Abstract
Chronic obstructive pulmonary disease (COPD) is a common lung disease that negatively affects health-related quality of life (QoL). Utility values, which measure QoL by weighting health states with societal preferences, are required for the cost-utility models that drive economic evaluations and policy decisions. Moayeri et al. published a systematic review and meta-analysis of utilities (EQ-5D) in COPD in June 2016. The current study investigated changes in mean utilities in more recent studies thereafter, exploring heterogeneity in utilities across diverse clinical and study characteristics. Systematic searches of databases, such as MEDLINE and Embase were undertaken from 1 July 2015 until 20 May 2024. A random-effects meta-analysis of utilities (EQ-5D) was performed which addressed inter-study heterogeneity and subgroup analyses. The pooled general mean (95% CI) utility value was 0.761 (0.726–0.795) from 43 studies, whereas Moayeri et al. reported 0.673 (0.653–0.693) from 32 studies. This improvement in mean utilities could be due to increased awareness, early detection, and better medical interventions over the past decade, but demonstrates that a general utility value should be approached with caution given significant heterogeneity. Four meta-regressions were performed on each subgroup: region, method of elicitation, reported comorbidities, and disease stage; of which, method of elicitation, disease stage, and region were found to be significant moderators of utilities. It is, therefore, important to use meta-analysed utilities for cost-utility analyses that reflect the context and patient population of the model. Moreover, these results provide additional evidence for the precision and sensitivity of EQ-5D-5L over EQ-5D-3L.
Introduction
Chronic obstructive pulmonary disease (COPD) is a common lung disease that causes airflow obstruction and breathing difficulties [Citation1]. Long-term exposure to air pollution has been identified as a risk factor for COPD [Citation2], along with tobacco smoking, occupational exposure, asthma and genetics [Citation3]. The prevalence and impact of COPD have been associated with poor socioeconomic status in the UK and in many other countries [Citation4]. The progressive course of the disease negatively affects a sufferer’s health-related quality of life (QoL) [Citation5], usually referred to as utility values which are measured on a scale between 0 (death) and 1 (perfect health) that represent the strength of preference weights for different health outcomes.
Cost-utility analyses are used by the National Institute for Health and Care Excellence (NICE) to evaluate the cost-effectiveness of interventions and make evidence-based recommendations [Citation6]. Utilities are used as a weighting for health outcomes in cost-utility analyses which use quality-adjusted life-years (QALYs), a composite measure of quality and length of life. However, challenges may arise when utilities are not available, lack sufficient quality, or demonstrate heterogeneity. This challenge is particularly relevant in the context of COPD, a group of lung conditions that lead to breathing difficulties, such as emphysema, chronic bronchitis, and chronic obstructive airway disease. Identifying the most appropriate utility values for determining the cost-effectiveness of interventions for COPD-related issues can be problematic.
Estimation of utilities is a difficult area of research. Both generic (e.g. EQ-5D) and disease-specific QoL measurements (e.g. St. George Respiratory Questionnaire) are used to estimate utilities for patients with COPD. However, utilities produced by generic measures are preferred in cost-utility analyses as they allow comparison across diseases [Citation7], and when they are not available, some disease-specific measures can be mapped onto utilities produced by generic instruments [Citation8]. NICE prefers using utilities based on the generic EQ-5D instrument in health economic analyses to provide consistency across guidance and appraisals [Citation9, Citation10]. The EQ-5D has two versions: EQ-5D-3L and EQ-5D-5L. Respondents assess their impairment in five health domains: mobility, self-care, usual activities, pain/discomfort, and anxiety/depression. The 3L version has three response levels per domain, while the 5L version offers five levels for increased sensitivity. The NICE methods manual currently favours EQ-5D-3L until a quality 5L value set is available [Citation11].
While there is a substantial body of literature measuring utility values of people with COPD, there is significant variability in utility values across studies. This issue of utility variability within COPD has been previously acknowledged [Citation7] and poses a challenge to ensuring consistency in cost-utility analyses. When a large number of utility values are available, a systematic approach is needed to identify the most relevant value considering factors, such as the method, robustness, and publication date of the evidence, as well as the specific research question and population of interest. It is important for researchers conducting cost-utility analyses to base their choice of utilities on the most reliable and up-to-date sources. When possible, researchers should strive to include all available high-quality evidence that is relevant to the specific research question and population of interest in their cost-utility models [Citation12].
Moayeri et al. conducted a systematic literature review and meta-analysis of utilities (EQ-5D) in the area of COPD and found significant heterogeneity between studies (I2 = 97.7%) [Citation7]. Building on this, the primary aim of the current study was to investigate potential changes in mean utility values in more recent studies. It also sought to explore potential variations in utility values across diverse clinical and study characteristics with a focus on heterogeneity and variability. Additionally, the study aimed to pool utility estimates from homogeneous studies and conduct a meta-regression to explore whether any differences between studies can be explained by specific pre-defined moderators, which were based on factors known to influence health utility values, such as disease progression, country setting and choice of instrument [Citation13].
Methods
Study design
This review complies with the PRISMA checklist [Citation14]. A review protocol was produced (available from the corresponding author on request) and set out the process to provide an updated version of the systematic review and meta-analysis conducted by Moayeri et al. [Citation7]. As such, the search strategy and inclusion and exclusion criteria from Moayeri et al. [Citation7] were replicated in this study.
Search strategy
A systematic search adapted from the databases used by Moayeri et al. [Citation7] was conducted on 20 May 2024. Those databases included MEDLINE, Embase, Web of Science, CINAHL, ProQuest (which includes PsycINFO and other 61 databases), the Cochrane Library Database (which includes NHS Economic Evaluation Database, Health Technology Assessment Database, Cochrane Database of Systematic Reviews and other three databases), International Society for Pharmacoeconomics and Outcomes Research (ISPOR) and Google Scholar, as well as dissertation and web sites of key academic institutions, such as NICE (National Institute of Health and Care Excellence), CCOHTA (Canadian Cooperating Office for Health Technology Assessment), SBU (The Swedish Council on Technology Assessment in Health Care), Health Economic Evaluations Database (HEED, ceased publishing in 2014) and the Cost Effectiveness Analysis Registry at Tufts-New England Medical Centre. Adapted databases (included in ) were searched from 1 July 2015 until 20 May 2024. The search terms included a combination of MeSH and free text terms based on Moayeri et al. [Citation7].
Inclusion and exclusion criteria
Studies were included only if they used EQ-5D to estimate utility values for patient level research in COPD.
In addition, studies were identified as being eligible for inclusion if they were:
studies on health utility that were published from July 2015 until 20 May 2024;
studies in which their sample population was specifically categorised as COPD as defined by standard criteria for COPD diagnosis and spirometric confirmation (should clearly be addressed in methodology of included studies);
English language studies and non-English language studies with English abstracts;
abstracts (e.g., seminar abstracts) and reports if adequate data for analysis were provided;
studies with more than 10 participants.
Studies were excluded according to the following criteria:
simulation-based studies
editorials/opinion pieces, letters, systematic reviews and meta-analyses;
studies that reported utilities from proxies, not individual participant data (e.g., reported by family member or a health professional);
studies that obtained utility estimates from the literature, if there was not enough information on the derivation of utility;
studies that did not distinguish COPD from other types of obstructive pulmonary disease, such as asthma or cystic fibrosis;
studies using utility values mapped from other reported QoL studies;
studies that reported utility values from non-stable and exacerbation state COPD patients.
In addition, studies were examined in which results were measured from populations duplicated in other studies and excluded those studies that were less comprehensive and less recent than other studies measuring the same population.
Study selection and data extraction
Two authors (NS: 100% and YZ: 100%) reviewed titles and abstracts of the identified studies. Where it was unclear whether a study should be included it was carried forward to the next stage. During this stage, NS and YZ independently reviewed the full text of the relevant studies, with a 100% review rate each. Studies that met the predefined inclusion criteria were then carried forward to the data extraction process. Any disagreements about study eligibility were discussed with another author (SM) and resolved by consensus.
Data extraction from all included studies was performed independently by NS (100%) and YZ (100%), and any disagreements were resolved by consensus among NS, YZ, and SM. Data were extracted in Microsoft Excel, and the following data were recorded: principal author, year of publication, number of patients, country of origin, clinical characteristics and demographic of patients, age (mean and standard deviation), sex, comorbidities, study design, data collection method, health state utility value measure and utility estimate (mean and standard deviation, or where unavailable upper and lower confidence intervals), FEV1/VC (mean and standard deviation) and FEV% predicted (mean and standard deviation). The method adopted by Moayeri et al. [Citation7] was replicated in this study where baseline characteristics were used to avoid any potential influence of the intervention on utility estimates.
Data synthesis
Extracted data was grouped according to [Citation1]: disease stage [Citation2], population (including age, sex, and region) [Citation3], comorbidities, and [Citation4] method of utility elicitation (i.e. EQ-5D-5L vs. EQ-5D-3L). A first look at the data indicated that disease stage, country, comorbidities, and method of elicitation may be sources of heterogeneity. Given that the country category yielded subgroups with too few data points for a meaningful analysis, the continent was used as the region category. Separate meta-analyses were conducted thereafter, one for each subgroup.
Point estimates and 95% confidence intervals (CIs) for utility values were calculated and displayed in forest plots. Moayeri et al.’s [Citation7] restriction of their meta-analysis to EQ-5D to avoid heterogeneity was replicated in this study; and additional heterogeneity introduced by elicitation methodology was explored in subgroup analyses of EQ-5D-5L and EQ-5D-3L.
Meta-analyses of subgroups were conducted with the meta package in R v4.3.0. To account for the expected between-study heterogeneity, a random-effects model was used to estimate the distribution of true mean utility values. The I2 statistic = 100% × (Q − df)/Q and 95% CI was used to indicate a relative measure of between-study variation as well as sampling error. Aligning with Moayeri et al. [Citation7], I2 values of 30–60, 50–90, and 75–100% were classified as moderate, substantial, and considerable heterogeneity, respectively. If standard deviations of utility values were not reported, they were calculated from 95% confidence intervals. Where studies did not report enough data for this calculation, they were excluded.
The t-test and analysis of variance (ANOVA) were applied to compare estimated utility means between subgroups. Moayeri et al. [Citation7] did not perform any meta-regression, possibly because their meta-analysis did not possess a sufficient sample size following the recommendation to include at least ten studies per covariate [Citation15]. However, given that for this study, the subgroups region, disease stage, method of elicitation, and comorbidities each had sufficient data points, we determined that conducting a meta-regression for each subgroup would be a valuable tool to evaluate the factors contributing to variability among utility values. A model was constructed for each subgroup separately such that the region model assessed the impact of regional differences on the outcome; the disease stage model assessed the impact of disease stage on the outcome; and so on. The meta-regression was used to interpret the impact of the inclusion of covariates on residual heterogeneity (defined as the variance in mean utility value) for each subgroup. These analyses were performed with the rma function of the metafor package in R v4.3.0.
Results
Study characteristics
A total of 5912 records were retrieved through database searching, of which 3044 were included after duplicates were removed. After title and abstract screening, 2945 records were removed and 99 remained to be screened on full text, after which 34 records were excluded.
Sixty-five studies reported EQ-5D based utility values for adults with COPD, either in stages or as an overarching condition, and were included in the review. However, 23 of these studies were excluded from the meta-analysis because they did not report mean utility values (and further did not provide enough information to infer mean utility values), did not sample from more than ten participants, or treated COPD as a comorbidity rather than the primary condition. A further two Korean studies (and all their observations) were excluded given that utility values were extracted from overlapping datasets (the Korean National Health and Nutrition Examination Survey) which would otherwise have skewed the meta-analysis. Some studies reported multiple data points e.g. studies that included utility values for different levels of COPD severity. Thus, a total of forty-two studies were included which yielded 104 data points that were used in the meta-analysis.
Moayeri et al. [Citation7] excluded studies that were deemed to have extreme utility values (>0.96); and therefore, the same approach was taken in the current study. However, no studies were found with extreme utility values, which were not previously excluded for other reasons.
Study characteristics are detailed in . Age and sex data were reported for 37 and 39 studies, respectively. Of these study participants, 64.94% were male and the average age per study was 66.6 years. Of the studies that reported FEV1% predicted, the study average was 54.5%, which indicated moderate airflow obstruction according to the Global Initiative for Chronic Lung Disease (GOLD) [Citation16].
Table 1. Study characteristics.
details the estimated mean utility value per study data point. Most data points were collected in Asia (n = 54) and the most common method of elicitation was EQ-5D-3L (n = 79). There was an almost equal spread across reporting of comorbidities (52 reported, whereas 51 did not). For data points associated with the disease stage, the largest category was mild, and the smallest category was very severe.
Table 2. Mean utility values per study data point.a
Meta-analyses
Meta-analyses were conducted for groups of observations that were agreed by the authors on a priori grounds. Specifically, based on existing literature [Citation13], studies were grouped according to region, stage of COPD, elicitation method (EQ-5D-5L vs. EQ-5D-3L), and the presence of comorbidities. These groupings are referred to as moderators below. shows the results of random-effects models for each subgroup, with k representing the number of data points.
Table 3. Estimated mean utility values by region, disease severity, method of elicitation, and reported comorbidities.
below shows the mean utility value for each subgroup and mean utility value overall for each model: common (fixed) effect and random-effects. Both mean values are reported here, but the main analysis focuses on the random-effects model. This decision was made as a result of substantial heterogeneity (I2 = 89%) between data points.
Figure 2. Summary forest plot of subgroups and moderators. I2 represents between data point heterogeneity for all 99 data points.
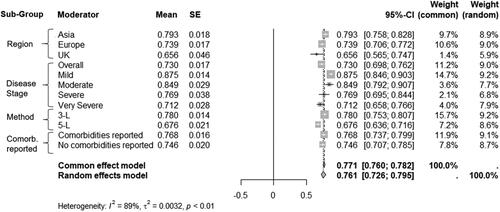
The random-effects model yielded a mean utility value of 0.761 (95% CI: 0.726–0.795) for the current study which is significantly higher than the mean utility value of 0.673 (95% CI: 0.653–0.693) reported by Moayeri et al. [Citation7]. Notably, the confidence intervals do not overlap, indicating a statistically significant difference between the two mean utility estimates.
Test of moderators
For the analysis of subgroup moderators, four meta-regressions with categorical moderators were used to test the significance of the subgroup moderators in each of the four subgroups.
For the method of elicitation (EQ-5D-3L vs. EQ-5D-5L), disease stage, and region subgroups, it was found that the moderators in each subgroup had a statistically significant impact on the effect sizes in the meta-analysis. In other words, whether the method of elicitation was EQ-5D-3L or EQ-5D-5L is associated with the differences in utility values across the studies in that subgroup. Similarly, whether the disease stage was mild, moderate, severe, or very severe, and whether the region was Asia, UK, or Europe, had a significant effect on utility values across the disease stage and region subgroups, respectively. There was not enough evidence to determine whether the reporting of comorbidities was associated with the differences in utility values for this subgroup.
The meta-regression analyses revealed that the method, disease stage, and region covariates significantly explained a portion of the heterogeneity among data points in each subgroup. Specifically, the residual heterogeneity (defined as the variance in mean utility value) decreased by 15.89% when accounting for the moderators in the disease stage model, by 11.23% in the method model, and by 10.97% in the region model, indicating that the included covariates in each model account for a significant portion of the variability in effect sizes for each subgroup.
Egger’s test was used to assess potential publication bias in the meta-analysis. There was low evidence of potential publication bias (p-value = 0.002); however, when between-study heterogeneity (tau-squared) is large, bias detection tests are not likely to be accurate [Citation60]. As such, Egger’s test of study bias was undertaken again for subgroups where between-study heterogeneity (tau-squared) was smaller than that of all studies taken together. below indicates that the hypothesis that there is no publication bias for each subgroup’s meta-analysis is unjustified.
Table 4. Egger’s tests for publication bias per sub-group.
Discussion
This study aimed to examine whether there have been changes in the mean utility values for COPD reported in the systematic review and meta-analysis conducted by Moayeri et al. [Citation7]. Moayeri et al. included 32 papers spanning from the respective dates of inception before July 2015, whereas the current study included 43 papers spanning over almost nine years thereafter. This indicates a considerable increase in research around health-related QoL of patients with COPD. Moreover, this study found a significantly higher mean utility value than the study conducted by Moayeri et al. [Citation7]. Several potential reasons for the difference observed in mean utility values in more recent studies may be worth noting, for instance, increased awareness and better adherence to treatment plans, early detection, advancements in medical treatments and interventions, thus improving the health-related QoL of patients with COPD [Citation61].
In line with the findings from Moayeri et al. [Citation7], this study also found significant heterogeneity in utility values among patients with COPD. To better understand this variability, the analysis included a set of predefined moderators covering the region, stage of COPD disease, method of measuring utility, and whether or not comorbidities are reported. These moderators were based on previous research on factors that might influence the magnitude of utility values [Citation13]. Age was not included as a potential modifier as the studies included in the current analysis are restricted to the adult population.
Whether or not comorbidities were reported did not exhibit a significant impact on the meta-analysed mean utilities despite previous research suggesting that comorbidities affect health-related QoL, particularly in chronic conditions [Citation62]. However, given that some studies did not provide data on comorbidities, it was not possible to determine whether they could be accurately categorised for an analysis based on actual comorbidities. Therefore, the scope of this meta-regression was limited to reported comorbidities rather than actual comorbidities. The finding that reported comorbidities were not a significant modifier of utility values suggests that consistency of comorbidity reporting across studies is important for future research on the impact of comorbidities on QoL.
The method of elicitation, region, and disease stage moderators had a significant impact on utility values. In this study, the meta-analysed mean utility value for EQ-5D-5L (0.676) was substantially lower than the mean utility value for EQ-5D-3L (0.780); and the uncertainty around the EQ-5D-5L estimate was smaller than that of EQ-5D-3L. This finding suggests that the increased sensitivity of EQ-5D-5L allows respondents to express health impacts that may not be adequately captured by the EQ-5D-3L questionnaire; and aligns with previous research on the comparative precision of EQ-5D-5L at both the individual and group level [Citation63]. It may be informative to compare studies using EQ-5D-5L with COPD-specific QoL measurement instruments, such as the St. George Respiratory Questionnaire, to gain further insights.
Despite the broad grouping of studies into continental regions, and the heterogeneity therein given cultural and economic disparities, regional differences were still found to have a significant impact on health-related QoL. Studies conducted in the UK report a mean utility value of 0.656 (95% CI: 0.565 to 0.747), compared to studies based in Asia, which report a mean utility value of 0.793 (95% CI: 0.758 to 0.828). The non-overlapping 95% confidence intervals indicate significant differences between the regions. This finding is consistent with prior literature, which reports that even after adjusting for demographic and clinical characteristics, Asian patients with hepatocellular carcinoma had significantly higher QoL scores than those of European patients [Citation64]. Though cultural values have been hypothesised to explain differences in utility values between geographic regions [Citation65], variation remains largely unexplained though generally substantial.
As utility values are intended to measure patient experience of disease, it is not surprising that the disease stage moderator had a significant impact on the results. The meta-analysed mean utility values for disease stages were 0.875, 0.849, 0.769, and 0.712 for mild, moderate, severe, and very severe, respectively, consistent with the recognised pattern of decreasing utility as disease severity increases. Importantly, the confidence intervals for mild and severe stages do not overlap, indicating a significant distinction between these disease stages. This finding underscores the impact of disease progression on utility values, a point also highlighted in a recent review of utility values for metastatic breast cancer [Citation13], which similarly identified disease progression as a key influencing factor.
The search strategy of this study was not restricted by disease stage or study type, allowing the capture of a wide range of studies. However, despite this broad approach, only five out of the 43 included studies (∼12%) were intervention studies, which predominantly focused on patients with moderate to severe disease. This disparity is likely due to the common practice of excluding patients with mild airflow limitation in intervention studies, which differs from the inclusion of these patients in other observational studies. This limitation is a crucial consideration for modellers who wish to utilise these results in economic evaluations of interventions, as it may impact the accuracy and generalisability of their findings.
In comparison with the findings from Moayeri et al. [Citation7], this study has some notable clinical and research implications. The current study reveals that the method of elicitation, disease stage, and region each had a significant effect on utility values. Notably, the EQ-5D-5L value had a lower point estimate and less uncertainty compared with EQ-5D-3L indicating improved sensitivity; more severe disease stages were associated with lower utility values; and Asian-based studies reported higher utility values than those with European and UK participants. Reporting of co-morbidities on the other hand, did not have a significant effect. These findings emphasise the importance of considering the approach used to identify utility values for parameterisation of health economic models. It is crucial for modellers to systematically examine the specific factors that may influence reported utility values. The results from this review can inform future cost-utility analyses evaluating the cost-effectiveness of interventions; however, given the heterogeneity between studies the different subgroup mean utility values, and the nuances resulting from study type differences, a meta-analysed utility value that combines mean utility values across all subgroups identified here should be approached with caution.
Author contributions
LO, SM, and NS developed the study concept and design. NS drafted the study protocol in conjunction with SM and LO. LA designed the search strategies and conducted the electronic searches. NS and YZ independently screened and appraised the relevant studies and extracted the data from the included studies; any disagreements were resolved by SM. LO, NS, and SM planned the analysis. NS conducted all statistical analyses and drafted the manuscript in conjunction with LO and SM. All authors commented on the draft and read and approved the final manuscript. The authors alone are responsible for the content and writing of the manuscript.
Disclosure statement
No potential conflict of interest was reported by the author(s).
Data availability statement
No additional data is available.
Additional information
Funding
References
- Overview | Chronic obstructive pulmonary disease in over 16s: diagnosis and management | Guidance | NICE [Internet]. NICE; 2018 [cited 2024 Apr 2]. Available from: https://www.nice.org.uk/guidance/ng115
- Wang L, Xie J, Hu Y, et al. Air pollution and risk of chronic obstructed pulmonary disease: the modifying effect of genetic susceptibility and lifestyle. EBioMedicine. 2022;79:103994. doi: 10.1016/j.ebiom.2022.103994.
- Risk factors | Background information | Chronic obstructive pulmonary disease | CKS | NICE [Internet]; 2024 [cited 2024 Apr 2]. Available from: https://cks.nice.org.uk/topics/chronic-obstructive-pulmonary-disease/background-information/risk-factors/
- Steiner MC, Lowe D, Beckford K, et al. Socioeconomic deprivation and the outcome of pulmonary rehabilitation in England and Wales. Thorax. 2017;72(6):530–537. doi: 10.1136/thoraxjnl-2016-209376.
- Ståhl E, Lindberg A, Jansson S-A, et al. Health-related quality of life is related to COPD disease severity. Health Qual Life Outcomes. 2005;3(1):56. doi: 10.1186/1477-7525-3-56.
- 7 Incorporating Economic Evaluation | Developing NICE guidelines: the manual | Guidance | NICE [Internet]. NICE; 2014 [cited 2024 Apr 2]. Available from: https://www.nice.org.uk/process/pmg20/chapter/incorporating-economic-evaluation
- Moayeri F, Hsueh YSA, Clarke P, et al. Health state utility value in chronic obstructive pulmonary disease (COPD); the challenge of heterogeneity: a systematic review and meta-analysis. COPD. 2016;13(3):380–398. doi: 10.3109/15412555.2015.1092953.
- Starkie HJ, Briggs AH, Chambers MG, et al. Predicting EQ-5D values using the SGRQ. Value Health. 2011;14(2):354–360. doi: 10.1016/j.jval.2010.09.011.
- 1 Introduction | Developing NICE guidelines: the manual | Guidance | NICE [Internet]. NICE; 2014 [cited 2024 Apr 2]. Available from: https://www.nice.org.uk/process/pmg20/chapter/introduction
- Foreword | Guide to the methods of technology appraisal 2013 | Guidance | NICE [Internet]. NICE; 2013 [cited 2024 Apr 2]. Available from: https://www.nice.org.uk/process/pmg9/chapter/foreword
- NICE [Internet]. Position statement on use of the EQ-5D-5L value set for England (updated October 2019) | Technology appraisal guidance | NICE guidance | Our programmes | What we do | About. NICE; 2024 [cited 2024 Apr 2]. Available from: https://www.nice.org.uk/about/what-we-do/our-programmes/nice-guidance/technology-appraisal-guidance/eq-5d-5l
- Peasgood T, Brazier J. Is meta-analysis for utility values appropriate given the potential impact different elicitation methods have on values? Pharmacoeconomics. 2015;33(11):1101–1105. doi: 10.1007/s40273-015-0310-y.
- Hao Y, Wolfram V, Cook J. A structured review of health utility measures and elicitation in advanced/metastatic breast cancer. Clinicoecon Outcomes Res. 2016;8:293–303. doi: 10.2147/CEOR.S100448.
- PRISMA [Internet]. [cited 2024 Apr 2]. Available from: http://prisma-statement.org/prismastatement/checklist.aspx?AspxAutoDetectCookieSupport=1
- 9.6.4 Meta-regression [Internet]. [cited 2024 Apr 2]. Available from: https://handbook-5-1.cochrane.org/chapter_9/9_6_4_meta_regression.htm
- Global Initiative for Chronic Obstructive Lung Disease-GOLD [Internet]. Global Initiative for Chronic Obstructive Lung Disease; 2024 [cited 2024 Apr 2]. Available from: https://goldcopd.org/
- Ahn JH, Chung JH, Shin K-C, et al. The effects of repeated inhaler device handling education in COPD patients: a prospective cohort study. Sci Rep. 2020;10(1):19676. doi: 10.1038/s41598-020-76961-y.
- Alcazar-Navarrete B, Fuster A, García Sidro P, et al. Relationship between clinical control, respiratory symptoms and quality of life for patients with COPD. Int J Chron Obstruct Pulmon Dis. 2020;15:2683–2693. doi: 10.2147/COPD.S265470.
- Altawalbeh SM, Almomani BA, Alefan Q, et al. The influence of adverse drug effects on health-related quality of life in chronic obstructive pulmonary disease patients. Int J Pharm Pract. 2022;30(5):457–465. doi: 10.1093/ijpp/riac052.
- Bae E, Choi SE, Lee H, et al. Validity of EQ-5D utility index and minimal clinically important difference estimation among patients with chronic obstructive pulmonary disease. BMC Pulm Med. 2020;20(1):73. doi: 10.1186/s12890-020-1116-z.
- Baltasar-Fernandez I, Losa-Reyna J, Carretero A, et al. Residual effects of 12 weeks of power-oriented resistance training plus high-intensity interval training on muscle dysfunction, systemic oxidative damage, and antioxidant capacity after 10 months of training cessation in older people with COPD. Scand J Med Sci Sports. 2023;33(9):1661–1676. doi: 10.1111/sms.14428.
- Boland MRS, van Boven JFM, Kruis AL, et al. Investigating the association between medication adherence and health-related quality of life in COPD: methodological challenges when using a proxy measure of adherence. Respir Med. 2016;110:34–45. doi: 10.1016/j.rmed.2015.11.008.
- van Boven JFM, Stuurman-Bieze AGG, Hiddink EG, et al. Effects of targeting disease and medication management interventions towards patients with COPD. Curr Med Res Opin. 2016;32(2):229–239. doi: 10.1185/03007995.2015.1110129.
- Burns DK, Wilson ECF, Browne P, et al. The cost effectiveness of maintenance schedules following pulmonary rehabilitation in patients with chronic obstructive pulmonary disease: an economic evaluation alongside a randomised controlled trial. Appl Health Econ Health Policy. 2016;14(1):105–115. doi: 10.1007/s40258-015-0199-9.
- Chen YT, Ying YH, Chang K, et al. Study of patients’ willingness to pay for a cure of chronic obstructive pulmonary disease in Taiwan. Int J Environ Res Public Health. 2016;13(3):273. doi: 10.3390/ijerph13030273.
- Choi JT, Seo JH, Ko MH, et al. Validation of Korean version of the London Chest Activity of Daily Living Scale in patients with chronic obstructive pulmonary disease. Ann Rehabil Med. 2018;42(2):329–335. doi: 10.5535/arm.2018.42.2.329.
- Ding B, Small M, Bergström G, et al. A cross-sectional survey of night-time symptoms and impact of sleep disturbance on symptoms and health status in patients with COPD. Int J Chron Obstruct Pulmon Dis. 2017;12:589–599. doi: 10.2147/COPD.S122485.
- Fishwick D, Lewis L, Darby A, et al. Determinants of health-related quality of life among residents with and without COPD in a historically industrialised area. Int Arch Occup Environ Health. 2015;88(6):799–805. doi: 10.1007/s00420-014-1008-8.
- Garcia-Gordillo MÁ, Collado-Mateo D, Olivares PR, et al. A cross-sectional assessment of health-related quality of life among patients with chronic obstructive pulmonary disease. Iran J Public Health. 2017;46(8):1046–1053.
- Hansen H, Beyer N, Frølich A, et al. Inter-day test-retest reproducibility of the CAT, CCQ, HADS and EQ-5D-3L in patients with severe and very severe COPD. Patient Relat Outcome Meas. 2021;12:117–128. doi: 10.2147/PROM.S306352.
- Hoogendoorn M, Jowett S, Dickens AP, et al. Performance of the EQ-5D-5L plus respiratory bolt-on in the Birmingham chronic obstructive pulmonary disease cohort study. Value Health. 2021;24(11):1667–1675. doi: 10.1016/j.jval.2021.05.006.
- Hoyle CK, Tabberer M, Brooks J. Mapping the COPD assessment test onto EQ-5D. Value Health. 2016;19(4):469–477. doi: 10.1016/j.jval.2016.01.005.
- Husain MO, Chaudhry IB, Blakemore A, et al. Prevalence of depression and anxiety in patients with chronic obstructive pulmonary disease and their association with psychosocial outcomes: a cross-sectional study from Pakistan. SAGE Open Med. 2021;9:20503121211032813. doi: 10.1177/20503121211032813.
- Jiménez-Reguera B, Maroto López E, Fitch S, et al. Development and preliminary evaluation of the effects of an mHealth web-based platform (HappyAir) on adherence to a maintenance program after pulmonary rehabilitation in patients with chronic obstructive pulmonary disease: randomized controlled trial. JMIR mHealth uHealth. 2020;8(7):e18465. doi: 10.2196/18465.
- Kokturk N, Polatli M, Oguzulgen IK, et al. Adherence to COPD treatment in Turkey and Saudi Arabia: results of the ADCARE study. Int J Chron Obstruct Pulmon Dis. 2018;13:1377–1388. doi: 10.2147/COPD.S150411.
- Koo HK, Kang HK, Song P, et al. Systemic white blood cell count as a biomarker associated with severity of chronic obstructive lung disease. Tuberc Respir Dis. 2017;80(3):304–310. Jul doi: 10.4046/trd.2017.80.3.304.
- Kwon HY, Kim E. Factors contributing to quality of life in COPD patients in South Korea. Int J Chron Obstruct Pulmon Dis. 2016;11:103–109. doi: 10.2147/COPD.S90566.
- Kwon YS. Moderating effect of depression on the relationship between subjective health and quality of life in patients with COPD. Ind J Publ Health Res Dev. 2018;9(11):787. doi: 10.5958/0976-5506.2018.01557.7.
- Lee SH, Kwon HY. Prevalence of osteoporosis in Korean patients with chronic obstructive pulmonary disease and their health-related quality of life according to the Korea National Health and Nutrition Examination Survey 2008–2011. J Bone Metab. 2017;24(4):241–248. doi: 10.11005/jbm.2017.24.4.241.
- Lee K, Lee H, Lee K, et al. Gender differences in pulmonary function, physical activity, and quality of life of patients with COPD based on data from the Korea National Health and Nutrition Examination Survey 2015 to 2019 from the perspective of pulmonary rehabilitation. Medicine. 2022;101(44):e31413. doi: 10.1097/MD.0000000000031413.
- Lim J, Choi SE, Bae E, et al. Mapping analysis to estimate EQ-5D utility values using the COPD assessment test in Korea. Health Qual Life Outcomes. 2019;17(1):97. doi: 10.1186/s12955-019-1148-3.
- Merino M, Villoro R, Hidalgo-Vega Á, et al. Health-related quality of life of patients diagnosed with COPD in Extremadura, Spain: results from an observational study. Health Qual Life Outcomes. 2019;17(1):189. doi: 10.1186/s12955-019-1244-4.
- Miravitlles M, Barrecheguren M, Román-Rodríguez M. Frequency and characteristics of different clinical phenotypes of chronic obstructive pulmonary disease. Int J Tuberc Lung Dis. 2015;19(8):992–998. doi: 10.5588/ijtld.15.0021.
- Nguyen TS, Nguyen TLH, Pham TTV, et al. Impact of pharmaceutical care in the improvement of medication adherence and quality of life for COPD patients in Vietnam. Respir Med. 2019;153:31–37. doi: 10.1016/j.rmed.2019.05.006.
- Nolan CM, Longworth L, Lord J, et al. The EQ-5D-5L health status questionnaire in COPD: validity, responsiveness and minimum important difference. Thorax. 2016;71(6):493–500. doi: 10.1136/thoraxjnl-2015-207782.
- Paly VF, Naya I, Gunsoy NB, et al. Long-term cost and utility consequences of short-term clinically important deterioration in patients with chronic obstructive pulmonary disease: results from the TORCH study. Int J Chron Obstruct Pulmon Dis. 2019;14:939–951. doi: 10.2147/COPD.S188898.
- Richeldi L, Schino P, Bargagli E, et al. TRITRIAL: the impact of fixed triple therapy with beclometasone/formoterol/glycopyrronium on health status and adherence in chronic obstructive pulmonary disease in an Italian context of real life. Int J Chron Obstruct Pulmon Dis. 2024;19:475–487. doi: 10.2147/COPD.S445858.
- Martinez Rivera C, Costan Galicia J, Alcázar Navarrete B, et al. Factors associated with depression in COPD: a multicenter study. Lung. 2016;194(3):335–343. Jun doi: 10.1007/s00408-016-9862-7.
- Roncero C, Campuzano AI, Quintano JA, et al. Cognitive status among patients with chronic obstructive pulmonary disease. Int J Chron Obstruct Pulmon Dis. 2016;11:543–551. doi: 10.2147/COPD.S100850.
- Sohanpal R, Pinnock H, Steed L, et al. A tailored psychological intervention for anxiety and depression management in people with chronic obstructive pulmonary disease: TANDEM RCT and process evaluation. Health Technol Assess. 2024;28(1):1–129. doi: 10.3310/PAWA7221.
- Sundh J, Johansson G, Larsson K, et al. Comorbidity and health-related quality of life in patients with severe chronic obstructive pulmonary disease attending Swedish secondary care units. Int J Chron Obstruct Pulmon Dis. 2015;10:173–183. doi: 10.2147/COPD.S74645.
- Talboom-Kamp EP, Verdijk NA, Kasteleyn MJ, et al. High level of integration in integrated disease management leads to higher usage in the e-vita study: self-management of chronic obstructive pulmonary disease with web-based platforms in a parallel cohort design. J Med Internet Res. 2017;19(5):e185. doi: 10.2196/jmir.7037.
- Thuppal S, Markwell S, Crabtree T, et al. Comparison between the EQ-5D-3L and the SF-6D quality of life (QOL) questionnaires in patients with chronic obstructive pulmonary disease (COPD) undergoing lung volume reduction surgery (LVRS). Qual Life Res. 2019;28(7):1885–1892. doi: 10.1007/s11136-019-02123-x.
- Verberkt CA, van den Beuken-van Everdingen MHJ, Dirksen CD, et al. Cost-effectiveness of sustained-release morphine for refractory breathlessness in COPD: a randomized clinical trial. Respir Med. 2021;179:106330. doi: 10.1016/j.rmed.2021.106330.
- Wacker ME, Jörres RA, Karch A, et al. Assessing health-related quality of life in COPD: comparing generic and disease-specific instruments with focus on comorbidities. BMC Pulm Med. 2016;16(1):70. doi: 10.1186/s12890-016-0238-9.
- Wacker ME, Jörres RA, Karch A, et al. Relative impact of COPD and comorbidities on generic health-related quality of life: a pooled analysis of the COSYCONET patient cohort and control subjects from the KORA and SHIP studies. Respir Res. 2016;17(1):81. doi: 10.1186/s12931-016-0401-0.
- Wu M, Zhao Q, Chen Y, et al. Quality of life and its association with direct medical costs for COPD in urban China. Health Qual Life Outcomes. 2015;13(1):57. doi: 10.1186/s12955-015-0241-5.
- Xiao T, Wu K, Chen Y, et al. Quality of life and its associated factors for mild chronic obstructive pulmonary disease patients of urban community settings. Ann Palliat Med. 2020;9(4):1420–1430. doi: 10.21037/apm-19-655.
- Zanforlini BM, Ceolin C, Trevisan C, et al. Clinical trial on the effects of oral magnesium supplementation in stable-phase COPD patients. Aging Clin Exp Res. 2022;34(1):167–174. doi: 10.1007/s40520-021-01921-z.
- Page MJ, Sterne JAC, Higgins JPT, et al. Investigating and dealing with publication bias and other reporting biases in meta-analyses of health research: a review. Res Synth Methods. 2021;12(2):248–259. doi: 10.1002/jrsm.1468.
- Vanfleteren LEGW, Ullman A, Fabbri LM. Time for a longer and better life for patients with COPD. Eur Respir J. 2018;51(1):1702569. Available from: https://erj.ersjournals.com/content/51/1/1702569
- Heyworth IT, Hazell ML, Linehan MF, et al. How do common chronic conditions affect health-related quality of life? Br J Gen Pract. 2009;59(568):e353–e358. doi: 10.3399/bjgp09X453990.
- Janssen MF, Bonsel GJ, Luo N. Is EQ-5D-5L better than EQ-5D-3L? A head-to-head comparison of descriptive systems and value sets from seven countries. Pharmacoeconomics. 2018;36(6):675–697. doi: 10.1007/s40273-018-0623-8.
- Chie WC, Blazeby JM, Hsiao CF, et al. Differences in health-related quality of life between European and Asian patients with hepatocellular carcinoma. Asia Pac J Clin Oncol. 2017;13(5):e304–e311.
- Roudijk B, Donders ART, Stalmeier PFM, et al. Cultural values: can they explain differences in health utilities between countries? Med Decis Making. 2019;39(5):605–616. Jul doi: 10.1177/0272989X19841587.