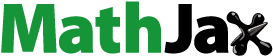
Abstract
Preclinical studies suggest interleukin (IL)-1α/β is involved in the pathogenesis of chronic obstructive pulmonary disease (COPD). However, recent trials of anti-IL-1 therapies showed limited benefit for COPD. To clarify, we primarily examined total and direct effects of IL-1 and its receptors/coreceptors/receptor antagonists (IL-1/IL-1Rs) on airflow obstruction (AO) using Mendelian randomization (MR), and secondarily explored reverse causation using bidirectional MR. We selected independent cis protein quantitative trait loci (cis-pQTLs) as genetic instruments for IL-1/IL-1Rs from two proteomic genome-wide association studies (n = 11,594) of European ancestry (mean age ∼47 years). We applied those cis-pQTLs to the International COPD Genetics Consortium (n = 15,256 cases, 47,936 controls) of ∼81.9% European descent (∼57 years). No IL-1/IL-1Rs were significantly associated with AO after correction for multiple testing. However, a higher genetically predicted IL-1 receptor antagonist (IL-1Ra) was nominally associated with a 20% reduction in AO risk using univariable MR, with a larger direct effect (∼31%, i.e. not via IL-1α/β) using multivariable MR. Furthermore, higher total IL-18 binding protein (IL-18BP) was nominally associated with lower AO. Nominal total effects were also noted for higher IL-1α with lower AO and higher IL-1R1 with higher AO. Higher IL-1Ra and IL-18BP might have a role in preventing AO, but need to be contextualized.
Supplemental data for this article is available online at https://doi.org/10.1080/15412555.2021.1955848 .
Introduction
Chronic obstructive pulmonary disease (COPD) is a leading cause of morbidity and mortality worldwide [Citation1]. In addition to airway remodeling, COPD involves chronic airway inflammation, which is characterized by persistent respiratory symptoms and airflow obstruction (AO) due to the abnormal release of inflammatory cytokines and dysregulated immune cell activity [Citation2]. Damage from inhaled noxious particles, such as from smoking, air pollution, and fuel combustion, induces interleukin (IL)-1 release, comprising IL-1α/β, IL-18, IL-33, IL-36α/β/γ, IL-37 [Citation3], along with other cytokines. IL-1 is thought to be implicated in COPD by interacting with their receptors/coreceptors and receptor antagonists [Citation2, Citation4]. COPD is incurable and existing treatments only relieve symptoms. Given anti-IL-1/IL-1Rs therapies are widely used as anti-inflammatories condition, such as rheumatoid arthritis, a better understanding of their etiological roles in COPD could inform their potential for preventing COPD [Citation5].
Clinical trials of anti-inflammatory therapeutics, such as MEDI8969 [IL-1R1 monoclonal antibody] [Citation6], canakinumab [IL-1β monoclonal antibody] [Citation7], mepolizumab [IL-5 monoclonal antibody] [Citation8], benralizumab [IL-5 receptor monoclonal antibody] [Citation9], CNTO6785 [IL-17 monoclonal antibody] [Citation10], MK7123 [cytokine receptor CXCR2 antagonist] [Citation11], and tumor necrosis factor α antagonist [Citation12], have shown limited benefit in preventing the development or exacerbation of COPD. The relatively small number of COPD cases in these trials, competing risks, and the inclusion of late-stage COPD patients may partially explain the null results [Citation2]. Patients with stable COPD may have eosinophilic or neutrophilic inflammation [Citation13,Citation14], and elevated IL-1β and IL-17 [Citation15], making it unclear whether IL-1-mediated chronic inflammation increases the risk of developing COPD or alternatively whether COPD promotes the dysregulation of IL-1/IL-1Rs.
Observationally, IL-1/IL-1Rs are related to the risk of developing COPD [Citation2,Citation4,Citation16,Citation17]. For example, a recent study summarizing preclinical evidence has suggested that IL-1α and IL-1β, dysregulating IL-1 signaling, play important roles in the pathogenesis of COPD [Citation2]. Moreover, IL-1α, regulating the airway epithelium and lung fibroblasts, appears to be associated with COPD risk [Citation17]. However, these studies are open to confounding by factors such as health status or smoking. Mendelian randomization (MR) provides an alternative approach by using genetic variants to proxy an exposure, so as to obtain less confounded estimates. We used MR to obtain total effects and direct effects of 12 interleukins (IL-1α/β, IL-1Ra, IL-1R1, IL-1Racp, IL-18, IL-18BP, IL-18Rα, IL-36α/β/γ, and IL-37) on AO; and secondarily to assess whether liability to AO affects these interleukins. Here, we considered AO as the outcome, which helps identify people at risk of COPD exacerbations and mortality [Citation18], because only pre-bronchodilator spirometry is used by the International COPD Genetics Consortium (ICGC) to define COPD [Citation19]. Pre-bronchodilator measurements are often used in clinical practice and epidemiological studies [Citation1,Citation8,Citation9], although post-bronchodilator spirometry measurements are recommended by the Global Initiative for Chronic Obstructive Lung Disease (GOLD) [Citation20]. We also used tuberculosis as a negative control outcome to assess the credibility of the MR estimates.
Material and methods
Study design
Here, we used two-sample univariable and multivariable MR to assess three possible relations between IL-1/IL-1Rs and AO. First, IL-1/IL-1Rs might affect the development of COPD (). Second, patients with AO might have higher IL-1/IL-1Rs than other people (). Third, the effect of IL-1 on AO could be partially mediated by its receptors and receptor antagonists (i.e. IL-1Rs, ). As such, IL-1 would have an indirect effect on AO, perhaps via IL-1Rs. MR rests on three key assumptions. First, relevance, i.e. the genetic instruments predict the exposure. Second, independence, i.e. the genetic instruments are not confounded. Third, exclusion restriction, i.e. the genetic instruments are only linked to the outcome via affecting the exposure.
Figure 1. Directed acyclic graphs depicting three possible scenarios that could explain cause effects between interleukin (IL)-1/IL-1 receptors/receptor antagonists (IL-1Rs) and airflow obstruction (AO). (A) IL-1/IL-1Rs have effects on AO. (B) AO has reverse effects on IL-1/IL-1Rs. (C) IL-1 has an indirect effect on AO, perhaps via its receptors (IL-1Rs). Under the multivariable MR framework, the direct effect of IL-1 on AO is estimated as β, whilst its indirect effect is estimated as αγ. The total effect can be estimated as β+αγ .
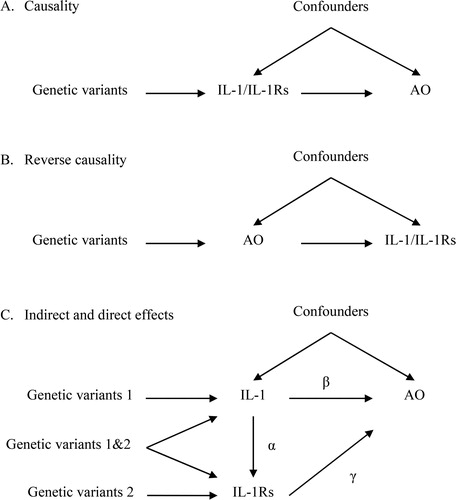
Data source
Proteomics GWAS studies
Two proteomic genome-wide association studies (GWAS) studies recruited in up to 11,594 European participants (including 3301 from the INTERVAL study [Citation21] and up to 8293 from YFS and FINRISK survey [Citation22]) with an average age of ∼47.0 years. The proteomic GWAS was adjusted for age, sex, body mass index, time between blood draw and processing, and the first three or ten genetic principal components. Genetic predictors of cis protein quantitative trait loci (cis-pQTLs, which affect protein abundance with little or no attenuated effect on messenger RNA or ribosome levels concerning trans-pQTLs [Citation23]) were obtained from summary statistics for IL-1 (i.e. IL-1α [log-transformed relative fluorescent unit, log (RFU) [Citation24]; IL-1β [standard-deviation (SD, ∼2.2 pg/ml in YFS)], IL-1Ra [SD, ∼3.5 ng/ml in FINRISK2002], IL-18 [SD, ∼38.0 pg/ml in YFS], IL-36α [log(RFU)], IL-36β [log(RFU)], IL-36γ [log(RFU)], and IL-37 [log(RFU)]) and IL-1Rs (i.e. IL-1R1 [log(RFU)], IL-1Racp [log(RFU)], IL-18Rα [log(RFU)], IL-18 binding protein [IL-18BP, log(RFU)]).
ICGC
ICGC recruited 82,438 participants (24,754 COPD cases and 57,684 controls) into a two-stage genome-wide association meta-analysis [Citation19]. Considering that the definitions of COPD cases and non-COPD controls in ICGC were based on pre-bronchodilator spirometry data: forced expiratory volume in 1 s (FEV1) < 80% and FEV1 to forced vital capacity (FVC) ratio of <0.7 for COPD cases and FEV1 > 80% and FEV1/FVC > 0.7 for non-COPD controls, misdiagnosis of COPD may happen according to recommendations in GOLD [Citation20]. Hence, we considered cases with AO and controls based on the pre-bronchodilator spirometry data in ICGC [Citation18]. Specifically, in stage 1 (discovery), a total of 22 studies with genome-wide associations and 4 COPD case-control or cohort studies with spirometry, including 15,256 AO cases and 47,936 normal controls, were included in a fixed-effects meta-analysis after adjustment for age, sex, pack-years of smoking, ever and current smoking status, and ancestry-based principal components. In the stage 1 analysis, the mean age of participants was ∼56.4 years, ∼50.7% were women, and ∼81.9% were of European descent. Also, 71.0% were ever smokers with an average 22.2 pack-years. In the stage 2 analysis which included the UK BiLEVE study as a validation of the stage 1 findings, the mean age of participants was ∼57.9 years, ∼48.1% were women, 55.3% ever smokers with an average 22.5 pack-years. More detail on baseline characteristics of participants included in ICGC is described elsewhere [Citation19]. We selected genetic variants for AO using ICGC stage 1 analysis to avoid potential bias due to possible population stratification [Citation25], given the INTERVAL study and ICGC might have different allele frequencies for those cis-pQTLs and thereby confound the instrument-outcome association. We conducted MR analyses using the publicly available summary statistics from dbGaP under study accession: phs000179.v6.p2.
Statistical analysis
Instruments selection
shows the analytical framework of two-sample MR used in this study. We selected pQTLs potentially predicting IL-1/IL-1Rs on the basis of a threshold of p <5 × 10–6 [Citation26]. We checked these pQTLs for accurate identification and description in RegulomeDB (https://regulomedb.org/regulome-search/) to reduce random variability [Citation27]. Cis-pQTLs were identified by excluding pQTLs with expression quantitative trait loci (eQTLs) [Citation23] in either RegulomeDB or PhenoScanner (http://www.phenoscanner.medschl.cam.ac.uk/) to avoid unknown pleiotropy. We identified independent cis-pQTLs (r2 < 0.01) based on the 1000 genomes European reference panel obtained from LDlink (https://ldlink.nci.nih.gov/). To reduce the possibility of selection bias and unknown pleiotropy, we further excluded SNPs associated with other causes of death and possible confounders of IL-1/IL-1Rs on COPD (e.g. smoking and eosinophils) in PhenoScanner at the genome-wide significance
). We calculated the F-statistic as the square of cis-pQLTs on exposure divided by the square of its standard error and excluded those with an F-statistic less than the rule of thumb of 10 to minimize weak instrument bias [Citation28].
Two-sample MR analyses
We applied the identified cis-pQLT genetic variants to summary statistics from ICGC. We replaced poorly genotyped cis-pQLTs with available proxy variants in high linkage disequilibrium (r2 < 0.80) obtained using LDlink. We aligned genetic variants for IL-1/IL-1Rs and COPD on the same effect allele by flipping the non-palindromic strand variants, and removed palindromic (MAF threshold = 0.3) and incompatible variants. MR estimates for IL-1/IL-1Rs on AO were obtained by pooling Wald estimates (i.e. the ratio of the genetic variant-outcome effect and the genetic variant-exposure effect) using inverse-variance weighting with random-effects meta-analysis, to obtain total effects. We detected heterogeneity using Cochran’s Q-test.
In sensitivity analyses, fixed-effects with inverse-variance weighting, a weighted median [Citation29], MR-Egger [Citation30], and MR robust adjusted profile score (MR-RAPS) [Citation31] were used. The weighted median method provides consistent estimates when at least 50% of the weight is from valid instrumental variants [Citation29]. MR-Egger detects the presence of genetic pleiotropy using the nonzero MR Egger intercept [Citation30]. MR-RAPS addresses systematic and idiosyncratic pleiotropy (i.e. balanced pleiotropic effects with an allowance of some large values), where no genetic variant satisfies the exclusion restriction assumption, and yields robust estimates in the presence of weak instruments [Citation31].
We used bidirectional MR [Citation32] to check the direction of causality (); and multivariable MR based on robust weighted linear regression [Citation33] to investigate whether IL-1/IL-1Rs directly effects the risk of developing AO after adjustment for genetically predicted effects of other IL-1/IL-1Rs (). In the multivariable MR, only genetic variants independent across the relevant exposures were used. We calculated the likely lower bound of conditional F-statistic to assess multivariable instrument strength, assuming no correlation between IL-1 and IL-1Rs [Citation34]. We also used multivariable MR Egger oriented on the exposure of interest and the modified Q-statistic to examine the presence of unknown pleiotropy with an assumption about no correlation between exposure, which yields conservative causal estimates [Citation34]. Furthermore, we validated our results using summary statistics for doctor-diagnosed COPD from the UK Biobank [Citation35].
Finally, we used tuberculosis from the FinnGen study [Citation36] as a negative control outcome to cross-validate the MR estimates of IL-1/IL-1Rs on AO, which are particularly vulnerable to biases [Citation37–40]. Tuberculosis is a negative control outcome because it not only shares the same potential biases but also has a known cause (mycobacterium tuberculosis infection) [Citation41,Citation42]. That is, IL-1/IL-1Rs would not be expected to affect the risk of developing tuberculosis in the absence of mycobacterium tuberculosis infection. As such, any associations found for IL-1/IL-1Rs with tuberculosis would indicate biased MR estimates.
Power analysis
Considering that genetic instruments explained a median variation in IL-1/IL-1Rs of 5.8% in the proteomics GWAS [Citation21,Citation22], the study has ∼80% power at 5% alpha to detect an odds ratio of 0.90 for AO and 0.61 for tuberculosis using the online calculator (https://shiny.cnsgenomics.com/mRnd/) [Citation43,Citation44].
Statistical software
All statistical analyses were conducted using R version 3.6.2 software platform (https://cran.r-project.org/) with the HalopR (https://github.com/cluoma/haloR), ieugwasr (https://mrcieu.github.io/ieugwasr/index.html), TwoSampleMR (https://mrcieu.github.io/TwoSampleMR/index.html), and robustMVMR (https://cran.r-project.org/web/packages/robustMVMR/index.html) packages. We used p values at the Bonferroni-corrected threshold to define statistical significance, which was computed as 0.05/3 (for three sub-IL-1 families)/2 (for two outcomes)/2 (for the bidirectional MR) = 0.004. p values between 0.004 and 0.05 were reported as nominal. We adhered to STROBE-MR: Guidelines for strengthening the reporting of MR studies for reporting our results [Citation38].
Results
A total of 123 genetic variants were identified as instruments for IL-1/IL-1Rs from the two proteomic GWAS (Supporting Information Table S1), including one variant (rs61335305) that predicted both IL-1β and IL-1Ra. None of these genetic variants were associated with potential confounders or other causes of death at 5 × 10−8. Of these 123 genetic variants, 57 remained after excluding palindromic variants and variants incompatible with COPDGene, as shown in Supporting Information Tables S2-S3. The average F-statistic ranged from 14.6 to 215.3, indicating lack of weak instruments. A total of 13 genetic variants were identified as instruments for liability to AO from ICGC stage 1. From which, we excluded rs17486278 because it is associated with cigarettes per day [Citation45] a potential confounder of IL-1/IL-1Rs on COPD and rs754388 because it is associated with eosinophils count [Citation46] and may be pleiotropic, leaving 11 genetic instruments for liability to AO (Supporting Information Tables S4-S5). The average F-statistic varied from 29.0 to 104.8, indicating instrument strength.
Total and direct effects of IL-1/IL-1Rs on AO
show estimates for 12 genetically predicted IL-1/IL-1Rs-mediated inflammation cytokines on AO and the negative control outcome of tuberculosis using both univariable and robust multivariable MR. No significant associations of IL-1/IL-1Rs with AO were noted after correction for multiplicity. However, genetically predicted higher IL-1α was nominally associated with a 13% reduction in risk of developing AO, with no heterogeneity identified (Q-statistic = 3.13, p = 0.792). The MR Egger intercepts showed no evidence of pleiotropy (Supporting Information Table S6). However, such an association diminished after adjustment for genetically predicted effects of other IL-1/IL-1Rs. Multivariable MR Egger intercepts indicated possible pleiotropy (p = 0.004), but not the modified Q-statistic (p = 0.675). The conditional F-statistic suggested weak instrumental strength, as shown in Supporting Information Table S7. Total effects based on the conventional multivariable MR [Citation47,Citation48] yielded similar estimates (Supporting Information Table S8).
Figure 3. Causal estimates of genetically predicted interleukin (IL)-1α/β, IL-1 receptor antagonist (IL-1Ra), IL-1 recetpor-type 1 (IL-R1), and IL-1 receptor accessory protein (IL-1Racp) with airflow obstruction (AO) and the negative control outcome of tuberculosis using univariable and multivariable Mendelian randomization analyses. Multivariable MR* adjusted either IL-1
α/β or IL-1Ra; and multivariable MR** adjusted either IL-1
α/β, IL-1Ra, IL-1R1, IL-1Racp, IL-18, IL-18BP, IL-18Rα
, IL-36α/β/γ
, or IL-37. The modified Q-statistic for multivariable MR* estimates was 6.63 with p = 0.675, and the corresponding value for multivariable MR** estimates was 33.48 with p = 0.945. IL-18: interleukin-18; IL-18BP: interleukin-18 binding protein; IL-18Rα: interleukin-18 receptor 1; IL-36α/β/γ: interleukin-36 α/β/γ; IL-37: interleukin-37.
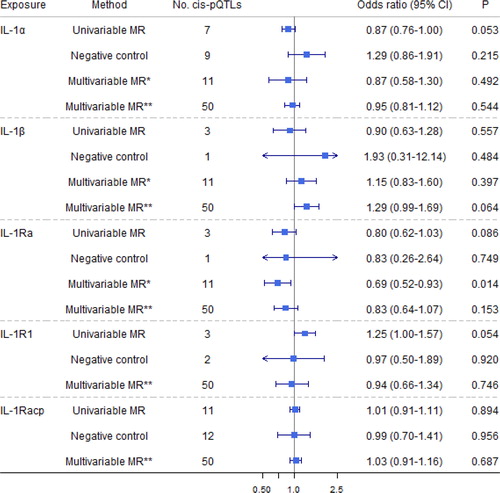
Figure 4. Causal estimates of genetically predicted interleukin (IL)-18, IL-18 binding protein (IL-18BP), IL-18 receptor 1 (IL-18Rα
), and IL-37 with airflow obstruction (AO) and the negative control outcome of tuberculosis using univariable and multivariable Mendelian randomization analyses. Multivariable MR* adjusted either IL-18, IL-18BP, IL-18Rα
, or IL-37; and multivariable MR** adjusted either IL-1
α/β, IL-1Ra, IL-1R1, IL-1Racp, IL-18, IL-18BP, IL-18Rα
, IL-36α/β/γ
, or IL-37. The modified Q-statistic for multivariable MR* estimates was 4.36 with p = 0.824, and the corresponding value for multivariable MR** estimates was 33.48 with p = 0.945. IL-1α: interleukin-1 α; IL-1β: interleukin-1 β; IL-1Ra: interleukin-1 receptor antagonist; IL-1R1: interleukin-1 receptor-type 1; IL-37: interleukin-37.
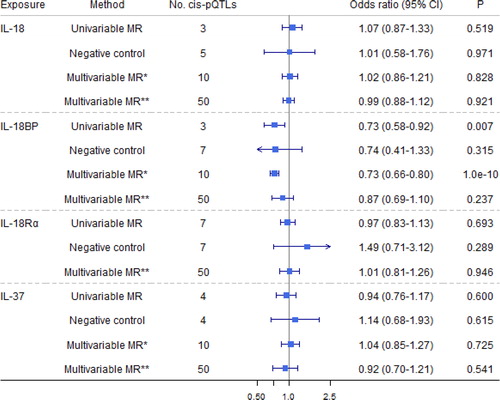
Figure 5. Causal estimates of genetically predicted interleukin (IL)-36α, IL-36β
, and IL-36γ
with airflow obstruction (AO) and the negative control outcome of tuberculosis using univariable and multivariable Mendelian randomization analyses. Multivariable MR* adjusted either IL-36α
, IL-36β
, or IL-36γ
; and multivariable MR** adjusted either IL-1
α/β, IL-1Ra, IL-1R1, IL-1Racp, IL-18, IL-18BP, IL-18Rα
, IL-36
α/β/γ, or IL-37. The modified Q-statistic for multivariable MR* estimates was 1.52 with p = 0.997, and the corresponding value for multivariable MR** estimates was 33.48 with p = 0.945. IL-1α: interleukin-1 α; IL-1β: interleukin-1 β; IL-1Ra: interleukin-1 receptor antagonist; IL-18: interleukin-18; IL-18BP: interleukin-18 binding protein; IL-1R1: interleukin-1 receptor-type 1; IL-18Rα: interleukin-18 receptor 1; IL-37: interleukin-37.
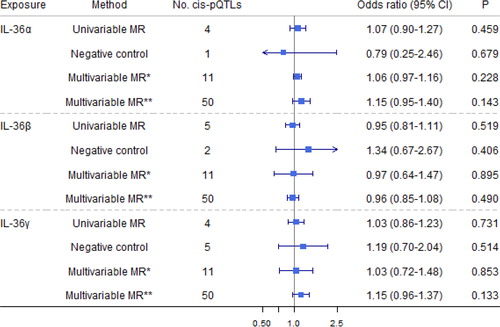
Genetically predicted IL-1Ra was nominally associated with a 20% decreased risk of AO (), with no heterogeneity (Q-statistic = 3.15, p = 0.207) or pleiotropy identified (p = 0.708). Such an association remained after adjustment for genetically predicted effects of IL-1α and IL-1β but diminished after adjustment for other IL-1/IL-1Rs. Genetically predicted IL-1β nominally increased risk of AO after adjustment for genetically predicted effects of other IL-1/IL-1Rs, but with no total effect. Genetically predicted higher IL-1R1 was nominally associated with a 25% higher risk of AO with no heterogeneity (Q-statistic = 0.84, p = 0.659) or pleiotropy (p = 0.536). However, such an association diminished after adjustment for genetically predicted other IL-1/IL-1Rs. Multivariable MR Egger intercepts (p = 0.913) and the modified Q-statistic (p = 0.945) did not indicate pleiotropy. Our results are consistent with the total effect of IL-1Ra on the risk of doctor-diagnosed COPD obtained from summary-level statistics in UK Biobank (Supporting Information Table S9).
Genetically predicted IL-18BP was nominally associated with a 27% decreased risk of AO (), with no heterogeneity (Q-statistic = 0.07, p = 0.966) or pleiotropy (p = 0.867). Such an association remained after adjustment for genetically predicted effects of IL-18 and IL-37 but diminished after adjusting for other IL-1/IL-1Rs. Multivariable MR Egger intercepts (p = 0.041, Supporting Information Table S7) suggested possible pleiotropy, but not the modified Q-statistic (p = 0.945). Sensitivity analyses yielded consistent results (Supporting Information Table S6).
Total effects of liability to AO on IL-1/IL-1Rs
No associations of liability to AO on IL-1/IL-1Rs were observed (). The MR Egger intercepts did not indicate pleiotropy. In addition, sensitivity analyses yielded consistent results, as shown in Supporting Information Table S10.
Figure 6. Causal estimates of genetically predicted airflow obstruction (AO) on interleukin (IL)-1 and their receptors and receptor antagonists using univariable Mendelian randomization analyses. IL-1α: interleukin-1 α; IL-1β: interleukin-1 β; IL-1Ra: interleukin-1 receptor antagonist; IL-18: interleukin-18; IL-18BP: interleukin-18 binding protein; IL-1R1: interleukin-1 receptor-type 1; IL-18Rα: interleukin-18 receptor 1; IL-36α/β/γ: interleukin-36 α/β/γ; IL-37: interleukin-37.
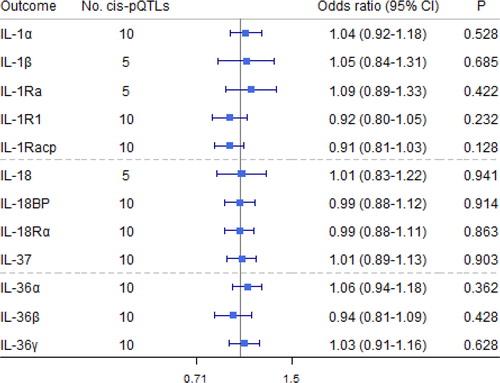
Negative control analyses
No associations of IL-1/IL-1Rs with tuberculosis were observed ( and Supporting Information Table S6).
Discussion
Principal findings
This first MR study comprehensively assessing the roles of IL-1/IL-1Rs in the risk of developing AO provided genetic evidence for some suggestive causal associations of higher IL-1Ra and higher IL-18BP with decreased risk of AO. There was also some evidence for possible associations of higher IL-1α with decreased risk of AO and higher IL-1R1 with increased risk of AO. Furthermore, higher IL-1β was weakly associated with increased risk of AO independent of other IL-1/IL-1Rs.
Comparison with other studies
Our results are consistent with a previous review showing neutralizing antibodies to IL-18 (i.e. mimicking IL-18BP to block the effect of IL-18) have efficacy in preclinical models of inflammation and COPD or AO [Citation49]. Evidence of higher IL-18BP decreased risk of AO was particularly strong after adjusting for genetically predicted effects of IL-18 and IL-37 (). Moreover, IL-18 is associated with some auto-immune diseases, such as inflammatory bowel disease [Citation50] and immune-mediated diseases [Citation51], further supporting our results. Nevertheless, more testing of the IL-18 related pathways might be helpful to understand its role in AO better.
Strengths and limitations
This MR study considered several possible scenarios so as to obtain causal effects of IL-1/IL-1Rs on AO. We also used tuberculosis as the negative control outcome to assess the credibility of our instruments. Given the highly correlated IL-1 family, we used robust multivariable MR, which is less subject to conditionally weak instrument bias [Citation52]. Furthermore, our findings are less likely to open to selection bias because of the relatively young average age (∼57 years) of participants in ICGC (i.e. few deaths would have occurred before the recruitment). The opposite direction concerning direct effects of IL-1β and IL-1Ra with AO further substantiates our findings, given the antagonistic effects of IL-1β and IL-1Ra on AO [Citation2].
However, several limitations are noted. First, MR requires stringent assumptions of relevance, independence, and exclusion-restriction. To satisfy the relevance assumptions, we restricted the instruments to well-genotyped and curated cis-pQTLs strongly predicting IL-1/IL-1Rs. Any “winner’s curse” due to selecting instruments at a significance level of 5 × 10−6 might bias toward null [Citation53]. To satisfy independence, we checked for potential confounders. Use of non-overlapping GWAS make our findings less likely biased by population stratification. To satisfy exclusion-restriction, we checked for known pleiotropy, but unknown pleiotropy might exist because of the limited understanding of these selected cis-pQTLs for predicting IL-1/IL-1Rs. Selection bias due to inevitably selecting survivors of their genetic make-up, AO and any competing risk of AO may remain. The multivariable MR Egger intercepts also suggest no genetic pleiotropy (Supporting Information Table S7). Second, genetic instruments typically only explain a small proportion of the variance in the exposure, as here. This might result in underpowered results, although multivariable MR often has more power than univariable MR by including more instruments at the cost of relatively more stringent assumption on horizontal pleiotropy. Third, the genetic instruments were adjusted for body mass index meaning that any effects of interleukins on AO via body mass index may not be evident [Citation54]. However, such an effect might be limited as participants being unwell or with illness or infection were ineligible for donation in the INTERVAL study [Citation55]. Fourth, canalization buffering genetic factors may exist and bias the causal estimates with unknown magnitude and direction. Fifth, effects of IL-1/IL-1Rs may vary by COPD subtype, including small airway disease and emphysema. We could not conduct subtype-specific analyses due to the lack of sub-type specific COPD GWAS. Sixth, given these GWAS mainly included healthy people of European descent, our findings may not extend to other populations, although causes are always consistent but may not be relevant in different populations [Citation56]. Seventh, MR estimates proxy lifetime exposure to natural genetic variation rather than effects of short-term interventions.
In addition, we considered cases with AO and controls based on pre-bronchodilator spirometry in ICGC with fixed values of 80% for FEV1 and 0.7 for FEV1/FVC, instead of the 5th percentile of post-bronchodilator spirometry [Citation20,Citation57,Citation58]. The mean age of participants in stage 1 in ICGC was ∼56.4 years (ranging from 40.5 to 79.5 years in sub-studies) [Citation19]. We cannot rule out the possibility that using pre-bronchodilator spirometry may misclassify some asthmatic participants as having AO, whereas overdiagnosis in the elderly and underdiagnosis of AO in younger participants could occur due to the use of age-biased fixed ratio FEV1/FVC instead of the 5th percentile as an unbiased lower limit of normal [Citation57,Citation58]. Although, the outcome was classified in ICGC independent of the exposure (interleukin), inevitably, some non-differential misclassification of AO exists, which may bias toward the null [Citation59]. To further validate our results, we applied the selected genetic instruments for IL-1/IL-1Rs to doctor-diagnosed COPD (1658 cases and 110,925 controls) in the UK Biobank and to early-onset COPD (diagnosed age <65 years; 1231 cases and 67,360 controls) and late-onset COPD (diagnosed age 60+ years; 1030 cases and 67,360 controls) in FinnGen using univariable MR. Consistent results were found, as shown in Supporting Information Table S9.
Mechanistically, IL-1Ra, competing for binding to IL-1R1 with IL-1α/β, is thought to regulate the function of IL-1α/β (e.g. blocking the effects of IL-1α/β), which contributes to repair, remodeling, and fibrosis of the lung [Citation60]. IL-18 is partially regulated by IL-18BP in Th1 and Th2 cell development, inducing pulmonary inflammation and lung injury, and involving the pathogenesis of AO or COPD [Citation61]. The alternative biological pathway (e.g. T helper lymphocytes-mediated immune response [Citation62,Citation63] and pattern recognition receptors-mediated innate immune response [Citation64]) from IL-1/IL-1Rs to AO or COPD might exist as indicated by the consistent total effects of increased IL-1α and increased IL-1Ra on the decreased risk of AO. For instance, IL-1/IL-1Rs has been shown to be strongly associated with increased neutrophils and eosinophils as well as COPD exacerbations in COPD patients [Citation14,Citation65]. As such, despite the opposite effects of IL-1α and IL-1Ra on AO as would be expected, the interplay between IL-1 and its receptors/receptor antagonists are likely complex.
Public health implications
Primary prevention is important to reduce the COPD-related disease burden, but management and treatment of COPD remains challenging, largely due to being a heterogeneous, late-onset disease with complex pathophysiology [Citation66–68]. Clarifying the role of interleukins provides additional insight. Replication using non-Western populations would be valuable to cross-validate our findings when such large-scale GWAS become available. IL-1Ra is increased by the drug anakinra, which is used as a treatment for rheumatoid arthritis, and possibly acts on immune function via raising testosterone in men [Citation69], especially among those with metabolic syndrome and low testosterone [Citation70]. Anakinra was also considered as a possible treatment for cardiovascular disease, but genetically mimicking anakinra appears to increase risk [Citation71,Citation72], which might need to be taken into account.
Conclusion
This MR study provides evidence suggesting increasing IL-1Ra and IL-18BP might have roles in preventing AO in people of European descent, perhaps via IL-1α/β, IL-18, and IL-37. A better understanding of the etiological roles of IL-1/IL-1Rs in chronic diseases could be relevant to reducing the worldwide burden of COPD.
Ethics approval and consent to participate
Each study has been specifically approved by the Ethical Committees of the original studies and all the participants provided a written informed consent. This analysis of publicly available data does not require ethical approval.
Authors contribution
ZY and MKK analyzed and interpreted the data regarding COPD, and were major contributors in writing the manuscript. All authors read and approved the final manuscript.
Consent for publication
Not applicable.
AO | = | airflow obstruction |
cis-pQTLs | = | cis protein quantitative trait loci |
CI | = | confidence interval |
COPD | = | chronic obstructive pulmonary disease |
FVC | = | forced vital capacity |
eQTLs | = | expression quantitative trait loci |
GWAS | = | genome-wide association study |
ICGC | = | International COPD Genetics Consortium |
IL-1 | = | interleukin-1 |
IL-1α | = | interleukin-1α |
IL-1β | = | interleukin-1β |
IL-1R1 | = | interleukin-1 receptor-type 1 |
IL-1Ra | = | interleukin-1 receptor antagonist |
IL-1Racp | = | interleukin-1 receptor accessory protein |
IL-18 | = | interleukin-18 |
IL-18BP | = | interleukin-18 binding protein |
IL-18Rα | = | interleukin-18 receptor 1 |
IL-36α | = | interleukin-36α |
IL-36β | = | interleukin-36β |
IL-36γ | = | interleukin-36γ |
IL-37 | = | interleukin-37 |
MR | = | Mendelian randomization |
MVMR | = | multivariable Mendelian randomization |
OR | = | odds ratio |
RFU | = | relative fluorescent unit |
SD | = | standard deviation |
Acknowledgment
This research used data generated by the COPDGene study, which was supported by NIH grants U01 HL089856 and U01 HL089897. The COPDGene project is also supported by the COPD Foundation through contributions made by an Industry Advisory Board comprised of Pfizer, AstraZeneca, Boehringer Ingelheim, Novartis, and Sunovion. This research has been conducted using the data (dbGaP study accession: phs000179.v6.p2) granted through the Database of Genotypes and Phenotypes (dbGaP) under application number 23474. The authors also thank the participants and investigators of the INTERVAL and FinnGen studies.
Declaration of interest
The authors have declared no conflicts of interest.
Funding
This research received no specific grant from any funding agency in public, commercial, or not-for-profit sectors.
Data availability
The summary statistics for genetic associations of IL-1α, IL-1R1, IL-1Racp, IL18BP, IL18Rα, IL-36α, IL-36β, IL-36γ, IL-37 are available in the INTERVAL study (http://www.phpc.cam.ac.uk/ceu/proteins/). The summary statistics for genetic associations of IL-1β, IL-1Ra, IL-18 are available in YFS and FINRISK survey (https://grasp.nhlbi.nih.gov/FullResults.aspx). The summary genetic statistics for genetic associations of chronic obstructive pulmonary disease are available in the Genetic Epidemiology of COPD (dbGaP study accession: phs000179.v6.p2). The annotated QTLs are available in RegulomeDB (https://regulomedb.org/regulome-search/) and PhenoScanner (http://www.phenoscanner.medschl.cam.ac.uk/). The 1000 genomes European reference panel is available via LDlink (https://ldlink.nci.nih.gov/).
Code availability
Software codes and data are available on request.
References
- Global Burden of Disease Chronic Respiratory Disease Collaborators. Prevalence and attributable health burden of chronic respiratory diseases, 1990-2017: a systematic analysis for the Global Burden of Disease Study 2017. Lancet Respir Med. 8(6):585–596.
- Osei ET, Brandsma CA, Timens W, et al. Current perspectives on the role of interleukin-1 signalling in the pathogenesis of asthma and COPD. Eur Respir J. 2020;55(2):1900563. DOI:10.1183/13993003.00563-2019
- Mantovani A, Dinarello CA, Molgora M, et al. Interleukin-1 and related cytokines in the regulation of inflammation and immunity. Immunity. 2019;50(4):778–795. DOI:10.1016/j.immuni.2019.03.012
- Pauwels NS, Bracke KR, Dupont LL, et al. Role of IL-1α and the Nlrp3/caspase-1/IL-1β axis in cigarette smoke-induced pulmonary inflammation and COPD. Eur Respir J. 2011;38(5):1019–1028. DOI:10.1183/09031936.00158110
- Dinarello CA, Simon A, van der Meer JW. Treating inflammation by blocking interleukin-1 in a broad spectrum of diseases. Nat Rev Drug Discov. 2012;11(8):633–652. DOI:10.1038/nrd3800
- Calverley PMA, Sethi S, Dawson M, et al. A randomised, placebo-controlled trial of anti-interleukin-1 receptor 1 monoclonal antibody MEDI8968 in chronic obstructive pulmonary disease. Respir Res. 2017;18(1):153. DOI:10.1186/s12931-017-0633-7
- Novartis. Safety and efficacy of multiple doses of canakinumab (ACZ885) in chronic obstructive pulmonary disease (COPD) patients; 2007. [cited 2020 Oct 15]. Available from: https://clinicaltrials.gov/ct2/show/NCT00581945
- Pavord ID, Chanez P, Criner GJ, et al. Mepolizumab for eosinophilic chronic obstructive pulmonary disease. N Engl J Med. 2017;377(17):1613–1629. DOI:10.1056/NEJMoa1708208
- Criner GJ, Celli BR, Brightling CE, et al. Benralizumab for the prevention of COPD exacerbations. N Engl J Med. 2019;381(11):1023–1034. DOI:10.1056/NEJMoa1905248
- Eich A, Urban V, Jutel M, et al. A Randomized, placebo-controlled phase 2 trial of CNTO 6785 in chronic obstructive pulmonary disease. COPD. 2017;14(5):476–483. DOI:10.1080/15412555.2017.1335697
- Rennard SI, Dale DC, Donohue JF, et al. CXCR2 Antagonist MK-7123. A phase 2 proof-of-concept trial for chronic obstructive pulmonary disease. Am J Respir Crit Care Med. 2015;191(9):1001–1011. DOI:10.1164/rccm.201405-0992OC
- Aaron SD, Vandemheen KL, Maltais F, et al. TNFα antagonists for acute exacerbations of COPD: a randomised double-blind controlled trial. Thorax. 2013;68(2):142–148. DOI:10.1136/thoraxjnl-2012-202432
- Agusti A, Fabbri LM, Singh D, et al. Inhaled corticosteroids in COPD: friend or foe?Eur Respir J. 2018;52(6):1801219. DOI:10.1183/13993003.01219-2018
- Yun JH, Lamb A, Chase R, et al. Blood eosinophil count thresholds and exacerbations in patients with chronic obstructive pulmonary disease. J Allergy Clin Immunol. 2018;141(6):2037–2047. DOI:10.1016/j.jaci.2018.04.010
- Zou Y, Chen X, Liu J, et al. Serum IL-1beta and IL-17 levels in patients with COPD: associations with clinical parameters. COPD. 2017;12:1247–1254. DOI:10.2147/COPD.S131877
- Colarusso C, Terlizzi M, Molino A, et al. Role of the inflammasome in chronic obstructive pulmonary disease (COPD). Oncotarget. 2017;8(47):81813–81824. DOI:10.18632/oncotarget.17850
- Osei ET, Noordhoek JA, Hackett TL, et al. Interleukin-1α drives the dysfunctional cross-talk of the airway epithelium and lung fibroblasts in COPD. Eur Respir J. 2016;48(2):359–369. DOI:10.1183/13993003.01911-2015
- Colak Y, Nordestgaard BG, Vestbo J, et al. Comparison of five major airflow limitation criteria to identify high-risk individuals with COPD: a contemporary population-based cohort. Thorax. 2020;75(11):944–954. DOI:10.1136/thoraxjnl-2020-214559
- Hobbs BD, de Jong K, Lamontagne M, et al. Genetic loci associated with chronic obstructive pulmonary disease overlap with loci for lung function and pulmonary fibrosis. Nat Genet. 2017;49(3):426–432. DOI:10.1038/ng.3752
- Global Initiative for Chronic Obstructive Lung Disease. Global strategy for the diagnosis, management, and prevention of chronic obstructive pulmonary disease; 2020. [cited 2021 Jun 16]. Available from: https://goldcopd.org/2020
- Sun BB, Maranville JC, Peters JE, et al. Genomic atlas of the human plasma proteome. Nature. 2018;558(7708):73–79. DOI:10.1038/s41586-018-0175-2
- Ahola-Olli AV, Wurtz P, Havulinna AS, et al. Genome-wide association study identifies 27 loci influencing concentrations of circulating cytokines and growth factors. Am J Hum Genet. 2017;100(1):40–50. DOI:10.1016/j.ajhg.2016.11.007
- Battle A, Khan Z, Wang SH, et al. Genomic variation. Impact of regulatory variation from RNA to protein. Science. 2015;347(6222):664–667. DOI:10.1126/science.1260793
- Gold L, Ayers D, Bertino J, et al. Aptamer-based multiplexed proteomic technology for biomarker discovery. PLoS One. 2010;5(12):e15004. DOI:10.1371/journal.pone.0015004
- Cardon LR, Palmer LJ. Population stratification and spurious allelic association. Lancet. 2003;361(9357):598–604. DOI:10.1016/S0140-6736(03)12520-2
- Manolio TA. Genomewide association studies and assessment of the risk of disease. N Engl J Med. 2010;363(2):166–176. DOI:10.1056/NEJMra0905980
- Boyle AP, Hong EL, Hariharan M, et al. Annotation of functional variation in personal genomes using RegulomeDB. Genome Res. 2012;22(9):1790–1797. DOI:10.1101/gr.137323.112
- Bowden J, Greco M F. D., Minelli C, et al. Assessing the suitability of summary data for two-sample Mendelian randomization analyses using MR-Egger regression: the role of the I2 statistic. Int J Epidemiol. 2016;45(6):1961–1974. DOI:10.1093/ije/dyw220
- Bowden J, Davey Smith G, Haycock PC, et al. Consistent estimation in Mendelian randomization with some invalid instruments using a weighted median Estimator. Genet Epidemiol. 2016;40(4):304–314. DOI:10.1002/gepi.21965
- Bowden J, Davey Smith G, Burgess S. Mendelian randomization with invalid instruments: effect estimation and bias detection through Egger regression. Int J Epidemiol. 2015;44(2):512–525. DOI:10.1093/ije/dyv080
- Zhao QY, Wang JS, Hemani G, et al. Statistical inference in two-sample summary-data Mendelian randomization using robust adjusted profile score. Ann Stat. 2020;48(3):1742–1769.
- Timpson NJ, Nordestgaard BG, Harbord RM, et al. C-reactive protein levels and body mass index: elucidating direction of causation through reciprocal Mendelian randomization. Int J Obes (Lond). 2011;35(2):300–308. DOI:10.1038/ijo.2010.137
- Baum CF, Schaffer ME, Stillman S. Instrumental variables and GMM: estimation and testing. Stata J. 2003;3(1):1–31. DOI:10.1177/1536867X0300300101
- Sanderson E, Davey Smith G, Windmeijer F, et al. An examination of multivariable Mendelian randomization in the single-sample and two-sample summary data settings. Int J Epidemiol. 2019;48(3):713–727. DOI:10.1093/ije/dyy262
- Sudlow C, Gallacher J, Allen N, et al. UK biobank: an open access resource for identifying the causes of a wide range of complex diseases of middle and old age. PLoS Med. 2015;12(3):e1001779. DOI:10.1371/journal.pmed.1001779
- FinnGen. Documentation of R3 release; 2020.
- Davies NM, Holmes MV, Davey Smith G. Reading Mendelian randomisation studies: a guide, glossary, and checklist for clinicians. BMJ. 2018;362:k601. DOI:10.1136/bmj.k601
- Smith GD, Davies Nm, Dimou N, et al. STROBE-MR: guidelines for strengthening the reporting of Mendelian randomization studies. PeerJ Preprints. 2019;7:e27857v1. DOI:10.7287/peerj.preprints.27857v1.
- Hemani G, Zheng J, Elsworth B, et al. The MR-Base platform supports systematic causal inference across the human phenome. Elife. 2018;7:e34408. DOI:10.7554/eLife.34408
- Sanderson E, Richardson TG, Hemani G, et al. The use of negative control outcomes in Mendelian randomization to detect potential population stratification. Int J Epidemiol. 2021 Feb 11:dyaa288. DOI:10.1093/ije/dyaa288. Online ahead of print.
- Arnold BF, Ercumen A. Negative control outcomes: a tool to detect bias in randomized trials. JAMA. 2016;316(24):2597–2598. DOI:10.1001/jama.2016.17700
- Lipsitch M, Tchetgen Tchetgen E, Cohen T. Negative controls: a tool for detecting confounding and bias in observational studies. Epidemiology. 2010;21(3):383–388. DOI:10.1097/EDE.0b013e3181d61eeb
- Brion MJ, Shakhbazov K, Visscher PM. Calculating statistical power in Mendelian randomization studies. Int J Epidemiol. 2013;42(5):1497–1501. DOI:10.1093/ije/dyt179
- Freeman G, Cowling BJ, Schooling CM. Power and sample size calculations for Mendelian randomization studies using one genetic instrument. Int J Epidemiol. 2013;42(4):1157–1163. DOI:10.1093/ije/dyt110
- TobaccoGenetics C. Genome-wide meta-analyses identify multiple loci associated with smoking behavior. Nat Genet. 2010;42(5):441–447.
- Astle WJ, Elding H, Jiang T, et al. The allelic landscape of human blood cell trait variation and links to common complex disease. Cell. 2016;167(5):1415–1429.e19. DOI:10.1016/j.cell.2016.10.042
- Burgess S, Thompson SG. Multivariable Mendelian randomization: the use of pleiotropic genetic variants to estimate causal effects. Am J Epidemiol. 2015;181(4):251–260. DOI:10.1093/aje/kwu283
- Burgess S, Dudbridge F, Thompson SG. Re: "Multivariable Mendelian randomization: the use of pleiotropic genetic variants to estimate causal effects". Am J Epidemiol. 2015;181(4):290–291. DOI:10.1093/aje/kwv017
- Dima E, Koltsida O, Katsaounou P, et al. Implication of Interleukin (IL)-18 in the pathogenesis of chronic obstructive pulmonary disease (COPD). Cytokine. 2015;74(2):313–317. DOI:10.1016/j.cyto.2015.04.008
- Mokry LE, Zhou S, Guo C, et al. Interleukin-18 as a drug repositioning opportunity for inflammatory bowel disease: a Mendelian randomization study. Sci Rep. 2019;9(1):9386. DOI:10.1038/s41598-019-45747-2
- McGowan LM, Davey Smith G, Gaunt TR, et al. Integrating Mendelian randomization and multiple-trait colocalization to uncover cell-specific inflammatory drivers of autoimmune and atopic disease. Hum Mol Genet. 2019;28(19):3293–3300. DOI:10.1093/hmg/ddz155
- Yang Z, Schooling CM, Kwok MK. 2021. Genetic evidence for causal associations between interleukin (IL)-1 family members/receptors and lung cancer. Submitted.
- Zheng J, Baird D, Borges MC, et al. Recent developments in Mendelian randomization studies. Curr Epidemiol Rep. 2017;4(4):330–345. DOI:10.1007/s40471-017-0128-6
- Hartwig FP, Tilling K, Smith GD, et al. Bias in two-sample Mendelian randomization when using heritable covariable-adjusted summary associations. Int J Epidemiol. 2021 Feb 23;dyaa266. DOI:10.1093/ije/dyaa266. Online ahead of print.
- Moore C, Sambrook J, Walker M, et al. The INTERVAL trial to determine whether intervals between blood donations can be safely and acceptably decreased to optimise blood supply: study protocol for a randomised controlled trial. Trials. 2014;15:363. DOI:10.1186/1745-6215-15-363
- Lopez PM, Subramanian SV, Schooling CM. Effect measure modification conceptualized using selection diagrams as mediation by mechanisms of varying population-level relevance. J Clin Epidemiol. 2019;113:123–128. DOI:10.1016/j.jclinepi.2019.05.005
- Miller MR, Quanjer PH, Swanney MP, et al. Interpreting lung function data using 80% predicted and fixed thresholds misclassifies more than 20% of patients. Chest. 2011;139(1):52–59. DOI:10.1378/chest.10-0189
- Quanjer PH, Stanojevic S, Cole TJ, et al. Multi-ethnic reference values for spirometry for the 3-95-yr age range: the global lung function 2012 equations. Eur Respir J. 2012;40(6):1324–1343. DOI:10.1183/09031936.00080312
- Pierce BL, VanderWeele TJ. The effect of non-differential measurement error on bias, precision and power in Mendelian randomization studies. Int J Epidemiol. 2012;41(5):1383–1393. DOI:10.1093/ije/dys141
- Borthwick LA. The IL-1 cytokine family and its role in inflammation and fibrosis in the lung. Semin Immunopathol. 2016;38(4):517–534. DOI:10.1007/s00281-016-0559-z
- Imaoka H, Hoshino T, Takei S, et al. Interleukin-18 production and pulmonary function in COPD. Eur Respir J. 2008;31(2):287–297. DOI:10.1183/09031936.00019207
- George L, Brightling CE. Eosinophilic airway inflammation: role in asthma and chronic obstructive pulmonary disease. Ther Adv Chronic Dis. 2016;7(1):34–51. DOI:10.1177/2040622315609251
- Santarlasci V, Cosmi L, Maggi L, et al. IL-1 and T helper immune responses. Front Immunol. 2013;4:182.
- Rovina N, Koutsoukou A, Koulouris NG. Inflammation and immune response in COPD: where do we stand?Mediators Inflamm. 2013;2013:413735. DOI:10.1155/2013/413735
- Baines KJ, Fu JJ, McDonald VM, et al. Airway gene expression of IL-1 pathway mediators predicts exacerbation risk in obstructive airway disease. COPD. 2017;12:541–550. DOI:10.2147/COPD.S119443
- Camiciottoli G, Bigazzi F, Paoletti M, et al. Pulmonary function and sputum characteristics predict computed tomography phenotype and severity of COPD. Eur Respir J. 2013;42(3):626–635. DOI:10.1183/09031936.00133112
- Mirza S, Benzo R. Chronic obstructive pulmonary disease phenotypes: implications for care. Mayo Clin Proc. 2017;92(7):1104–1112. DOI:10.1016/j.mayocp.2017.03.020
- Kakavas S, Kotsiou OS, Perlikos F, et al. Pulmonary function testing in COPD: looking beyond the curtain of FEV1. NPJ Prim Care Respir Med. 2021;31(1):23. DOI:10.1038/s41533-021-00236-w
- Schooling CM, Zhao JV, Au Yeung SL, et al. Investigating pleiotropic effects of statins on ischemic heart disease in the UK Biobank using Mendelian randomisation. Elife. 2020;9:e58567. DOI:10.7554/eLife.58567
- Ebrahimi F, Urwyler SA, Straumann S, et al. IL-1 antagonism in men with metabolic syndrome and low testosterone: a randomized clinical trial. J Clin Endocrinol Metab. 2018;103(9):3466–3476. DOI:10.1210/jc.2018-00739
- Ridker PM. From C-reactive protein to interleukin-6 to interleukin-1: moving upstream to identify novel targets for atheroprotection. Circ Res. 2016;118(1):145–156. DOI:10.1161/CIRCRESAHA.115.306656
- Interleukin 1 Genetics Consortium. Cardiometabolic effects of genetic upregulation of the interleukin 1 receptor antagonist: a Mendelian randomisation analysis. Lancet Diabetes Endocrinol. 2015;3(4):243–253.