Abstract
Home-based lung function measurements can be used to capture day-to-day variations in symptoms in patients with chronic obstructive pulmonary disease (COPD). Although dynamic hyperinflation (DH) is clinically relevant, existing home-based measurements do not include its assessment. DH can be measured through inspiratory capacity (IC) measurements before and after metronome-paced tachypnea test (MPT). The goal of this study is to determine the accuracy of unsupervised home-based IC and DH measurements in COPD.
Sixteen COPD patients performed IC and DH measurements during 4 home visits. Visit 1 was considered a training session. During all visits supervised and unsupervised IC at rest (ICREST) and after MPT (ICMPT) were measured. DH was calculated as the difference between ICREST and ICMPT, and as a percentage of ICREST. Bland-Altman analyses and ANOVA tests were performed to determine the effect of supervision and repeated measures over time.
The biases between supervised and unsupervised ICREST, ICMPT, ΔIC and ΔIC% were 0.007 L, 0.007 L, 0 mL and −0.09% in the last visit, respectively. Limits of agreement of ICREST and ΔIC% decreased from ±0.261 mL to ±0.201 mL, and from ±13.84% to ±10.81% between visit 1 and 4, respectively. No significant effect of supervision or over time was found.
After a robust training and a learning phase, COPD patients are able to perform IC measurements in an accurate manner in both rest and after MPT. This yield accurate assessment of DH, in an unsupervised home-based setting.
Introduction
Chronic obstructive pulmonary disease (COPD) is characterized by normal day-to-day variations in respiratory symptoms. Regular outpatient visits in respiratory clinics often include measurements of lung function in these patients. These measurements, however, merely present a snapshot of the overall condition of the patient, rather than capturing daily functional or symptom variations. Home-based lung function measurements can be used to gain more insight in the day-to-day condition of a patient. Also, home-based measurements have been hypothesized to aid in early detection and prediction of COPD exacerbations, and may have added value in monitoring effects of (pharmaceutical) interventions [Citation1]. Before home-based measurements can be further explored as possible extensions of COPD care, determining to whether patients can accurately perform these measurements in an unsupervised manner is conditional.
Existing home-based lung function measurements are mainly limited to expiratory variables such as forced expiratory volume in one second (FEV1) and forced vital capacity. Various studies [Citation1, Citation2] show that unsupervised measurements of FEV1 are accurate in comparison to office-based supervised spirometry, and only slightly smaller than supervised measurements. Yet, it is known that these parameters do not correlate well with symptoms, such as dyspnea and exercise intolerance [Citation3]. Static and dynamic hyperinflation (DH) stronger correlate with symptoms and are therefore more clinically relevant to measure [Citation4, Citation5]. DH is defined as a temporary increase in end-expiratory lung volume, determined by an increased time constant of the lungs during exhalation [Citation6]. Exhalation time can become insufficient in ventilatory demanding tasks, or prior to/during an exacerbation [Citation6–8]. Nonetheless, measurement of DH is not often included in home-based measurements, probably because its assessment is more laborious and might be more causing incorrect outcomes. A frequently used test to measure DH is the metronome-paced tachypnea test (MPT) [Citation9], in which ventilation is voluntarily raised by doubling the resting breathing frequency. DH is then evaluated comparing inspiratory capacity (IC) between rest and after MPT.
Implementation of MPT for monitoring DH as part of existing telemonitoring systems may be feasible if patients are able to perform these home-based measurements validly and unsupervised. Only little is known about the accuracy of unsupervised IC measurements in rest. Two small studies have shown that variation in unsupervised ICs in rest decreases after multiple measurement days, however, they remain smaller than clinically supervised ICs in rest [Citation10, Citation11]. To the knowledge of the authors, no studies have been performed demonstrating the accuracy of unsupervised measurements of IC after MPT and DH. Therefore, the goal of this study was to make a comparison between supervised and unsupervised measurements of DH based on IC maneuvers in rest and after MPT in patients with COPD. We hypothesized that COPD patients can perform home-based unsupervised MPT and IC measurements with a small bias in comparison to supervised measurements.
Methods
Subjects
This study was conducted at Radboud University Medical Center in Nijmegen (The Netherlands) and was approved by the medical-ethical committee on Research Involving Human Subjects of Arnhem-Nijmegen (NL77269.091.21). Subjects were included from the outpatient clinic if they were diagnosed with COPD and mastered the Dutch language. Subjects were excluded if they were physically unable to perform lung function or experienced an exacerbation within 6 weeks prior to the study. All patients signed informed consent. COPD severity was graded according to the Global Initiative for Chronic Lung Disease (GOLD) [Citation12].
Design
Home visits
A total of four home visits within four weeks were scheduled. Visit 1 was considered a training visit in which oral and written instructions for MPT and IC measurements were provided after which the participant performed two supervised measurements, followed by two unsupervised measurements. During visit 2, 3, and 4, the participant performed one unsupervised measurement followed by one supervised measurement. The sequence of the measurements was changed on purpose considering visit 1 and visits 2-4 to determine that the sequence of the measurements did not result in a significant bias.
IC Measurements
A calibrated mobile spirometer (Vyntus™ SPIRO, Vyaire™) with disposable antiviral and antibacterial filter connected to a laptop was used to measure lung volumes. During IC measurements, participants were instructed to use a nose clip and maintain a relaxed upright seating position. One measurement consisted of three IC maneuvers at rest and three MPTs each followed by an IC maneuver (). During MPT, a metronome was used for 25 s to achieve a breathing frequency twice the resting breathing frequency. Recovery after MPT was at least thirty seconds.
Figure 1. Spirometry protocol for one measurement. BF = Breathing Frequency; MPT = Metronome-paced Tachypnea; IC = Inspiratory Capacity.
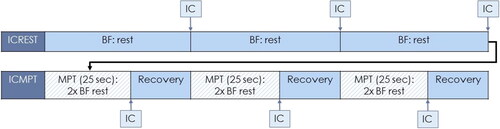
In both ICREST and ICMPT, an IC measurement was rejected if the deviation was more than 10% or 0.150 L compared to the other two IC measurements [Citation13]. ICREST was defined as the mean of two or three IC maneuvers before first MPT. ICMPT was defined as the mean of two or three IC maneuvers performed after three MPTs. DH was defined as the difference between ICREST and ICMPT in absolute volume (ΔIC) and as a percentage of ICREST (ΔIC%) [Citation14]. Supervised DH measurements were compared to unsupervised DH measurements. The participant was encouraged to inhale maximally on indication of the researcher in the supervised measurements only. In the unsupervised measurements, maximal inhalation occurred on indication of a timer during rest or when the metronome stopped (i.e. after 25 s).
Statistics
Statistical analyses were performed using IBM SPSS Statistics Version 25. Data were tested for normality and presented accordingly with mean (SD) or median (IQR).
The effect of supervision and over time on ICREST, ICMPT, ΔIC or ΔIC% was assessed using a type II two-way ANOVA for repeated measures. Interaction between supervision and time was investigated and accordingly an ANOVA with or without interaction was performed. Bland-Altman analysis was used to assess similarity between the supervised and unsupervised parameters (ICREST, ICMPT, ΔIC and ΔIC%). A priori, we used the clinical standard [Citation13] to set rules for agreement. Agreement between supervised and unsupervised measurements of ICREST and ICMPT was considered good when (1) limits of agreement were smaller than 0.150 L and (2) the bias was close to zero. For ΔIC%, we used the studies of Lahaije et al. [Citation15] and Mannée et al. [Citation14] to set the rules for agreement. Agreement was considered good when (1) limits of agreement were smaller than 11.1% and (2) the bias was close to 0%.
The coefficient of variation (std/mean) of all tests was determined and an average coefficient of variation was presented for ICREST and ICMPT in both supervised and supervised measurements. The number of rejected ICs (rejection according to the earlier mentioned method) was reported.
Results
Subjects
Thirty-one potential candidates were approached by the researcher. Sixteen COPD patients agreed upon participation and were visited at their homes between June and Augusts 2021. One participant was excluded due to an exacerbation after the first visit. The remaining fifteen participants had a mean age of 63.0 years (SD 7.2) and a mean BMI of 26.7 kg/m2 (SD 6.3). Eleven (69%) of the participants were female. Main part of the patients was characterized with severe and very severe COPD according to the GOLD stages (GOLD 1 (n = 1), 2 (n = 2), 3 (n = 8) and 4 (n = 4). An overview of the individual patient characteristics is available in .
Table 1. Patients characteristics per subject.
Accuracy of IC at rest and after MPT
The mean supervised and unsupervised ICREST were 1.844 L and 1.842 L in visit 2, respectively (). Comparable results were found in other visits (). The repeated measures two-way ANOVA showed no significant effect of supervision (p = 0.936), effect over time (p = 0.632) or interaction (p = 0.964). A bias of 0.003 L was observed between both measurements (). Comparable negligible biases were found in the comparisons of supervised ICREST and unsupervised ICREST measurements of visit 3 and 4 ().
Table 2. Mean ICREST and ICMPT, ΔIC and ΔIC% supervised and unsupervised. SD = Standard Deviation.
Table 3. Bias and limits of agreement for ICREST, ICMPT, ΔIC and ΔIC% from visits 2, 3 and 4.
The mean supervised and unsupervised ICMPT were 1.388 L and 1.411 L in visit 2, respectively (). In other visits comparable results were found (). The repeated measures two-way ANOVA showed no significant effect of supervision (p = 0.637), effect over time (p = 0.989) or interaction (p = 0.759). A bias of −0.023 L () was observed between both measurements in visit 2. A comparable bias was found in the comparison of supervised ICMPT and unsupervised ICMPT measurements of visit 3 and 4 ().
In visit 2, the predefined threshold of ± 0.150 L was exceeded by limits of agreement of ± 0.261 L and ± 0.233 L for ICREST and ICMPT, respectively. Comparable limits of agreement were found in visit 3 and 4 (). During ICREST measurements, four out of fifteen (26.7%) participants exceeded the 0.150 L threshold in visit 2. On visits 3 and 4, 20% and 13.3% exceeded the 0.150 L threshold, respectively. Comparable results were found during ICMPT measurements (data not shown). Two patients exceeded the limits of agreement in all visits. The population average for the coefficient of variation (std/mean) was 0.03 (0.01) for both supervised ICREST and ICMPT, and 0.03 (0.02) for unsupervised ICREST and 0.04 (0.02) for unsupervised ICMPT. These results were found in visit 2, and comparable results were observed in subsequent visits (data not shown). Based on the ATS/ERS criteria for reproducibility, 2.2%, 7.4%, 3.0% and 6.7% of the 135 IC maneuvers (total 26 out of 540 maneuvers) had to be removed from further analysis for the supervised ICREST, supervised ICMPT, unsupervised ICREST and unsupervised ICMPT, respectively.
Accuracy of ΔIC and ΔIC%
DH occurred in all participants. In visit 2, the mean ΔIC were −456 mL and −431 mL, respectively. The mean supervised and unsupervised ΔIC% were −24.53% and −22.96%, respectively (). In other visits comparable results were found (). The repeated measures two-way ANOVA showed no significant effect of supervision (p = 0.585), effect over time (p = 0.479) or interaction (p = 0.875) for ΔIC. For ΔIC%, the repeated measures two-way ANOVA showed no significant effect of supervision (p = 0.588), effect over time (p = 0.784) or interaction (p = 0.813).
Bland Altman plots show the agreement between ΔIC and ΔIC% derived from supervised measurements and ΔIC and ΔIC% from unsupervised measurements in visit 2 and 4 ( and ). The bias of ΔIC was −25 mL for visit 2 and 0 mL for visit 4. A bias of −1.57% was observed in visit 2 (). Comparable results were found for visit 3 (data not shown). Visit 4 showed a bias of −0.09% ().
Figure 2. Bland Altman plot comparing ΔIC (mL) supervised and ΔIC (mL) unsupervised measured at visit 2 on right. Bias (solid) = –0.025 mL; Limits of agreement (solid) = upper: 0.252 mL, lower: –0.303 mL; Bland Altman plot comparing ΔIC% supervised and ΔIC% unsupervised measured at visit 2 on left. Bias (solid) = 1.57%; Limits of agreement (solid) = upper: 12.27%, lower: –15.41%; Threshold = 11.1% (dashed). Threshold = 150 mL (dashed). Regression lines (not displayed) were non-significant.
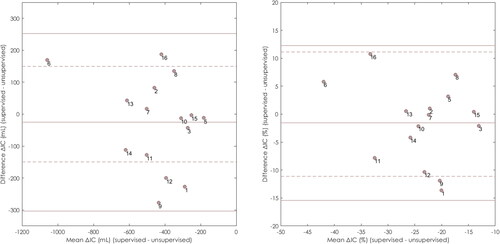
Figure 3. Bland Altman plot comparing ΔIC (mL) supervised and ΔIC (mL) unsupervised measured at visit 4 on right. Bias (solid) = 0.000 mL; Limits of agreement (solid) = upper: 0.205 mL, lower: –0.205 mL; Bland Altman plot comparing ΔIC% supervised and ΔIC% unsupervised measured at visit 4 on left. Bias (solid) = 0.09%; Limits of agreement (solid) = upper: 10.72%, lower: –10.89%; Threshold = 11.1% (dashed). Threshold = 150 mL (dashed). Regression lines (not displayed) were non-significant.
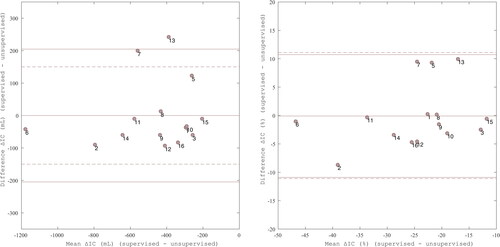
The predefined threshold of ± 150 mL was exceeded by limits of agreement of ± 278 mL and ± 333 mL in visit 2 and 3, respectively. Five out of fifteen subjects (33.3%) in visit 2, and five out of fifteen subjects (33.3%) in visit 3 exceed the 150 mL threshold. In visit 4, only two subjects exceeded the threshold, and the limits of agreement were ± 205 mL within the 150 mL threshold (). The predefined threshold of ± 11.1% was exceeded by limits of agreement of ± 13.84% and ± 16.91% in visit 2 and 3, respectively. Two out of fifteen subjects (13.3%) in visit 2, and three out of fifteen subjects (20.0%) in visit 3 exceed the 11.1% threshold. In visit 4, the limits of agreement of ± 10.81% were within the −11.1% and 11.1% thresholds ().
Discussion
In this study, we show that unsupervised home-based measurements of resting IC and after MPT were accurately performed by COPD patients after a training session. A bias close to zero was found comparing supervised and unsupervised measurements with decreasing limits of agreement over the course of three follow-up visits within a time span of 4 weeks. This yielded accurately measured ΔIC and ΔIC%. ANOVA showed that supervised and unsupervised measurements showed the same results and that repeated measures (learning effect) did not change the outcomes. However, it is important to add a robust and dedicated training to familiarize patients with the measurements and to increase precision. From this study, we can conclude that it is feasible to measure IC and DH in patients with COPD in an unsupervised environment.
To our knowledge only one previous study compared supervised and unsupervised IC maneuvers in patients with COPD. Holmner et al. [Citation10] compared supervised IC measured in the lab and the consecutive unsupervised IC measurements at home performed by 13 patients. Whereas in that study all unsupervised IC measurements turned out lower than those from the lab, we found no difference between supervised and unsupervised IC measurements in our study, as showed with the ANOVA. This might be explained by the duration of initial training. Holmner et al. [Citation10] applied a 15-20 min training, in which the IC measurements were only a minor element. We dedicated a whole visit of 1.5 h to properly instruct the subjects to perform IC and MPT measurements in combination with multiple measurements to practice the maneuvers. A robust and dedicated familiarization training thus appears a prerequisite.
Although the bias between supervised and unsupervised was close to zero, it should be acknowledged that our predefined threshold of 0.150 L was exceeded in all visits. This may have been caused by a learning phase, natural variation, or improper execution of the measurements in individual patients despite training, as we will discuss below.
First, the difference between supervised and unsupervised measurements of IC in rest seemed to decrease over the visits (decreasing limits of agreement), indicating the presence of a learning effect. Öhberg et al. [Citation16] performed a pilot study (n = 4) to evaluate the repeatability of unsupervised home based pulmonary measurements, including IC at rest. Likewise, they concluded that there was a learning effect, based on a decrease in within-test variability of IC in rest over time. In the current study, the decrease in limits of agreement is likely to have originated from a decrease in individual bias between supervised and unsupervised measurements. We did not find the same learning effect in measurements after MPT, which might be explained by the limited possibility for a patient to vary their breathing pattern prior to the IC maneuvers in MPT. Second, our threshold was selected based on the ATS/ERS standards [Citation13], which stated that the within test variability of serial IC maneuvers should not exceed 150 mL (or 10%). Although this threshold may be realistic for intertest variability, it may not represent the re-test variability (limits of agreement of test-retest comparison). This is supported by the research of Visser et al. [Citation17], who found a coefficient of repeatability of IC in rest of 19%, comparing the baseline test with a test repeated in one hour. As the supervised and unsupervised measurements in our study were also performed within one hour, limits of agreement found in the current study may be the result of the re-test variability. This suggests that the pre-determined thresholds may be too strictly chosen. Finally, two patients exceeded the threshold in all four visits, resulting in overall wider limits of agreement for the whole group. These patients showed a slightly higher coefficient of variation (data not shown). Therefore, we hypothesize that it is likely that some patients may not be able to perform these measurements adequately. Patients ineligible for these measurements may be identified in the learning phase.
The results of the Bland-Altman analysis for ΔIC and ΔIC% were comparable to the previously described resting and MPT analyses. Unsupervised ΔIC and ΔIC% were measured accurately with a bias close to zero. Although limits of agreement exceeded the chosen threshold in 2 out of 3 visits, this was likely to be the result of the three above mentioned reasons (learning phase, natural variation, two recurrently underachieving patients). Therefore, we may conclude that COPD patients are able to accurately perform home-based unsupervised measurements of MPT-induced DH with training and after the learning phase has been completed.
Home-based unsupervised MPT-induced DH measurements can potentially be used to gain more insight in the day-to-day condition of the patient. It could provide DH monitoring without regular outpatient visits. This can improve the quality of life of COPD patients as lung physicians can remotely assess their patients’ lung function over time and take adequate actions when needed.
Limitations
The main limitation of this study is the small sample size. A larger sample size may decrease the variation, unless the observed variation also reflects the true natural variation.
Although this study showed that unsupervised measurements of IC, ΔIC and ΔIC% are accurate, this is not enough to implement these measurements as an extension of clinical care or in telemonitoring systems at this moment. Studies including pulmonary function (FEV1 and peak expiratory flow rate) at home rely on the definition of “normal fluctuations” of both parameters over time [Citation16]. Accordingly, the minimal clinically important difference for home-based dynamic hyperinflation should be determined in order to draw firm conclusions on the fluctiations found in an individual patient. The natural variation is important to consider when determining the MCID as a value below the natural variation would be unusable. As we discussed earlier, the day-to-day variation in IC over time is a scarcly investigated topic. Future studies should extend Holmners research [Citation10] and ours by investigation of IC in rest, IC after MPT, ΔIC and ΔIC% after proper training and “learning phase”.
Conclusion
COPD patients are able to perform home-based unsupervised measurements of IC in rest and after MPT. For all measurements we found that on average supervised and unsupervised measurements were equal; with a bias close to zero. The decrease in limits of agreement found between visit 1 and 4 suggests that it is important to include a training and learning phase, as patients require some time to get used to performing unsupervised measurements. In conclusion, measurements of static and dynamic hyperinflation in patients with COPD are feasible to perform in an unsupervised environment.
Declaration of interest statement
The authors report no conflict of interest.
Acknowledgements
None.
Funding
The author(s) reported there is no funding associated with the work featured in this article.
References
- Rodriguez-Roisin R, Tetzlaff K, Watz H, et al. Daily home-based spirometry during withdrawal of inhaled corticosteroid in severe to very severe chronic obstructive pulmonary disease. Int J Chron Obstruct Pulmon Dis. 2016;11:1973–1981. DOI:10.2147/COPD.S106142
- Mortimer KM, Fallot A, Balmes JR, et al. Evaluating the use of a portable spirometer in a study of pediatric asthma. Chest. 2003;123(6):1899–1907. DOI:10.1378/chest.123.6.1899
- Wedzicha JA, Donaldson GC. Exacerbations of chronic obstructive pulmonary disease. Respir Care. 2003;48(12):1204–1213. discussion 1213-5.
- O’Donnell DE, Lam M, Webb KA. Spirometric correlates of improvement in exercise performance after anticholinergic therapy in chronic obstructive pulmonary disease. Am J Respir Crit Care Med. 1999;160(2):542–549. DOI:10.1164/ajrccm.160.2.9901038
- O’Donnell DE, Laveneziana P. Dyspnea and activity limitation in COPD: mechanical factors. Copd. 2007;4(3):225–236. DOI:10.1080/15412550701480455
- Ferguson GT. Why does the lung hyperinflate? Proc Am Thorac Soc. 2006;3(2):176–179. DOI:10.1513/pats.200508-094DO
- Gibson GJ. Pulmonary hyperinflation a clinical overview. Eur Respir J. 1996;9(12):2640–2649. DOI:10.1183/09031936.96.09122640
- O’Donnell DE, Webb KA, Neder JA. Lung hyperinflation in COPD: applying physiology to clinical practice. [journal article]COPD Res Pract. 2015;1(1):4. DOI:10.1186/s40749-015-0008-8
- Gelb AF, Gutierrez CA, Weisman IM, et al. Simplified detection of dynamic hyperinflation. Chest. 2004;126(6):1855–1860. DOI:10.1378/chest.126.6.1855
- Holmner Å, Öhberg F, Wiklund U, et al. How stable is lung function in patients with stable chronic obstructive pulmonary disease when monitored using a telehealth system? A longitudinal and home-based study. BMC Med Inform Decis Mak. 2020;20(1):87. DOI:10.1186/s12911-020-1103-6
- Öhberg F, Wadell K, Blomberg A, et al. Home-Based system for recording pulmonary function and Disease-Related symptoms in patients with chronic obstructive pulmonary disease, COPD-A pilot study. SM J Pulm Med. 2016;2:1011.
- Mirza S, Clay RD, Koslow MA, et al. COPD guidelines: a review of the 2018 GOLD report. Mayo Clin Proc. 2018;93(10):1488–1502. DOI:10.1016/j.mayocp.2018.05.026
- Miller MR, Hankinson J, Brusasco V, ATS/ERS Task Force, et al. Standardisation of spirometry. Eur Respir J. 2005;26(2):319–338. DOI:10.1183/09031936.05.00034805
- Mannée D, Vis E, Hoekstra-Kuik A, et al. Is the Metronome-Paced tachypnea test (MPT) ready for clinical use? Accuracy of the MPT in a prospective and clinical study. Respiration. 2019;97(6):569–575. DOI:10.1159/000496290
- Lahaije AJ, Willems LM, van Hees HW, et al. Diagnostic accuracy of metronome-paced tachypnea to detect dynamic hyperinflation. Clin Physiol Funct Imaging. 2013;33(1):62–69. DOI:10.1111/j.1475-097X.2012.01164.x
- Öhberg F, Wadell K, Blomberg A, et al. Home-based system for recording pulmonary function and disease-related symptoms in patients with chronic obstructive pulmonary disease, COPD: a pilot study. SM Journal of Pulmonary Medicine. 2016;2(1):1–6.
- Visser FJ, Ramlal S, Pelzer B, et al. Random variation of inspiratory lung function parameters in patients with COPD: a diagnostic accuracy study. BMC Pulm Med. 2010;10(1):28. DOI:10.1186/1471-2466-10-28