Abstract
Chronic obstructive pulmonary disease (COPD) is a heterogeneous disease with variable mortality risk. The aim of our investigation was to validate a simple clinical algorithm for long-term mortality previously proposed by Burgel et al. in 2017. Subjects with COPD from two cohorts, the Swedish PRAXIS study (n = 784, mean age (standard deviation (SD)) 64.0 years (7.5), 42% males) and the Rotterdam Study (n = 735, mean age (SD) 72 years (9.2), 57% males), were included. Five clinical clusters were derived from baseline data on age, body mass index, dyspnoea grade, pulmonary function and comorbidity (cardiovascular disease/diabetes). Cox models were used to study associations with 9-year mortality. The distribution of clinical clusters (1–5) was 29%/45%/8%/6%/12% in the PRAXIS study and 23%/26%/36%/0%/15% in the Rotterdam Study. The cumulative proportion of deaths at the 9-year follow-up was highest in clusters 1 (65%) and 4 (72%), and lowest in cluster 5 (10%) in the PRAXIS study. In the Rotterdam Study, cluster 1 (44%) had the highest cumulative mortality and cluster 5 (5%) the lowest. Compared with cluster 5, the meta-analysed age- and sex-adjusted hazard ratio (95% confidence interval) for cluster 1 was 6.37 (3.94–10.32) and those for clusters 2 and 3 were 2.61 (1.58–4.32) and 3.06 (1.82–5.13), respectively. Burgel’s clinical clusters can be used to predict long-term mortality risk. Clusters 1 and 4 are associated with the poorest prognosis, cluster 5 with the best prognosis and clusters 2 and 3 with intermediate prognosis in two independent cohorts from Sweden and the Netherlands.
Supplemental data for this article is available online at https://doi.org/10.1080/15412555.2022.2039608 .
Introduction
Chronic obstructive pulmonary disease (COPD) is one of the leading causes of death and associated with high healthcare and societal costs worldwide [Citation1]. The estimated prevalence of COPD in different populations varies widely depending on the criteria used. For example, a prevalence of around 9–10% could be found based on objective measurements, while the prevalence of doctor’s diagnosed COPD was around 5%, indicating the disease is still under-recognised [Citation2]. According to the Global Initiative for Obstructive Lung Disease (GOLD), a post-bronchodilator fixed ratio of forced expiratory volume in 1 s (FEV1)/forced vital capacity (FVC) lower than 0.70 is required to establish the diagnosis in subjects with respiratory symptoms and relevant exposure, and therefore clinical suspicion of COPD [Citation3].
The clinical grading used for prognosis of COPD encompasses airflow obstruction severity (FEV1), degree of respiratory symptoms and history of exacerbations [Citation4], including hospitalisations. However, other factors than these – for example comorbidities such as heart failure (HF), coronary cardiovascular disease (CVD), diabetes, depression and lung cancer, as well as lung function decline[Citation5] – have a major impact on prognosis.
COPD is a highly heterogeneous disease with large differences in exacerbation rate or mortality between patients. It is therefore important to find new predictive factors that would guide classification of patients to different risk groups. Many studies have tried to explore the disease heterogeneity; one of the first attempts involved dividing subjects into two groups – ‘pink puffers’ and ‘blue bloaters’ – based on physical findings [Citation6]. Several previous studies have applied cluster analysis [Citation7] to derive distinct patient subgroups and find their associations with clinically relevant outcomes such as cohort mortality [Citation8–10] or predicted mortality scores[Citation11, Citation12], hospital admissions [Citation10] and frequency of exacerbations [Citation13, Citation14]. Nevertheless, the majority of studies have lacked external validation and the results of cluster analysis have given no tool for individual patient allocation to the described phenotypes. A systematic review could identify two consistent major clinical phenotypes with poor prognosis across multiple studies: younger patients with severe COPD and low probability of cardiovascular comorbidities and older individuals with moderate respiratory disease and high prevalence of comorbidities, obesity and inflammatory markers [Citation15].
Burgel et al. [Citation16] recently investigated COPD cohorts using cluster analysis and identified five subgroups with different rates of 3-year all-cause mortality. Further classification and regression trees (CART) [Citation17] were used to develop an algorithm to allocate patients to those subgroups. The data needed for these clinical clusters can be collected at regular patient follow-ups and integrate respiratory variables and comorbidities (cardiovascular diseases, diabetes and obesity). Although the authors validated their findings in an independent COPD cohort with regard to 3-year mortality, no validation has been performed beyond the 3-year timeframe investigated in the original publication.
Our aim was therefore to evaluate the prognostic value of this clinical clustering algorithm over an extended follow-up time, 9–12 years, in two prospective cohorts in Sweden and the Netherlands.
Methods
Study design
Patient data from Swedish and Dutch COPD cohorts, presented in the next section, were used to allocate subjects into specific phenotype groups in accordance with the algorithm published by Burgel et al. [Citation16], based on age, body mass index (BMI), lung function, comorbidities and dyspnoea ().
COPD cohorts and COPD diagnosis
The Rotterdam Study is a prospective population-based cohort study ongoing since 1990 in Ommoord, a suburb of the city of Rotterdam, Netherlands. The objective of the study is to clarify the risk factors (genetic, environmental and lifestyle) of diseases in an ageing population. Diseases targeted are cardiovascular, respiratory, endocrine, hepatic, neurological, ophthalmic, psychiatric, dermatological, otolaryngological and locomotor [Citation18]. The study consists of four cohorts with a total of more than 15,000 subjects aged ≥ 45 years. Every 3–4 years, participants undergo a home interview and clinical examinations at the research centre. For the purpose of this study, we analysed participants from the fifth round of the Rotterdam Study, visiting the research centre during 2009–2014. A total of 5,487 participants had performed spirometry, of whom 5459 also consented to a follow-up. FEV1/FVC ratio < 0.7, for example, airflow obstruction, was found in 912 subjects. COPD was defined as presence of airflow obstruction in absence of a clinical asthma diagnosis, leaving 806 individuals in the present study. Among them, 71 individuals could not been classified in accordance with the Burgel algorithm, due to missing data from the modified Medical Research Council (mMRC) dyspnoea scale score (Online ).
The PRAXIS study is a prospective COPD cohort created in 2005. It includes primary and secondary care patients, randomly selected, from 56 primary health care centres and 14 hospitals in central Sweden, that answered a detailed questionnaire [Citation19]. COPD diagnosis was based on medical records, for example, physician-diagnosed COPD in the medical records. A total of 1,088 individuals fulfilled the inclusion criteria for our study. However, 304 of them could not been classified in the Burgel clusters due to missing data, leaving 784 individuals in the present analyses (Online ).
Short-term oral corticosteroid use
Information on short-term oral corticosteroid use in the 6 months prior to entering the study was available for both cohort and used as a marker for recent exacerbations. In the PRAXIS study, this data was taken from questionnaires. In the Rotterdam Study, complete information on all filled prescriptions for steroids and/or antibiotics on a day-to-day basis was obtained from pharmacies and validated.
Exacerbations
Information about exacerbations during the 6 months prior to inclusion in the study was retrieved from the questionnaire in the PRAXIS study. Exacerbations were defined as emergency visits to primary care or secondary care (outpatient or hospitalisation) due to deterioration of their lung disease, or need for an oral steroid course, in the 6 months preceding inclusion.
Dyspnoea
Dyspnoea grade was measured on the mMRC dyspnoea scale and obtained through questionnaires. This scale has been validated before and is often used in research and clinical settings [Citation20].
Comorbidities
Information about cardiovascular comorbidities (hypertension, ischaemic heart disease and chronic heart failure), asthma and diabetes was taken from patients’ records in the PRAXIS study [Citation21]. In the Rotterdam Study, relevant data for each patient were obtained at baseline and at follow-up, via a combination of objective measures, reviews of medical record reviews from primary and secondary care and prescription registries. For example, hypertension in the Rotterdam Study was defined as a systolic blood pressure (SBP) > 140 mmHg, diastolic blood pressure (DBP) > 90 mmHg, or use of blood pressure-lowering medication for the indication hypertension. SBP and DBP were measured in the right upper arm with the subjects in a seated position. The average of two consecutive measurements was taken. Definitions of cardiovascular comorbidities in the Rotterdam Study are described in detail elsewhere [Citation22].
Smoking habits
Current smoking status was also questionnaire-assessed and the patients were divided into current and non-smokers for the purpose of this study.
Lung function tests
FEV1 was expressed as % predicted pre-bronchodilator spirometry in the Rotterdam Study, using Global Lung Initiative reference equations [Citation23]. Lung function tests were performed by trained paramedical personnel in accordance with the American Thoracic Society/European Respiratory Society Task Force 2005 document regarding standardisation of spirometry [Citation24]. A portable spirometer (SpiroPro; Erich Jaeger GmbH; Hoechberg, Germany) was used from 2002 to 2008 and a Master Screen® PFT Pro (Care Fusion, the Netherlands) was used from 2009 onward [Citation25, Citation26].
FEV1 was recorded in the PRAXIS study as the highest % predicted of any pre- or post-bronchodilator values from spirometry reports or medical records, using European Community for Steel and Coal reference values [Citation27], from the review of medical records between 2000 and 2004. These values has been registered only as percent predicted and no exact date of the spirometry was recorded in the database. As the data were collected from clinical practice, several different spirometry devices, such as pneumotachographs, ultrasound sensors or turbine transducers, from several manufacturers were used [Citation28].
Statistical analysis
Descriptive statistics were used for analysing the prevalence of each cluster and characteristics of individuals within each cluster. Survival analyses for all-cause mortality were performed at 3 and 9 years for both cohorts and also at 12 years for the PRAXIS study. We used both Kaplan-Meier curves and Cox models. Meta-analyses of the Cox models for 3- and 9-year survival were also performed. Adjustments were made for age, sex or both age, sex and short-term oral corticosteroid use. STATA software version 16 (StataCorp LLC, College Station, TX, USA) and R version 4.3.0.2 were used for the analyses.
Ethics
In both studies, all patients signed informed consent prior to participation. The Rotterdam Study was approved by the Ethical Committee of the Erasmus Medical Centre (Rotterdam, the Netherlands) and the review board of the Netherlands Ministry of Health, Welfare and Sports (1068889-159521-PG). The PRAXIS study was approved by the Regional Ethical Review Board in Uppsala, Sweden (Dnr 2004: M-445 and Dnr 2010/090).
Results
The characteristics of subjects with COPD in both cohorts at inclusion are presented in . In the Rotterdam study, subjects were slightly older, had better lung function (FEV1 (% predicted)), less dyspnoea (mMRC), and less reported use of short-term oral corticosteroids during the preceding 6 months, but more cardiovascular comorbidities, especially hypertension ().
Table 1. Baseline characteristics of the clinical COPD phenotype groups (mean (SD) or % unless otherwise stated).
The algorithm for allocation of patients into five clinical clusters (1–5) in the PRAXIS and Rotterdam studies is presented in and the characteristics of the subjects included in the respective clusters are presented in . In the PRAXIS study, cluster 5 was characterised by the lowest mortality rate at all timepoints, while the highest 9-year mortality rates of 65% and 72% were observed in clusters 1 and 4, respectively. In the Rotterdam study, the patients could be classified into only four clinical clusters (1, 2, 3 and 5), as no subjects fulfilled the criteria for cluster 4 (no cardiovascular comorbidities, severe dyspnoea and FEV1 ≤ 35% predicted) (). The lowest mortality rate was found in cluster 5. Cluster 1 had the highest mortality rate at both 3 and 9 years; cluster 3 had intermediate mortality rates (). Cluster 2 had mortality rates in between cluster 5 and cluster 3 (). The findings reported above can be seen in the Kaplan-Meier plots, performed for each study separately with 9-year follow-up data in the Rotterdam Study and 12-year follow-up data in the PRAXIS study ().
Figure 2. (A) Kaplan-Meier analysis for assessing all-cause mortality over the study period in the PRAXIS cohort. (B) Kaplan-Meier analysis for assessing all-cause mortality over the study period in the Rotterdam Study cohort.
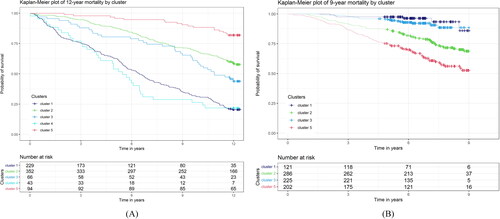
Table 2A. Baseline characteristics of the clinical COPD phenotype groups in the PRAXIS cohort (mean (SD) or % unless otherwise stated).
Table 2B. Baseline characteristics of the clinical COPD phenotype groups in the Rotterdam Study cohort (mean (SD) or % unless otherwise stated).
Hazard ratios (HR) for mortality at 3, 9 and 12 years for the different clinical clusters were calculated using Cox regression, separately for each cohort (). In both cohorts, the worst prognosis during overall follow-up, based on the HR for mortality, was observed in clusters 1 and 4, and the best prognosis in cluster 5. Clusters 2 and 3 showed intermediate rates ().
Table 3. Relative mortality risk, assessed using a Cox regression model in the PRAXIS and Rotterdam Study cohorts (hazard ratio (95% CI)).
Age- and sex-adjusted HR were also calculated () and the results were consistent for most of the comparisons, with the exception of non-significant association of cluster 3 with 3-year survival in both studies. For the PRAXIS study, we performed an additional adjustment for asthma and the associations presented in remained significant (Online ). Lastly, further adjustment for short-term oral corticosteroid use during the six months prior to the study did not change the results presented in , with the exception of non-significant association of cluster 1 with 3-year survival and of cluster 3 with 9-year survival in the Rotterdam Study (). In the PRAXIS study, we also applied a model where adjustments for age and previous exacerbations were used, instead of adjustments for oral corticosteroids, with similar results (data not shown).
Table 4. Relative mortality risk in clinical COPD clusters, assessed using a Cox regression model, adjusted for age and sex in the PRAXIS and Rotterdam cohorts (hazard ratio (95% CI)).
Table 5. Relative mortality risk in clinical COPD clusters, assessed using a Cox regression model, adjusted for age, sex and for short-term oral corticosteroid use during the last six months in the PRAXIS and Rotterdam Study cohorts (hazard ratio (95% CI)).
Meta-analyses of the age- and sex-adjusted Cox regression models for the two studies are presented in and Online . Both clusters 1 and 3 had higher mortality risk than cluster 5 at 3 years, but cluster 2 did not (). Clusters 1, 2 and 3 had higher mortality risk at 9 years compared with cluster 5. Moreover, cluster 1 had worse prognosis than both clusters 2 and 3, with regard to both 3- and 9-year mortality (Online ). Lastly, cluster 3 had worse prognosis than cluster 2, with regard to both 3- and 9-year mortality (Online ).
Table 6. Meta-analysis for relative mortality risk at 3 and 9 years, adjusted for age and sex. Cox regression model (HR, 95% CI).
A sensitivity analysis of using LLN instead of 0.70 as cut-off for abnormal FEV1/FVC-ratio, and COPD diagnosis, was performed in the Rotterdam study. By using LLN instead of 0.70, only 286 subjects had confirmed COPD diagnosis and these were distributed as following: 90 in cluster 1, 96 in cluster 2, 66 in cluster 3 and 34 in cluster 5. Mortality rates at 3 and 9 years are presented in and these were similar to the ones presented in of clusters 1, 2 and 3. No HR could be calculated in comparison with cluster 5 as all subjects in cluster 5 were alive at 9-years’ follow-up.
Discussion
We applied and validated the previous clinical clusters proposed by Burgel et al., based on comorbidities, lung function, age, BMI and dyspnoea, and could confirm the predictive value of these clusters for long-term mortality, up to 9 and 12 years, in two different European COPD cohorts, respectively. We also found these results to be consistent after adjustments for age, sex, previous exacerbation rates and concurrent asthma. The worst prognosis, in both cohorts, was found for cluster 1, which comprised individuals with cardiovascular comorbidities and/or diabetes and high grade of dyspnoea and elderly individuals (> 70 years of age) with FEV1 < 50% predicted. Cluster 4, comprising highly dyspnoeic individuals with FEV1 ≤ 35% predicted, but without cardiovascular comorbidities or diabetes, was only present in the Swedish patient cohort, and also had a poor prognosis. On the other hand, cluster 5, including individuals without comorbidities, with a low grade of dyspnoea and preserved lung function (FEV1 ≥ 60% predicted) was associated with the best prognosis in both cohorts.
Different score systems have been proposed to determine which COPD patients are at higher risk of mortality or worsening of disease. The BODE index, which encompasses four variables (BMI, grade of airflow obstruction, dyspnoea grade and exercise capacity) is a well-known score (on a scale of 0–10 points) to predict mortality [Citation29]. The two extreme quartiles of the BODE index, quartile I (0–2 points) and quartile IV (7–10 points), may correlate with our study’s clusters 5 and 1, respectively. The quartiles were related to mortality of around 20% and 80%, respectively, at a 52-month follow-up [Citation29]. Another known index, consisting of dyspnoea, obstruction, smoking and exacerbation (DOSE), showed increased risk of death: HR 8.00 for score 6–7 compared with HR 3.48 for score 0–3 in COPD patients from primary and secondary care within a period of five years [Citation30]. Moreover, a meta-analysis on nearly 15,000 patients from 24 different cohorts studies concluded that the ADO (age, dyspnoea and airflow obstruction) index best predicted 3-year mortality in COPD patients, followed by the updated BODE index[Citation31, Citation32]. Other scores are B-AE-D (BMI, acute exacerbations, dyspnoea) [Citation33] and e-BODE (addition of exacerbations to BODE) [Citation33]. A more recent study suggested that BODE could be combined with information on comorbidities in order to increase its predictive value for mortality, creating the so-called COPD comorbidity test (COTE) index [Citation34] – an approach close to the Burgel’s algorithm [Citation16]. Worse survival was noted in the presence of comorbidities for each BODE quartile when studying mortality for up to 54 months. Recently, we found indications that cardiovascular comorbidity, especially heart failure, myocardial infarction and stroke, predicted mortality in a Swedish real-world primary care study of COPD patients[Citation35]. In line with this, a newer index has been proposed to be applied in the primary care setting and validated for short-term mortality – BARC (body mass index and blood results (B), age (A), respiratory variables, such as obstruction, exacerbations and smoking (R) and comorbidity (C)) [Citation36]. A recent study suggested benefits from using comorbidities to improve the performance of ADO and DOSE in estimating mortality for up to 3 years in a primary care setting [Citation37]. A recent meta-analysis on different prognostic instruments concluded that future research should focus on external validation of these prognostic instruments along with cost-effectiveness analyses [Citation38].
Cluster 1 was consistently associated with poorer prognosis in both cohorts up to 9 or 12 years of follow-up. The cardiovascular and diabetes comorbidities have been shown to relate to poorer prognosis in several studies [Citation39, Citation40]. Low values of FEV1, as well as higher grades of dyspnoea, are also associated with higher mortality and are already included in several prognostic score systems, that is, BODE or DOSE [Citation29, Citation30, Citation41]..
Cluster 4 was present only in the Swedish cohort and was also associated with poor prognosis. The absence of cluster 4 in the Rotterdam study might be explained by the elderly population and a higher prevalence of hypertension (as blood pressure was measured during the clinical visit), which contributed to the majority of the participants with COPD in the Rotterdam Study having cardiovascular comorbidities as well. Moreover, the fact that these individuals were randomly selected from the population might have resulted in a very small proportion of subjects with severely impaired lung function (FEV1 ≤ 35% predicted).
Cluster 5, characterised by the absence of the comorbidities, low grade of dyspnoea (mMRC 0–1) and FEV1 ≥ 60%, had the best prognosis, with 90% survival at 9 year follow-up. In past studies, little attention has been paid to the clusters with good prognosis, although we are aware that some COPD patients have a very stable disease and do not have exacerbations [Citation42].
A major strength of the present study was that we were able to validate the Burgel clinical clusters in two different European cohorts: a Swedish patient cohort and a Dutch population-based cohort. There is a rationale for validating the instrument in several cohorts, as previous studies regarding the BODE index could demonstrate differing performance in different populations [Citation43]. Another strength is the fact that we could validate the clusters for both short-term mortality (3 years) and long-term mortality (9–12 years). The mortality rates at 3-year follow-up were comparable between our two cohorts and with the replication cohort from Burgel’s paper, where the majority of patients were recruited from population-based studies. Our study also had some limitations. For example, lung function in the PRAXIS study was not registered at study inclusion; the last recorded spirometry was retrieved from patient records. Moreover, different reference values for spirometry were used in our studies, depending on the respective national clinical practices. The COPD definition in the Rotterdam Study was mainly based on the pulmonary function testing results for a routine visit to research centre and did not require relevant exposure or respiratory symptoms, differing from the PRAXIS study, where only doctor-diagnosed patients with COPD were included. A limitation of the PRAXIS study is the fact that a large proportion of subjects were lacking the results of a postbronchodilatory spirometry in the medical records reviewed between 2000 and 2004. This might be due to the fact that they had a diagnosis confirmed by spirometry before study entry or that patients with most severe disease have been unable or unwilling to perform a lung function test. Sundh et al. demonstrated in the present population that COPD subjects without lung function were actually characterised by a higher mortality than subjects with available lung function [Citation30]. We interpret the poorer prognosis in these subjects without spirometry as an indirect confirmation that the subjects had COPD. Moreover as the results were consistent across the two studies, this suggests that the clusters are valid in different populations. Lastly, the present clusters do not include exacerbations in their definitions, although exacerbations are known to be strong predictors for future exacerbations and mortality [Citation4]. However, we found that the prognostic value for clinical clusters was independent of previous use of short-term oral corticosteroid treatment, used as a proxy for previous exacerbations.
In conclusion, we could validate previously proposed clinical phenotypes with regard to long-term mortality in two different European cohorts. We could validate clusters associated with both good and bad prognosis. These clinical clusters are based on data that is easy available at the patient visit as it does not requiring extensive characterisation of patients or specific methods or add-ons to the clinical visit. We propose that these clusters should be used to a larger extent in both primary and specialist care for better planning of follow-up and healthcare utilisation. This needs to be investigated in further studies, including health economic evaluation.
Declaration of interest
SG reports personal fees from Roche, outside the submitted work. SW reports grants from GlaxoSmithKline, outside the submitted work. BS reports personal fees from AstraZeneca, personal fees from Novartis, personal fees from Boehringer Ingelheim, personal fees from GlaxoSmithKleine, personal fees from Meda, personal fees from TEVA, personal fees from Chiesi, all outside the submitted work. KL reports personal fees from Novartis, personal fees from AstraZeneca, personal fees from BoehringerIngelheim, personal fees from GlaxoSmithKline, personal fees from Chiesi, all outside the submitted work. GB reports personal fees from Astra Zeneca, personal fees from Amgen, personal fees from Boehringer-Ingelheim, personal fees from Chiesi, personal fees from GlaxoSmithKline, personal fees from Novartis, personal fees from Sanofi, personal fees from Teva, all outside the submitted work. All other authors have no conflicts of interest to disclose with regard to the present work.
Funding
The PRAXIS study is supported by grants from the county councils of the Uppsala–Örebro Health Care region, the Swedish Heart and Lung Association, the Swedish Asthma and Allergy Association, the Bror Hjerpstedts Foundation, the Centre for Clinical Research, Dalarna, the Uppsala–Örebro Regional Research Council, and by Region Örebro County through ALF research funding.
The funding sources had no involvement in the collection, analysis, writing, interpretation or the decision to submit the paper for publication.
The Rotterdam Study is supported by the Erasmus MC and Erasmus University Rotterdam; the Netherlands Organisation for Scientific Research (NWO) (grant no. 918-46-615); the Netherlands Organisation for Health Research and Development (ZonMW); the Research Institute for Diseases in the Elderly (RIDE); the Netherlands Genomics Initiative; the Ministry of Education, Culture and Science; the Ministry of Health, Welfare and Sports; the European Commission (DG XII; grant no. 601055); and the Municipality of Rotterdam.
References
- Soriano JB, Abajobir AA, Abate KH, et al. Global, regional, and national deaths, prevalence, disability-adjusted life years, and years lived with disability for chronic obstructive pulmonary disease and asthma, 1990–2015: a systematic analysis for the global burden of disease study 2015. Lancet Resp Med. 2017;5(9):691–706. DOI:10.1016/S2213-2600(17)30293-X
- Halbert RJ, Natoli JL, Gano A, et al. Global burden of COPD: systematic review and Meta-analysis. Eur Respir J. 2006; Sep28(3):523–532. DOI:10.1183/09031936.06.00124605
- Vogelmeier CF, Criner GJ, Martinez FJ, et al. Global Strategy for the diagnosis, management, and prevention of chronic obstructive lung 406 disease 2017 report: GOLD executive summary. Eur Respir J. (3)2017; 49:1700214.
- R-Cataluna JJ, Martinez-Garcia MA, Roman Sanchez P, et al. Severe acute exacerbations and mortality in patients with chronic obstructive pulmonary disease. Thorax. 2005; Nov60(11):925–931. DOI:10.1136/thx.2005.040527
- Odeyemi YE, Lewis O, Ngwa J, et al. Does low fev1 in addition to fixed ratio and/or lower limit of normal of FEV1/FVC improve prediction of mortality in COPD? The NHANES-III-linked-mortality cohort. J Natl Med Assoc. 2019;111(1):94–100. DOI:10.1016/j.jnma.2018.06.006
- Dornhorst AC. Respiratory insufficiency. Lancet. 1955;268(6876):1185–1187.
- Wardlaw AJ, Silverman M, Siva R, et al. Multi-dimensional phenotyping: towards a new taxonomy for airway disease. Clin Exp Allergy. 2005;35(10):1254–1262. DOI:10.1111/j.1365-2222.2005.02344.x
- Burgel PR, Roche N, Paillasseur JL, et al. Clinical COPD phenotypes identified by cluster analysis: validation with mortality. Eur Respir J. INITIATIVES BPCO Scientific Committee 2012; Aug40(2):495–496. DOI:10.1183/09031936.00228511
- Burgel PR, Paillasseur JL, Peene B, et al. Two distinct chronic obstructive pulmonary disease (COPD) phenotypes are associated with high risk of mortality. PLoS One. 2012;7(12):e51048. DOI:10.1371/journal.pone.0051048
- Garcia-Aymerich J, Gomez FP, Benet M, et al. Identification and prospective validation of clinically relevant chronic obstructive pulmonary disease (COPD) subtypes. Thorax. 2011;66(5):430–437. DOI:10.1136/thx.2010.154484
- Burgel PR, Paillasseur JL, Caillaud D, et al. Clinical COPD phenotypes: a novel approach using principal component and cluster analyses. Eur Respir J. 2010;36(3):531–539. DOI:10.1183/09031936.00175109
- Vanfleteren LE, Spruit MA, Groenen M, et al. Clusters of comorbidities based on validated objective measurements and systemic inflammation in patients with chronic obstructive pulmonary disease. Am J Respir Crit Care Med. 2013;187(7):728–735. DOI:10.1164/rccm.201209-1665OC
- Disantostefano RL, Li H, Rubin DB, et al. Which patients with chronic obstructive pulmonary disease benefit from the addition of an inhaled corticosteroid to their bronchodilator? A cluster analysis. BMJ Open. 2013;3(4):e001838. DOI:10.1136/bmjopen-2012-001838
- Cho MH, Washko GR, Hoffmann TJ, et al. Cluster analysis in severe emphysema subjects using phenotype and genotype data: an exploratory investigation. Respir Res. 2010;11:30. DOI:10.1186/1465-9921-11-30
- Pinto LM, Alghamdi M, Benedetti A, et al. Derivation and validation of clinical phenotypes for COPD: a systematic review. Respir Res. 2015;16:50. DOI:10.1186/s12931-015-0208-4
- Burgel PR, Paillasseur JL, Janssens W, et al. A simple algorithm for the identification of clinical COPD phenotypes. Eur Respir J. 2017;50(5):1701034. DOI:10.1183/13993003.01034-2017
- Breiman L. Classification and regression trees. Belmont, CA: Wadsworth International Group; 1984. (The Wadsworth statistics/probability series).
- Ikram MA, Brusselle G, Ghanbari M, et al. Objectives, design and main findings until 2020 from the rotterdam study. Eur J Epidemiol. 2020;35(5):483–517. DOI:10.1007/s10654-020-00640-5
- Sundh J, Aberg J, Hasselgren M, et al. Factors influencing pharmacological treatment in COPD: a comparison of 2005 and 2014. Eur Clin Respir J. 2017;4(1):1409060. DOI:10.1080/20018525.2017.1409060
- Bestall JC, Paul EA, Garrod R, et al. Usefulness of the medical research council (MRC) dyspnoea scale as a measure of disability in patients with chronic obstructive pulmonary disease. Thorax. 1999; Jul54(7):581–586. DOI:10.1136/thx.54.7.581
- Giezeman M, Hasselgren M, Lisspers K, et al. Influence of comorbid heart disease on dyspnea and health status in patients with COPD - a cohort study. Int J Chron Obstruct Pulmon Dis. 2018;13:3857–3865. DOI:10.2147/COPD.S175641
- Leening MJ, Kavousi M, Heeringa J, et al. Methods of data collection and definitions of cardiac outcomes in the rotterdam study. Eur J Epidemiol. 2012;27(3):173–185. DOI:10.1007/s10654-012-9668-8
- Quanjer PH, Stanojevic S, Cole TJ, et al. Multi-ethnic reference values for spirometry for the 3-95-yr age range: the global lung function 2012 equations. Eur Respir J. 2012;40(6):1324–1343. DOI:10.1183/09031936.00080312
- Miller MR, Hankinson J, Brusasco V, et al. Standardisation of spirometry [practice guideline]. Eur Respir J. 2005;26(2):319–338. DOI:10.1183/09031936.05.00034805
- Terzikhan N, Verhamme KM, Hofman A, et al. Prevalence and incidence of COPD in smokers and non-smokers: the rotterdam study. Eur J Epidemiol. 2016;31(8):785–792. DOI:10.1007/s10654-016-0132-z
- Hankinson JL, Odencrantz JR, Fedan KB. Spirometric reference values from a sample of the general U.S. population. Am J Respir Crit Care Med. 1999;159(1):179–187. DOI:10.1164/ajrccm.159.1.9712108
- Quanjer PH, Tammeling GJ, Cotes JE, et al. Lung volumes and forced ventilatory flows. Report working party standardization of lung function tests, European community for steel and coal. Eur Respir J Suppl. 1993;16:5–40.
- Arne M, Lisspers K, Stallberg B, et al. How often is diagnosis of COPD confirmed with spirometry? Respir Med. 2010; 104(4):550–556. DOI:10.1016/j.rmed.2009.10.023
- Celli BR, Cote CG, Marin JM, et al. The body-mass index, airflow obstruction, dyspnea, and exercise capacity index in chronic obstructive pulmonary disease. N Engl J Med. 2004;350(10):1005–1012. DOI:10.1056/NEJMoa021322
- Sundh J, Janson C, Lisspers K, et al. The dyspnoea, obstruction, smoking, exacerbation (DOSE) index is predictive of mortality in COPD. Prim Care Respir J. 2012;21(3):295–301. DOI:10.4104/pcrj.2012.00054
- Puhan MA, Garcia-Aymerich J, Frey M, et al. Expansion of the prognostic assessment of patients with chronic obstructive pulmonary disease: the updated BODE index and the ADO index. Lancet. 2009;374(9691):704–711. DOI:10.1016/S0140-6736(09)61301-5
- Guerra B, Haile SR, Lamprecht B, et al. Large-scale external validation and comparison of prognostic models: an application to chronic obstructive pulmonary disease. BMC Med. 2018;16(1):33. DOI:10.1186/s12916-018-1013-y
- Kim J, Lee CH, Hwang SS, et al. The ability of different scoring systems to predict mortality in chronic obstructive pulmonary disease patients: a prospective cohort study. Respiration. 2019;98(6):495–502. DOI:10.1159/000502826
- Divo M, Cote C, de Torres JP, et al. Comorbidities and risk of mortality in patients with chronic obstructive pulmonary disease. Am J Respir Crit Care Med. 2012;186(2):155–161. DOI:10.1164/rccm.201201-0034OC
- Ellingsen J, Johansson G, Larsson K, et al. Impact of comorbidities and commonly used drugs on mortality in COPD - Real-World data from a primary care setting. Int J Chron Obstruct Pulmon Dis. 2020;15:235–245. DOI:10.2147/COPD.S231296
- Bloom CI, Ricciardi F, Smeeth L, et al. Predicting COPD 1-year mortality using prognostic predictors routinely measured in primary care. BMC Med. 2019;17(1):73. DOI:10.1186/s12916-019-1310-0
- Morales DR, Flynn R, Zhang J, et al. External validation of ADO, DOSE, COTE and CODEX at predicting death in primary care patients with COPD using standard and machine learning approaches. Respir Med. 2018;138:150–155. DOI:10.1016/j.rmed.2018.04.003
- Bellou V, Belbasis L, Konstantinidis AK, et al. Prognostic models for outcome prediction in patients with chronic obstructive pulmonary disease: systematic review and critical appraisal. BMJ. 2019;367:l5358. DOI:10.1136/bmj.l5358
- Sawalha S, Hedman L, Backman H, et al. The impact of comorbidities on mortality among men and women with COPD: report from the OLIN COPD study. Ther Adv Respir Dis. 2019;13:175346661986005. DOI:10.1177/1753466619860058
- Baker EH, Janaway CH, Philips BJ, et al. Hyperglycaemia is associated with poor outcomes in patients admitted to hospital with acute exacerbations of chronic obstructive pulmonary disease. Thorax. 2006;61(4):284–289. DOI:10.1136/thx.2005.051029
- Jones RC, Donaldson GC, Chavannes NH, et al. Derivation and validation of a composite index of severity in chronic obstructive pulmonary disease: the DOSE index. Am J Respir Crit Care Med. 2009;180(12):1189–1195. DOI:10.1164/rccm.200902-0271OC
- Calverley PM, Anzueto AR, Dusser D, et al. Treatment of exacerbations as a predictor of subsequent outcomes in patients with COPD. COPD. 2018;13:1297–1308. DOI:10.2147/COPD.S153631
- Marin JM, Alfageme I, Almagro P, et al. Multicomponent indices to predict survival in COPD: the COCOMICS study. Eur Respir J. 2013;42(2):323–332. DOI:10.1183/09031936.00121012