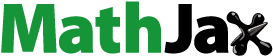
Abstract
This paper studies the impact of investor sentiment on the Bitcoin returns and conditional volatility taking into account the Covid-19 outbreak by using different investor sentiment proxies and by employing the EGARCH model. Estimation results show that investor sentiment has a positive impact on the Bitcoin returns and their volatility, especially after the Covid-19 outbreak. The VAR model is employed to investigate whether investor sentiment and Bitcoin returns are related in a dynamic setting and to make distinguish between rational and irrational investor sentiments. The results from the VAR model show that both rational and irrational investor sentiments have an impact on Bitcoin returns indicating that the Bitcoin market is also driven by emotions and noise traders have an impact on the data generating process of Bitcoin returns. The positive impact of investor sentiment can be attributed to the fear of missing out (FOMO) behavior of speculative and irrational investors.
Introduction
In 2008 Satatoshi Nakamoto introduced the first cryptocurrency known as Bitcoin that offers the promise of lower transaction fees than traditional online payment mechanisms and the system runs on a decentralized peer-to-peer network, unlike government-issued currencies. The market value of one bitcoin was less than five cents in 2010 and reached a peak of nearly $63,400 in 2021. Bitcoin is a new and popular phenomenon however big institutions are not a part of the Bitcoin market, in particular, small businesses and individuals are bitcoin investors. Some individual investors holding Bitcoin are cryptocurrency enthusiasts, criminals, and mainly speculative investors. (Foley, Karlsen, and Putniņš (Citation2019)) found that approximately 25% of all bitcoin users are involved in illegal activity. Bitcoin is designed as a digital currency however according to Yermack (Citation2013), Bitcoin is a speculative investment. Baur, Hong, and Lee (Citation2018) studied whether Bitcoin is a medium of exchange or an asset by analyzing the statistical properties of Bitcoin and concluded that Bitcoin is uncorrelated with traditional asset classes such as stocks, bonds, and commodities, and Bitcoin is mainly used as a speculative investment and not as an alternative currency or medium of exchange. Wang et al. (Citation2019) studied whether Bitcoin can be regarded as a hedging or a safe haven asset by comparing Bitcoin to six classical asset classes and their findings indicate that Bitcoin ought to be used for speculative investing, not for hedging purposes, due to its large returns and high volatility. The speculative use of Bitcoin and astonishing price increases attracted noise traders. The most basic implication of noise-trader theory is that irrational investors acting coherently on a noisy signal that can cause systematic risk. If noise traders affect prices, the noisy signal is sentiment, and the risk they cause is volatility, then sentiment should be correlated with volatility (Brown Citation1999).
One of the most important financial innovations of the last decade is Bitcoin therefore it is important to investigate the determinants that affect Bitcoin return and its volatility. The existing literature mainly focused on the impacts of the following determinants: macroeconomic and financial determinants, supply and demand as money price determinants, the difficulty of Bitcoin mining as the technological determinant, and Google search volume as attractiveness determinant. In this paper, the focus lies in identifying the impact of investor sentiment from the perspective of behavioral finance to understand and identify Bitcoin as a new asset. It is very essential to investigate the impact of investor sentiment because of the speculative use of Bitcoin by noisy traders.
The outbreak of Covid-19 started in December 2019 in Wuhan, China. The World Health Organization (WHO) declared the Covid-19 epidemic as a pandemic in March 2020. In March, measures and lockdowns are implemented all over the world to control the spread of Covid-19. Lockdown announcements, increasing death and patient numbers all over the world caused some concerns because of fear of illness, death, losing incomes, jobs, and beloved ones. Naseem et al. (Citation2021) analyzed investor psychology and stock market behavior during COVID-19 and found that investor psychology was negatively related to those stock markets during the pandemic outbreak indicating that investor sentiment caused by these concerns affected stock markets. The investor sentiment caused by these pandemic-related concerns may affect Bitcoin prices. To investigate the impact of investor sentiment during the Covid-19 period, this paper analyses the available data and attempts to answer the following main research question: how does investor sentiment affect Bitcoin returns and its volatilities during the Covid-19 outbreak? Data is divided into 3 periods: all periods, before March 2020, and from March 2020 to investigate the impact of investor sentiment on Bitcoin returns and conditional volatilities especially taking into account the possible impact of the Covid-19 pandemic.
In the existing literature, only one investor sentiment proxy is used to analyze the impact of investor sentiment on bitcoin returns and return volatilities. There is no perfect proxy for the investor sentiment therefore three different proxies are used to check if different investor sentiment proxies give similar results. The first proxy is the Bitcoin trading volume because Baker and Stein (Citation2004) argued that when a market is unusually liquid and the market liquidity can be measured by the trading volume and the trading volume is available for relatively long periods. The second proxy is the Crypto Fear & Greed Index which is daily and special for crypto investors. The third proxy is the American Association of Individual Investors Index which is weekly.
The Vector Autoregressive (VAR) model is employed to investigate whether investor sentiment and Bitcoin returns are related in a dynamic setting and to make distinguish between rational and irrational investor sentiments.
The study of Katsiampa (Citation2017) shows that the CGARCH model is the best based on the goodness-of-fit for the Bitcoin price volatility analysis therefore it is decided to select the CGARCH model. Moreover, this paper investigates the asymmetric volatility effect therefore, EGARCH, GJR-GARCH, and AP-ARCH models are included as well. Another contribution of this research is the selection of the best GARCH model when the investor sentiment proxy is included in the mean and variance equations. Component Generalized Autoregressive Conditional Heteroscedastic GARCH (CGARCH), Exponential GARCH (EGARCH), Glosten Jagannathan Runkle GARCH (GJR-GARCH), and Asymmetric Power Autoregressive Conditional Heteroskedastic (AP-ARCH) models. To select the best model the Bitcoin trading volume is chosen as the investor sentiment proxy because this proxy has more data which is important to compare GARCH models.
The paper contributes to the literature in the following distinct ways:
The first contribution is the inclusion of the impact of the Covid-19 pandemic to study the impact of investor sentiment on Bitcoin prices by dividing data into periods. This contribution is unique because, as far as known, the impact of the investor sentiment on Bitcoin taking into account the Covid-19 pandemic has not been studied yet.
This paper includes three different investor sentiment proxies which is the second contribution.
So far the impact of irrational investor sentiment is ignored in Bitcoin and investor sentiment studies. Making a distinction between rational and irrational investor sentiments is the third contribution.
This paper is structured as follows: the second section gives an overview of the existing literature. In the third section, the description of the data is given. In the fourth section, the different econometric models are presented. In the fifth section, the empirical results of the different econometric models are presented and discussed. Finally, the sixth section provides conclusions.
Literature review
In order to better understand the topic, a review of relevant literature is provided in this section. In the first subsection, the impact of investor sentiment is discussed. In the second subsection, the impacts of non-sentiment factors: macroeconomic and financial variables are underlined. The last subsection is about the influence of the Covid-19 pandemic.
The impact of investor sentiment factors
One of the first papers that studied the impact of investor sentiment on Bitcoin volatility is by Bukovina and Marticek (Citation2016). They used the autoregressive AR(1) model to analyze the impact of sentiment on Bitcoin volatility and selected the Sentdex sentiment index data for which submissions and comments are analyzed via Natural Language Processing (NLP) techniques. NLP techniques are used to extract information from texts. The most widely used technique in NLP is sentiment analysis which is based on customer surveys, reviews, and social media comments where people express their opinions. Sentiment signals are defined from −3 to 6 where −3 is the most negative sentiment and 6 is the most positive sentiment. The results show that only a minor part of total volatility is explained by the sentiment index. However, during periods of high volatility, the impact of sentiment increases. Another machine learning-related study is conducted by Lopez-Cebarcos et al. (Citation2019). They made use of GARCH and EGARCH models to study the impact of investor sentiment, S&P 500 returns, and VIX returns on the Bitcoin return volatility. They used the Standford Core NLP measure for the investor sentiment variable which is defined from −2 to 2 where −2 is the most negative sentiment and 2 is the most positive sentiment. Their results indicate that all explanatory variables affect Bitcoin volatility in stable periods and Bitcoin becomes attractive to speculative investors.
Figá-Talamanca and Patacca (Citation2019) studied the impact of investor sentiment on the mean and the variance of cryptocurrency returns by using an autoregressive moving average (ARMA), GARCH, and EGARCH models. The main difference between the studies of Figá-Talamanca and Patacca (Citation2019) and Lopez-Cebarcos et al. (Citation2019) is the measure of investor sentiment. For the investor sentiment proxy, Figá-Talamanca and Patacca (Citation2019)used trading volume and Google search index. Their results indicate that that trading volume has an impact on both the mean and the volatility of the cryptocurrency returns, whereas the search index mainly has an impact on the volatility. Another study based on the GARCH models is conducted by Eom et al. (Citation2019). They studied the distributional and dynamic properties as well as the predictability of Bitcoin return and volatility on daily data from October 2011 to May 2017 by employing GARCH, GJR-GARCH and Rational GARCH (RGARCH) models. In addition, the investor sentiment impact is studied to improve the predictability of Bitcoin and to check whether Bitcoin is an investment asset or a monetary asset. The Google trend index is used as the investor sentiment variable. The results did not indicate a significant impact of investor sentiment on Bitcoin returns whereas there is a significant positive impact of investor sentiment on Bitcoin volatility. Briefly, the results indicate that investor sentiment has a significant information value for explaining changes in Bitcoin volatility and Bitcoin appears to be an investment asset rather than a monetary asset.
Nasir et al. (Citation2019) studied the predictability of Bitcoin volume and returns using Google search volume by employing the Vector Autoregression (VAR) framework, a copulas approach, and non-parametric drawings on weekly data from 2013 to 2017. Their results indicate that higher search volume has a positive impact on Bitcoin returns and trading volume. Another similar study is conducted by Zhu et al. (Citation2021) and the findings are similar that investor attention is the granger cause to changes in Bitcoin market both in return and realized volatility.
Naeem, Mbarki, and Shahzad (Citation2021) studied the impact of investor sentiment for six major cryptocurrency returns by using two proxies: the FEARS index and Twitter happiness sentiment. The happiness sentiment index significantly predicts returns of selected cryptocurrencies, whereas FEARS index predictability is weaker and short-term. In a recent paper, Bouri et al. (Citation2021) studied volatility spillover across fifteen major cryptocurrencies taking into account the impact of investor sentiment. Twitter feed is used for investor sentiment proxy in the dynamic conditional correlation-generalized autoregressive conditional heteroscedasticity (DCC-GARCH) model to measure the impact of investor sentiment on the volatility spillover across cryptocurrencies. Results show that when investors are extremely unhappy, the market volatility increases and market connectedness is observed whereas when investors are extremely happy, total connectedness is low. When the volatility spillover across assets is low then these assets can be used as hedging instruments of each other. The finding of this paper suggests that when investors are happy then diversification opportunities are possible between cryptocurrencies. The findings of both studies indicate that happiness or fear feelings have different impacts and the level of happiness matters as well.
A role for the US-China Trade War can be also considered as a sentiment variable. Plakandaras, Bouri, and Gupta (Citation2020) studied whether the information on the US-China trade war can be used to predict the Bitcoin returns by employing the least absolute shrinkage and selection operator machine learning technique, ordinary least square regression, and support vector regression models. Results show that there is weak evidence of the trade war factor in forecasting Bitcoin returns indicating that trade-related uncertainties have no significant impact on future Bitcoin returns.
In this section, an overview of the studies that focused on investor sentiment to analyze the returns and conditional volatilities of Bitcoin is given. In general, the results indicate that investor sentiment has an impact on the Bitcoin return and its volatility, however, in these studies, the impact of the investor sentiment during a structural break like a pandemic is missing. As explained in the introduction section and this is an important topic to focus on.
The impact of non-sentiment factors
The previous subsection contains a review of the existing literature that focused on the investor sentiment variable. This subsection contains a review of the existing literature that focused on also macroeconomic and financial variables besides sentiment variables.
Liu and Tsyvinski (Citation2018) studied the risk-return tradeoff of three cryptocurrencies: Bitcoin, Ripple, and Ethereum. For the risk factors, they selected the CAPM, Fama French 3-factor, Carhart 4-factor, Fama French 5-factor, and Fama French 6-factor models. Results show that selected cryptocurrencies have no exposure to stock market, macroeconomic factors, currencies and commodities. The cryptocurrency returns can be predicted by factors momentum and investor attention.
Similarly, Dempere (Citation2019) studied the predictive impact of Google trend values, Chinese Yuan exchange rates, S&P500 index, gold, and oil returns on Bitcoin, Ethereum, and Ripple by employing the PGARCH, EGARCH, TGARCH, and GARCH models. Results show that the returns of each selected cryptocurrency have a significant impact on each other. The impact of Google trend values of the search terms “Bitcoin” and “Ripple,” are significant however it is not the case for “Ethereum.” The returns of the exchange rate of the Chinese Yuan are significant as well. Returns of oil prices have a significant effect on Ethereum and Bitcoin however, it is not the case with Ripple. Returns of the S&P500 index are significant for selected cryptocurrencies whereas returns of gold have no significant impact on the selected cryptocurrencies. Returns of oil are significant with Bitcoin only.
Georgoula et al. (Citation2015) used time-series analysis to study the impacts of economic variables, technological factors, and mood variables on Bitcoin prices. Bitcoins circulation is used as the total stock of money supply, S&P500 is used as the general state of the global economy. Twitter feeds, Wikipedia search queries, and hash rates are used as sentiment proxies. Wikipedia search is associated with the public interest in Bitcoin, and the hash rate is associated with the mining difficulty. They performed sentiment analysis on daily data by using Support vector machines (SVMs). SVMs are a set of methods used for classification, outlier detection, and regression. The results of a series of short-run regressions indicate that the Twitter sentiment ratio is positively correlated with Bitcoin prices and the number of Wikipedia search queries and the hash rate have a positive impact on Bitcoin prices. The Bitcoin price is positively associated with the number of Bitcoins in circulation and negatively associated with the S&P 500 stock market index. Bitcoin is negatively affected by the exchange rate between the euro and the USD.
The findings related to macroeconomic and financial factors are mixed. A possible explanation can be the selection of different variables, periods, and methods. The results show that investor sentiment-related variables like investor Google search trends have impacts on the cryptocurrencies. In general, the findings of Dempere (Citation2019) Liu and Tsyvinski (Citation2018) indicate that different cryptocurrencies have similar characteristics and determinants.
The covid-19 outbreak and volatility spillovers between the assets
To create the optimal portfolio, it is important to investigate not only the impact of the investor sentiment but also volatility spillovers between Bitcoin and other assets. Although the main focus of this paper is not the volatility spillover, it is better to give an overview of the literature that focused on the volatility spillovers between Bitcoin and other assets during the Covid-19 outbreak so that the price dynamic of Bitcoin during the Covid-19 period can be presented from different perspectives. It is important to understand the volatility spillover transmission between Bitcoin and other asset classes during the COVID-19 outbreak to adjust the asset allocation to meet the risk diversification goals.
The COVID-19 outbreak affected the price dynamics of many asset classes. international Brent oil prices declined substantially from $66.5/barrel on January 1, 2020, to around $18/barrel on April 22, 2020, one of the largest drops in 20 years (Yousaf et al. Citation2021). Yousaf et al. (Citation2021) analyzed the return and volatility transmissions between oil and Bitcoin before and during the COVID-19 periods. The results show that the return transmissions are observed between oil and Bitcoin. There is a significant volatility spillover from Bitcoin to oil during the pre-COVID-19 period, whereas no evidence of volatility spillover between oil and Bitcoin is shown during the COVID-19 period. Yousaf and Ali (Citation2021) analyzed the return and volatility spillovers between S&P 500 and cryptocurrencies (Litecoin, Bitcoin, and Ethereum) before and during the COVID-19 periods. The results of this study show that the return and volatility spillovers are not significant during and before the COVID-19 periods whereas there is a unidirectional return transmission from S&P 500 to all the cryptocurrencies during the COVID-19 period. The volatility spillover is unidirectional from S&P500 to Litecoin and the volatility spillovers are not significant between S&P 500-Bitcoin and other two cryptocurrencies during the COVID-19 period. Similarly, Hsu, Sheu, and Yoo (Citation2021) analyzed volatility spillover effects between cryptocurrency and traditional currency and gold markets during the whole sample period and also during the COVID-19 outbreak. The results show that there are significant volatility spillover effects between cryptocurrency and traditional currency or gold markets during and before the COVID-19 outbreak. The findings of these studies reveal that there are volatility spillovers between Bitcoin and some asset classes during the Covid-19 period suggesting that it is better to adjust the investments in Bitcoin for the portfolios consisting of Bitcoin and S&P 500, Bitcoin and gold, and Bitcoin and traditional currency. The hedging strategies are affected by the impact of the Covid-19 pandemic because Bouri, Gkillas, and Gupta (Citation2020) studied the correlation between US stock returns and Bitcoin returns taking into account the trade uncertainty of the US. The results reveal that the realized correlation is negatively impacted by increases in trade uncertainty implying that Bitcoin can be used as a hedging instrument to the stock market. In conclusion, Bitcoin can be seen as a hedging instrument before the pandemic however the connectedness dynamic between the stock market and Bitcoin changed after the pandemic.
As far as known, the impact of the investor sentiment on Bitcoin taking into account the Covid-19 pandemic has not been studied yet, however, there are some recent studies focused on the impact of investor sentiment on stock markets. As Naseem et al. (Citation2021) state that mental health aspects of the COVID-19 outbreak have affected individual lives as well as the financial markets therefore it is interesting to study the impact of investor sentiment on cryptocurrency markets focusing on Bitcoin as the leading cryptocurrency. Naseem et al. (Citation2021) analyzed investor psychology and stock market behavior during COVID-19 by employing principal component analysis. For this study, Shanghai, Nikkei 225, and Dow Jones stock markets are selected. The results showed that investor psychology was negatively related to those stock markets during the pandemic outbreak and pessimistic emotions caused significant decreases in stock returns.
Data
In this section, the calculation of the returns, descriptive statistics, and diagnostic tests are given. This section is divided into two subsections: data for the estimations in GARCH models and the VAR model.
Data for GARCH models
There is no perfect proxy for the investor sentiment therefore three different proxies are used to check if different investor sentiment proxies give similar results. The first investor sentiment proxy is Bitcoin trading volume. Uygur and Tas (Citation2012) use trading volume changes of market indices to study the impact of investor sentiment on stock prices. For this research trading volume of Bitcoin is used for the investor sentiment proxy since Ankenbrand and Bieri (Citation2018) find that cryptocurrencies showed the highest resemblance to the stock market of all other examined assets. Baker and Stein (Citation2004) argue that when a market is unusually liquid, the price is dominated by irrational investors. The market liquidity can be measured by the trading volume. The daily opening prices and volumes of Bitcoin are obtained from yahoo finance. Daily Bitcoin opening prices and trading volumes are acquired over the period from January 2014 to August 2020.
The second proxy is the daily Crypto Fear & Greed Index is acquired over the period from February 2018 to August 2020 and delivered by alternative.me. The Bitcoin Fear and Greed Index (fear&greed) is an important crypto metric that represents the emotional state of the crypto markets. The fear and greed index is based on the premise that excessive fear can result in Bitcoin prices are far below their intrinsic values while greed can result in Bitcoin prices are far above what they should be worth. This index is a good proxy for Crypto investor sentiment but it has a disadvantage that this index is available only from 2018.
The third proxy is the weekly American Association of Individual Investors Index (AAII) is acquired over the period from November 2013 to August 2020 and obtained from ycharts.com. The AAII Investor Sentiment Survey has based on the mood of individual investors. It is the short-term outlook of the investors for the stock prices. This index is given in percentages.
Bitcoin returns, Bitcoin trading volatility index, and fear&greed index are calculated based on the following formula:
where
is Bitcoin price, volume or index value at time t.
Data is divided into 3 periods: all periods, before March 2020, and from March 2020 data to study the possible impact of the pandemic. Descriptive statics and ADF p-values for three proxies are given in the following tables ().
Table 1. Descriptive statistics and ADF test of bitcoin returns and volumes.
Table 2. Descriptive statistics and ADF test of fear & greed index.
Table 3. Descriptive statistics and ADF Test of AAII index.
For three periods, Bitcoin returns have a mean close to zero and exhibit negative skewness, suggesting a slight prevalence of negative returns. The normality is tested by the Jarque-Bera normality test. The null hypothesis of a normal distribution is strongly rejected. The student-t distribution is used to account for the non-normality. Positive excess kurtosis indicates a fat-tailed distribution and negative skewness indicates that the mean of the returns is less than the median, and the returns’ distribution is left-skewed. The augmented Dickey-Fuller test (ADF) tests the null hypothesis that a unit root is present in the series. Hence, the conclusion based on the ADF is that the series of all returns do not have a unit root and are therefore assumed to be stationary.
Data for the VAR model
Monthly data is obtained from data.oecd.org over the period from February 2018 to August 2020. Descriptive statics for the macro-economic variables, bitcoin returns, and feer&greed index are given in . The following variables are used:
Table 4. Descriptive statistics of the VAR model variables.
The monthly percent change in the industrial production index (IPI) as an economic growth proxy.
One-month risk-free rate (RF)
S&P500 returns (MR) as market return proxy:
The monthly percent change in the consumer price index (CPI).
The fear&greed index an investor sentiment proxy The feer&greed index is chosen as the investor sentiment proxy because this index is based on the moods of crypto investors therefore it can be more representative.
Methodology
In this section, econometrics models are given. This section is divided into two subsections: the GARCH models and the VAR model. In this paper, three are three investor sentiment proxies, five GARCH models, and three different periods. To prevent 45 different estimations, first of all, the best GARCH model will be chosen based on the Bitcoin volatility proxy. After chosen the best model, the estimation will be made based on different investor sentiment proxies and periods.
GARCH models
Engle (1982) introduced the ARCH model for estimating the conditional variance with dynamic properties. Bollerslev (Citation1986) developed the GARCH model that is an autoregressive moving average model based on the weighted average of past squared residuals. The GARCH(1,1) model is included to check if extensions of the basic GARCH model perform better than the basic GARCH model. Katsiampa (Citation2017) concluded the CGARCH model is the best model to estimate Bitcoin return volatilities therefore the CGARCH model is chosen in this paper as well however Katsiampa (Citation2017) didn’t include the investor sentiment variable in GARCH models. In financial series, the impact of bad news and the good news is asymmetric. It is often observed that volatility increases when negative asset returns are observed and this effect is commonly known as the “leverage effect” (Engle and Ng Citation1993). The asymmetry effect is not included in the basic GARCH model. To study the impact of the asymmetric volatility effects, EGARCH, GJR-GARCH, and AP-ARCH models are employed. Estimated results are compared to investigate which model performs the best and to check whether selected GARCH models give consistent results.
The GARCH (1) model
The GARCH(1,1) model does not account for asymmetry in the data and only describes the variance of the error term as a function of the magnitudes of previous error terms and their variances. However, the variance equation is different for each GARCH model therefore the mean equation is explained only in the subsection, GARCH Models. The investor sentiment variable is included in the mean and variance equations to investigate the impact of investor sentiment on Bitcoin returns and its volatility. The mean equation of the GARCH(1,1) model is given as follows:
(1)
(1)
Where is the return,
is a constant.
is the one day lagged investor sentiment variable with its coefficient
indicating the effect of investor sentiment on the return. Lagging of independent variables is often necessary in order for the model to be able to predict what will be the effect of the independent variable in period t based on knowledge of what happened in period t-1.
is an error term and
denotes all past returns observations. The error term follows the Student's t-distribution. The variance equation of GARCH(1,1) model is given as follows:
(2)
(2)
Where and
denote the ARCH and GARCH coefficients, and
indicates the effect of investor sentiment on the conditional volatility. For the requirement of volatility stationarity, it must be the case that
The CGARCH (1) model
Engle and Lee (Citation1993) generalized the basic GARCH model to a component GARCH model that assumes that the volatility consists of two components: transitory (short-term) volatility and permanent (long-term) volatility. The CGARCH model allows the sign and the size of the standardized residual to have separate effects on the volatility. The variance equation and the specification of are given as follows:
(3)
(3)
(4)
(4)
Where is a permanent element to capture the time-varying trend in volatility and it converges slowly constant
with powers of
since this parameter is often between 0.99 and 1.
is the intercept,
is the transitory component ARCH term,
is the transitory component GARCH term. Stationarity condition:
is the permanent component autoregressive term and
is the permanent component shock term. IS is the investor sentiment variable with its coefficient
indicating the effect of investor sentiment on the conditional volatility.
The EGARCH (1) model
The EGARCH model proposed by Nelson (1991) uses the natural logarithmic dependent variable that allows for a positive sign of the variance without imposing restrictions on the coefficients. Another advantage of this model is the capture of the asymmetric effect on the variance caused by negative and positive news. The leverage effect can be explained by the fact that negative shocks will have a greater impact on volatility than positive shocks (Nelson 1991). The variance equation of the EGARCH (1) model is given in the following equation.
(5)
(5)
Where and
since the error term follows the Student's t-distribution. the process is stationary if
(Enders, 2010). The coefficient
displays the degree of asymmetry. If
the model is perfectly symmetric. If γ < 0 negative shocks will increase the volatility more than positive shocks. If γ > 0 positive shocks increase the volatility more than negative shocks.
The GJR-GARCH (1) model
To incorporate the leverage effect, the asymmetric GJR-GARCH model is developed by Glosten, Jagannathan & Runkle (Citation1993). A leverage variable and an indicator variable added in the variance equation of the GARCH(1,1) model. The variance equations of GJR-GARCH(1,1) model is given as follows:
(6)
(6)
is 1 when the return of the period
is below the mean return and
is 0 when the return of the period
is above the mean return so that the impact of bad news and good news can be checked. For the persistence of the variance equation, it is required that
For the positive coefficient of
it is required that
Significant
term indicates that asymmetric model is the best. The sign of the leverage effect is the opposite compared to the EGARCH. If
no asymmetric volatility. If γ > 0 negative shocks will increase the volatility more than positive shocks. If γ < 0 positive shocks increase the volatility more than negative shocks.
The AP-ARCH (1) model
Ding, Granger, and Engle (Citation1993) introduced the AP-ARCH model that changes the second order of the error term into a more flexible varying exponent and includes asymmetric volatility effect. The variance equations of the AP-ARCH(1,1) model is given as follows:
(7)
(7)
The stationarity conditions of the AP-ARCH model are: and
The sign of the leverage effect is the same as the EGARCH model. If
the model is perfectly symmetric. If γ < 0 negative shocks will increase the volatility more than positive shocks. If γ > 0 positive shocks increase the volatility more than negative shocks.
The VAR model
Investor sentiment regression
The main purpose of using the VAR model is the making distinction between rational and irrational sentiment components based on the method used by Baker and Wurgler (Citation2006). The irrational sentiment component is derived from the following regression.
(8)
(8)
Where is investor sentiment,
represents market return,
represents consumer price index,
represents industrial production index,
is the error term. The residuals of this regression model represent the component of the investor sentiment that can not be explained by economic variables.
are parameters to be estimated.
The VAR model
The VAR model is used to estimate the link between the Bitcoin returns, rational sentiment, and irrational sentiment. The unrestricted VAR model is given as follows:
(9)
(9)
Where is a vector of endogenous variables,
is vector of constants,
are estimated coefficient matrices,
is vector of innovations.
The Granger causality
The Granger causality test proposed in 1969 by C. Granger to determine whether one variable is useful in forecasting another variable. Variable 1 is defined as having Granger causality on variable 2 if the given previous information of variable 2, as well as the past values of variable 1, enable to forecast the current value of variable 2. The null hypothesis is to test that the joint coefficients are equal zero, including the lagged values. The procedure for the Granger causality test is to store all values in the VAR model and then calculate and report small sample F statistics for the null hypotheses.
Results
The structure of the results section is similar to the structure of the methodology section. The first subsection contains the results based on the GARCH model and the second subsection contains the results based on the VAR model.
GARCH models
In the first section estimations of GARCH models based on the trading volume proxy will be given to select the best model and to compare whether different models give similar results.
Selection of the best GARCH model
gives the estimation results for GARCH models. Coefficient is significant in all GARCH models at the 1 percent significance level except the EGARCH model which is not significant even at the 10 percent level. A significant positive investor sentiment coefficient indicates that there is a positive impact of the investment sentiment on the Bitcoin returns which implies that when investor sentiment increases, then Bitcoin returns increase. Significant positive investor sentiment impact on the return is in line with the findings of Georgoula et al. (Citation2015), Nasir et al. (Citation2019), Figá-Talamanca and Patacca (Citation2019),Liu and Tsyvinski (Citation2018) and Dempere (Citation2019).
Table 5. Results of bitcoin data.
Coefficient is significant in all GARCH models indicating that there is a positive impact of the investment sentiment on the conditional volatility of Bitcoin returns that implies that when investor sentiment increases, then conditional volatilities of Bitcoin returns increase. Significant positive investor sentiment impact on the conditional volatility is in line with the findings of Lopez-Cebarcos et al. (Citation2019), Figá-Talamanca and Patacca (Citation2019), and Eom et al. (Citation2019).
The log-likelihood is higher for richer models, so based on the log-likelihood, the EGARCH(1,1) model is the best. When model fits are ranked according to their AIC and BIC values, the model with the lowest value being considered to be the best. Based on the AIC and BIC selection criteria, the EGARCH(1,1) model is the best model. In conclusion, based on these three criteria, EGARCH(1,1) performs and this disproves the results of Katsiampa (Citation2017) who found the optimal heteroscedasticity model for Bitcoin returns to be the CGARCH model. The inclusion of investor sentiment variable and different samples may be the explanation of this difference. The EGARCH model is used for the estimations based on other two investor sentiment proxies because the estimations based on all GARCH models are similar and the EGARCH is the best model.
gives p-values of Box-Ljung and Li-MAK tests. The residuals of the estimations are tested for autocorrelation by utilizing the Ljung- Box test and there is no information to suggest that there is significant autocorrelation in the Bitcoin returns at the 10 percent level. The squared residuals are tested for remaining ARCH effects not captured by the variance equation using the Li-Mak test. Li-Mak test results indicate that models appear to be correctly specified in terms of ARCH and GARCH terms in the variance equation as there is no evidence to suggest that there remain significant ARCH effects in either series of returns at the 1 percent level.
The overview of the volatility stationarity requirements is given in . All requirements are satisfied except the GJR-GARCH model for which the requirement for the persistence of the variance equation to be less than one is not met since is bigger than 1. This indicates that variance model of the GJR-GARCH model is not valid. Box-Ljung test, Li-MAK test and stationarity checks confirm that the models are correctly specified except the GJR-GARCH model.
measures the persistence in the conditional volatility. The relatively high significant coefficient indicates persistent volatility clustering meaning that the expected volatility in future periods will be influenced by volatility shocks that happen today.
Table 6. Stationarity checks of the GARCH models for bitcoin.
The asymmetry factors are non-zero and statistically significant indicating the presence of asymmetry. The asymmetry factors of EGARCH and AP-ARCH models are strongly positive and statistically significant. This finding is not consistent with the findings of Baur, Hong, and Lee (Citation2018) because they analyzed the majority of the 20 cryptocurrencies but found weak evidence for Bitcoin. Strongly positive and significant asymmetry factors of EGARCH and AP-ARCH models indicate that positive shocks have a greater impact on the volatility than negative shocks of the same magnitude. That can be attributed to the fear of missing out (FOMO) behavior of speculative investors. The FOMO concept was first identified and named in 2000 by Dr. Dan Herman. In particular, in this paper, FOMO refers to the fear that a Bitcoin investor feels by missing out on a potentially profitable Bitcoin investment or trading opportunity. The FOMO feeling is particularly prevalent when Bitcoin prices rise in value significantly over a relatively short time. In conclusion, the results show that the Bitcoin market is driven more by emotions rather than rationality, so FOMO is a huge factor to consider when trading in Bitcoin. This finding is in line with the findings of Naeem, Mbarki, and Shahzad (Citation2021) that the happiness sentiment index has more predictive power than the fear index in the determination of returns of selected cryptocurrencies. The happiness sentiment index can be seen as good news and the fear index can be seen as bad news.
The impact of the covid-19 outbreak
The results of the previous subsection show that the EGARCH model is the best. The EGARCH model is used to analyze the impact of the Covid-19 outbreak based on other investor sentiment proxies. Results based on the Bitcoin volatility, fear&greed, and AAII proxies are given in respectively for three different periods: all periods, before and after the Covid-19 outbreak. The stationarity condition is satisfied for all cases because the residuals of the estimations are tested for autocorrelation by utilizing the Ljung- Box test and there is no information to suggest that there is significant autocorrelation. In the case of Bitcoin trading volatility and AAII proxies, AR(1) is included so that the estimations of the after period are stationary. Li-Mak test results indicate that models appear to be correctly specified in terms of ARCH and GARCH terms in the variance equation as there is no evidence to suggest that there remain significant ARCH effects.
Table 7. Results based on the bitcoin volatility proxy.
Table 8. Results Based on the fear&greed Proxy.
Table 9. Results based on the AAII Proxy.
The investor sentiment coefficient is significant and positive for after the Covid-19 outbreak period based on all three investor sentiment proxies which indicates that there is a positive significant impact of the investment sentiment on the Bitcoin returns.
is significant and higher compared to other periods indicating that there is a positive impact of the investment sentiment on the conditional volatility of Bitcoin returns as well. The impact of investor sentiment on Bitcoin returns and conditional volatilities is stronger after the Covid-19 outbreak. This significant impact of investor sentiment on the volatility is more present after the Covid-19 outbreak. This can be an indication of a triggered irrational investment behavior toward Bitcoin due to the COVID-19 outbreak. This finding is in line with the findings of Naseem et al. (Citation2021) that psychological aspects of the COVID-19 outbreak have affected financial markets and caused drastic price changes.
Asymmetric volatility effects are investigated as well. It is found that asymmetric volatility effects are present indicating that bad news and good news don’t have the same impact. The signs of significant asymmetry factors indicate that good news has more impact than bad news in whole period and before the covid-19 outbreak period based on the Bitcoin trading volatility and the fear&greed proxies. This can be related to the hedging and safe haven property of Bitcoin as indicated by the paper of Bouri, Gkillas, and Gupta (Citation2020). However, the signs of significant asymmetry factors are negative for estimation made for after the Covid-19 outbreak data. The use of Bitcoin as a hedging instrument is affected by the Covid-19 pandemic. A possible explanation is panic-related investor behavior. This is in line with the findings of Naseem et al. (Citation2021) that pessimistic emotions caused significant decreases in stock returns.
The findings of this paper also support return transmission and the volatility spillover findings of Yousaf et al. (Citation2021), Yousaf and Ali (Citation2021), and Hsu, Sheu, and Yoo (Citation2021). Return and volatility transmissions are observed between cryptocurrencies and other assets during the Covid-19 period and this study shows that there is a significant impact of the investor sentiment on Bitcoin price and its volatilities. The findings are related because the transmission between the assets, the drastic price declines in markets triggered irrational investor behavior, and impacted the price dynamics of Bitcoin. To present the impact of irrational investor behavior in detail, the results of the VAR model will be explained in the following subsection.
The VAR model
First of all, variables are tested for unit roots by conducting the ADF test. All variables don’t have unit roots (). The irrational investor sentiment variable is created from the regression model. shows the results of the regression model where the market return is lagged in this way to attempt to capture the reaction of the S&P 500 returns to investor sentiment. The selected lag order is 1 based on the Hannan-Quinn information criterion (). The VAR model satisfies the stability condition because the roots are less than 1 () and the LM test confirms that there is no autocorrelation which confirms the correct specification of the VAR model (). The VAR model estimations are given in . Both VAR estimation and VAR Granger Causality/Block Exogeneity Wald tests () show that both the changes of rational and irrational sentiments are likely to cause the changes of Bitcoin returns at significance levels of 1%.
Table 10. VAR granger causality tests.
Conclusions
This paper aimed to study the impact of the investor sentiment on the Bitcoin returns and conditional volatility taking into account the impact of the Covid-19 pandemic by using three different proxies for the investor sentiment to check if different investor sentiment proxies give similar results. The first proxy is the Bitcoin trading volume which is used to determine the best GARCH model from GARCH, CGARCH, EGARCH, GJR-GARCH, and AP-ARCH models. In particular, EGARCH, GJR-GARCH, and AP-ARCH models are selected to investigate asymmetric volatility effects. The results show that the EGARCH model outperforms better than other selected models based on the log-likelihood, AIC, and BIC selection criteria. Other proxies for investor sentiment are the Crypto Fear & Greed index and the American Association of Individual Investors index. Estimations based on these two proxies are made by employing the EGARCH models hence it was chosen as the best model. Estimation results show that investor sentiment has a positive impact on the Bitcoin returns and conditional volatility, especially after Covid-19. The positive impact of investor sentiment can be attributed to the fear of missing out (FOMO) behavior of speculative and irrational investors. In this paper, FOMO particularly refers to the fear that a Bitcoin investor feels by missing out on a potentially profitable Bitcoin investment or trading opportunity.
The VAR model is employed to investigate whether investor sentiment and Bitcoin returns are related in a dynamic setting and to make distinguish between rational and irrational investor sentiments. The results from the VAR model and VAR Granger Causality tests indicate that both rational and irrational investor sentiments have an impact on Bitcoin returns.
Asymmetric volatility effects are investigated as well. It is found that asymmetric volatility effects are present indicating that bad news and good news don’t have the same impact. The signs of significant asymmetry factors indicate that good news has more impact than bad news in all periods and before the covid-19 outbreak based on the Bitcoin trading volatility and the fear&greed proxies. Significant positive investor sentiment coefficients, leverage effect estimates indicate that the good news has more impact on Bitcoin prices than the bad news that can be attributed to the FOMO behavior of speculative and irrational investors. However, the sign of the leverage effect is negative after the Covid-19 outbreak which indicates that panic-related investor behavior was present in the markets due to Covid-19 pandemic-related uncertainties. In conclusion, the Bitcoin market is also driven by emotions and volatility transmissions are observed between cryptocurrencies and other assets during the Covid-19 period (Yousaf et al. Citation2021), Yousaf and Ali (Citation2021), and Hsu, Sheu, and Yoo (Citation2021)).
The results from this paper can have multiple applications. For governments and investors, it is important to know that irrational investor sentiment has an impact on Bitcoin returns and its volatility especially during the era of the Covid-19 pandemic. When investor sentiment plays an important role in the determination of an asset, the current value of this asset may not reflect the real value therefore instructions and investors may have to face unexpected losses. Also, investors might benefit from having a better idea of how cryptocurrency markets react during a crisis period like a pandemic. Knowing what happens to volatility is also important because volatility is the risk measure and all investment and hedging strategies depend on this information. Investors should take into account the impact of irrational investor behavior and transmissions between Bitcoin and some asset classes and readjust their portfolios to meet the risk diversification goals.
References
- Ankenbrand, T., and D. Bieri, 2018. “Assessment of Cryptocurrencies as an Asset Class by Their Characteristics.” Investment Management and Financial Innovations 15 (3):169–81. doi:10.21511/imfi.15(3).2018.14
- Baker, M., and J. Stein. 2004. “Market Liquidity as a Sentiment Indicator.” Journal of Financial Markets 7 (3):271–99.
- Baker, M., and J. Wurgler. 2006. “Investor Sentiment and the Cross-Section of Stock Returns.” The Journal of Finance 61 (4) :1645–80. doi:10.1111/j.1540-6261.2006.00885.x
- Baur, D., K. Hong, and A. D. Lee. 2018. “Bitcoin: Medium of Exchange or Speculative Assets?” Journal of International Financial Markets, Institutions and Money 54:177–89. doi:10.1016/j.intfin.2017.12.004
- Bollerslev, T. 1986. “Generalized Autoregressive Conditional Heteroskedasticity.” Journal of Econometrics 31 (3):307–27.
- Bouri, E., D. Gabauer, R. Gupta, and A. K. Tiwari. 2021. “Volatility Connectedness of Major Cryptocurrencies: The Role of Investor Happiness.” Journal of Behavioral and Experimental Finance 30 (1):100463. doi:10.1016/j.jbef.2021.100463
- Bouri, E., K. Gkillas, and R. Gupta. 2020. “Trade Uncertainties and the Hedging Abilities of Bitcoin.” Economic Notes 49 (3). doi:10.1111/ecno.12173
- Brown, G. W. 1999. “Volatility, Sentiment, and Noise Traders.” Financial Analysts Journal 55 (2):82–90. doi:10.2469/faj.v55.n2.2263
- Bukovina, J., and M. Marticek. 2016. “Sentiment and Bitcoin Volatility.” In MENDELU Working Papers in Business and Economics 2016-58. Mendel University in Brno, Faculty of Business and Economics. doi:10.1016/j.frl.2019.101399
- Dempere, J. 2019. “Factors Affecting the Return and Volatility of Major Cryptocurrencies.” 2019 Sixth HCT Information Technology Conference. doi:10.1109/ITT48889.2019.9075117
- Engle, R., and G. Lee. 1993. “A Permanent and Transitory Component Model of Stock Return Volatility.” Working Paper No. 92-44R.
- Engle, R. F., and V. K. Ng. 1993. “Measuring and Testing the Impact of News on Volatility.” The Journal of Finance 48 (5):1749–78.
- Eom, T., T. Kaizoji, H. K. Sang, and L. Pichl. 2019. “Bitcoin and Investor Sentiment: Statistical Characteristics and Predictability.” Physica A: Statistical Mechanics and Its Applications 514:511–21. doi:10.1016/j.physa.2018.09.063
- Figá-Talamanca, G., and M. Patacca. 2019. “Does Market Attention Affect Bitcoin Returns and Volatility?” Decisions in Economics and Finance 42 (1):135–55. doi:10.1007/s10203-019-00258-7
- Foley, S., J. R. Karlsen, and T. J. Putniņš. 2019. “Sex, Drugs, and Bitcoin: How Much Illegal Activity is Financed through Cryptocurrencies?” The Review of Financial Studies 32 (5):1798–853. doi:10.1093/rfs/hhz015
- Georgoula, I., D. Pournarakis, C. Bilanakos, D. Sotiropoulos, and G. M. Giaglis. 2015. “Using Time-Series and Sentiment Analysis to Detect the Determinants of Bitcoin Prices.” Development Economics: Macroeconomic Issues in Developing Economies eJournal. Proc. Mediterranean Conf. Inf. Syst. (MCIS), p. 20. doi:10.2139/ssrn.2607167
- Glosten, L. R., R. Jagannathan, and D. E. Runkle. 1993. “On the Relation Between the Expected Value and the Volatility of the Nominal Excess Return on Stocks.” The Journal of Finance 48 (5):1779–801.
- Hsu, S., C. Sheu, and J. Yoo. 2021. “Risk Spillovers between Cryptocurrencies and Traditional Currencies and Gold under Different Global Economic Conditions.” The North American Journal of Economics and Finance 57 (2):101443. doi:10.1016/j.najef.2021
- Katsiampa, P. 2017. “Volatility Estimation for Bitcoin: A Comparison of GARCH Models.” Economics Letters 158:3–6. doi:10.1016/j.econlet.2017.06.023
- Liu, Y., and A. Tsyvinski. 2018. “Risks and Returns of Cryptocurrency.” NBER Working Paper 34 (6):2689–727.
- Lopez-Cebarcos, M. A., A. M. Perez-Pico, J. Pineiro- Chousa, and A. Sevic. 2019. “Bitcoin Volatility, Stock Market and Investor Sentiment. Are They Connected?” Finance Research Letters 38 (1). doi:10.1016/j.frl.2019.101399
- Naeem, M. A., I. Mbarki, and J. Shahzad. 2021. “Predictive Role of Online Investor Sentiment for Cryptocurrency Market: Evidence from Happiness and Fears International.” International Review of Economics & Finance 73 (1):496–514. doi:10.1016/j.iref.2021.01.008
- Naseem, S., M. Mohsin, W. Hui, G. Liyan, and K. Penglai. 2021. “The Investor Psychology and Stock Market Behavior during the Initial Era of COVID-19: A Study of China, Japan, and the United States.” Frontiers in Psychology 12:626934. doi:10.3389/fpsyg.2021.626934
- Nasir, M. A., T. L. D. Huynh, S. P. Nguyen, and D. Duong. 2019. “Forecasting Cryptocurrency Returns and Volume Using Search Engines.” Financial Innovation 5 (1):1–13. doi:10.1186/s40854-018-0119-8
- Plakandaras, V., E. Bouri, and R. Gupta. 2020. “Forecasting Bitcoin Returns: Is There a Role for the US–China Trade War?” Journal of Risk 23 (3):75–93.
- Uygur, U., and O. Tas. 2012. “Modeling the Effects of Investor Sentiment and Conditional Volatility in International Stock Markets.” Journal of Applied Finance Banking 2 (5):239–60.
- Wang, G., Y. Tang, C. Xie, and S. Chen. 2019. “Is Bitcoin a Safe Haven or a Hedging Ssset? Evidence from China.” Journal of Management Science and Engineering 4 (3):173–88. doi:10.1016/j.jmse.2019.09.001
- Yermack, D. 2013. “Is Bitcoin a Real Currency? An Economic Appraisal.” NBER Working Paper. doi:10.3386/w19747
- Yousaf, I., and S. Ali. 2021. “Linkages between Stock and Cryptocurrency Markets during the Covid-19 Outbreak: An Intraday Analysis.” The Singapore Economic Review. doi:10.1142/S0217590821470019
- Yousaf, I., S. Ali, E. Bouri, and T. Saeed. 2021. “Information Transmission and Hedging Effectiveness for the Pairs Crude Oil-Gold and Crude oil-Bitcoin during the COVID-19 Outbreak.” Economic Research-Ekonomska Istraživanja 34:1–22. doi:10.1080/1331677X.2021.1927787
- Zhu, P., X. Zhang, Y. Wu, H. Zheng, and Y. Zhang. 2021. “Investor Attention and Cryptocurrency: Evidence from the Bitcoin Market.” PLOS One 16 (2):e0246331. doi:10.1371/journal.pone.0246331
Appendix
Table A1. ADF test results.
Table A2. Regression results.
Table A3. Lag selection.
Table A4. The VAR Model AR root tests.
Table A5. VAR residual serial correlation LM Tests.
Table A6. The VAR model estimations.