthcare costs. Due to coding errors or upcoding, inaccurate information may be included in healthcare claims; the presence of a diagnosis code may not be conclusive of disease, as it may have been incorrectly coded or included as rule-out criteria rather than actual proof of disease. In this regard, for patients with comorbid disease it is possible that other codes may have been recorded in the claims data rather than those denoting underlying COPD exacerbations. Furthermore, as some of the unobserved exacerbations were identified using symptoms reported in the medical charts, this may explain why interpretation of these symptoms did not always correlate to COPD exacerbation codes in the corresponding claims data. Similarly, as diagnoses are not included on pharmacy claims, the reason for the prescription of antibiotics or corticosteroids was inferred based on proximity to medical visits, however this inference was sometimes incorrect. For example, some visits had COPD diagnoses recorded on the claims with an antibiotic prescription filled within 1 week; upon review, some of these antibiotic prescriptions turned out to be preparatory for surgical or dental procedures. In the cases where surgical procedures were performed, those could be incorporated into the algorithm. However, since dental care (barring accidents) is not included in the claims data, no alternative use for the antibiotics could be inferred from the claims data.
The data sets also included an inherent selection bias, thus limiting generalizability of the results. Since the claims database we analyzed consisted of patients with commercial, employer, or Medicare advantage plans, this may not reflect differential prevalence rates that could be found in uninsured or Medicaid-insured populations; and furthermore, the rates are not directly comparable to the US population since there are differences in distribution by age, sex, and region. The claims sample with observed exacerbations appeared to have more severe COPD than the general COPD population; the average number of annual exacerbations of 1.48 per person may be representative of GOLD stage 3–4 disease (for which 1.34–2.00 annual exacerbations per person have been reported [Citation20]). Furthermore, the average number of annual exacerbations was 1.35 for patients who had an unobserved episode, compared with 2.8 for patients with observed episodes, suggesting that the patients with unobserved episodes did not have such severe COPD, which may explain why the predicted cost to treat these episodes was lower. A relatively large proportion of both the claims sample (30%) and the medical chart sample (18–19%) had undergone hospitalization with severe AECOPD, which are unlikely to represent hospitalization rates in the general COPD population. Future analyses may benefit from adjustments to the algorithm with regard to differences between definitions of AECOPD based on healthcare utilization and those including symptoms, in addition to hospitalization rates, as proposed by Erdal and colleagues [Citation19].
The data only comprised patients with continuous eligibility in a sample including few health plans and medical chart data obtained from a convenience sample of providers who were willing to participate. Additionally, as claim forms primarily contain only information necessary for compensation and often exclude details that are not essential for billing, some information may not have been recorded in either the claims data or the medical charts. Thus, true unobserved events from patients without contact with a healthcare provider would not be available from either claims-based or medical chart review. For this reason, the study may have yielded low estimates of the number and burden of unobserved exacerbations. This may represent a substantial proportion of the general COPD population, as in a retrospective analysis of individuals with airflow limitation; while 7.9% reported having had a COPD exacerbation in the prior year, 6.2% reported needing to visit a doctor, meaning that nearly a fifth of individuals experiencing a COPD exacerbation did not seek any medical assistance for their exacerbations [Citation30].
Conclusions
These findings represent an initial step in the creation of a useful algorithm for studying the epidemiology, costs and other aspects of AECOPD events, including assessment outcomes of differing management approaches, cost-effectiveness and mortality data. Our study revealed not only unobserved and incorrectly identified AECOPD episodes, but estimated the substantial financial impact associated with the inherent limitations of healthcare claims data as a primary source.
Although medical record evaluation was used to develop and refine the claims-based algorithm, this did not constitute a full validation and we recommend that future studies are conducted to fully validate the claims-based algorithm developed in this study.
Declarations
Disclosure of interest
At time of manuscript submission, Richard Stanford was employed by GlaxoSmithKline plc and owned shares in GlaxoSmithKline plc. Nicole Engel-Nitz, Timothy Bancroft and Breanna Essoi are employees of Optum, a consulting company that has received research funds from GlaxoSmithKline plc; Nicole Engel-Nitz and Timothy Bancroft own shares in UnitedHealth Group.
Compliance with ethics of experimentation
The study protocol, medical chart abstraction form and manual were approved by the study sponsor (GlaxoSmithKline plc.), by Optum, the affiliated health plan’s research review board (provided April 10, 2015) and the New England Institutional Review Board #15-258 (provided July 09, 2015). This study was undertaken only after the study protocol and study documents were approved and Optum was granted a Waiver of Authorization by the privacy board and a waiver of the informed consent requirement by the institutional review board. Health plan market medical directors were informed of the medical record review study and received the study synopsis, a copy of the network physician abstraction request letter, and a list of providers that were to be contacted for participation.
Acknowledgements
The authors would like to thank Feng Cao, PhD, Yiyu Fang, PhD and Thomas Horstman for programming the analytic datasets; James Hartje, MS, for programming the chart abstraction database and the analytic dataset; Nancy Coffman-Kadish, MS, Ciox Health for assistance in collection of medical charts and data abstraction and entry; and Aylin Altan, PhD, for consultation.
Data availability statement
The data contained in our database contains proprietary elements owned by Optum and, therefore, cannot be broadly disclosed or made publicly available at this time. The disclosure of these data to third-party clients assumes certain data security and privacy protocols are in place, and that the third-party client has executed our standard license agreement which includes restrictive covenants governing the use of the data.
Additional information
Funding
References
- World Health Organization. The top 10 causes of death . 2018. Available from: http://www.who.int/news-room/fact-sheets/detail/the-top-10-causes-of-death
- Global Initiative for Chronic Obstructive Lung Disease. Global strategy for the diagnosis, management, and prevention of chronic obstructive pulmonary disease . 2019. Available from: www.goldcopd.org
- Srivastava K , Thakur D , Sharma S , et al. Systematic review of humanistic and economic burden of symptomatic chronic obstructive pulmonary disease. Pharmacoeconomics. 2015;33(5):467–488. DOI:10.1007/s40273-015-0252-4
- American Thoracic Society . What is an exacerbation? Am J Respir Crit Care Med. 2014;189:P11–P12.
- Marks A . Managing acute exacerbations of COPD. US Pharmacist. 2009;34(7):HS-11–HS-15.
- Hawkins PE , Alam J , McDonnell TJ , et al. Defining exacerbations in chronic obstructive pulmonary disease. Expert Rev Respir Med. 2015;9(3):277–286. DOI:10.1586/17476348.2015.1046438
- Montes de Oca M , Laucho-Contreras ME . Is it time to change the definition of acute exacerbation of chronic obstructive pulmonary disease? What do we need to add? Med Sci (Basel). 2018;6(2):50. DOI:10.3390/medsci6020050
- Oba Y , Keeney E , Ghatehorde N , et al. Dual combination therapy versus long‐acting bronchodilators alone for chronic obstructive pulmonary disease (COPD): a systematic review and network meta‐analysis. Cochrane Systematic Review. Available from: https://www.cochranelibrary.com/cdsr/doi/10.1002/14651858.CD012620.pub2/full
- Anzueto A . Impact of exacerbations on COPD. Eur Respir Rev. 2010;19(116):113–118. DOI:10.1183/09059180.00002610
- Jones PW , Lamarca R , Chuecos F , et al. Characterisation and impact of reported and unreported exacerbations: results from ATTAIN. Eur Respir J. 2014;44(5):1156–1165. DOI:10.1183/09031936.00038814
- Frei A , Siebeling L , Wolters C , et al. The inaccuracy of patient recall for COPD exacerbation rate estimation and its implications: Results from central adjudication. Chest. 2016;150(4):860–868. DOI:10.1016/j.chest.2016.06.031
- Khwaja HA , Syed H , Cranston DW . Coding errors: a comparative analysis of hospital and prospectively collected departmental data. BJU Int. 2002;89(3):178–180. DOI:10.1046/j.1464-4096.2001.01428.x
- Toy EL , Gallagher KF , Stanley EL , et al. The economic impact of exacerbations of chronic obstructive pulmonary disease and exacerbation definition: a review. COPD. 2010;7(3):214–228. DOI:10.3109/15412555.2010.481697
- Shah T , Press VG , Huisingh-Scheetz M , et al . COPD readmissions: addressing COPD in the era of value-based Health Care. Chest. 2016;150(4):916–926. DOI:10.1016/j.chest.2016.05.002
- US Department of Labor, Bureau of Labor Statistics . Consumer Price Index. Medical care in U.S. city average, all urban consumers, not seasonally adjusted. Series ID: CUUR0000SAM. Available from: http://data.bls.gov/cgi-bin/surveymost?cu
- Celli BR , MacNee W , Agusti, AATS , et al. Standards for the diagnosis and treatment of patients with COPD: a summary of the ATS/ERS position paper. Eur Respir J. 2004;23(6):932–946. DOI:10.1183/09031936.04.00014304
- Stein BD , Bautista A , Schumock GT , et al. The validity of International Classification of Diseases, Ninth Revision, Clinical Modification diagnosis codes for identifying patients hospitalized for COPD exacerbations. Chest. 2012;141(1):87–93. DOI:10.1378/chest.11-0024
- Wu EQ , Birnbaum HG , Cifaldi M , et al. Development of a COPD severity score. Curr Med Res Opin. 2006;22(9):1679–1687. DOI:10.1185/030079906X115621
- Erdal M , Johannessen A , Eagan TM , et al. Incidence of utilization- and symptom-defined COPD exacerbations in hospital- and population-recruited patients. Int J Chron Obstruct Pulmon Dis. 2016;11:2099–2108. DOI:10.2147/COPD.S108720
- Hurst JR , Vestbo J , Anzueto A , et al. Susceptibility to Exacerbation in Chronic Obstructive Pulmonary Disease. N Engl J Med. 2010;363(12):1128–1138. DOI:10.1056/NEJMoa0909883
- Han MLK , Quibrera PM , Carretta EE , et al. Frequency of exacerbations in patients with chronic obstructive pulmonary disease: an analysis of the SPIROMICS cohort. Lancet Respir Med. 2017;5(8):619–626. DOI:10.1016/S2213-2600(17)30207-2
- Worth H , Buhl R , Criée CP , et al. GOLD 2017 treatment pathways in 'real life': An analysis of the DACCORD observational study. Respir Med. 2017;131:77–84. DOI:10.1016/j.rmed.2017.08.008
- Pasquale MK , Sun SX , Song F , et al. Impact of exacerbations on health care cost and resource utilization in chronic obstructive pulmonary disease patients with chronic bronchitis from a predominantly Medicare population. Int J Chron Obstruct Pulmon Dis. 2012;7:757–764. DOI:10.2147/COPD.S36997
- Elixhauser A , Au DH , Podulka J . Healthcare cost and utilization project (HCUP) statistical briefs [Internet]. Rockville (MD): Agency for Healthcare Research and Quality (US); 2006, Feb–2011 Sep.
- Perera PN , Armstrong EP , Sherrill DL , et al. Acute exacerbations of COPD in the United States: inpatient burden and predictors of costs and mortality. COPD. 2012;9(2):131–141. DOI:10.3109/15412555.2011.650239
- Jinjuvadia C , Jinjuvadia R , Mandapakala C , et al. Trends in outcomes, financial burden, and mortality for acute exacerbation of chronic obstructive pulmonary disease (COPD) in the United States from 2002 to 2010. COPD. 2017;14(1):72–79. DOI:10.1080/15412555.2016.1199669
- Global Initiative for Asthma and Global Initiative for Chronic Obstructive Lung Disease. Asthma, COPD and Asthma-COPD overlap syndrome . 2015. Available from: https://goldcopd.org/asthma-copd-asthma-copd-overlap-syndrome/
- Wurst KE , St Laurent S , Hinds D , et al. Disease burden of patients with asthma/COPD overlap in a US claims database: impact of ICD-9 coding-based definitions. COPD. 2017;14(2):200–209. DOI:10.1080/15412555.2016.1257598
- Ferver K , Burton B , Jesilow P . The use of claims data in healthcare research. Open Public Health J. 2009;2(1):11–24. DOI:10.2174/1874944500902010011
- Montes de Oca M , Tálamo C , Halbert RJ , et al. Frequency of self-reported COPD exacerbation and airflow obstruction in five Latin American cities: the Proyecto Latinoamericano de Investigacion en Obstruccion Pulmonar (PLATINO) study. Chest. 2009;136(1):71–78. DOI:10.1378/chest.08-2081