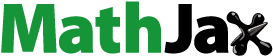
ABSTRACT
We present seminal empirical evidence on market-wide herding from historical markets for the St. Petersburg stock exchange between 1865 and 1914. Our findings indicate the presence of herding in Imperial Russia’s largest equity market, which tends to vary among industries and grow stronger during months of negative performance and declining volatility. Controlling for the 1893-reform that prompted wider social participation in equity trading, we find that herding surfaces exclusively in the post-reform years, with no evidence of herding arising pre-reform. Our results showcase that the behavior of investors in historical stock exchanges exhibits patterns similar to those of modern-day ones.
Introduction
Herd behavior is one of the most broadly reported trading patterns in financial markets (Chen Citation2013; Choi and Skiba Citation2015), with its presence often appearing relatively stronger – in terms of both magnitude and significance – among emerging and frontier markets, compared to their developed counterparts (Chang, Cheng, and Khorana Citation2000; Gelos and Wei Citation2005). This has largely been attributed to their incomplete regulatory designs, which tend to hamper their transparency and raise issues of availability and credibility for information-disclosure (Antoniou, Ergul, and Holmes Citation1997; Economou et al. Citation2015b); in addition, such markets are characterized by the substantial participation of domestic retail investors, who are (on average) of low sophistication (Barber et al. Citation2014) and prone to noise trading (Li and Wang Citation2010). However, it is important to acknowledge that emerging/frontier markets are not exclusively encountered during the post-1970s’ evolution of the global economic and financial environment. Indeed, the early financial markets established from the 17th century onward were also typified by issues similar to those of modern emerging/frontier markets (lack of transparency; rumor mongering; manipulation; insider trading; rudimentary institutional designs) and were dominated by company-insiders and retail investors with a strong speculative disposition (Kindleberger and Aliber Citation2005), suggesting that herding would be expected to be a likely feature of pre-20th century markets.
However, despite the large body of finance literature (Neal Citation1982; Galbraith Citation1994; Kindleberger and Aliber Citation2005; Dale, Johnson, and Tang Citation2005; Borodkin and Perelman Citation2011; Corzo, Prat, and Vaquero Citation2014; Bassino and Lagoarde-Segot Citation2015) covering equity markets from as early as the 17th until the early 20th centuries that has produced narrative evidence of investors’ herd behavior during various phases of those markets’ evolution, it is interesting to note that, no research to date has empirically verified the presence of market-wide herdingFootnote1 in stock exchanges during those centuries, despite the availability of recently-compiled databases reaching as far back as the 18th century (Goetzmann, Ibbotson, and Peng Citation2001) that have allowed research in historical capital markets to gain momentum over the past two decades.Footnote2 Our study addresses this issue by providing seminal empirical evidence on investors’ herding at the market-wide level from the St. Petersburg stock exchange drawing on month-end prices for the universe of firms listed there between January 1865 and July 1914 and tests for a series of research questions.
First, we test whether herding was significant in Imperial Russia’s prime equity market, in view of the dominance of nonprofessional investors in that market. Second, we explore whether herding exhibits variations across industries, in light of widespread evidence on industry-effects in herding from modern equity markets.Footnote3 Third, we examine the extent to which herding presents itself with asymmetries, by gauging whether herding varies (both for the whole market and individual industries) across different market/industry states (up/down market/industry months; increasing/decreasing market/industry volatility months).Footnote4 Fourth, we assess the impact over herding conferred by the regulatory reforms of the early 1890s in Russia’s financial sector that prompted the wider participation of individual investors in equity trading, in view of evidence on the propensity of this investor-type to engage in herding (Kumar and Lee Citation2006; Dorn, Huberman, and Sengmueller Citation2008; Kumar Citation2009; Burghardt Citation2011; Jame and Tong Citation2014; Li, Rhee, and Wang Citation2017) under the influence of behavioral biases (Barber, Odean, and Zhu Citation2009a, Citation2009b; Barber and Odean Citation2013).
Our results suggest that investors herded significantly in the St. Petersburg stock exchange throughout our sample period; their herding is asymmetric, appearing more frequently for months of falling market returns and decreasing market volatility. Herding is observed consistently for the Financial and Trade & Industrial industries for the full sample period and across several industry states; its presence for Railways and Steamships is confined to specific states of each industry (positive performance and low-volatility months for Railways; negative performance months for Steamships). Herding is present (absent) in the aftermath of (prior to) the 1893-reforms at the market-wide level as well as for Steamships and Trade & Industrial companies; no evidence of herding surfaces pre or post 1893 for Financials and Railways.
Our work contributes significantly to the historical finance literature, by offering empirical verification of herding at the market-wide level in a historical market for the first time in the literature; as mentioned earlier, this is important, in view of the fact that any reference to herding phenomena in 18th/19th century markets is of a narrative nature only (Neal Citation1982; Galbraith Citation1994; Kindleberger and Aliber Citation2005; Dale, Johnson, and Tang Citation2005; Borodkin and Perelman Citation2011; Corzo, Prat, and Vaquero Citation2014; Bassino and Lagoarde-Segot Citation2015). From a behavioral finance perspective, our findings confirm that herd behavior in earlier centuries’ stock markets bore features (industry effects; asymmetries) similar to those encountered in modern markets, thus suggesting that investors’ behavior has changed little over time. In addition, the fact that the bulk of herding is observed for the post-1893 years confirms extant legal-theoretical evidence (Gerding Citation2007; Hirshleifer Citation2008), according to which, the adoption of regulatory policies catering to the prevailing social mood can foment the emergence of herding phenomena in equity markets.
The rest of this paper is structured as follows: the second section discusses herding as a concept and its key sources and empirical evidence (in the section Herding) followed by an overview of the evolution of the St. Petersburg stock exchange in the 19th and early 20th centuries in the context of developments in the wider Russian economy (in the section St. Petersburg Stock Exchange); the third section presents the data utilized alongside some descriptive statistics and introduces the empirical design employed to test for herding. The fourth section discusses the results, and the fifth section presents a series of robustness checks. The sixth section provides some concluding remarks and highlights several implications of our study.
Theoretical background
Herding
Investors herd when they discard their private signals or fundamentals, choosing instead to mimic the behavior of others, following interactive observation of others’ actions or action-payoffs (Hirshleifer and Teoh Citation2003). Whether this behavior is intentional or not has constituted the subject of much research, the latter having identified a series of factors that can motivate intentional or spurious herding (Bikhchandani and Sharma Citation2001; Holmes, Kallinterakis, and Leite-Ferreira Citation2013; Gavriilidis, Kallinterakis, and Leite-Ferreira Citation2013a; Economou et al. Citation2015b; Galariotis, Rong, and Spyrou Citation2015).
Intentional herding is the product of an actual or perceived asymmetry in the market environment that encourages investors to copy their peers’ trades in order to reduce this asymmetry. The latter may be informational, in which case investors who are less informed or possess inferior information-processing skills may mimic the trades of those they consider better-informed, in anticipation of informational payoffs (Devenow and Welch Citation1996). In the extreme, if growing numbers of investors end up being less willing to rely on their private signals and choose to free-ride on their peers’ trades, instead, this will deplete the public pool of information and is likely to foment the emergence of informational cascades (Banerjee Citation1992; Bikhchandani, Hirshleifer, and Welch Citation1992). Many a time, however, investors herd intentionally due to asymmetries of a professional nature; this is the case of low-quality investment professionals (e.g., fund managers) tracking the trades of their high-quality peers in order to attain career/reputational payoffs (Scharfstein and Stein Citation1990; Dasgupta, Prat, and Verardo Citation2011; Jiang and Verardo Citation2018). Considering the relative performance evaluation to which fund managers are normally subject, less-able managers have an obvious incentive to mimic their better-able peers, in order to improve on their image (or, alternatively, conceal their low ability).
Herding, however, need not necessarily be intentional; evidence suggests it is often the result of investors’ exposure to a common factor motivating correlation in their trades. This may be due to relative homogeneity (Teh and De Bondt Citation1997), whereby similarities among investment professionals (e.g., in terms of their education/qualifications, the indicators they analyze and their processing, as well as their regulatory framework) prompt similarities in their trades. What is more, correlation in investors’ trades can arise if investors’ information sets are correlated (investigative herding; Froot, Scharfstein, and Stein Citation1992), if they follow similar investment strategies (style investing; Barberis and Shleifer Citation2003), or if they chase popular sectors (fads; Brunnermeirer and Nagel Citation2004). In addition, investors can exhibit correlation in their trading behavior as a result of the impact of behavioral biases and heuristics (Barber, Odean, and Zhu Citation2009a, Citation2009b), which motivate tacit coordination of their trades.
From an empirical perspective, herding has been confirmed internationally at both the micro and macro levels. At the micro level, there exists widespread evidence of institutional investors engaging in such a practice across several markets, developedFootnote5, emergingFootnote6 and frontierFootnote7 ones, with herding tending to appear more frequently among the latter two; evidence of stronger herding among emerging/frontier markets has also emerged from research at the macro-level.Footnote8 Research (Gelos and Wei Citation2005) has argued that this is likely due to the incomplete institutional designs of emerging/frontier markets that render their transparency lower and increase their informational ambiguity, thus prompting investors to seek informative signals in the trades of their peers. What is more, herding has further been found to present itself with size (often appearing significant among the smallestFootnote9 or largestFootnote10 stocks), industryFootnote11 and asymmetricFootnote12 effects internationally; herding has also been found to be affected by financial crisesFootnote13, while its cross-market dynamics have also been confirmed.Footnote14 It is also worth noting here that herding has been reported at the micro level among retail investors (Kumar and Lee Citation2006; Dorn, Huberman, and Sengmueller Citation2008; Kaniel, Saar, and Titman Citation2008; Barber, Odean, and Zhu Citation2009a, Citation2009b) as well; what is more, several studies have attempted to identify the extent to which herding is intentional or spurious, both at the micro (Holmes, Kallinterakis, and Leite-Ferreira Citation2013; Gavriilidis, Kallinterakis, and Leite-Ferreira Citation2013a; Economou et al. Citation2015b; Celiker, Chowdhury, and Sonaer Citation2015) and macro (Galariotis, Rong, and Spyrou Citation2015; Cui, Gebka, and Kallinterakis Citation2019) levels.
St. Petersburg stock exchange
The establishment of stock exchanges in Imperial Russia lagged behind other developed economies of the time. The St. Petersburg stock exchange was the first to be founded in the country,Footnote15 with the stock exchanges of Arkhangelsk, Odessa, Warsaw and Moscow launched much later (Borodkin, Konovalova, and Perelman Citation2006). Prior to the 1830s, there were no regulatory provisions reigning over issues of public listing rules and transactions, with the then-extant regulations being primarily concerned with the timing of trading sessions and the role of specific professionals (brokers, notaries and auctioneers) in their process (Lizunov Citation2015). These years saw very limited activity in equity trading, with the bulk of transactions involving commodities and, to a lesser extent, ship insurance, currencies, and fixed-income instruments (Borodkin and Perelman Citation2011).
A factor key to the promotion of equity trading was the adoption of a corporate law in 1836, which incited several rounds of debates concerning its modernization for decades and remained in force until the 1917 Revolution. In essence, it stipulated the establishment of joint stock companies by concession (Borodkin, Konovalova, and Perelman Citation2006; Borodkin and Perelman Citation2011); according to this system, the incorporation-application of each company had to be submitted for review to the Ministry of Finance and, upon approval, signed into law by the Czar, in effect leading every corporate charter to become a separate law on its own. This concessionary legal system led to the advent of corporations in Imperial Russia, with the number of their incorporations retaining its upward trend in the following decades (with the exception of the 1880s)Footnote16, more so in the aftermath of the Crimean WarFootnote17 (which saw the evolution of several industries - financial; light industriesFootnote18; transportation - in the country).
To the extent that those newly incorporated companies vied for capital financing opportunities, a lot of them chose to list on the St. Petersburg stock exchange, leading to a sharp rise in public listings – and the exchange’s first major bubble in 1857–1858 (motivated by the trades of members of the state bureaucracy and the military; Owen Citation2013). As the uptrend in the number of listings on the stock market continued unabated, the stock exchange witnessed new surges in speculation in the 1868–1869 and 1871–1873 periods; although forward and futures trading on shares was proscribed since December 1836 (“Rules on Share-Issuing Companies”), its unofficial practice was reported to be commonplace and this prompted discussions among officials (both in the Ministry of Finance and the St. Petersburg stock exchange) in September 1869 as per the treatment of this speculative fervor (Lizunov Citation2015).
The 1890s witnessed the economic revival of Russia as well as an acceleration of its industrialization process, largely supported by an influx of European investment capital (Owen Citation2013). This economic/industrial boom led to an exponential growth in new companies’ incorporations (Borodkin and Perelman Citation2011) and listings on the St. Petersburg stock exchange, where a phenomenal price-rally kickstarted by late 1893. This rally was fueled by a confluence of factors, including the decline in government bond yields from 5% to 4% (which prompted investors to enter the stock market in search of higher returns), the adoption of the gold standard in 1895–1897 (which endowed foreign investors with enhanced confidence in Russian business, as it shielded them from the highly volatile rouble) and the extended line of credit supplied by banks to their customers enabling them to purchase shares (“on call” funds; see Borodkin and Perelman Citation2011 for a more detailed presentation of this tool).Footnote19
However, research (Goetzmann and Huang Citation2018) suggests that key to the commencement of the rally in late 1893 was the ratification of two laws on July 8th, 1893 (“On the Prohibition of Certain Transactions in the Buying and Selling of Gold Currency, Bills of Exchange, and Suchlike Items of Value Priced in Gold Currency”; “On Certain Changes in the Resolutions Concerning the Stock Exchanges”), which, among other issues (see Lizunov Citation2015) allowed futures trading in equities. Although futures trading constituted unofficial practice till that point, its legalization prompted investors from a broader social cross sectionFootnote20 to enter equity trading, thus culminating in a surge of speculative activity from the Fall of 1893 onward and a concomitant rise in liquidity (Goetzmann and Huang Citation2018). The latter was observed more strongly among smaller capitalization stocks and allowed investors to witness a rise in their wealth, something tacitly confirmed via the significant momentum profits reported in Goetzmann and Huang (Citation2018) for the St. Petersburg stock exchange post-1893. This speculation led to wild price-fluctuations in the latter half of the 1890s and gripped investors in Russia for the most part of that decade, motivating the attention of authorities, who were concerned by its destabilizing potential.
In that respect, Temporary Rules for the Securities Department of the St. Petersburg Stock Exchange were issued in January 1900 to combat speculation, while June 27th, 1900 saw the ratification by the Czar of the “Order on the Formation of a Securities Department at the St. Petersburg Stock Exchange”, supplemented by a law “On the Responsibility of Individuals Introducing Securities into Circulation on the St. Petersburg Stock Exchange” (June 12th, 1902) and “Rules on the Listing of Securities in the Securities Department” approved by the Ministry of Finance on September 5th, 1902 (Lizunov Citation2015). This set of legislations led to the separation of equities from commodities trading on the St. Petersburg stock exchange, with equities trading now coming under the oversight of a special department of the Ministry of Finance (Borodkin and Perelman Citation2011). The presence of speculation, however, did not abate in the 1900s; although the Russo-Japanese war (1904–1905) and the 1905 Revolution led to the flight of capital from the country, Russian equities documented rather strong performance between 1902 and 1905 (Borodkin, Konovalova, and Perelman Citation2006). A temporary slump in November 1905 was followed by a price rebound in early 1906, only to be followed by a longer downmarket period that extended well into 1909; the final surge in stock prices prior to the First World war was reported from 1910 onward, amid a window of rapid economic growth (Borodkin, Konovalova, and Perelman Citation2006; Owen Citation2013).
Data and methodology
Our dataset was sourced from the website of the International Center for Finance at the Yale School of Management and comprises end-of-month closing prices of 543 firms listed in the St. Petersburg stock exchange between January 1865 and July 1914.Footnote21 To empirically assess the presence of herding, we rely on the approach proposed by Chang, Cheng, and Khorana (Citation2000), which infers herding via the relationship between the cross-sectional dispersion of equity returns and the absolute performance of the market. If securities’ pricing is rational, the relationship between the cross-sectional dispersion of returns and absolute market performance would be expected to be linear and positive; therefore, the cross-sectional return-dispersion would rise with absolute market returns, as securities’ sensitivity to market movements is not uniform (Black Citation1972). In the presence of herding, however, one would expect securities’ returns to track the market’s performance and exhibit clustering around the average market return. This would culminate in a decline for the cross-sectional return-dispersion and, assuming this herding to motivate extreme absolute market returns, the relationship between the cross-sectional dispersion of returns and absolute market performance would turn concave. Chang, Cheng, and Khorana (Citation2000) tested empirically for these possibilities drawing on the following specification:
(1)
(1)
In the specific context of our study, reflects the average performance of all actively traded stocks in the St. Petersburg stock exchange in month t;
is calculated as:
(2)
(2)
Here, n is the number of actively traded stocks in month t and the log-differenced return of stock i in month t. Assuming rationality in asset pricing, we would therefore (as mentioned above) expect
and
to be linearly and positively related, with the latter implying significantly positive (insignificant) values of
(
). In the presence of herding, the relationship between
and
would become concave (i.e. non-linear), the latter implying significantly negative values for
To assess whether herding varies between up and down markets, we utilize the following specification:
(3)
(3)
Here, = 1, if
is positive, zero otherwise; in this case, significantly negative values for
(
) would denote the existence of herding during months of positive (negative) average market performance.Footnote22
To gauge whether herding varies between months of increasing and decreasing market volatilityFootnote23, we employ the following specification:
(4)
(4)
Here, = 1 for months of increasing volatility, zero otherwise. We calculate volatility using the squared value of
similar to EquationEquation (3)
(3)
(3) , significantly negative values for
(
) would denote the existence of herding during months when volatility has increased (decreased) versus the immediately previous month.
To examine whether the regulatory reforms of 1893 conferred an effect over the presence of herding, we rely on the following specification:
(5)
(5)
where
= 1 for the period after July 1893, zero before that. Again here, significantly the 1893 regulatory reform.
To explore whether herding varies across different industriesFootnote24, we classify our sample’s stocks into four industries, namely Financials, Railways, Steamships and Trade & Industrials and repeat all of the above estimations for each industry separately.Footnote25
presents a series of descriptive statistics pertaining to the cross-sectional absolute dispersion of returns () and average return (
) of the St. Petersburg stock exchange as a whole and each of the four industries separately. As the figures presented there indicate, the average performance of the total market and all four industries is positive for the whole sample period, with a similar picture emerging from the median values. Financials is the least risky industry, bearing the lowest standard deviation of the four, with the highest value of this indicator observed for Steamships (the industry with the highest mean return). All
-series exhibit departures from normality, considering the significant values of their skewness (always negative in value, with the exception of Steamships) and kurtosis (whose magnitude clearly reflects leptokurtosis) measures, with a similar picture emerging for the
-series as well.
Results–discussion
We begin the presentation of our empirical findings with the results from the estimation of EquationEquation (1)(1)
(1) for the full sample period, both for the total market and for all four industries, as depicted in . All
-estimates are significantlyFootnote26 positive, thus confirming the linearly positive relationship between
and
stipulated by rational asset pricing models. However, as the absolute performance of the market and each industry rises in magnitude, it appears that this relationship changes in structure, becoming significantly negative and nonlinear for the total market, Financials and Trade & Industrials, as indicated by the significantly negative
-values for those estimations. This suggests the presence of herding for the St. Petersburg stock exchange as a whole, as well as for those two industries; conversely, no herding is detected for Railways and Steamships, where
assumes significantly positive values.Footnote27 These results denote that herding was present among investors in Imperial Russia’s largest equity market, something perhaps unsurprising, given the dominance of its trading dynamics by nonprofessional investors prone to speculative trading, as delineated in the earlier section, St. Petersburg Stock Exchange. This is interesting, more so considering the widespread evidence (Kumar and Lee Citation2006; Dorn, Huberman, and Sengmueller Citation2008; Kumar Citation2009; Burghardt Citation2011; Jame and Tong Citation2014; Li, Rhee, and Wang Citation2017) on the tendency of individual investors to herd in their trades, largely motivated by behavioral biases (Barber, Odean, and Zhu Citation2009a, Citation2009b; Barber and Odean Citation2013), as it suggests that the trading behavior of this investor-type has changed little over the centuries. In addition, the variations in herding significance across industries are in line with evidence on industry-effects in herding from modern equity markets (see e.g., Andrikopoulos et al. Citation2017).
Table 1. Summary statistics.
Table 2. Herding (unconditional estimations).
presents the estimates from EquationEquation (3)(3)
(3) , from which we can gauge a rather interesting concentration of herding during months of negative performance; indeed, significantly negative
-values surface for the total market and three of the four industries (except Railways). We also report significant herding during months of positive performance (reflected through significantly negative
-values) for the total market and Railways. The statistically significant difference between
and
for all estimations suggests that herding is strongly asymmetric in the St. Petersburg stock exchange when conditioned on market/industry performance, in line with a multitude of evidence from modern equity markets.Footnote28 The more frequent presence of herding during market/industry slumps may be due to risk aversion prompting investors to herd with their peers on the sell-side, possibly due to panic or to avoid incurring larger losses in case of prolonged slumps.Footnote29 Although this is reflected in three industries’ herding estimates, herding at the total market level appears much stronger during up- compared to downmarket months (
is substantially larger in absolute terms than
), thus confirming literature evidence (Gavriilidis, Kallinterakis, and Leite-Ferreira Citation2013a) on market and industry herding dynamics within the same market not necessarily sharing similar patterns.
Table 3. Herding conditional on monthly performance.
presents the results from herding estimations conditional on increasing/decreasing monthly volatility (EquationEquation 4(4)
(4) ); herding at the total market level is present for months of both rising and falling volatility, more strongly so for the latter (
is around nine times larger in absolute terms than
), thus suggesting a rather strong tendency on behalf of Russian investors toward herding on months whose volatility has declined month-on-month. This is further confirmed from the industry results, with Financials and Trade & Industrials mirroring the total market results and Railways exhibiting herding on decreasing volatility months only (no herding is documented for Steamships for either volatility-state).Footnote30 With the difference between
and
being statistically significant for the total market, Railways and Trade & Industrials, this indicates that herding exhibits asymmetries when conditioned on volatility.Footnote31 The stronger presence of herding for months of decreasing volatility may be due to lower volatility rendering it easier for uninformed investors to track the trades of their informed peers (Holmes, Kallinterakis, and Leite-Ferreira Citation2013)Footnote32; alternatively, lower volatility may have furnished investors with a clearer view of the overall direction of prices, thus allowing them to herd on that direction with greater ease.
Table 4. Herding conditional on monthly volatility.
When testing for the effect of the 1893-reforms over herding in the St. Petersburg stock exchange, it becomes obvious from the estimates presented in that herding in that market surfaces only for the period after July 1893; indeed, this is the case for the total market and two industries (Steamships; Trade & Industrials), with the difference between and
being statistically significant in all three cases.Footnote33 The appearance of herding exclusively in the post-reform years is in accordance with extensive evidence (Borodkin and Perelman Citation2011; Owen Citation2013; Lizunov Citation2015; Goetzmann and Huang Citation2018) on the role of these reforms in fomenting speculation in the market by encouraging the participation of investors from a wide social cross section in equity trading (which, until then, was mainly in the hands of members of the upper social classes and corporate insiders). The fact that herding rises in the aftermath of a reform that helped increase the participation of individual investors in the stock market confirms evidence from modern markets (Kumar and Lee Citation2006; Dorn, Huberman, and Sengmueller Citation2008; Barber, Odean, and Zhu Citation2009a, Citation2009b; Kumar Citation2009; Burghardt Citation2011; Barber and Odean Citation2013; Jame and Tong Citation2014; Li, Rhee, and Wang Citation2017) on the propensity of retail traders to herd in their equity investments. With individual investors being the key candidates for noise trading (Barber, Odean, and Zhu Citation2009a), the rise in liquidity experienced by the market following those reforms (Borodkin, Konovalova, and Perelman Citation2006; Goetzmann and Huang Citation2018) is in line with the established (Black Citation1986) positive relationship between volume and noise trading. What is more, to the extent that this reform catered to the growing speculative appetite in social mood at the time, our results are in line with evidence from research on financial regulation (Gerding Citation2007; Hirshleifer Citation2008) illustrating how mood-friendly regulatory approaches can end up fostering herding phenomena in equity markets.
Table 5. Herding conditional on the regulatory reform of July 1893.
Additional tests
The Chang, Cheng, and Khorana (Citation2000) herding model grew out of the seminal market herding model proposed by Christie and Huang (Citation1995), which confined its focus to the behavior of the cross-sectional dispersion of returns during periods of extreme market returns (identified with returns lying in the extreme tails of the return-distribution). More specifically, the cross-sectional dispersion of returns was proxied in their model via the cross-sectional standard deviation of returns, calculated as:
(6)
(6)
All variables of EquationEquation (6)(6)
(6) are defined same as in EquationEquation (2)
(2)
(2) . Christie and Huang (Citation1995) then tested for herding via the following specification:
(7)
(7)
Where DDOWN (DUP) is a dummy variable equal to unity, if the market’s return belongs to the extreme lower (upper) tail of the distribution of market returns (zero otherwise). Similar to what we mentioned earlier regarding the Chang, Cheng, and Khorana (Citation2000) model, rational asset pricing (Black Citation1972) would predict a positive relationship between the cross-sectional dispersion of returns and absolute market returns (given stock’s varying sensitivities to market movements); as a result, the higher the absolute magnitude of market returns, the higher the value of the cross-sectional return dispersion. If this is the case, then the coefficients of the two dummies in EquationEquation (7)(7)
(7) would be expected to be significantly positive. However, this need not always be the case; periods of high absolute market returns may well entail market stress, and this may prompt investors to sideline their private signals and herd with their peers; if so, stock returns would end up clustering around the average market return, rendering the cross-sectional return dispersion lower in value. Under these circumstances, we would expect the coefficients of the two dummies in EquationEquation (7)
(7)
(7) to assume significantly negative values.
Drawing on the Christie and Huang (Citation1995) model, we test for the presence of herding at the 1% and 5% tails of the distribution of the average market return () of all listed stocks of the St. Petersburg stock exchange during the 1865–1914 window. Our tests are conducted for the total market and each of the four industries and involve the full sample window, as well as the pre- and post-1893 reform sub-periods.Footnote34 Results are presented in and , from where we readily observe that neither α1 nor α2 are significantly negative for any of the estimations performed, thus negating the possibility of herding. Of course, one should bear in mind that the Christie and Huang (Citation1995) model, courtesy of its linear structure, cannot capture non-linear dynamics (which have been often found to be associated with herding)Footnote35, thus confirming the advantageousness of the Chang, Cheng, and Khorana (Citation2000) model in empirical herding estimations.Footnote36
Table 6. Herding (estimated via Christie and Huang Citation1995).
Table 7. Herding (estimated via Christie and Huang Citation1995) Pre and Post July 1893.
Concluding remarks
Although herding phenomena have been widely narrated in the historical finance literature in the context of several episodes before the 20th century in a variety of capital markets, no research to date has empirically investigated the presence of market-wide herding in historical stock exchanges, despite their similarities (rudimentary regulation; dominant presence of retail speculators) with modern-day emerging/frontier markets (for which herding is frequently detected). Our study fills this gap by offering seminal empirical evidence on market-wide herding from the St. Petersburg stock exchange drawing on monthly prices of all stocks listed on that market between January 1865 and July 1914. Results suggest that investors in Imperial Russia’s largest equity market herded significantly, with their herding appearing more frequently during months of negative performance and declining volatility and varying among industries. Controlling for the 1893-reform that prompted wider social participation in equity trading, we find that the significance of herding surfaces exclusively in the post-reform years, with no evidence of herding arising pre-reform. To the extent that the St. Petersburg stock exchange was dominated during our sample period by nonprofessional investors engaged in speculation, our results are in line with evidence from modern financial markets both on individual investors being prone to herding and (in view of the post-reform results) on the role of regulation in fostering herding phenomena when catering to social mood.
The evidence presented in this study bears important implications for researchers, both those from the historical finance research stream and those from the behavioral finance one. With regards to the former, our findings showcase that their research area’s scope would benefit from expanding to cover issues of behavioral trading patterns in historical markets, since empirically testing for such patterns would help assess the validity of the extant narrative evidence on investors’ behavior from past centuries and provide novel insight into the motives of such behavior. Such research would be feasible, more so considering both the increased availability of databases pertaining to historical stock exchanges and the availability of empirical designs from the study of those patterns in modern financial markets. As far as researchers from behavioral finance are concerned, the results outlined in this paper denote that empirically exploring aspects of investors’ behavior in historical markets can endow us with novel insight as to how behavioral trading unfolded in markets characterized by rudimentary institutional frameworks and how the evolution of the latter affected those trading patterns (as our post-1893 reform findings so amply demonstrated). This last part is also of key relevance to regulatory authorities, which could consider drawing on such empirical evidence from past centuries to assess the possible behavioral implications of their policies, more so in market settings of nascent institutional designs.Footnote37
Notes
1 The only other study we are aware of that has empirically investigated herding for pre- World war two years is that by Bohl et al. (Citation2012); using a very small sample (19) of listed stocks in Germany for the 1920–1923 period, they found that investors herded significantly towards them during that period; Herding is not the only behavioural trading pattern for which there exists scant empirical research regarding historical markets; almost no empirical work exists for any other trading pattern of investors’ behaviour for them. An exception to this is Pierdzioch (Citation2004), who demonstrated that German investors exhibited significant feedback trading during the 1880–1913 period. Studies relying on ownership data (Acheson and Turner Citation2011; Rutterford, Sotiropoulos, and Van Lieshout Citation2017) have confirmed the presence of home bias in UK investors’ holdings in the 19th century, yet did not research the effect of home bias over their trading decisions (something important, since home bias can foster correlation in the trades of investors within the same region; Feng and Seasholes Citation2004).
2 This has culminated in a growing body of literature devoted to the empirical testing (and, largely, confirmation) of a variety of stylized return-patterns of modern stock exchanges in market settings from earlier centuries. Examples of such patterns include autocorrelation (Annaert and Van Hyfte Citation2006; Bassino and Lagoarde-Segot Citation2015), non-normality (Campbell et al. Citation2018), beta-instability (Mensah Citation2013, Citation2015), momentum (Annaert and Mensah Citation2014; Geczy and Samonov Citation2016; Goetzmann and Huang Citation2018), mean reversion (Goetzmann, Ibbotson, and Peng Citation2001; Annaert and Van Hyfte Citation2006), liquidity premium (Burhop and Gelman Citation2010; Moore Citation2010; Gernandt, Palm, Waldenström Citation2012), equity premium (Annaert, Buelens, and Deloof Citation2015) and asymmetric volatility (Goetzmann, Ibbotson, and Peng Citation2001).
3 For more on the potential reasons underlying industry-variations in herding, see Andrikopoulos et al. (Citation2017) and the discussion in the section, Data and Methodology.
4 Herding can be asymmetric contingent on market/industry performance and volatility due to a confluence of reasons; for a detailed discussion of those see Gavriilidis, Kallinterakis, and Leite-Ferreira (Citation2013a) and the discussion in the third section, Data and Methodology.
5 With respect to developed markets, much research hails from the US, with its findings being rather period-dependent. Earlier studies on US pension (Lakonishok, Shleifer, and Vishny Citation1992) and mutual funds (Grinblatt, Titman, Wermers Citation1995; Wermers Citation1999) covering the pre-2000 years found limited herding among US fund managers; conversely, studies including the post-2000 years (Sias Citation2004; Choi and Sias Citation2009; Liao, Huang, and Wu Citation2011; Celiker, Chowdhury, and Sonaer Citation2015; Cui, Gebka, and Kallinterakis Citation2019) have reported greater magnitudes of institutional herding. Possible explanations for this include growing indexing among fund managers (Stambaugh Citation2014) and reduction in skill over time (Barras Scaillet, and Wermers Citation2010). As far as other developed markets are concerned, evidence in favour of institutional herding has surfaced in Germany (Kremer and Nautz Citation2013; Walter and Weber Citation2006), Spain (Gavriilidis, Kallinterakis, and Leite-Ferreira Citation2013a), Portugal (Holmes, Kallinterakis, and Leite-Ferreira Citation2013; Gavriilidis, Kallinterakis, and Leite-Ferreira Citation2013b) and the United Kingdom (Wylie Citation2005; Blake, Sarno, and Zinna Citation2017).
6 Evidence of institutional herding in emerging markets has been documented in Chile (Olivares Citation2008), Poland (Voronkova and Bohl Citation2005), South Korea (Choe, kho, and Stulz Citation1999; Kim and Wei Citation2002a, Citation2002b) and Taiwan (Hung, Lu, and Lee Citation2010).
7 See the study by Economou et al. (Citation2015b) for institutional herding in Bulgaria and Montenegro.
8 See, for example the evidence from African markets (Guney, Kallinterakis, Komba Citation2017), the Asia-Pacific region (Chiang et al. Citation2013), China (Tan et al. Citation2008), European market samples (Economou, Kostakis, Philippas Citation2011; Mobarek, Mollah, and keasey Citation2014), the Euronext-group (Economou et al. Citation2015a; Andrikopoulos et al. Citation2017), Poland (Goodfellow, Bohl, and Gebka Citation2009), Taiwan (Demirer, Kutan, and Chen Citation2010) and the global evidence by Chiang and Zheng (Citation2010).
9 Small-capitalization stocks entail lower analyst-following and, hence, higher informational uncertainty; investors wishing to tackle the latter may choose to mimic their peers’ trades, if they deem the latter’s content as informative enough. For empirical evidence on institutional herding among small stocks, see Lakonishok, Shleifer, and Vishny (Citation1992), Wermers (Citation1999), Sias (Citation2004), Wylie (Citation2005) and Hung, Lu, Lee (Citation2010). For evidence of herding among smaller stocks at the macro level, see Chang, Cheng, and Khorana (Citation2000) and Caparrelli, D’Arcangelis, and Cassuto (Citation2004).
10 Funds may herd towards large capitalization stocks due to regulatory reasons (the case, e.g., of pension funds facing an institutionally restricted opportunity set of stocks to invest into; see Voronkova and Bohl Citation2005 and Olivares Citation2008) or indexing reasons (the case of fund managers’ performance being benchmarked against an index, which prompts them to mirror its composition in their portfolios and rebalance their portfolios accordingly following any changes in its composition). For more on this, see the discussion in Walter and Weber (2006) and Blake, Sarno, and Zinna (Citation2017).
11 Industry herding has been reported at the micro (Choi and Sias Citation2009; Gavriilidis, Kallinterakis, and Leite-Ferreira Citation2013a; Celiker, Chowdhury, and Sonaer Citation2015) and macro (Zhou and Lai Citation2009; Gebka and Wohar Citation2013; Andrikopoulos, Gebka, and Kallinterakis Citation2021) levels; for more on the reasons motivating possible variations in industry herding see the discussion in Andrikopoulos et al. (Citation2017).
12 Herding (primarily at the market-wide level, but occasionally also at the micro level) has been found to vary with market performance, market volatility, market volume and market sentiment. No uniform pattern has been identified thus far as regards these asymmetries (see e.g., the discussion in Guney, Kallinterakis, and Komba Citation2017).
13 Herding has occasionally been found to be stronger (Kim and Wei Citation2002a; Chiang and Zheng Citation2010; Mobarek, Mollah, and Keasey Citation2014; Cui, Gebka, and Kallinterakis Citation2019) and on other occasions weaker (Choe, Kho, and Stulz Citation1999; Hwang and Salmon Citation2004) following the outbreak of financial crises.
14 See, for example, the study of Chiang et al. (Citation2013) on cross-market herding in the Asia-Pacific region.
15 No specific year is identified with its foundation, the latter being assumed to coincide with 1703 (the foundation year of St. Petersburg as a city); as Borodkin and Perelman (Citation2011) note, the first formal reference to the St. Petersburg stock exchange in official documentation dates to 1721.
16 The April 1877–March 1878 Russo-Turkish war prompted a rise in the government’s budget deficit and the devaluation of the rouble, and was followed by a period of economic stagnation that lasted throughout the 1880s (Owen Citation2013).
17 The Crimean War (1853–1856) led to the proliferation of railroads, steamships, factories and banks across Russia (Owen Citation2013). For more on the evolution of companies’ incorporations in the 19th and early 20th centuries in Imperial Russia, see Borodkin and Perelman (Citation2011).
18 These industries revolved mainly around textiles and beet sugar; see Owen (Citation2013).
19 During this period, St. Petersburg banks engaged in extensive investment banking activities, with much of their revenue hailing from securities’ dealings (Borodkin and Perelman Citation2011).
20 Prior to the 1890s, equity trading was largely confined among members of the upper social classes and corporate insiders (Borodkin, Konovalova, and Perelman Citation2006; Goetzmann and Huang Citation2018).
21 For more information on the compilation of the database, please refer to Goetzmann and Huang (Citation2018).
22 Factors that can render herding stronger during bullish markets include noise trading (unsophisticated investors tend to be attracted to market rallies – see e.g. Grinblatt and Keloharju Citation2001; Lamont and Thaler Citation2003), positive mood (it reduces the perception of riskiness, prompting investors to employ heuristics – such as, e.g. mimicking others - when processing risky decisions; Gavriilidis, Kallinterakis, and Ozturkkal Citation2020), optimistic sentiment (it motivates investors to jump on its bandwagon or, alternatively, prey on it; see e.g. Liao, Huang, and Wu Citation2011; Economou et al. Citation2015a, Citation2015b), external habit formation (the profits enjoyed by their peers during bullish markets can motivate investors to herd with them in order to avoid missing out – see e.g. Guney, Kallinterakis, and Komba (Citation2017) and overconfidence (if investors realize profits during bullish markets, they may attribute them to their skills and, consequently, trade more aggressively in tandem; see e.g. Barber et al. Citation2007). On the other hand, herding can grow stronger during bearish markets due to risk aversion (the case of investors sell-herding with their peers during market slumps in order to curtail their losses) and reputational reasons (“bad” fund managers may mimic the trades of their “good” peers during market slumps, in order to demonstrate that any losses incurred are not due to their investment choices – as these would appear similar to those of their “good” peers – but rather the result of the market’s adverse movements; Scharfstein and Stein Citation1990).
23 Rising volatility periods can motivate herding regardless of whether this volatility is the product of noise (noise traders would be likely to herd; see e.g. Barber and Odean Citation2013) or a higher information flow (if the informational environment grows too complex, investors may choose to herd with their peers to tackle this complexity). On the other hand, declining volatility periods render it easier for uninformed investors to track – and, potentially mimic – their informed peers’ trades (Holmes, Kallinterakis, and Leite-Ferreira Citation2013), while also making it easier for investors to decipher the market’s direction – and, again potentially, herd on it.
24 Industry-variations in herding may be the result of reputational reasons, risk aversion, sector indexing, fads and style rotation; for a detailed discussion of those factors, see Andrikopoulos et al. (Citation2017).
25 In this case, would correspond to the industry’s average performance and its squared value would now signify industry volatility in EquationEquation (4)
(4)
(4) .
26 For the purposes of this study, statistical significance is established at the 10% level, i.e. for p-values less than 0.1.
27 The significantly positive -values for these two industries indicate the presence of “counter herding”, whereby investors strongly deviate from the market’s consensus as far as these industries are concerned. Although it is impossible to assert the reasons underlying the lack of herding for Railways and Steamships, one may argue that their later appearance in Russian economic life (both as innovations and industries) may have led them to appear as less established (compared to Financials and Trade & Industrials) in the perception of investors, who may have viewed their prospects as less clear to herd on.
28 See e.g. Chang, Cheng, and Khorana (Citation2000), Gavriilidis, Kallinterakis, and Leite-Ferreira (Citation2013a), Economou et al. (Citation2015a), Economou et al. (Citation2015b) and Guney, Kallinterakis, and Komba (Citation2017).
29 Such a convergence to the market’s consensus is less observed on the up-market side, whereby coefficients turn positive for Financials, Steamships and Trade-& Industrials, an indication of counter herding.
30 is positive for Railways and Steamships, an indication of counter herding during increasing volatility months; the negative
coefficient for Steamships is statistically insignificant.
31 Similar to earlier research; see e.g. Gavriilidis, Kallinterakis, and Leite-Ferreira (Citation2013a), Economou et al. (Citation2015a), Economou et al. (Citation2015b) and Guney, Kallinterakis, and Komba (Citation2017).
32 Given the absence of institutional investors (key candidates for informed traders in modern financial markets) from the 19th-century St. Petersburg stock exchange, that would likely involve individual investors tracking the trades of corporate insiders.
33 is overwhelmingly positive, a reflection of counter herding during the pre-reform years.
34 Due to the small number of observations (595 monthly observations), we have not tested for asymmetric herding vis-à-vis market performance and market volatility (as this would allow for very few observations on either of the extreme tails of the market return distribution). Specifically conditioning herding on market performance would also be clearly inappropriate in the specific context of the Christie and Huang (Citation1995) model, since the latter assesses herding during extreme positive and extreme negative market returns in the same specification (it would make no sense, for example, to test for herding in the extreme positive and negative tails of the market return distribution for months of positive market performance – since these months would entail no negative values).
35 See e.g., the references in Guney, Kallinterakis, and Komba (Citation2017).
36 Hwang and Salmon (Citation2004) proposed an alternative market herding model based on extracting herding via the cross-section of monthly firm-betas. The reason we cannot employ this model as a robustness check here hinges on the fact that our database does not contain high frequency (e.g., daily) data, from which to estimate monthly betas. The lack of data on a proper market index and a risk-free rate further deters us from utilizing this empirical design; Several herding studies (Galariotis, Rong, and Spyrou Citation2015; Cui, Gebka, and Kallinterakis Citation2019; Andrikopoulos, Gebka, and Kallinterakis Citation2021) have identified whether market herding is intentional or not using the Chang, Cheng, and Khorana (Citation2000) model employed in this paper. The method basically involves partitioning CSADm,t into its fundamental and non-fundamental components, using each separately as the dependent variable for herding estimations. To the extent that this partitioning involves the employment of common risk factors (e.g., Fama-French five factors), this renders the examination of intentional v. spurious herding not feasible in the context of this study, in view of the complete absence of data on common risk factors for the St. Petersburg stock exchange during our sample period; Aside from not capturing non-linear dynamics, an additional issue identified with the Christie and Huang (Citation1995) framework pertains to the susceptibility of CSSD to the presence of outliers (Economou, Kostakis, and Philippas Citation2011).
37 An example here would be frontier stock exchanges, whose regulatory frameworks are still at an evolutionary stage of development (Guney, Kallinterakis, and Komba Citation2017), similar to pre-20th century markets.
References
- Acheson, G. G., and J. D. Turner. 2011. “Investor Behaviour in a Nascent Capital Market: Scottish Bank Shareholders in the Nineteenth Century.” Economic History Review 64 (1):188–213. doi:10.1111/j.1468-0289.2010.00524.x
- Andrikopoulos, P., B. Gebka, and V. Kallinterakis. 2021. “Regulatory Mood-Congruence and Herding: Evidence from Cannabis Stocks.” Journal of Economic Behavior and Organization 185:842–64. doi:10.1016/j.jebo.2020.10.019
- Andrikopoulos, P., V. Kallinterakis, M. P. Leite Ferreira, and T. Verousis. 2017. “Intraday Herding on a Cross-Border Exchange.” International Review of Financial Analysis 53:25–36. doi:10.1016/j.irfa.2017.08.010
- Annaert, J., F. Buelens, and M. Deloof. 2015. “Long-Run Stock Returns: Evidence from Belgium 1838-2010.” Available at SSRN: https://ssrn.com/abstract=2156803; 10.2139/ssrn.2156803.
- Annaert, J., and L. Mensah. 2014. “Cross-Sectional Predictability of Stock Returns, Evidence from the 19th Century Brussels Stock Exchange (1873–1914).” Explorations in Economic History 52:22–43. doi:10.1016/j.eeh.2013.10.002
- Annaert, J., and W. Van Hyfte. 2006. “Long-Horizon Mean Reversion for the Brussels Stock Exchange: Evidence for the 19th Century.” Working Paper, University of Ghent.
- Antoniou, A., N. Ergul, and P. R. Holmes. 1997. “Market Efficiency, Thin Trading and Non-Linear Behaviour: Evidence from an Emerging Market.” European Financial Management 3 (2):175–90. doi:10.1111/1468-036X.00038
- Banerjee, A. V. 1992. “A Simple Model of Herd Behavior.” Quarterly Journal of Economics 107:797–817. doi:10.2307/2118364
- Barberis, N., and A. Shleifer. 2003. “Style Investing.” Journal of Financial Economics 68 (2):161–99. doi:10.1016/S0304-405X(03)00064-3
- Barber, B. M., Y. Lee, Y. Liu, and T. Odean. 2007. “Is the Aggregate Investor Reluctant to Realise Losses? Evidence from Taiwan.” European Financial Management 13 (3) :423–47. doi:10.1111/j.1468-036X.2007.00367.x
- Barber, B. M., Y. Lee, Y. Liu, and T. Odean. 2014. “The Cross-Section of Speculator Skill: Evidence from Day Trading.” Journal of Financial Markets 18:1–24. doi:10.1016/j.finmar.2013.05.006
- Barber, B. M., and T. Odean. 2013. “The Behavior of Individual Investors.” In Handbook of the Economics and Finance, edited by G. M. Constantinides, M. Harris, and R. Stulz, 1533–69. Amsterdam: Elsevier.
- Barber, B. M., T. Odean, and N. Zhu. 2009a. “Do Retail Trades Move Markets?” Review of Financial Studies 22 (1):151–86. doi:10.1093/rfs/hhn035
- Barber, B. M., T. Odean, and N. Zhu. 2009b. “Systematic Noise.” Journal of Financial Markets 12:547–69. doi:10.1016/j.finmar.2009.03.003
- Barras, L., O. Scaillet, and R. Wermers. 2010. “False Discoveries in Mutual Fund Performance: Measuring Luck in Estimated Alphas.” Journal of Finance 65:179–216. doi:10.1111/j.1540-6261.2009.01527.x
- Bassino, J.-P., and T. Lagoarde-Segot. 2015. “Informational Efficiency in the Tokyo Stock Exchange, 1931-1940.” Economic History Review 68 (4):1226–49. doi:10.1111/ehr.12096
- Bikhchandani, S., D. Hirshleifer, and I. Welch. 1992. “A Theory of Fads, Fashion, Custom, and Cultural Change as Informational Cascades.” Journal of Political Economy 100:992–1026. doi:10.1086/261849
- Bikhchandani, S., and S. Sharma. 2001. “Herd Behaviour in Financial Markets.” IMF Staff Papers 47 (3):279–310.
- Black, F. 1972. “Capital Market Equilibrium with Restricted Borrowing.” Journal of Business 45:444–54. doi:10.1086/295472
- Black, F. 1986. “Noise.” Journal of Finance 41 (3):529–43.
- Blake, D., K. Sarno, and G. Zinna. 2017. “The Market for Lemmings: The Herding Behavior of Pension Funds.” Journal of Financial Markets 36:17–39. doi:10.1016/j.finmar.2017.03.001
- Bohl, M. T., C. Burhop, A. C. Klein, and P. L. Siklos. 2012. “Herding During the German Hyperinflation.” Working paper.
- Borodkin, L., A. Konovalova, and G. Perelman. 2006. “St. Petersburg Stock Exchange in the Time of Economic Depression, War and Revolution: Does Instability Matter?” Paper Presented at the XIV International Economic History Congress, Helsinki, Finland.
- Borodkin, L. I., and G. Perelman. 2011. “St. Petersburg Exchange: On the Road to Maturity. Industrial Stock Index, 1897-1914.” In Financial Centres and International Capital Flows in the Nineteenth and Twentieth Centuries, edited by L. Quennouelle-Corre and Y. Cassis, 96–126. Oxford: Oxford University Press.
- Brunnermeirer, M., and S. Nagel. 2004. “Hedge Funds and the Technology Bubble.” Journal of Finance 59 (5):2013–40. doi:10.1111/j.1540-6261.2004.00690.x
- Burghardt, M. 2011. “Retail Investor Herding.” In Retail Investor Sentiment and Behavior, 93–118. Wiesbaden: Gabler Verlag.
- Burhop, C., and S. Gelman. 2010. “Transaction Costs, Liquidity and Expected Returns at the Berlin Stock Exchange, 1892-1913.” Working Paper, Max Planck Institute, Bonn.
- Campbell, G., W. Quinn, J. D. Turner, and Q. Ye. 2018. “What Moved Shared Prices in the Nineteenth-Century London Stock Market?” Economic History Review 71 (1):157–89. doi:10.1111/ehr.12429
- Caparrelli, F., A. M. D’Arcangelis, and A. Cassuto. 2004. “Herding in the Italian Stock Market: A Case of Behavioral Finance.” Journal of Behavioral Finance 5 (4):222–30. doi:10.1207/s15427579jpfm0504_5
- Celiker, U., J. Chowdhury, and G. Sonaer. 2015. “Do Mutual Funds Herd in Industries?” Journal of Banking and Finance 52:1–16. doi:10.1016/j.jbankfin.2014.11.006
- Chang, E. C., J. W. Cheng, and A. Khorana. 2000. “An Examination of Herd Behavior in Equity Markets: An International Perspective.” Journal of Banking and Finance 24:1651–79. doi:10.1016/S0378-4266(99)00096-5
- Chen, T. 2013. “Do Investors Herd in Global Stock Markets?” Journal of Behavioral Finance 14 (3):230–9. doi:10.1080/15427560.2013.819804
- Chiang, T., L. Tan, J. Li, and E. Nelling. 2013. “Dynamic Herding Behavior in Pacific-Basin Markets: Evidence and Implications.” Multinational Finance Journal 17:165–200. doi:10.17578/17-3/4-3
- Chiang, T. C., and D. Zheng. 2010. “An Empirical Analysis of Herd Behavior in Global Stock Markets.” Journal of Banking and Finance 34:1911–21. doi:10.1016/j.jbankfin.2009.12.014
- Choe, H., B. C. Kho, and R. M. Stulz. 1999. “Do Foreign Investors Destabilize Stock Markets? The Korean Experience in 1997.” Journal of Financial Economics 54:227–64. doi:10.1016/S0304-405X(99)00037-9
- Choi, N., and R. W. Sias. 2009. “Institutional Industry Herding.” Journal of Financial Economics 94:469–91. doi:10.1016/j.jfineco.2008.12.009
- Choi, N., and H. Skiba. 2015. “Institutional Herding in International Markets.” Journal of Banking and Finance 55:246–59. doi:10.1016/j.jbankfin.2015.02.002
- Christie, W. G., and R. D. Huang. 1995. “Following the Pied Piper: Do Individual Returns Herd around the Market?” Financial Analysts Journal 51:31–7. doi:10.2469/faj.v51.n4.1918
- Corzo, T., M. Prat, and E. Vaquero. 2014. “Behavioral Finance in Joseph de La Vega’s Confusión de Confusiones.” Journal of Behavioral Finance 15:341–50. doi:10.1080/15427560.2014.968722
- Cui, Y., B. Gebka, and V. Kallinterakis. 2019. “Do Closed-End Fund Investors Herd?” Journal of Banking and Finance 105:194–206. doi:10.1016/j.jbankfin.2019.05.015
- Dale, R. S., J. E. V. Johnson, and L. Tang. 2005. “Financial Markets Can Go Mad: Evidence of Irrational Behaviour during the South Sea Bubble.” Economic History Review 58 (2) :233–71. doi:10.1111/j.1468-0289.2005.00304.x
- Dasgupta, A., A. Prat, and M. Verardo. 2011. “The Price Impact of Institutional Herding.” Review of Financial Studies 24 (3) :892–925. doi:10.1093/rfs/hhq137
- Demirer, R., A. M. Kutan, and C.-D. Chen. 2010. “Do Investors Herd in Emerging Stock Markets? Evidence from the Taiwanese Market.” Journal of Economic Behavior and Organizations 76:283–95. doi:10.1016/j.jebo.2010.06.013
- Devenow, A., and I. Welch. 1996. “Rational Herding in Financial Economics.” European Economic Review 40:603–15. doi:10.1016/0014-2921(95)00073-9
- Dorn, D., G. Huberman, and P. Sengmueller. 2008. “Correlated Trading and Returns.” Journal of Finance 63:885–920. doi:10.1111/j.1540-6261.2008.01334.x
- Economou, F., K. Gavriilidis, V. Kallinterakis, and A. Goyal. 2015a. “Herding Dynamics in Exchange Groups: Evidence from Euronext.” Journal of International Financial Markets, Institutions and Money 34:228–44.
- Economou, F., K. Gavriilidis, V. Kallinterakis, and N. Yordanov. 2015b. “Do Fund Managers Herd in Frontier Markets-and Why?” International Review of Financial Analysis 40:76–87. doi:10.1016/j.irfa.2015.03.017
- Economou, F., A. Kostakis, and N. Philippas. 2011. “Cross-Country Effects in Herding Behavior: Evidence from Four South European Markets.” Journal of International Financial Markets, Institutions and Money 21:443–60. doi:10.1016/j.intfin.2011.01.005
- Feng, L., and M. S. Seasholes. 2004. “Correlated Trading and Location.” Journal of Finance 59 (5):2117–44. doi:10.1111/j.1540-6261.2004.00694.x
- Froot, K., D. Scharfstein, and J. C. Stein. 1992. “Herd on the Street: Informational Inefficiencies in a Market with Short-Term Speculation.” Journal of Finance 47:1461–84. doi:10.1111/j.1540-6261.1992.tb04665.x
- Galariotis, E., W. Rong, and S. Spyrou. 2015. “Herding on Fundamental Information: A Comparative Study.” Journal of Banking and Finance 50:589–98. doi:10.1016/j.jbankfin.2014.03.014
- Galbraith, J. K. 1994. A Short History of Financial Euphoria. New York: Penguin Books.
- Gavriilidis, K., V. Kallinterakis, and M. P. Leite-Ferreira. 2013a. “Institutional Industry Herding: Intentional or Spurious?” Journal of International Financial Markets, Institutions and Money 26:192–214.
- Gavriilidis, K., V. Kallinterakis, and M. P. Leite-Ferreira. 2013b. “On the Impact of Style Investing over Institutional Herding: Evidence from a Highly Concentrated Market.” Investment Management and Financial Innovations 10 (4):27–42.
- Gavriilidis, K., V. Kallinterakis, and B. Ozturkkal. 2020. “Does Mood Affect Institutional Herding?” Journal of Behavioral and Experimental Finance 26:100290. doi:10.1016/j.jbef.2020.100290
- Gebka, B., and M. E. Wohar. 2013. “International Herding: Does It Differ across Sectors?” Journal of International Financial Markets, Institutions and Money 23:55–84.
- Geczy, C. C., and M. Samonov. 2016. “Two Centuries of Price-Return Momentum.” Financial Analysts Journal 72:32–56. doi:10.2469/faj.v72.n5.1
- Gelos, R. G., and S. J. Wei. 2005. “Transparency and International Portfolio Holdings.” Journal of Finance 60:2987–3020. doi:10.1111/j.1540-6261.2005.00823.x
- Gerding, E. F. 2007. “The Next Epidemic: Bubbles and the Growth and Decay of Securities Regulation.” Connecticut Law Review 38:393–453.
- Gernandt, O., T. Palm, and D. Waldenström. 2012. “Liquidity Premia during the Industrial Breakthrough: Evidence from the Stockholm Stock Exchange, 1901-1919.” European Review of Economic History 16 (3) :247–69. doi:10.1093/ereh/hes002
- Goetzmann, W. N., and S. Huang. 2018. “Momentum in Imperial Russia.” Journal of Financial Economics 130 (3):579–91. doi:10.1016/j.jfineco.2018.07.008
- Goetzmann, W. N., R. G. Ibbotson, and L. Peng. 2001. “A New Historical Database for the NYSE 1815 to 1925: Performance and Predictability.” Journal of Financial Markets 4:1–32. doi:10.1016/S1386-4181(00)00013-6
- Goodfellow, C., M. Bohl, and B. Gebka. 2009. “Together We Invest? Individual and Institutional Investors’ Trading Behavior in Poland.” International Review of Financial Analysis 18:212–21. doi:10.1016/j.irfa.2009.03.002
- Grinblatt, M., and M. Keloharju. 2001. “What Makes Investors Trade?” Journal of Finance 56 (2):589–616. doi:10.1111/0022-1082.00338
- Grinblatt, M., S. Titman, and R. Wermers. 1995. “Momentum Investment Strategies, Portfolio Performance, and Herding: A Study of Mutual Fund Behaviour.” American Economic Review 85:1088–105.
- Guney, Y., V. Kallinterakis, and G. Komba. 2017. “Herding in Frontier Markets: Evidence from African Stock Exchanges.” Journal of International Financial Markets, Institutions and Money 47:152–75. doi:10.1016/j.intfin.2016.11.001
- Hirshleifer, D. 2008. “Psychological Bias as a Driver of Financial Regulation.” European Financial Management 14 (5) :856–74. doi:10.1111/j.1468-036X.2007.00437.x
- Hirshleifer, D., and S. T. Teoh. 2003. “Herd Behavior and Cascading in Capital Markets: A Review and Synthesis.” European Financial Management 9:25–66. doi:10.1111/1468-036X.00207
- Holmes, P. R., V. Kallinterakis, and M. P. Leite-Ferreira. 2013. “Herding in a Concentrated Market: A Question of Intent.” European Financial Management 19:497–520. doi:10.1111/j.1468-036X.2010.00592.x
- Hung, W., C.-C. Lu, and C. F. Lee. 2010. “Mutual Fund Herding and Its Impact on Stock Returns: Evidence from the Taiwan Stock Market.” Pacific-Basin Finance Journal 18:477–93. doi:10.1016/j.pacfin.2010.06.001
- Hwang, S., and M. Salmon. 2004. “Market Stress and Herding.” Journal of Empirical Finance 11:585–616. doi:10.1016/j.jempfin.2004.04.003
- Jame, R., and Q. Tong. 2014. “Industry-Based Style Investing.” Journal of Financial Markets 19:110–30. doi:10.1016/j.finmar.2013.08.004
- Jiang, H., and M. Verardo. 2018. “Does Herding Behavior Reveal Skill? An Analysis of Mutual Fund Performance.” Journal of Finance 73 (5) :2229–69. doi:10.1111/jofi.12699
- Kaniel, R., G. Saar, and S. Titman. 2008. “Individual Investor Trading and Stock Returns.” Journal of Finance 63 (1):273–310. doi:10.1111/j.1540-6261.2008.01316.x
- Kim, W., and S.-J. Wei. 2002a. “Offshore Investment Funds: Monsters in Emerging Markets?” Journal of Development Economics 68 (1):205–24. doi:10.1016/S0304-3878(02)00012-3
- Kim, W., and S.-J. Wei. 2002b. “Foreign Portfolio Investors before and during a Crisis.” Journal of International Economics 56 (1):77–96. doi:10.1016/S0022-1996(01)00109-X
- Kindleberger, C. P., and R. Z. Aliber. 2005. Manias, Panics and Crashes. Hoboken, NJ: Wiley.
- Kremer, S., and D. Nautz. 2013. “Causes and Consequences of Short-Term Institutional Herding.” Journal of Banking and Finance 37:1676–86. doi:10.1016/j.jbankfin.2012.12.006
- Kumar, A. 2009. “Dynamic Style Preferences of Individual Investors and Stock Returns.” Journal of Financial and Quantitative Analysis 44:607–40. doi:10.1017/S0022109009990020
- Kumar, A., and C. M. C. Lee. 2006. “Retail Investor Sentiment and Return Comovements.” Journal of Finance 61:2451–86. doi:10.1111/j.1540-6261.2006.01063.x
- Lakonishok, J., A. Shleifer, and R. Vishny. 1992. “The Impact of Institutional Trading on Stock Prices.” Journal of Financial Economics 32:23–43. doi:10.1016/0304-405X(92)90023-Q
- Lamont, O. A., and R. H. Thaler. 2003. “Can the Market Add and Subtract? Mispricing in Tech Stock Carve-Outs.” Journal of Political Economy 111 (2):227–68. doi:10.1086/367683
- Liao, T.-L., C.-J. Huang, and C.-W. Wu. 2011. “Do Fund Managers Herd to Counter Investor Sentiment?” Journal of Business Research 64:207–12. doi:10.1016/j.jbusres.2010.01.007
- Li, W., G. Rhee, and S. S. Wang. 2017. “Differences in Herding: Individual vs. Institutional Investors.” Pacific-Basin Finance Journal 45:174–85. doi:10.1016/j.pacfin.2016.11.005
- Li, W., and S. S. Wang. 2010. “Daily Institutional Trades and Stock Price Volatility in a Retail Investor Dominated Emerging Market.” Journal of Financial Markets 13 (4):448–74. doi:10.1016/j.finmar.2010.07.003
- Lizunov, P. V. 2015. “The Ministry of Finance and the St. Petersburg Stock Exchange in the Nineteenth and Early Twentieth Centuries.” Russian Studies in History 54 (2):95–105. doi:10.1080/10611983.2015.1117892
- Mensah, L. 2013. “The Behavior of Beta in the 19th Century.” Accounting and Finance Research 2 (4):34–46. doi:10.5430/afr.v2n4p34
- Mensah, L. 2015. “Size, Beta, Average Stock Return Relationship, 19th Century Evidence.” Journal of Finance and Bank Management 3 (1):117–33. doi:10.15640/jfbm.v3n1a11
- Mobarek, A., S. Mollah, and K. Keasey. 2014. “A Cross-Country Analysis of Herd Behavior in Europe.” Journal of International Financial Markets, Institutions and Money 32:107–27. doi:10.1016/j.intfin.2014.05.008
- Moore, L. 2010. “Financial Market Liquidity, Returns and Market Growth: Evidence from Bolsa and Börse, 1902–1925.” Financial History Review 17:73–98. doi:10.1017/S0968565010000016
- Neal, L. 1982. “Efficient Markets in the Eighteenth Century? Stock Exchanges in Amsterdam and London.” Business and Economic History 11:81–101.
- Olivares, J. A. 2008. “Rear-View-Mirror Driving in Defined Contribution Systems: The Strange Formula of the Chilean Pension Funds.” Applied Economics 40:2009–19. doi:10.1080/00036840600936345
- Owen, T. C. 2013. “Measuring Business Cycles in the Russian Empire.” Economic History Review 66 (3):895–916. doi:10.1111/j.1468-0289.2012.00673.x
- Pierdzioch, C. 2004. “Feedback Trading and Predictability of Stock Returns in Germany.” Working Paper No. 1213, Kiel Institute for World Economics, 1880–913. ”
- Rutterford, J., D. P. Sotiropoulos, and C. Van Lieshout. 2017. “Individual Investors and Local Bias in the UK, 1870-1935.” Economic History Review 70 (4):1291–320. doi:10.1111/ehr.12482
- Scharfstein, D. S., and J. C. Stein. 1990. “Herd Behavior and Investment.” American Economic Review 80:465–79.
- Sias, R. W. 2004. “Institutional Herding.” Review of Financial Studies 17:165–206. doi:10.1093/rfs/hhg035
- Stambaugh, R. F. 2014. “Presidential Address: Investment Noise and Trends.” Journal of Finance 69 (4):1415–53. doi:10.1111/jofi.12174
- Tan, L., T. C. Chiang, J. R. Mason, and E. Nelling. 2008. “Herding Behavior in Chinese Stock Markets: An Examination of a and B Shares.” Pacific Basin Finance Journal 16:61–77. doi:10.1016/j.pacfin.2007.04.004
- Teh, L. L., and W. F. M. De Bondt. 1997. “Herding Behavior and Stock Returns: An Exploratory Investigation.” Swiss Journal of Economics and Statistics 133:293–324.
- Voronkova, S., and M. T. Bohl. 2005. “Institutional Traders’ Behaviour in an Emerging Stock Market: Empirical Evidence on Polish Pension Fund Investors.” Journal of Business, Finance and Accounting 32:1537–60. doi:10.1111/j.0306-686X.2005.00639.x
- Walter, A., and M. Weber. 2006. “Herding in the German Mutual Fund Industry.” European Financial Management 12:375–406. doi:10.1111/j.1354-7798.2006.00325.x
- Wermers, R. 1999. “Mutual Fund Herding and the Impact on Stock Prices.” Journal of Finance 54:581–622. doi:10.1111/0022-1082.00118
- Wylie, S. 2005. “Fund Manager Herding: A Test of the Accuracy of Empirical Results Using UK Data.” Journal of Business 78:381–403. doi:10.1086/426529
- Zhou, R. T., and R. N. Lai. 2009. “Herding and Information Based Trading.” Journal of Empirical Finance 16:388–93. doi:10.1016/j.jempfin.2009.01.004