Abstract
Geospatial data play an increasingly important role in natural resources management, conservation, and science-based projects. The management and effective use of spatial data becomes significantly more complex when the efforts involve a myriad of landscape-scale projects combined with a multiorganizational collaboration. There is sparse literature to guide users on this daunting subject; therefore, we present a framework of considerations for working with geospatial data that will provide direction to data stewards, scientists, collaborators, and managers for developing geospatial management plans. The concepts we present apply to a variety of geospatial programs or projects, which we describe as a “scalable framework” of processes for integrating geospatial efforts with management, science, and conservation initiatives. Our framework includes five tenets of geospatial data management: (1) the importance of investing in data management and standardization, (2) the scalability of content/efforts addressed in geospatial management plans, (3) the lifecycle of a geospatial effort, (4) a framework for the integration of geographic information systems (GIS) in a landscape-scale conservation or management program, and (5) the major geospatial considerations prior to data acquisition. We conclude with a discussion of future considerations and challenges.
INTRODUCTION
Geospatial data form the foundation of many resource management and science activities, particularly when working at a landscape scale. We use the term geospatial to refer to geographic information systems/sciences, remote sensing, and any other process related to describing and analyzing information associated with a defined area on the ground. We define a landscape-scale program as one that spans more than one jurisdictional unit of the organizations involved, for example, multiple states, regional offices, and districts. Land managers rely on scientific decision-making through the development of management plans and adaptive management strategies. In turn, scientists provide managers with resource information at the species landscape and ecosystem scales. Scientists and managers alike depend on geospatial data that serve as inputs for decision-making and analysis, and these tools help connect otherwise disparate information. Thus, a comprehensive and thoroughly planned framework that encompasses the management, integration, archiving, and distribution of geospatial data will improve the success for land managers, conservationists, and scientists during and beyond a project's life cycle.
Data management requirements vary considerably among projects including the project objectives, spatiotemporal extent, volume of data generated, and the number of collaborators (Michener Citation1997). Therefore, the amount of planning required for a particular program or project is reflective of the multitude of factors involved. We define a multiorganizational program as any project involving two or more parties with diverse objectives that represent federal, state, or local jurisdictions and nongovernmental organizations working together on a common program directive. Organizations may include academic, government, nongovernment, commercial, and nonprofit institutions. As noted above, a landscape-scale program spans more than one jurisdictional unit of the organizations involved. This distinction is critical given geospatial management considerations because many data sets do not overlap jurisdictional boundaries or GIS analysts develop similar data sets across noncongruent boundaries but using different standards and approaches. Examples of multiorganizational, landscape-scale programs in the United States include the Wyoming Landscape Conservation Initiative (http://www.wlci.gov), landscape conservation cooperatives (http://www.fws.gov/landscape-conservation/lcc.html), rapid ecoregional assessments (http://www.blm.gov/wo/st/en/prog/more/Landscape_Approach/reas.html), and environmental monitoring and assessment programs (http://www.epa.gov/emap/).
There are many choices for managing geospatial data in both small and large projects. The information described within this article will assist individuals and groups alike to make those decisions needed for a successful project. Because large collaborative programs involve many data developers and data users, a standard protocol for handling geospatial data will establish consistency in data and thereby improve data usability. With an increase in the number of participants, communication is integral to the success of any large-scale geospatial program. The larger the program, the greater the level of effort required to maintain active communication between parties. Therefore, program coordinators should include mechanisms for disseminating information between all parties by communicating standards, data procurement efforts, management plans, and implementation plans. Through proper planning, collaborators can structure management plans to evolve and encompass addendums. Project milestones provide an opportunity to evaluate successes and future hurdles to ensure the management plan is effectively meeting program objectives. Some of the components discussed in this article may not pertain to all programs, but part of developing a management plan is considering many geospatial components before deciding what is, or is not, relevant. We intend our approach to serve as a reference that will help guide geospatial data managers and program coordinators through all phases, from initial planning through data storage after a program's conclusion.
Currently, a comprehensive geospatial guide for multiorganizational, landscape-scale programs does not exist. However, there have been many advances in various aspects of geospatial data management in recent years, most notably in regard to initial project planning and distribution of the data. The UK Data Archive (http://www.data-archive.ac.uk/home) has developed a thorough guide to assist researchers in managing and sharing data (Van den Eynden et al. Citation2011). We encourage our audience to review this work. However, the aim of the UK Data Archive is to identify best management practices for individual researchers. A central theme of this paper is to provide a framework that meets the needs of a multiorganizational, landscape-scale program. In the 1990s, the ecological sciences recognized the burgeoning role of geospatial information in the field. Calls for the creation of data management plans that extend far beyond basic metadata began to appear in the literature. Acknowledgment of the complexity of projects with multiple players (Michener Citation1997) and even the need for metadata of nongeospatial data was recognized (Michener et al. Citation1997). William Michener, currently the director of e-Science Initiatives for University Libraries at the University of New Mexico, is responsible for much of this discussion. Recently, Michener and others put forth information management guidance for the U.S. Long-Term Ecological Research Program (Michener et al. Citation2011).
The long-term success of large-scale projects increases if data are readily accessible from geospatial libraries, information centers, or spatial data clearinghouses. To make this a reality, program coordinators should adequately plan, communicate, and successfully execute data management procedures in the early stages of any project. Proper data management and subsequent data sharing enhances the scientific process because it generates high quality data (Van den Eynden et al. Citation2011). Easily accessible data allow verification of results and facilitates new research to build on reliable, existing information. Given that many research projects are publicly funded, robust data management allows realization of the full potential of public investments.
Volunteered geographic information (VGI) and public participation GIS (PPGIS) describe crowdsourced mapping initiatives (e.g., OpenStreetMap) that reflect user-generated spatial data by nonauthoritative geographic individuals (Goodchild Citation2007). Neogeography refers to newer information technologies that impact mapmaking activities such as Google Maps or similar application programming interfaces (APIs) (Turner 2006, 2007; Elwood Citation2008), whereas VGI pertains to how data are collected and disseminated (Goodchild Citation2008). VGI data are considered complementary to program/project data collected by organizations such as the government (Goodchild and Glennon Citation2010) because VGI data are from the users’ perspectives and, although valuable, this perspective may not reflect the objectives of a program/project. The Geospatial Web, also known as GeoWeb, is a network that combines geographic data (e.g., VGI or other spatial data) with common information (e.g., nonspatial information common on the Internet). The use of the GeoWeb for research applications varies (Elwood 2008). A few examples include the examination of mobility patterns of people and public health responses to pandemics (Guo Citation2007), the identification of focus areas where large sources of georeferenced images exist (Currid and Williams Citation2009), and disaster/crisis management (Roche, Propeck-Zimmermann, and Mericskay Citation2011; Goodchild and Glennon Citation2010). GeoWeb and VGI data are relevant to government efforts and programs/project efforts with collaborators. Sharing information such as locations of studies and disseminating this information via the GeoWeb is one example. While developing a management plan, participants should consider the benefits of VGI and GeoWeb contributions to the project/program efforts because these applications can carry significant benefits for many reasons, as demonstrated for social science research and crisis management cases.
The objective of this article is to review the available information regarding geospatial considerations and provide a synthesis of these materials to serve as a framework for multiorganizational, landscape-scale programs. Specifically, our objectives include (1) describing the importance of data management and standardization, (2) discussing how varying levels of geospatial efforts lead to a scalable approach for management, (3) discussing the life cycle of a geospatial management plan, (4) identifying a stepwise framework for integrating GIS into a landscape-scale program, and (5) discussing the major geospatial considerations prior to data acquisition.
Importance of Data Management and Standardization
Planning becomes increasingly important to data usability with an increased need for GIS support. Business decisions, products, services, scientific reports, and policies are a few examples that stipulate reliable and accurate data creation and maintenance. Industries may not adopt geospatial standards for numerous reasons:
Many data products exist; therefore, data stewards do not unanimously adopt a single format.
Different requirements exist for different uses of data.
Geomatic communities, scientific communities that collect and analyze data relating to the earth's surface, include a wide variety of government (e.g., federal, state, and local), nongovernment, nonprofit, commercial, and educational organizations in which many have adopted their own standards.
The diversity and complexity of geomatics leads to difficulties in adopting standards that are broad enough, but also detailed enough to establish consistent protocols.
Rapid changes and advances in technology make it difficult to maintain enduring standards.
Programs can fail to reach their goals by not establishing and adhering to data management practices, and mismanagement of data can lead to significant loss of information. Alternately, programs can benefit from establishing good data management practices and standards for many reasons. For example, these resources can improve upon informed decision-making processes and reduce communication breakdowns by establishing data custodians, prioritizing data collection, and minimizing duplicative efforts. Effective management plans and standards will also enhance security practices, increase data value via standardizing data lifecycle frameworks, reduce costs, and increase efficiency, which can support new business opportunities.
Partners often recognize the importance of standardization and data management; however, the lack of a concise, cohesive, and recognized geospatial management plan can limit the success of a geospatial program. Therefore, the success of a program will largely depend on the development of a geospatial management plan wherein all parties actively participate in its development and implementation.
There is often ambiguity about data formats, data accuracies, coordinate systems, and geospatial concepts for both GIS and non-GIS users. Many types of scientists, natural resource staff, and other professionals do not have the same level of training and knowledge of geospatial topics. Therefore, establishing standards, providing education, and communicating with partners are important and cost-effective factors. Standards, in particular, are important because they promote consistency and clarity throughout data. This increases the usability of the data while making efficient use of limited resources. In the past decade, GIS has increasingly played important roles in litigation (Dischinger and Wallace Citation2005; Cho Citation2005; Bowles Citation2002; Onsrud Citation1992; Center for Spatial Law and Policy [http://www.spatiallaw.com]; Open Geospatial Consortium [OGC] Spatial Law and Policy Committee [http://www.opengeospatial.org/ogc/organization/bod/slpc]. Consequently, concise and standardized protocols are relevant for minimizing data errors; documenting appropriate data uses such as scales, resolutions, and minimum mapping units, culminating in scientifically supported management decisions.
Many online data sets require users to comply with a user agreement. For example, OpenStreetMap relied on “copyleft” licenses for data released before September 12, 2012, and OpenDatabase License (ODbL) for data released thereafter (OpenStreetMap 2014). As a result, the use of these data can affect a program's objectives. OpenStreetMap data were using U.S. government data, but the government requires that these data not be used outside the United States. Therefore, programs/projects and their understanding of data use is important to establish within management plans. Many variations of data licenses exist and, as a result, many potential legal issues surround the use of data, including VGI data (Saunders, Scassa, and Lauriault Citation2012; Scassa 2013). As but one example, legal issues may affect the host of a VGI site, the contributors to VGI, the user accessing the data, and the developers’ contributions. Geospatial programs and the use of spatial data should require familiarity and consideration of the potential legal ramifications for data (Scassa 2013).
Scalability and Geospatial Management Plans
Developing a management plan for integrating geospatial technologies is a scalable effort. For example, the efforts associated with data management, resource availability, and data sharing greatly increase if a program has the capacity to expand. An expansion in the program will affect the spatial and temporal footprint, the life span of program relevance, and the number of collaborators involved. These three types of scalability (spatial, temporal, and collaborative) are the core components typically associated with large, multiorganizational, landscape-scale, and long-term monitoring programs.
Spatial scalability refers to the ability to characterize small projects with a small area of interest and to large programs with multiple studies dispersed over large spatial extents. A large extent increases the amount of effort required to collect, create, manage, disseminate, and develop data and metadata. As the amount of data increases, personnel, hardware, and software infrastructure requirements also escalate. Understanding the amount of data as well as the scale and resolution for which the data are collected will help determine the complexity of managing the data.
Temporal scalability can refer to assessing changes in spatial information (i.e., monitoring), available capital (e.g., monetary resources, hardware, and software resources), human resources, program objectives, or changes in accord between partners. The complexity of temporal data affects their utilization and integration because data scales and resolutions often differ between historic, present, and future conditions due to changes in technologies; in other words, improved technologies lead to increased spatial or temporal resolution. Such differences not only add complexity to meeting stated requirements and suitable use of the data for program efforts, but they can also increase efforts for incorporating the data because the data include a variety of accuracies and thus appropriate uses.
Collaborative scalability refers to the additional complexity a program might encounter when there are numerous partners involved. A small GIS lab is vastly different from a multiorganizational program with many GIS personnel dispersed over a large geographic region. Although requirements for managing data do not change, implementation aspects will. Organizations differ as to how they track, manage, and disseminate information to the public and internal constituents. If one considers three or more organizations with different methods of working with geospatial data, it is easy to understand the complexity as well as the necessity of establishing how management plans will address data integration. It is unlikely that there will be full agreement on how to implement such an effort, but it is critical to develop a robust management plan that can handle different scenarios and accommodate all partners.
Life Cycle of a Geospatial Effort
Management plans often incorporate broad categories of project, data, and infrastructure life cycles. Each category requires further consideration of stages that define the life cycle. For example, data life cycles include defining data requirements, inventory, procurement, access, maintenance, evaluation, and archiving (Office of Management and Budget Citation2010). Identifying the life cycle stages is important for minimizing errors. Kervin et al. (Citation2013) reviewed data and metadata errors identified by peer reviewers of data papers published in the Ecological Society of America's Ecological Archives. They identified numerous categories of error at each life stage, which provides useful insight into where improvements in data collection and management are necessary. For example, they reported on publications between 2004 and 2012. On average, each data paper contained 20.3 errors; 92.5% resulted in errors associated with collection and organization, 96.2% contained description errors, and 52.8% contained quality assurance and quality control errors.
Including information about the various types of life cycles within a management plan can have many benefits. However, such efforts require a thorough understanding of the objectives as well as expert knowledge of how these life cycles affect long-term data management and future implementation. Some of these life cycles can include changes in objectives, data maintenance schedules, human resources, monetary resources, and accords between partners. Although some of these conditions will not apply to all programs, a management plan will be more successful if it can address how geospatial efforts should adapt to changing needs. For example, managers could design system architecture protocols to incorporate additional data, to handle versioning of data (i.e., multiple users editing the same large data set), or adapt to an increased number of users requiring access to data (database/Web resource loading). With insight into the life span and efforts of a program, coordinators can anticipate and effectively scale data management efforts without sacrificing the program's objectives. This is important because technical solutions are typically more effective when they are anticipated instead of being tackled retroactively.
Framework for Integrating GIS into a Landscape-Scale Program
We introduce ten major steps for developing a management plan (). The three phases of the ten-step process include evaluating geospatial needs, developing data management protocols, and communicating and coordinating the implementation of the plan. Every program is different and there is no single model that will fit all geospatial management needs. Therefore, implementing some or all of the outlined steps is program dependent, but reviewing these topics will help programs identify what may require implementation. Management plans should avoid time-sensitive standards, software-specific standards, and other factors that may become obsolete.
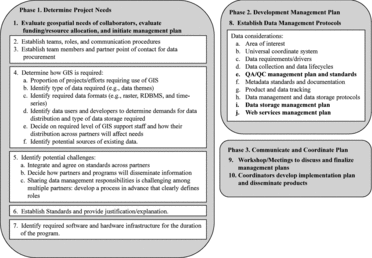
A detailed description of the 10-step process is provided (Appendix A); we intend this as a guideline and reference to facilitate the process of developing detailed management plans rather than a strict rule-set. Our objective is to facilitate considerations and discussions within the project-planning context, regarding the many factors that play important roles in geospatial content. In addition to this framework, we rely on the remaining portion of this article to expand on several topics that provide concepts specifically related to incorporating the use of geospatial data and the significance of establishing protocols within a management plan.
Major Geospatial Considerations Prior to Data Acquisition
Large, multiorganizational programs are difficult to organize, given the different needs of different partners and standard operating procedures (SOPs) that are likely already in place for various partners but which may not be fully compatible. Therefore, associating and distinguishing the various facets of partners’ geospatial requirements is important to outline during initial efforts. We describe a workflow to highlight this process, encompassing data mining, data tracking and maintenance, and data documentation (). Once partners identify their needs, a focus group (large or small) can discuss, dissect, and manipulate the framework in a constructive fashion to determine the final management plan. The following sections examine some of the major geospatial components associated with starting a multiorganizational effort.
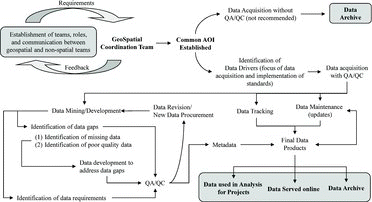
Implications of Identifying a Common Area of Interest
Area of Interest
It is critical to define an area of interest (AOI) for a project because it establishes the spatial extent for which data are collected. Before proceeding with a description of AOIs, it is necessary to understand a couple of terms used hereafter. The study area refers to the extent of a specific study or project, whereas the program AOI refers to an equal or larger extent than that of a single study area, such as where multiple studies exist. Selecting an AOI () is more complex than simply using the extent of the combined study areas, and data experts, scientists and managers, and coordination teams should consider project objectives as well as other factors that affect analyses to avoid undesirable “edge effects,” for example.
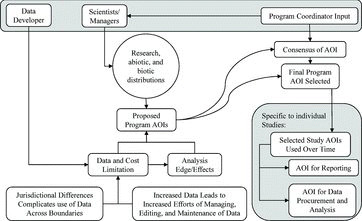
At the outset, project teams should assess data requirements, determine whether the data already exist at relevant and compatible scales and accuracies, and ascertain whether the planned analysis is feasible for the program AOI. Because of different requirements for different projects, selecting a single AOI is not always realistic. Study participants should determine their needs and recognize the limitations and efforts in collecting data from various sources and extents. Program managers should consider several questions when selecting an appropriate program AOI. Does the AOI capture the biotic and abiotic information in surrounding areas that might affect the study results? Does the AOI capture potential data interactions of biotic and abiotic variables? Are there additional costs of collecting data with the proposed AOI and are there alternatives? Does the AOI reasonably represent the data (grain and extent) required for meaningful spatial analysis? Establishing research needs, identifying spatial congruence of data, and understanding how a defined AOI can affect analyses is important for researchers’ knowledge, data management, and development of management plans.
Another factor to consider when selecting an appropriate program AOI is how the selected AOI will affect various types of analysis. If analysis requires information beyond a spatial extent of the study area, then it is necessary to have data extending beyond the study area in order to analyze what lies within the study area without edge effects. For example, a moving window that represents the extent to which the features are measured will not include accurate summarization of some features when the window—a common GIS process for landscape-scale projects—overlaps an artificial boundary (i.e., the study area). Therefore, if no data exist beyond the study area, there will be an edge effect due to false zeroes outside the AOI, resulting in inaccurate results along edges (). The concerns related to using congruent (identical) AOI boundaries (i.e., deliverable and analysis AOIs) are important to understand for the reasons above.
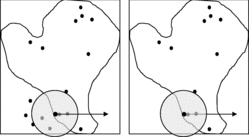
A common scenario is to identify the program AOI and then buffer a watershed or ecoregional data set intersecting the AOI. Data procurement occurs at the expanded program AOI, but collaborators report results within the program AOI. This process results in a biologically meaningful boundary (intersected boundary) and reduces edge effects (buffered boundary).
Define a Universal Coordinate System
Under most circumstances spatial data compilers will encounter a variety of map projections associated with data originating from a disparate group of data sources. Ascertaining an appropriate map projection is an important facet of starting any project, and a management plan should specify a standard map projection to facilitate use of data for all partners. This is likely a straightforward exercise for a small project, but difficult for a large AOI. Managers are required to investigate several questions when considering how to select an appropriate map projection. What is the size of the program AOI (e.g., global, continental, multistate, single state)? What distortions (e.g., area, shape, angle, and distance) are permissible? Which map projection will best capture the AOI while minimizing distortion? How will the map projection affect analytical results for both vector and raster data sets? Although we minimize our discussion of how reprojecting vector and raster data (Steinwand et al. Citation1995) affects data products, some cognition of these effects is necessary. Projecting vector data is a transformation of coordinates between two reference systems in which the coordinates are represented as a continuous scale, and features within the data are adjusted according to the defined relationship. One can assess map projection distortions using Tissot's indicatrix (Feeman Citation2002), but this index does not reflect how reprojecting vector and raster data can introduce or propagate additional errors. For example, the number of vertices making up lines and polygons will affect the magnitude of error, which this index does not capture. Because we are unable to identify any references on the effects of reprojecting vector data, we provide an example of reprojecting two data sets representing a single line stretched across the United States. One data set has no vertices along the arc and two end points, and the second data set has many vertices in addition to the two end points ().
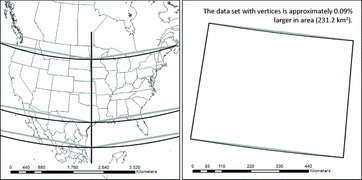
Additional considerations include the type of analysis one can use with a given map projection. For example, GIS analysts use an equidistant map projection when measuring distances and use an equal area map projection when measuring areas. Data managers should decide which map projections introduce the least amount of error and whether modifications (e.g., increasing vertices) to the data are necessary.
The effects of projecting raster data is complex and requires extensive knowledge of the data and projection properties. For example, projecting raster data sets can change a data set's composition (i.e., proportions of class values) and structure (i.e., spatial autocorrelation). Numerous methods exist for quantifying errors associated with projecting raster data. We encourage our audience to review the literature that discusses methods of quantifying changes of raster data properties during reprojection, such as window-based counting (Steinwand et al. Citation1995; Mulcahy Citation2000; Kimerling Citation2002), pixel-based counting (White Citation2006), global counting (Seong Citation2003), the scale factor model (Seong and Usery Citation2001), and random points (Seong Citation2005). These authors suggest that reprojecting raster data can introduce errors; therefore, data developers and scientists should not reproject raster data without understanding and quantifying the potential ramifications. One alternative that researchers can use is to perform analyses using the native map projection (a geographic coordinate system requires reprojection because it does not preserve distance and area across space) and then reproject only the results to the universal map projection specified in the management plan.
Data Requirements/Drivers
Base data sets are important to any geospatial program because they provide uniformity for all partners in the program and serve as the building blocks of a GIS, including general data layers for maps, content for presentations, and preliminary analysis. Data drivers are data sets required for a program that do not readily exist as available data products, yet many projects require these data; therefore, they will “drive” a significant portion of all analyses. Scientists should identify data drivers and their characteristics (e.g., scale and attribution) as important data sets that will contribute to the success of a program. For example, many individuals may identify road data sets as a base data set. However, road data that include attributes such as surface type, road width, and road condition mapped at a scale of 1:12,000 might be necessary for the studies occurring within the program AOI, yet such data are usually not readily available; therefore, they can be considered data drivers.
Many benefits exist in identifying data drivers. Spending time on identifying the criteria for selecting such data is critical to the success of projects, especially those with long-term monitoring programs. Focusing quality control efforts on the most highly demanded data could reduce the cost of data development, and importantly, increase the efficiency of inter- or intra-agency effort. All partners should form a consensus for identifying data drivers (), and one approach is to survey (Web-based is probably the most effective method) collaborators and determine which data sets they require. Once surveys are complete, program coordinators can summarize and circulate a synopsis of data requirements, priorities, and relevant information. Workshops can also facilitate efforts to establish the selection and prioritization of data drivers, but this approach is less effective as the scope of the program efforts increases.
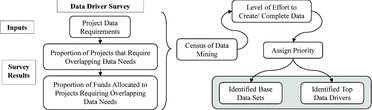
Data Collection and Data Life cycles: Ownership, Custodianship, and Currency
Although data collection seems an inherently simple task, this becomes less true when programs involve numerous partners. Several factors influence the usability of data, therefore they affect how data are collected. The format of data can dictate their use. Vector data, for example, can use multipart features (e.g., multiple polygons define a single database record), but multipart features can limit their use if information for individual polygons or information underlining each polygon is required for analysis. Some raster formats, such as NetCDF climate data, rely on a z-axis for temporal information, but many GIS and remote-sensing software applications cannot use this format for subsequent analysis. Therefore, disseminating data in these formats is not usable for many organizations or for analytics in GeoWeb applications. If the collection of spatial and aspatial information within a data set is incomplete, errors can be introduced in arriving at accurate conclusions, especially when the level of completeness varies spatially. Data scale, resolution, and horizontal positional accuracy influence how users can appropriately evaluate the information. For example, transportation data mapped at 1:100,000 scale (U.S. Census Tiger data) exclude a significant portion of transportation features, which influences the type of analysis and interpretation of biological interactions inferred from the data.
Raster data sets, such as the Gap Analysis Program, Regional Gap Analysis Program, and Landfire, may have a 30-m spatial resolution, but they are not intended for evaluating information at the pixel level (introduces effects of the modified areal unit problem). Differences of standards applied across boundaries and differences between data sets lead to usability difficulties for regional analysis or analyses that overlap multiple administrative boundaries because of mismatched attributes, data scales, and collected information. In addition to the aforementioned issues, many hurdles exist during the process of collecting data. These issues often arise due to lack of communication, changes in staff, procedures to procure data, and methods of disseminating data. Here are some suggestions to avoid these pitfalls:
Identify key personnel associated with each agency as data procurement nodes.
Identify the types of data that are applicable to program objectives.
Identify key data drivers (explained in Data Requirements/Drivers).
Organize data procurement efforts and establish standards for storing data and establish data quality and data control processes to employ once data are obtained.
Address the topics related to data management (explained in Major Geospatial Considerations Prior to Data Acquisition).
Collecting enormous amounts of data is a time-consuming and costly process. Before collecting data, managers should make decisions on how to manage data with regard to the data's life cycle (). Most programs have a finite life span; therefore, program managers should consider the owners and custodians of the data during and after the program's life span. Data managed properly will have a life beyond the current program, so they can benefit future projects. The data life cycle and business model (geospatial plan) rely on feedbacks from users and managers to enhance usability. Therefore, a management plan should consider the types of data users, user needs, research needs, data drivers, and the other tenants outlined in this article. The data life cycle describes data discovery, collection and development of data, inventory and evaluation of data, protocols for assessing and disseminating data, maintenance of data, and data archiving, as well as information flow between users, components of the business model, and management of data and their life cycle.
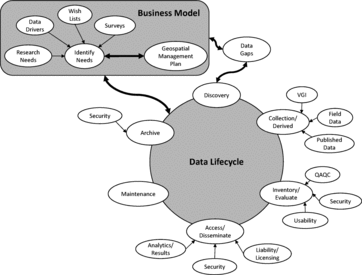
Data Quality Assurance and Quality Control
Data collection is subject to resource limitations, which can affect the quality of spatial information (Li, Zhang, and Wu 2012). The purpose of quality assurance (QA) and quality control (QC) is to minimize error and ensure that scientists and land managers understand the accuracy of the data, thus have confidence in their analytical results and subsequent decisions. QA is the establishment of standards and procedures to ensure data continuity between collaborators. QC is the process of maintaining standards by testing products against the established standards. Data quality is often difficult, time consuming, and expensive to quantify, particularly when data stewards collect information from various sources without accompanying metadata. Data with errors propagate with each additional processing step; therefore, results will contain greater error with each additional analysis. Establishing a standard protocol for evaluating data allows users to know what errors and limitations to expect during analysis ().
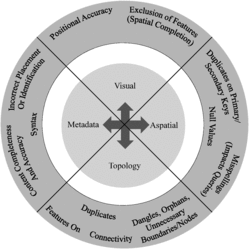
One of the biggest concerns facing a new program is the misconception of what data exist, completeness of the data, and data accuracy. Often participants think data products exist, but upon investigation limitations in extent, resolution, or content preclude use and application for a new purpose. Furthermore, data attributes may lack definitions, or aspatial fields may be incomplete or missing; thus, exploration of data sets is required to understand the accuracy and value of each data set for the intended use. Many issues related to data quality exist:
Lack of documentation for data or attributes leads to gaps of information for all users, preventing appropriate use of the data or an inability to use the data for meaningful applications.
Incomplete attribution (i.e., blank fields and missing data) can result in users questioning the completeness or accuracy of the data. Although the spatial information associated with geographic information data is critical, without attributes the data set in many cases is unusable.
Unknown positional accuracy results in users guessing the appropriate use of a data set. For example, if a user is evaluating soil attributes and the scale of the data is unknown, appropriate analysis decisions (e.g., construction viability versus regional assessment of water availability capacity) or management decisions (e.g., constructing a building on unstable soils) become questionable.
Lack of completeness of spatial features for specified scale representation is important, yet difficult to identify without appropriate documentation. For example, if one is evaluating a transportation data set digitized at a scale of 1:100,000, one can expect the data will capture only well-graded or paved roads that support heavy volumes. Another example is using raster products of 30-m resolution supporting a 1:100,000 scale. If users assume the accuracy to be 30 m, they are likely to encounter the modified areal unit problem (Jelinski and Wu Citation1996) and thus misinterpret the data used for analysis or for making management decisions.
Lack of topology can result in problems during spatial analysis such as calculating areas and perimeters. Requiring and documenting topology rules increases acceptance of the data characteristics and provides quality control for overall data accuracy. For example, polygons may overlap, but if documentation does not state overlapping polygons is allowed, this omission affects the user's perception of data quality and hinders accurate area calculations because overlapping areas are summed more than once.
Logical consistency: the structural integrity of a data set such as inclusion of appropriate vertices/nodes (i.e., road networks, direction of arcs).
Semantic accuracy: whether diction errors occur (e.g., grasslands may mean something different to an ecologist versus a rancher).
At the beginning of any program or project, managers can establish the standards for QA/QC, but those responsible for implementing these standards may require altering or enhancing them for workflow purposes. However, QA/QC procedures are expensive, so if resources are limited, we suggest implementing a strict QA/QC plan for the most widely used and important data sets (i.e., data drivers) and direct surplus resources to assess other data sets. The SOP produced by the U.S. Environmental Protection Agency (EPA; EPA 2003) for implementing a quality control and quality assurance process is an excellent example and template. This document provides important information on developing QA management plans and recommendations on how to approach such endeavors while working with geospatial data. In tandem with the EPA's SOP, we propose the use of a “data usability model” that provides an overall score, or report card, based on data attribute categories () to help guide end-users. A data usability model can provide a broad, level assessment of data quality with minimal effort, which will establish a quick assessment of data that coordination teams can use to prioritize needs for more rigorous QA/QC evaluation. Identifying the usability of geographic data is important but also complex. Brown et al. (Citation2013) explore these points by first identifying two categories of stakeholders, professional GIS and VGI, and then they identify the users for these two groups. Professional GIS stakeholders include geographic information users, developers, and data producers. VGI stakeholders include consumers, special interest groups, local communities, and professionals. The data usability challenges identified by Brown et al. include new directions of data use, data (quality, language, quantity, and detail), metadata, user needs, and standardization and interoperability. Multiagency program managers striving to achieve effectiveness, efficiency, and satisfaction from stakeholders and users should consider their audience, as well as how the data will serve the program objectives, as demonstrated by Brown et al.
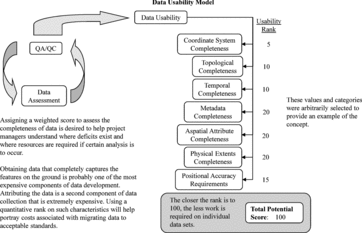
Metadata Requirements and Documentation
Metadata are records of information that describe the basic characteristics of data. Specifically, they explain the history, age, and character of the data, allowing one to make decisions regarding currency and maintenance plans. Metadata may also limit data liability by stating appropriate uses while also promoting accountability of data quality. Furthermore, they have a multitude of uses in the planning stage, allowing for retention of information about the data after personnel changes and limiting duplication of data development. The Federal Geographic Data Committee (FGDC) adopted the Content Standard for Digital Geospatial Metadata (CSDGM) in 1994 and revised the standard in 1998. Executive Order 12096 requires all federal agencies to use this standard for documenting geospatial data created from January 1995 to the present. Since that time, many state and local governments and private industries have also adopted the standard. Later, the International Organization for Standardization developed a new metadata standard that many organizations have adopted because of its support for Web services, flexibility, and representation of data, which the CSDGM cannot fully capture. The ISO metadata standards are similar to those of the FGDC. A list of the ISO standards and a crosswalk to the older FGDC standards include ISO 19110 (FGDC CSDGM Section 5 Entity and Attribute Information), ISO 19115 (FGDC CSDGM FGDC-STD-001-1998), ISO 19115.2 (FGDC Remote Sensing Extension FGDC-STD-012-2002), ISO 19119 (No FGDC equivalent, Service Extensions), ISO 19115(E) (FGDC CSDGM Biological Profile FGDC-STD-001.1-1999), and ISO 19157 (FGDC CSDGM Section 2 Data Quality Information).
Metadata are probably some of the most important components of working with any form of spatial data; without this information, data become difficult to use for cartographic applications, exploratory analysis, or research. Without compliant metadata, dissemination of information, and data between partners, scientists and the public are greatly affected. The time and effort required to deal with data lacking metadata is often an overlooked expense during project planning. For example, it can be time consuming to track down the most basic information needed to create metadata, yet very quick, therefore inexpensive, to create compliant metadata when GIS analysts first develop the data. Brown et al. (Citation2013) suggest that metadata are not developed as frequently as expected because they are not the focus of research objectives. Although we agree with their statement, metadata often are not generated because of the lack of easy-to-use and automated software tools. Most software does not automate the entity and attribute section and they tend to use metadata jargon that is not familiar to non-GIS professionals, for example biologists, who often work with spatial data. One newly released software tool (Ignizio, O’Donnell, and Talbert; in review) provides a graphical user interface that automates the creation of entity and attribute content, map projections, and many metadata components that data developers struggle with (GIS and non-GIS professionals). The developers’ objective was to provide a tool that avoids jargon and simplifies the process of metadata development. Metadata are critical components of data usability, and managers should include these requirements within data management plans.
If data do not have compliant metadata, managers can instead establish an acceptable level of metadata (). For example, “metadata light” can provide rudimentary information located via Web sites or correspondence with the data provider when complete metadata are unavailable and resources to generate compliant metadata do not exist. However, metadata light is not a long-term solution, and at some point, data released to the public from government agencies must contain FGDC-compliant metadata. Additionally, a program director might consider developing metadata templates to facilitate, standardize, and automate metadata development. Several GIS tools exist to facilitate the development of metadata. A few of these resources include ESRI ArcGIS Desktop (http://www.esri.com), Environmental Protection Agency metadata editor (http://edg.epa.gov/EME), National Park Service (http://www.nps.gov/gis/data_info/metadata.html), FGDC (http://www.fgdc.gov/metadata), OSGeo (http://wiki.osgeo.org/wiki/metadata_software), and U.S. Geological Survey Fort Collins Science Center Metadata Wizard (Ignizio, O’Donnell, and Talbert, in review; http://www.sciencebase.gov/metadatawizard).
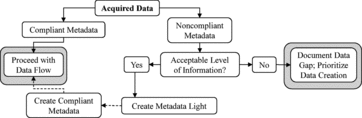
The USGS software gleans content from the tools listed above and attempts to enhance these tools by reducing the metadata standard jargon and automating the population of metadata using the geospatial content extracted from GIS data.
Product Tracking
Product tracking, which refers to tracking of data, map products, and deliverables, is the next component of any program management process. There are several challenges associated with product tracking, which include, but are not limited to, the following:
Data are housed by organizations at various levels, resulting in multiple versions of the same data sets.
An organization can obtain a data set, and then improve the data, but the changes do not filter back to the collaborators.
A lack of appropriate documentation (e.g., metadata) makes data tracking more difficult.
Tracking individual projects via funding, spatial locations, and data driver needs can help partners track ongoing and completed projects, but it creates multiple needs (i.e., complexity).
A centralized Web-based data search engine allows all parties to be cognizant of the type and extent of products created for a program. Furthermore, tracking of information products and efforts between all partners is essential for project management, and it provides continuity between staff for the life of the program.
Although tracking data deliverables via Web-based products is beneficial, smaller programs may not require such efforts. During the initial stages of a program/project, data managers may use data calls to procure data. However, data often require a review or QA/QC process; therefore, routing data calls through data managers can improve the quality of products used during the initial phases of a program. In this case, partners may decide to channel data requests through agency representatives to minimize data collection and duplicated efforts. We propose a conceptual model to aid in identifying an appropriate strategy to track data products ().
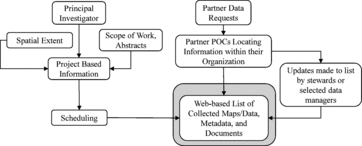
Data Management, Storage, and Dissemination Protocols
Any large-scale program will face challenges when it comes to data management and data storage. In fact, small projects will also face these challenges, but the choices are generally less expensive. We outline and illustrate in several components specific to managing data:
Provide ample storage and backups for anticipated data requirements.
Figure 12 An outline of considerations for disseminating information affecting data usability and efficiencies. The outline is scalable to match the level of complexity associated with a given geospatial program. Due to the numerous approaches available to people, the important concept of this figure is that programs should consider how information is disseminated and whether the information is usable (e.g., data format, jargon, data completeness, documentation completeness, accessibility to program participants and the public). Provide for updates and upgrades to content as well as storage equipment.
Because users collect data from many disparate sources, standards of data formats, metadata, and other spatially related standards are generally necessary.
Use Web-based applications for large programs/projects to provide wide access to the data for updates, downloads, searching and access to publications, ongoing research, and management plans. With Web-based applications, webmasters can maintain the appropriate level of access to content using roles, which ensures data security of sensitive materials.
Required editing of data by multiusers increases data management difficulties and cost (e.g., data are stored as flat files versus relational database management systems [RDBMS]), which coordinators should consider as part of the various methods at the beginning of a project.
Maintaining dynamic—or real-time—data, archiving data, tracking these data via metadata servers, and replicating data are critical when establishing a management plan.
Data management and storage is entwined () with data quality and metadata constraints. The most important component of data management is to arrive at a protocol to evaluate, organize, and make the data available and usable.
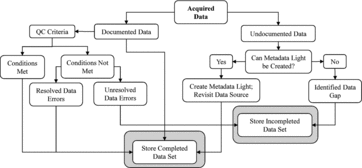
Geospatial data can exist in a myriad of data formats and proprietary sources. These different types of data (e.g., polygonal, line, and points, vector and raster) are proprietary formats (e.g., shapefiles, MapInfo files, CADD files, HDF, netCDF, ERDAS Imagine, ESRI GRID, MrSID, and GRASS), and their database formats (Oracle, PostgreSQL/PostGIS) can complicate how data are shared between partners. An understanding of the types of data a program will likely encounter can help archive data and provide for accessible and usable data by collaborators. In some circumstances, researchers procure sensitive data that require special management protocols that a management plan will address.
Data managers can store data using many different methods, and understanding some of these methods will help determine the best protocol to establish in a management plan. In the context of GIS, enterprise databases allow multiple concurrent users access of shared data resources. All databases rely on RDBMS, which permit an unlimited level of relationships between tables. Other important advantages of using enterprise databases include the ability to enforce greater security management practices, centralize data management, and share data between servers (i.e., off-site, real-time replication of data). Databases are not specific to storage of geographic data, so that data managers can manage and disseminate all types of data with an enterprise system. “Selecting the right software technology, building proper applications, establishing an effective database design, and procuring the right hardware all play a critical role in fulfilling system performance and scalability expectations” (Peters Citation2008, 5). Peters provides a thorough discussion on how to select appropriate resources based on program requirements. This resource also provides a set of templates referred to as the capacity planning tool (http://wiki.gis.com/wiki/index.php/Capacity_Planning_Tool), that can be used as a means of collecting user requirements, as standard workflow models that translate peak user loads to processing environments, and as teaching and learning aids.
Like RDBMS, geoservers and data clearinghouses provide mechanisms for storing, organizing, and disseminating data. For large-scale programs, coordinators may require centralized storage of information. For small-scale projects, a single identified data custodian and internal server may suffice. Identifying the data management needs in advance will improve access and usability of data. Enabling queries of metadata and data products will increase data and project visibility and increase program efficiencies. The advantages of sharing data are well documented (Van den Eynden et al. Citation2011; Goodchild, Fu, and Rich Citation2007). An effective way to share information is through spatial data clearinghouses—electronic facilities for housing and disseminating spatial data from numerous sources in an online portal—which have grown tremendously in recent years (Crompvoets et al. Citation2004). They are often referred to by a variety of names including geospatial data libraries, geolibraries, geoportals, and geospatial archives and clearinghouses (Goodchild, Fu, and Rich). Objectives of these efforts vary from general themed portals such as the Geospatial One-Stop (Goodchild, Fu, and Rich), to the National Geospatial Digital Archive, which specializes in at-risk geospatial data (Erwin and Sweetkind-Singer Citation2009).
Data Security
Dissemination of information, reports, and data allows scientists, conservationists, and managers to share ideas, distribute scientific findings, and develop policies. Most information is appropriate for release to the public, but some data and reports contain sensitive information. The Federal Geographic Data Committee provides guidelines for determining which geographic data pose security concerns (U.S. Geological Survey Citation2005). Risk management is an important component of managing information for business continuity, information technology, and information security (Esri System Design Strategies). Information security addresses threats to information from natural disasters, malicious internal and external attacks, malfunctions, and human error (Information Security, Esri). Information security management requires addressing confidentiality, integrity, and availability of information. These apply to hardware, software, communications, personal security, organizational security, and physical security (Information Security, Esri). In addition to understanding the threats and managing these threats, programs can incorporate standards developed by other organizations. OGC has developed three groups to address securing geospatial information: the GeoRights Management Domain Working Group, the Security DWG, and OGC GeoXACML standards Working Group (Matheus Citation2010). These groups are actively developing security standards related to geospatial Web services, geoprocessing workflows, and simple object access protocol communications for secure interconnections. Li et al. (Citation2010) highlight the lack of standard security measures for service-based geospatial data sharing and the challenges of multiagency data sharing. To address these shortcomings, they provide a security model workflow that identifies the components necessary for securing shared data. With increased use of cloud computing for geospatial applications, researchers are developing similar security models (Li et al. Citation2013; AlZain, Soh, and Pardede Citation2013). Securing information in the cloud, within enterprise (relational) databases for Web services and GeoWeb applications require different methods than securing local spatial data. Rajpoot (Citation2013) provides a security model of locally stored spatial data that can benefit cases wherein securing information of large data is not hosted or accessed online, and for smaller projects. Although the discussion and consideration of information security is beyond the scope of this article, it should be mentioned that many organizations are recognizing the significance of protecting information and ensuring its availability to end users while maintaining licensing and related legal issues. Consequently, programs should expect to benefit from investigating, understanding, and incorporating security measures within management plans.
CONCLUSION AND CHALLENGES
We addressed many of the facets related to integrating geospatial applications in multiscale programs and provided a framework using questions, diagrams, and topic lists to assist programs with developing geospatial management plans. Geospatial and infrastructure technologies are constantly changing with advances in cyberinfrastructure (Yang et al. Citation2010) and the advent of new data collection tools such as crowdsourcing and social media platforms (Levental Citation2012). Therefore, understanding and developing management plans for large-scale programs is dynamic. Continual education, and incorporation of modifications to geospatial frameworks are beneficial for an effective plan. We gleaned a list of topics and references () from this article to highlight the standards, documents, and resources we considered important and useful. Managers may decide to familiarize themselves with some of the key sources presented here, given the vast amount of information that exists on the topics discussed herein. We believe that without consideration of the many facets shared, and without a well-structured management plan, geospatial efforts, especially large-scale efforts, will be unsuccessful in meeting their objectives. Furthermore, input from all associated parties is essential to creating a workable and universally accepted plan by all collaborators. Once program coordinators build the geospatial framework, then researchers and land managers can use the data to ask, answer, and understand important ecological and management questions.
Table 1 Important Standards, Documents, and Resources Recognized for Developing Geospatial Management Plans
Our primary goal was to illustrate how the creation of a geospatial management plan is a scalable effort. Understanding the factors that affect how this integration occurs is the first step. The second step is to understand all the components and how to scale the components based on program requirements. Third, developing management plans and implementation plans will highlight the program efforts, which will lead to a more successful effort. And finally, communication between and involvement of all collaborators will facilitate the adoption of standards by all participants. Our approach is similar to an argument presented by Peters (Citation2008, 15) suggesting, “If system architecture design were a step-by-step process, the first step would be to review all your options before committing to any one of them.” We hope this document will serve as a reference and a starting point in developing a successful geospatial management plan for programs and projects.
ACKNOWLEDGEMENT
This work was supported by the Wyoming Landscape Conservation Initiative (WLCI, http://www.wlci.gov) and the U.S. Geological Survey at the Fort Collins Science Center (http://www.fort.usgs.gov). We thank Daniel Manier, Robert McDougal, and Tim Kern for constructive comments that improved the manuscript. Any use of trade, firm, or product names is for descriptive purposes only and does not imply endorsement by the U.S. government.
APPENDIX A. A Detailed Framework for Developing a Management Plan for the Integration of Geospatial Data within a Multiorganizational Program
Overview
Create a framework of questions that relate to integration of geospatial efforts in a landscape-scale, multiorganizational program based on the information presented in this table.
Geospatial Coordination Team Structuring
Establish a list of teams likely required for managing the different components identified for the program. Examples of such teams are listed here.
Geospatial Coordination Team: The role of this team (or individual, depending on scope of project) should ensure that the proper steps are taken to develop a management plan as well as maintain involvement of experts throughout the life cycle of the program. This team should also be responsible for coordinating all teams and relaying information between teams.
Geospatial Data Management Team: The role of this team (or individual, depending on scope of project) will include the oversight of numerous aspects of data management such as data collection, data development, development of SOPs, development of geospatial tools/programs to assist in implementation of data development and documentation, and so forth. This team should have representatives from each partner to behave as a point of contact for gathering information.
Web/Database Engineering Team: The role of this team (or individual, depending on scope of project) will establish the methods for data archiving, data storage, and data dissemination. Furthermore, this team will develop the necessary applications for disseminating data, documents, products, and other relevant information related to the project.
Identify representatives from each agency that can inform each of the major geospatial components. These individuals should include both data managers as well as subject experts. The roles of these individuals should be clearly outlined and approved so they can be involved in developing a geospatial management plan. Expect roles to change as this process evolves and keep participants actively involved in how their roles change.
Ascertain the Scope of Geospatial Needs
Outline how the multiorganizational program requires integration of geographic information systems (GIS)
Identify what steps of the program efforts require use of spatial data.
Identify what type of data is likely required (e.g., data themes such as soils and hydrology).
Identify the data formats likely required for the program (e.g., raster, relational databases, time-series for long-term monitoring).
Identify how data procurement efforts will be distributed across partners. What are the associated costs of each of these tasks? How will partners share efforts, costs, and responsibilities of collecting and managing data?
Identify required GIS support staff for all facets of the geospatial effort (e.g., GIS analysts, technicians, database managers, geospatial data managers, IT administration/support, and managers, Web developers, and how these resources will be distributed across partners).
Identify requirements of data storage infrastructure. If the data/metadata are hosted online or at one particular location, what agency will be responsible for managing such data (e.g., storing data and serving data to partners)? Are data mirrored off-site in the event servers or hardware are lost at any one location? What standards are required for serving the data/metadata? What permissions/restrictions are enforced for accessing the data to various partners/public users?
Identify required Web services.
Web service tools
Enterprise databases
GIS tools for online applications
Metadata servers
Reporting tools
Identify the data users and data developers to determine demands (e.g., database loads) for data distribution.
Develop a list of SOPs (e.g., GIS/RS, related infrastructure of geospatial tasks, metadata, and attributing). A list of some existing standards follows:
Content standard for digital geospatial metadata (CSDGM) is a Federal Geographic Data Committee (FGDC) standard.
National Standard for Spatial Data Accuracy (NSSDA) is an FGDC standard that denotes a methodology for testing for positional accuracy.
National Geospatial Program Standards (http://nationalmap.gov/gio/standards).
American Society for Photogrammetry and Remote Sensing (ASPRS) Positional Accuracy Handbook.
FGDC standards (http://www.fgdc.gov).
Tri-Service Spatial Data Standard (TSSDS) (a.k.a. Spatial Data Standards for Facilities, Infrastructure, and Environment (SDSFIE)). This standard has been adopted by many government agencies and it encompasses many scientific and nonscientific (e.g., AM/FM) data standards.
Outline the potential challenges of integrating geospatial efforts in a multi-organization program.
How will the program accomplish integrating the varying levels of standard operating procedures across partners? The difficulties often reside with accepting a minimum level of standardization because some partners will have less stringent standards while others require more stringent standards.
Additional challenges with arriving at standardization of geospatial data management is that standards may be required or exist for partners, but are not fully implemented. Knowing how to deal with such instances is important when initially establishing a collaborative effort because additional costs may be incurred.
Identify how dissemination of information between agencies will occur.
How will the roles of the different agencies play into the integration of geospatial efforts? How will agencies delegate/share responsibilities? How will agencies determine which roles are better suited for each agency based on available resources?
Identify why standard operating procedures are necessary in a multi-organization program.
Establishes easily identified and clearly distinguished roles to improve the integration of geospatial efforts across partners.
Establishes consistency and clarity throughout data.
Encourages a cost effective approach to data management.
Minimizes confusion about data formats, data accuracies, coordinate systems, and other GIS related topics for both GIS and non-GIS users.
Educates team members and promotes communication between partners.
Facilitates and expedites deliverables of acceptable quality.
Identify required GIS software/hardware and associated costs, and cost-sharing for a multi-organization program.
Purchasing of on-site and off-site (i.e., mirroring of data) architecture to support data management and web services.
Costs associated with maintenance and support of architecture.
Costs associated with software requirements for developing, archiving, disseminating, and processing data/metadata.
Data management concepts
Identify the major data considerations of a project required before collecting data:
Implications of identifying a common area of interest.
Define the geospatial area of interest(s) required to support the project initiative.
Does the study area capture the biotic or abiotic information in surrounding areas that affect the results?
What are the additional costs for collecting data with the proposed project AOI? Are there implications (costs) for variations in AOI size?
What are the biotic and abiotic requirements of the project with respect to data applications?
Define a universal coordinate system.
How does the size of the project AOI affect the determination of an appropriate map projection (e.g., global, continental, national, state or province)?
What distortions (area, shape, angle, and distance) are possible? Are there options or contingencies?
Which map projection will best capture the AOI while minimizing distortion?
How will the map projection affect analytical results for both vector and raster data sets?
Define data requirements/drivers.
What is the intended use and application for data? What are the intended data-related products?
What other projects might benefit from using the data set?
How will individual participants benefit from using the data set?
What is the appropriate scale, attribute completeness, topology completeness, metadata completeness required to meet the project's demands?
What is the level of effort required to complete/create the data as a data driver?
Who currently owns and maintains this data if it exists and should funding be allocated to this agency to facilitate completeness.
How are funds allocated to different projects and which of these projects require the different data sets?
Develop a survey (for large group projects) to identify data requirements and then establish a list of data drivers based on a specified criteria developed by coordination teams.
Data collection and data lifecycles.
Determine maintenance requirements (stewards and updates to data sets).
Determine what requirements exist for collecting temporal data and how this data will be best managed.
Ascertain the lifecycle of the program to establish the best method for collecting and disseminating data to partners (e.g., is it more efficient to let partners/data owners keep data locally or is one or more centralized data managers required because partners do not have a mechanism to disseminate data).
Human resources are constantly changing over time and the longer a program lasts the greater the number of turnover occurrences. Therefore, establishing standard operating procedures minimizes the effects of staff transitions for the program objectives, will minimize inefficiencies of data management, and create data usability.
Establish data quality and data control requirements.
Create QA/QC plan.
Establish the criteria of each data set (e.g., use of data dictionaries or data models).
Establish quality control methods to inspect features and attributes.
Investigate each component of a QA plan: project management, project design, project data assessment, and project reporting and oversight.
Create a list of QC tasks to quantify the data quality and established requirements. Generally, this can be broken down into two steps: an automated process using GIS tools and a manual or visual inspection of the data.
Measure errors, which are often subjective.
Check for feature completeness.
Check for feature accuracy (e.g., do GIS features describe what is actually on the ground at their locations?).
Check for attribute value accuracies (e.g., is the feature labeled correctly?).
Check for attribute value precision (e.g., what is required scale to capture some/or all features?).
Establish metadata requirements and data documentation.
Does any form of documentation or metadata exist for spatial data? Do the participants have existing requirements?
For the metadata that does exist, is the metadata content and syntax FGDC compliant?
What are the costs to make existing data have compliant metadata and which partners will be responsible for such a task? Will compliant metadata be developed for older data sets that are required for a project?
Who is responsible for QA/QC of metadata before data is published online or provided to the public?
Will data be hosted on a metadata server? If so, how will partners be made aware of available products?
Is ‘Metadata Light’ an option? Will metadata light provide short-term or long-term solutions? If metadata light is an option, how should it be defined?
What data are required to maintain compliant metadata?
Establish tracking of products and data.
Data is housed by organizations using different archiving standards. Centralizing information at one or more web service nodes may be desired.
Data sets may be obtained by one organization, then improved upon but not relayed back to the original organization. Therefore, determining how data is maintained and disseminated is important consideration.
A lack of metadata/documentation makes data tracking very difficult and therefore being cognizant of all components of managing geospatial data need consideration.
Without a coordinated effort, multiple project partners could contact data sources with the same request, and therefore, assigning data stewards to each partner will minimize multiple requests of the same data.
Tracking data and activities via spatial locations and data driver needs can help partners track ongoing projects to avoid conflicts in fieldwork, increase discussion between overlapping projects, and establish similar data needs as well as how funding may be allocated to developing data drivers.
Establish data management / data storage protocols.
Evaluate costs and efforts associated with providing ample storage and backups required for a large amount of data.
Establish standards of data formats, metadata, and other spatial related items.
Methods to facilitate data maintenance, downloads, and data searches need to be available (e.g., web-based applications for large programs are essential).
Maintaining dynamic (i.e., real-time) data, archiving of data, and tracking of this data via metadata servers, and replication is critical to establish within an SOP.
Several issues that often arise related to data include methods and protocols for sharing data with partners, including ease of access to data and different security constraints, data request forms, and data release waivers.
Workshop for involving all partners with developing management plan and implementation plan
After developing the framework of a management plan and identifying individuals that are able to assist in the process, have a workshop with clear objectives to finalize the geospatial management plan. It is important to involve all partners during this process if implementation of the management plan is to succeed.
Develop an implementation plan to outline how each agency will comply with the geospatial management plan and a projected time frame for completion. This plan should consider the lifecycle of the program, short- and long-term objectives, costs associated with implementation, roles of the various partners for implementation, and scheduling of each relevant component in the management plan.
REFERENCES
- AlZain, M.A., B. Soh, and E. Pardede. 2013. A survey on data security issues in cloud computing: From single to multi-clouds. Journal of Software8(5): 1068–1078.
- Bowles, T.2002. Remote sensing and geospatial data used as evidence. A survey of case law. 2L at University of Mississippi: School of Law. http://www.crowsey.com/pdf/caseLawSurvey.pdf (accessed November 15, 2013)
- Brown, M., S. Sharples, J. Harding, C.J. Parker, N. Bearman, M. Maguire, D. Forrest, M. Haklay, and M. Jackson. 2013. Usability of geographic information: Current challenges and future directions. Applied Ergonomics44(6): 855–865.
- Cho, G.2005. Geographic information science: Mastering the legal issues. Hoboken, NJ: Wiley & Sons.
- Crompvoets, J., A. Bregt, A. Rajabifard, and I. Williamson. 2004. Assessing the worldwide developments of national spatial data clearinghouses. International Journal of Geographical Information Science18(7): 665–689.
- Currid, E., and S. Williams. 2009. The geography of buzz: Art, culture and the social milieu in Los Angeles and New York. Journal of Economic Geography10(3): 423–451, . doi:10.1093/jeg/lbp032. . http://joeg.oxfordjournals.org/cgi/doi/10.1093/jeg/lbp032
- Dischinger, S. and L.A. Wallace. 2005. Geographic information systems: Coming to a courtroom near you. The Colorado Lawyer34(4): 11–21.
- Elwood, S.2008. Volunteered geographic information: Key questions, concepts and methods to guide emerging research and practice. GeoJournal72(3–4): 133–135.
- Elwood, S.2010. Geographic information science: Visualization, visual methods, and the geoweb. Progress in Human Geography35: 401–408.
- Environmental Protection Agency. 2003. Guidance for geospatial data quality assurance project plans. Washington, DC: U.S. Environmental Protection Agency, Office of Environmental Information.
- Erwin, T., and J. Sweetkind-Singer. 2009. The National Geospatial Digital Archive: A collaborative project to archive geospatial data. Journal of Map & Geography Libraries6(1): 6–25.
- Federal Geographic Data Committee. 2005. . http://www.fgdc.org (accessed March 1, 2013).
- Federal Geographic Data Committee. “Geospatial Metadata Standards—Federal Geographic Data Committee.” . http://www.fgdc.gov/metadata/geospatial-metadata-standards (accessed January 2, 2014).
- Feeman, T.G.2002. Portraits of the earth: A mathematician looks at maps. Providence, RI: American Mathematical Society.
- Goodchild, M.F.2007. Citizens as sensors: The world of volunteered geography. GeoJournal69: 211–221.
- Goodchild, M.F.2008. Commentary: Whither VGI?GeoJournal72: 239–244, . doi:10.1007/s10708-008-9190-4. . http://link.springer.com/10.1007/s10708-008-9190-4
- Goodchild, M.F., and J.A. Glennon. 2010. Crowdsourcing geographic information for disaster response: A research frontier. International Journal of Digital Earth3(3): 231–241, . doi:10.1080/17538941003759255; http://www.tandfonline.com/doi/abs/10.1080/17538941003759255
- Goodchild, M.F., P. Fu, and P. Rich. 2007. Sharing geographic information: An assessment of the Geospatial One-Stop. Annals of the Association of American Geographers97(2): 250–266.
- Guo, D.2007. Visual analytics of spatial interaction patterns for pandemic decision support. International Journal of Geographical Information Science21(8): 859–877, . doi:10.1080/13658810701349037; http://www.tandfonline.com/doi/abs/10.1080/13658810701349037
- Ignizio, D.A., M.S. O’Donnell, and C.B. Talbert. In Review. Metadata wizard: An easy-to-use tool for creating FGDC-CSDGM metadata for geospatial datasets in ESRI ArcGIS Desktop (U.S. Geological Survey Data Series). . https://www.sciencebase.gov/metadatawizard
- “Information Security.”. GIS Wiki. http://wiki.gis.com/wiki/index.php/Information_Security (accessed January 1, 2014).
- Jelinski, D.E., and J. Wu. 1996. The modifiable areal unit problem and implications for landscape ecology. Landscape Ecology11(3): 129–140.
- Kervin, K.E., W.K. Michener, and R.B. Cook. 2013. Common errors in ecological data sharing. Journal of eScience Librarianship2(2): 3–16.
- Kimerling, A.J.2002. Predicting data loss and duplication when resampling from equal-angle grids. Cartography and Geographic Information Science29(2): 111–126.
- Levental, S.2012. A new geospatial services framework: How disaster preparedness efforts should integrate neogeography. Journal of Map & Geography Libraries8(2): 134–162.
- Li, D., J. Zhang, and H. Wu. 2012. Spatial data quality and beyond. International Journal of Geographical Information Science26(12): 2277–2290.
- Li, G., C. Li, W. Yu, and J. Xie. 2010. Security accessing model for Web service based geo-spatial data sharing application. Paper presented at the 3rd ISDE Digital Earth Summit, Nessebar, Bulgaria, June 12–14.
- Li, X., Z. Liu, W. Liu, A. Xu, and L. Ma. 2013. A spatial data security model under the cloud environment. Advanced Materials Research765–767: 1267–1270.
- Longhorn, R.A., V. Henson-Apollonio, and J.W. White. 2002. Legal issues in the use of geospatial data and tools for agriculture and natural resource management: A primer. Mexico, D.F.: International Maize and Wheat Improvement Center (CIMMYT): 1–42.
- Matheus, A.2010. Securing geospatial information. GEO:connexion, . October 1.
- Michener, W.K.1997. Quantitatively evaluating restoration experiments: Research design, statistical analysis, and data management considerations. Restoration Ecology5(4): 324–337.
- Michener, W.K., J.W. Brunt, J.J. Helly, T.B. Kirchner, and S.G. Stafford. 1997. Nongeospatial metadata for the ecological sciences. Ecological Applications7(1): 330–342.
- Michener, W.K., J. Porter, M. Servilla, and K. Vanderbilt. 2011. Long term ecological research and information management. Ecological Informatics6(1): 13–24.
- Mulcahy, K.2000. Two new metrics for evaluating pixel-based change in data sets of global extent due to projection transformation. Cartographica: The International Journal for Geographic Information and Geovisualization37(2): 1–12.
- National Coastal Data Development Center. Metadata Standards. . http://www.ncddc.noaa.gov/metadata-standards/ (accessed January 1, 2014).
- Office of Management and Budget. Geospatial Line of Business, OMB Circular A-16 Supplemental Guidance. The White House. . November 10, 2010. . http://www.whitehouse.gov/sites/default/files/omb/memoranda/2011/m11-03.pdf
- Onsrud, H.J.1992. Evidence generated from GIS. GIS Law1(3): 1–9.
- OpenStreetMap Foundation. License/We are changing the license. . http://www.osmfoundation.org/wiki/License/We_Are_Changing_The_License (accessed January 1, 2014).
- Peters, D.2008. Building a GIS: System architecture design strategies for managers. Redlands, CA: Esri.
- Rajpoot, M.S.2013. A location-based secure access control mechanism for geospatial data. International Journal of Computer Applications79(11): 28–32.
- Roche, S., E. Propeck-Zimmermann, and B. Mericskay. 2011. GeoWeb and crisis management: Issues and perspectives of volunteered geographic information. GeoJournal78(1): 21–40, . doi:10.1007/s10708-011-9423-9; http://link.springer.com/10.1007/s10708-011-9423-9
- Saunders, A., T. Scassa, and T.P. Lauriault. 2012. Legal issues in maps built on third party base layers. Geomatica66(4): 279–290.
- Scassa, T.2013. Legal issues with volunteered geographic information. The Canadian Geographer/Le Geographe Canadien57: 1–10. doi:10.1111/j.1541-0064.2012.00444.x. http://doi.wiley.com/10.1111/j.1541-0064.2012.00444.x.
- Seong, J.C.2003. Modelling the accuracy of image data reprojection. International Journal of Remote Sensing24(11): 2309–2321.
- Seong, J.C.2005. Assessing resampling accuracy of categorical data using random points. Cartography and Geographic Information Science32(4): 393–400.
- Seong, J.C., and E.L. Usery. 2001. Assessing raster representation accuracy using a scale factor model. Photogrammetric Engineering and Remote Sensing. 67(10): 1186–1191.
- Steinwand, D.R., J.A. Hutchinson, and J.P. Snyder. 1995. Map projections for global and continental data sets and an analysis of pixel distortion caused by reprojection. Photogrammetric Engineering and Remote Sensing61(12): 1487–1497.
- Stine, K., R. Kissel, W.C. Barker, J. Fahlsing, and J. Gulick. 2008a. Volume I: Guide for mapping types of information and information systems to security categories.Gaithersburg, MD: National Institute of Standards and Technology, U.S. Department of Commerce.
- Stine, K., R. Kissel, W.C. Barker, A. Lee, and J. Fahlsing. 2008b. Volume I: Appendices to guide for mapping types of information and information systems to security categories.Gaithersburg, MD: National Institute of Standards and Technology, U.S. Department of Commerce.
- Turner, A.J.2006. Introduction to Neogeography. O'Reilly Media, Inc.
- Turner, A.J.2007. Neogeography-towards a definition. A weblog posting on High Earth Orbit, 6 December 2007. . Retrieved from http://highearthorbit.com/neogeography-towards-a-definition/.
- U.S. Geological Survey, 2005. Guidelines for providing appropriate access to geospatial data in response to security concerns plans. Washington, DC: Federal Geographic Data Committee, U.S. Geological Survey.
- Van den Eynden, V., L. Corti, M. Woollard, L. Bishop, and L. Horton. 2011. Managing and sharing data: Best practice for researchers.Wivenhoe Park, Colchester, Essex, UK: UK Data Archive, University of Essex. . http://data-archive.ac.uk/media/2894/managingsharing.pdf
- White, D.2006. Display of pixel loss and replication in reprojecting raster data from the sinusoidal projection. Geocarto International21(2): 19–22.
- Yang, C., R. Raskin, M. Goodchild, and M. Gahegan. 2010. Geospatial cyberinfrastructure: Past, present and future. Computers, Environment and Urban Systems34(4): 264–277.