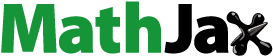
Abstract
Robert Shiller highlights the role popular stories play in driving economic behavior and argues the need to analyze these scientifically. However, their impacts are difficult to measure directly and often conflict. We show the strength of such stories resides in the emotions they generate, and that the tenor and persuasiveness of financial narratives and their association with the market can be empirically quantified. Specifically, we textually analyze financial media reports to identify the different powerful investor emotions manifest during three recent extreme market periods, dot.com mania, the Global Financial Crisis and the COVID-19 pandemic, constructing original context-specific emotion word dictionaries for this purpose. We find investor emotions are associated with up to 52% of market returns and 67% of market uncertainty during these market crises, and provide general evidence that investor emotional dynamics may be time and context invariant.
1. Introduction
Investor emotions are strongly associated with market dynamics. For example, the extreme market volatility associated with the COVID-19 pandemic dramatically illustrates the role these play in driving market behavior. The S&P 500 index peaked on 19 February 2020 after a run-up of 400% over the previous 11 years, even though the threat COVID-19 represented was already well known in principle. The VIX was then standing at only 15%, similar to that in previous years. However, stock market fluctuations over the following few weeks are difficult to explain in rational terms with average S&P 500 daily volatility levels of 4.6%, and with the VIX hovering around 60%-80% to the end of March when the market started to recover, even higher than at the peak of the Global Financial Crisis. Such day-to-day fluctuations are unlikely to be explained by changes in valuation expectations. Clearly, a more plausible explanation is that a whole raft of emotions were driving investor behavior, including anxiety, panic, and denial, seemingly changing from one moment to the next, as clearly reflected in news coverage and commentary in the mass media.
Shiller (Citation2019) argues that economists need to explore the role of popular narratives in trying to explain major economic events. Such often conflicting stories (including songs, jokes, theories, explanations, and plans) about salient events which people talk about and repeat are used to meet the human need to make sense of what is going on. They are contagious and emotionally charged, which gives them their power, and dynamic: they change over time.
The part played by the emotions of economic agents and social and group processes in extreme economic events including market bubbles, needs to be properly recognized. At the heart of Shiller’s (Citation2014) definition of bubbles are “the emotions of investors, and the nature of news and news media” (p. 1487), the latter being a key generator and disseminator of economic narratives. Their power, he argues, resides in the emotions they provoke in market participants, which drive their decisions. Importantly, it often does not matter whether such narratives have a factual basis or not, and whether they are coherent, for them to be believed and disseminated – wishful thinking often plays an important role in their spread. We act on such stories often without reflection or thought but emotionally.
To illustrate the powerful economic impact narratives can possess, Shiller (Citation2017) explores the economic history of the 1920-1921 Depression, the Great Depression of the 1930s and the Great Recession of 2007-2009 in terms of the respective popular narratives of the times. For each of these major economic crises, he provides a wide range of superficially plausible stories or explanatory narratives, both conventional and less-conventional, the most salient ones seemingly having high degrees of emotional resonance.Footnote1 In his book Narrative Economics, Shiller (Citation2019) takes a broader perspective, and examines nine perennial narrative themes that influence important economic events including stock market bubbles (chapter 16) which we also consider empirically in this paper.
Narratives about what actually happens during major market events such as financial crises and asset pricing bubbles (e.g., Mackay, Citation1852; Galbraith, Citation1993; Cassidy, Citation2002; Tuckett & Taffler, Citation2008; Aliber & Kindleberger, Citation2015; Shiller, Citation2019; Quinn & Turner, Citation2020; Taffler et al., Citation2022) are first and foremost descriptions of highly emotional speculative processes. Terms such as excited, euphoric, exuberant, manic, depressed, anxious, blame, illusion, delusion and panic etc., abound. This paper explores how the nexus of contemporaneous popular stories reflecting investor emotions is associated with their investment behavior during asset pricing bubbles and financial crises as proxied by market movements. To do this we explore how the financial media reports on, and seeks to explain, extreme market events.
Rather than seeking to privilege particular narratives as the most likely causes of economic events, Shiller (Citation2017) views economic behavior as being driven by their confluence in the form of “epidemics of narrative”, the common factor being their emotive power. Shiller (Citation2019) acknowledges it is difficult to distinguish correlation and causality in the relationship between popular economic narratives and economic behavior. In addition, such stories often conflict; how can we actually “sort through them” (p. 286) and make sense of what is really going on? One approach, he suggests, is to use multiple appropriately trained research assistants to track such narratives, and “to classify and quantify them according to their essential emotional driving force” (p. 287, emphasis added), although not spelling out how this might be done in practice and subjectivity removed.
In this paper, we follow Shiller in focusing on emotionally-charged narratives. However, since it is not possible to measure the intrinsic nature of economic narratives directly, we proxy their relationship with the market by focusing on the emotions they reflect and generate in the minds of market participants and the associated market dynamics. Specifically, we measure the power of economic narratives by employing textual analysis and carefully constructed emotion keyword dictionaries to identify the specific emotions conveyed in these, and their empirical association with the trading decisions of investors dynamically, as reflected in market prices.Footnote2 We note that a story may reflect a number of different emotions, and a particular emotion may be present in several stories. As such, it is only meaningful to measure the emotions salient at any point in time, not attribute these to particular narratives.
provides a schematic representation of the relationships we measure, explicitly recognizing their joint determination, and interrelated nature of the underlying processes. The figure illustrates how such things as events, speculations, and rumors etc. feed into the construction of economic narratives [i]. These generate the powerful emotions [ii] which drive investor decisions, and consequently, market prices and volatility [iii]. However, market behavior will also be reflected in stories written about the market in the media [iv], and will likewise impact investor emotions [v]. Similarly, their emotional states will influence financial journalists, and other commentators, and analysts [vi].Footnote3
This paper investigates the relationship between the emotions economic narratives reflect and market returns and market uncertainty during three major market crises when investor emotions are likely to be most salient: the internet, ‘new economy’ or ‘dot.com’ bubble of 1998-2002, the Global Financial Crisis (GFC) of 2007-2011, and the COVID-19 pandemic of 2020-2022. However, while dot.com mania was an equity market bubble largely restricted to internet stocks, the effects of the Global Financial Crisis and COVID-19 were much more severe with far wider impact. The bursting of the internet bubble was not immediately associated with a fall in output. Although GDP shrank in the first and third quarters of 2001, and the US economy was classified by the NBER as in recession for eight months from March to November 2001, the actual contraction was short lived. In contrast, the GFC was characterized by a sharp fall in GDP and collapse in the S&P 500, followed by a much slower recovery in economic output. In the case of the COVID-19 pandemic, GDP was, surprisingly, largely unaffected inter alia because of government support to alleviate its economic effects. In fact, NBER only classified the US economy in recession in February and March 2020.
Specifically, we explore the emotional characteristics of economic narratives to understand how these are associated with investor behavior and the market directly. We do this by measuring a range of investor emotions (excitement, anxiety, mania, panic, revulsion/blame, denial, and guilt) salient in the extreme market events we explore through analyzing a large corpus of relevant articles published in the financial media employing rich context-specific keyword dictionaries.
The interrelationships shown in , in practice, make it very difficult to disentangle causality, as Shiller (Citation2019) emphasizes. In any case, emotions and market movements are frequently synchronous. Emotions are processed almost instantaneously. Therefore, we would expect any emotional impact of powerful economic narratives to be incorporated very quickly in market prices. As such, this paper specifically focuses on the contemporaneous role played by narrative economics as mediated by the emotions they reflect and generate in explaining market behavior. We argue such understanding is important in its own right.
Our empirical analysis supports the direct link between the emotions reflected in economic narratives, and the investment behavior of economic actors. We show how both during the new economy bubble, and the Global Financial Crisis and COVID-19 pandemic, investors experienced deep-seated emotions as manifest in the market narratives current at the time. Specifically, we demonstrate how popular stories explain a significant proportion of market activity in these three extreme periods through the agency of the emotions they generate and reflect. This is of the order of 40% to 52% in the case of market returns, and between 29% and 67% for market uncertainty depending on how it is measured.
We find market returns are positively related with ‘exciting’ investor emotions (excitement and mania), and negatively with ‘anxious’ emotions (anxiety, panic, revulsion/blame, denial and guilt). We show how our exciting emotions dominate in the up or recovery phase of a market crisis, and our anxious emotions in its down phase. We also explore how market uncertainty, as proxied by market volatility, trading volume and the Baker et al. (Citation2016) news-based economic policy uncertainty (EPU) index, is dealt with emotionally. Our empirical results clearly demonstrate how increasing levels of uncertainty are associated with raised levels of anxiety, panic and linked emotions in the minds of investors, which affect their trading behavior. Our main conclusion is that investor emotions, as reflected in Shiller’s ‘economic narratives’, are important in explaining market behavior, and it is possible to measure these empirically. We also show that investor emotions as measured by our emotion keyword dictionaries are robust across different market crises and time periods.
We contribute to the literature in several ways. First, we build on Shiller’s insights into the role narratives play in explaining economic events by measuring empirically the different emotions these reflect, and their relationships with market dynamics during asset pricing bubbles. Second, our real-world findings contribute to the debate about the ecological validity of experimental asset markets. For example, Andrade et al. (Citation2016) and Breaban and Noussair (Citation2018) create simulated markets that generate price bubbles and crashes and explore the relationship between participants’ emotions and investment decisions. Both laboratory studies suggest that students’ emotional states and simulated market dynamics are correlated in a similar way to how market pricing bubbles are highly emotional events for investors in real world markets. Finally, we extend the tone-based sentiment analysis methodology widely used in finance (e.g., Henry & Leone, Citation2016; Loughran & McDonald, Citation2016), and show how it is possible to extract rich emotional data from financial media.
A parallel recent stream in the finance literature employs commercially available sentiment measures such as those provided by ThomsonReuters MarketPsych (e.g., Griffith et al., Citation2020; Shen et al., Citation2023a; Shen et al., Citation2023b) and RavenPack (e.g., Mangee, Citation2021; Bird et al., Citation2023). We also add to such studies by developing seven distinct emotion theory-driven keyword dictionaries to measure the fundamental emotions associated with market behavior. These dictionaries are provided as an Appendix to the paper and have the potential for wider application.
This paper proceeds as follows. In the next section we present our underlying theory and motivation, and establish our main propositions. Section 3 then describes our content analysis emotion keyword dictionary building process, our data and research method. Empirical tests of our main thesis follow in our results Section 4. Section 5 discusses and concludes.
2. Theory and motivation
2.1. Emotional narratives, storytelling, and market behavior
Storytelling is a fundamental process by which we make sense of the world around us. As a species, human beings can be described as “homo narrans” (Fisher, Citation1984, p. 6) or “homo fabulans – the tellers and interpreters of narrative” (Currie, Citation1998, p. 2). In fact, narratives constitute “the primary form by which human experience is made meaningful” (Polkinghorne, Citation1988, p. 1). Sense making is key to who we are.Footnote4
Importantly, narratives contain plots and characters with the plot transforming a chronicle or sequence of events into a story which generates an emotional response. It is this which is necessary for, and leads to, action knitting the narrative together so we can recognize the deeper significance of the event. Stories are powerful devices for managing meaning. Through the medium of story, the unexpected can be transformed into the expectable, and the unmanageable future rendered notionally manageable or controllable. The key is a story’s plausibility rather than its accuracy. Importantly, in stories unpredictability does not imply inexplicability.
Narrative rationality, or sense making, arises from peoples’ “inherent awareness of narrative probability, what constitutes a coherent story… and narrative fidelity, whether or not the stories they experience ring true”. (Fisher, Citation1984, p. 8). There is no requirement for them actually to be true! “Accuracy is nice but not necessary in sense making… what is necessary in sense making is a good story” (Weick, Citation1995, pp. 60-61).
A common feature of the myriad of financial crises and market bubbles described in Aliber and Kindleberger (Citation2015), which range from tulip bulbs, through the South Sea Bubble, canals, railroads, stock prices before the Great Crash, real estate, internet stocks and the recent property-led financial crisis, is the presence of an emotionally-driven trajectory. In each case patchy excitement about an innovation leads to euphoria (or mania), denial (or manic defense) and then, when reality ultimately intrudes, and the bubble bursts, panic is followed finally by shame and blame. Tuckett and Taffler (Citation2008) explore dot.com mania from a psychoanalytic perspective and demonstrate how throughout this process it is not a question of lack of information about the riskiness of the respective investments, but the way in which this is treated.Footnote5 In parallel, Shiller (Citation2014) argues that speculative bubbles are not about the “craziness” of investors but how they are “buffeted en masse from one superficially plausible theory about conventional valuation to another”, i.e., by popular investment narratives which often take the form of myth.
Importantly, observation of actual bubbles demonstrates how when they ultimately collapse this is not due to new facts but that the underlying reality can no longer be denied, and repressed anxieties rendered unconscious. The whole process then goes into reverse with investors now taking flight in a headlong panic. Anger and blame of others rather than feelings of personal guilt erupt allowing investors to avoid the painful realization of how they have been caught up in the temporarily very enriching and exciting wish-fulfilling fantasy. Psychologically, anxiety will change into even more painful feelings of loss, humiliation and shame for being actively involved in what has turned out to be only a chimera.
Particularly in periods of market crisis the role played by the media is key. Not only does it disseminate value-relevant information to market participants, but also provides (superficially) plausible explanations or meaning for the events as they unfold (Gamson et al., Citation1992). Kury (Citation2014) claims that investors, as readers/audiences, understand financial markets through the media; in other words, investors’ emotions can be influenced by the media and how journalists report on what is going on in the market (e.g., Engelberg & Parsons, Citation2011; Tetlock, Citation2011; Dougal et al., Citation2012; Peress, Citation2014; Adämmer & Schüssler, Citation2020; Call et al., Citation2022). In parallel, media stories reflect investors’ emotions as these are acted out in their investment decisions and reflected in market prices.
In this paper we utilize news reports, comments, opinions and press releases published in the business media to provide the popular investment narratives we work with. Specifically, we conduct formal content analysis of media reports on the stock market constructing seven different emotion word dictionaries to measure market emotions, and the interrelationships between them.
To test our thesis, we work with three market crises when the influence of economic narratives would be expected to be most pronounced; we derive our keyword dictionaries using media coverage of the first period and validate the generalizability of these by applying them out-of-sample inter-temporally in the second and third periods.
The meteoric rise in the prices of internet stocks, unexplainable in terms of fundamental value, and their equally spectacular fall, constitutes the first extreme economic event (Aggarwal et al., Citation2009; Bhattacharya et al., Citation2009) we analyze. shows how the Dow Jones Internet Price Index rose by 1100% in just over two-and-a-half years from July 1st 1997, when it was launched, to March 9th 2000, when it peaked, compared with a 57% increase in the S&P 500. The Internet sector then accounted for 6% of the market capitalization of all US public companies and 20% of all publicly traded equity volume (Ofek & Richardson, Citation2003). This Index then halved in value by mid-April, and by the first anniversary of its peak was down by 85%. At the end of September 2002, it stood at only 5% of its high, even below where it was in July 1997.
Figure 2. Dow Jones Internet Index and S&P 500 Composite (July 1997 to December 2004) (rebased to 100).
Note: The shaded period is the NBER recession period (Mar-01 to Nov-01).
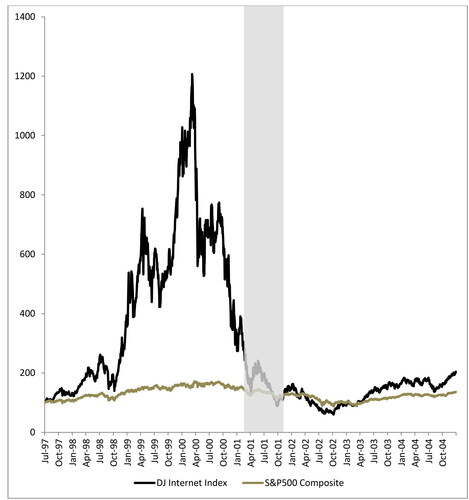
A similar scenario was played out during the Global Financial Crisis. This time, the driver was the sharp increase in house prices in the US. shows how the S&P/Case-Shiller US National Home Price Index went up by almost 60% between 2002, and its peak in 2006. However, as house prices went into reverse, the S&P 500 Index collapsed, falling from 1,565 on 9th October 2007 to 677 on 9th March 2009, a loss of 57% of its market value in a little under one-and-a-half years. This was accompanied by the most severe contraction of the US economy since the Great Depression with GDP shrinking in 5 of the 6 quarters from the first quarter of 2008. The market (and the economy) then started to recover with the S&P 500 index almost doubling by the middle of 2011 and house prices stabilizing, as illustrates.
Figure 3. The S&P/Case-Shiller National Home Price Index, S&P 500 Composite (rebased to 100), and trailing four quarters’ real GDP growth rate (January 2002 to June 2011).
Note: The shaded period is the NBER recession period (Dec-07 to Jun-09).
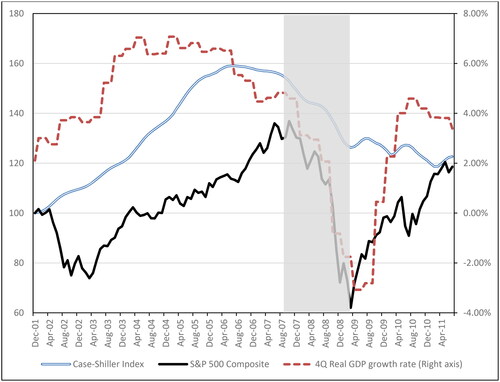
In our third market crisis period, driven by the COVID-19 pandemic, shows how the S&P 500 fell by 34% in not much more than a month between the 19th February and 23rd March 2020 to 2,237 despite only a handful of COVID cases being notified. It then rapidly recovered and by the end of December had increased by 70% coincident with COVID cases rising dramatically to 1.7 million in the week ending 13th January 2021 with over 25,000 Covid-related deaths. The S&P 500 peaked a year later on 3rd January 2022 at 4,796, more than double its trough 21 months earlier, and effectively concurrent with peak levels of COVID-19 cases of 5.5 million in the week ending 19th January 2022, and 21,000 Covid-related deaths. It subsequently fell again by 21% in the following half year to the end of June, paradoxically in parallel with COVID rates rapidly dropping to their lowest level of 194,000 in the week to 30th March, as illustrates, and with the Covid-related deaths figure now only 10% of that not much more than two months earlier.
Figure 4. US weekly COVID-19 cases and the S&P 500 Composite (rebased to 100) (January 2020 to June 2022).
Notes: The shaded period is the NBER recession period (February to March 2020). The weekly COVID-19 cases are from Centers for Disease Control and Prevention (CDC COVID Data Tracker: Daily and Total Trends).
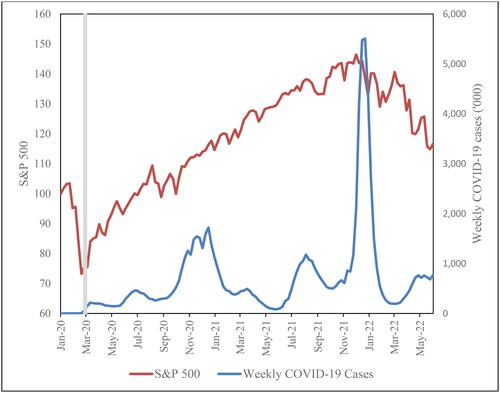
What role might economic narratives play in such dramatic changes in firm valuation occurring across a whole sector (in the first instance) or the whole market (in the second and third instances) over such a short period of time?
2.2. Propositions
Our main argument is that market crisis events and the associated constellation of narratives are intimately related through the powerful investor emotions they generate and reflect. If this holds, the implication is the need formally to take account of the emotions of economic agents in explanatory economic or financial models at least of such extreme events. We set up the following three propositions to test our thesis focusing on the internet bubble, Global Financial Crisis, and COVID-19 pandemic periods.
First, we predict that the economic narratives which reflect and shape investor emotions are closely associated with market dynamics with positive returns linked with ‘exciting’ emotions, and negative returns with ‘anxious’ ones. Second, as stock markets are efficient, future stock prices are essentially unpredictable and Knightian uncertainty (Knight, Citation1921) prevails. This inevitably leads to anxiety and associated emotions in the minds of market participants, whether acknowledged or unacknowledged, which impacts their investment decisions. We would expect investor emotions to be strongly related to market uncertainty as proxied by trading volumes and market volatility during market crisis events. Therefore, if this contention holds, investor emotional engagement with the market will be greater in more uncertain market conditions.
Third, during extreme market events, powerful feelings of excitement and potentially mania will be experienced by investors as stock prices shoot up. Conversely, when prices are collapsing, we would predict feelings of anxiety and related anxious emotions dominating. If this expectation is correct, there will be a stronger exciting (anxious) investor emotional engagement with the market during its up (down) phase than during its collapse (expansion).
3. Data and method
In this section, we first describe how we build the keyword dictionaries we use to measure market-wide emotions based on dot.com mania and which we subsequently apply to the GFC and COVID-19 crises periods. We then present our method to test our propositions.
3.1. Dictionary building process
Loughran and McDonald (Citation2011) and Henry and Leone (Citation2016) conclude that context-specific dictionaries outperform general ones. We adopt a conventional “bag-of-words” approach (Loughran & McDonald, Citation2016) in constructing our context-specific keyword dictionaries.Footnote6 Although Loughran and McDonald (Citation2016) argue that wordlists with associated weighting schemes may have potential benefits, Henry and Leone (Citation2016) show that equally weighting the importance of all words performs just as well in practice as wordlists designed in more complex ways. We adopt this more pragmatic approach in this study. El-Haj et al. (Citation2019) critically review computational linguistics methods for the analysis of financial discourse and the issues of generalizability, objectivity and replicability in the construction of keyword dictionaries as well as inter-text and inter-temporal validity. We deal with these issues by using large data samples in both dictionary construction and application, careful selection of our emotion keywords using keyword-in-context analysis and, most importantly, generating our dictionaries using data for dot.com mania and then validating their more general applicability in the analysis of the Global Financial Crisis and COVID epidemic up to two decades later. Although it is possible to speculate that advanced machine learning approaches might have generated better keyword lists than our bag-of-words method, Loughran and McDonald (Citation2020) point out the problems that can arise with machine learning in practice, and we view such questions as outside the remit of this paper.
Aliber and Kindleberger (Citation2015, pp. 39–46) detail three common stages in all extreme financial events ranging from the South Sea Bubble to the Global Financial Crisis. Building on this analysis Tuckett and Taffler's (Citation2008) much richer and detailed reading of dot.com mania provides a five-stage dynamic model with “… patchy excitement … leading to growing excitement, leading to manic or euphoric excitement, then turning to panic and finally resulting in blame … (and) invariably a wish to identify scapegoats … rather than guilt.” (p. 394). Building on this Taffler et al. (Citation2022) demonstrate a parallel trajectory in the case of the Chinese stock market bubbles of 2005–2008 and 2014–2016 confirming the same dynamic emotional process is at work as that in dot.com mania in a very different financial market environment.
We draw on this theoretical framework to identify the respective investor emotions salient during three recent market crises. Applying the above five-stage path dependent emotional trajectory we characterize the first stage of these dramatic market events in terms of different degrees of the superordinate emotion of excitement (associated with the emerging into view in stock market bubbles). In the second stage and third stages, as prices move up to their peak and even further away from fundamental value mania takes over, but inevitably associated with this is the denial of underlying reality (hence manic-denial). As the bubble collapses panic is followed by revulsion and its inevitable concomitant blame (of others for investor losses rather than themselves). The emotion of anxiety underpins all market activity on one level, whether consciously acknowledged or not, as there is always the threat of loss present irrespective of the market’s behavior at any one point in time.
Based on this paradigm we construct our keyword dictionaries to reflect the following salient investor emotions which constitute the key variables used in our analysis. Although different labels may be attached to underlying basic emotions by social psychologists, evolutionary psychologists and neuroscientists, ultimately the ecological validity of our particular context-specific word dictionaries, which, in fact, subsume those words relevant in a financial environment in these other word lists, is an empirical question. Specifically, we label our seven emotion variables:
Excitement
Mania
Anxiety
Panic
Revulsion/Blame
Denial
Guilt
For ease of exposition, we classify the first two generically as ‘exciting’ emotions, and the latter five as ‘anxious’ ones.
Our wordlists are mainly derived from media coverage of dot.com mania when each of our emotions is likely to be more intense. However, as we demonstrate below, applying these to subsequent market crisis events up to 20 years later, we find our wordlists work equally well, demonstrating their validity for the research questions we explore here.
Our keyword dictionaries are built by systematically analyzing financial media reports on a monthly basis from the beginning of the internet asset pricing bubble in October 1998 through March 2000, when it burst, until October 2002. We choose to work with this period because of the highly-charged nature of the internet bubble, and the whole gamut of powerful emotions directly manifest in a short period of time (e.g., Cassidy, Citation2002; Tuckett & Taffler, Citation2008). Importantly, this allows us to validate our dictionaries out-of-sample during market crises of a different nature occurring many years later.
In order to obtain a representative sample of media reports for dictionary development and analysis purposes, we search the Factiva database for each month from October 1998 to September 2002 using two groups of search terms:
Group 1: Technology, Internet, Computer, Com, New Economy, Web, E-commerce, Dotcom, PC, NASDAQ, Bubble
Group 2: Stock, Market, Stock Market, Share, Share Price, IPO, Crash
Articles selected for analysis need to be in English, include at least one term from both groups of search terms, and at least 500 words long.Footnote7 We also restrict our search to US media since the dot.com bubble was largely a US phenomenon. Specifically, we draw on the three most widely circulated daily newspapers in the US (The Wall Street Journal, The New York Times, and The Washington Post), Dow Jones Newswires, Barron’s, Forbes, and the Financial Times. These publications offer enough variety to provide a wide range of different stories and salient emotions as the dot.com bubble unfolded. We first sort the articles by relevance from the most to the least mentions of our search terms, and drop those which are merely summaries of current market developments, firm reports, and duplicates from analysis.
The first 15 most relevant articles each month are used in dictionary building, and all words with a clear emotional content in these 720 articles are separately extracted by each of the three authors working independently, tabulated and aggregated. This initial word list is then supplemented with new words taken from the pleasure, pain, excitation, emotion, moral approval, disapproval, fail, and negation categories from the merged Harvard IV General Inquirer and Lasswell Value keyword dictionaries.Footnote8
All words in this meta keyword list are then checked for appropriateness for our purposes by executing a keyword-in-context (KWIC) search by scanning these 720 articles, and assessing whether the words selected resonate emotionally in our context or not. Synonyms of the words in our list are then added from the Cambridge English Dictionary, and checked to see if they appear in this corpus in any frequency.
Care needs to be taken when counting words based on their word roots. For example, while we need to count all pure ‘rage’ words as well as ‘rages’ and ‘raged’, words such as ‘average’, ‘encourage’, and ‘coverage’ have to be ignored. To avoid such miscounting, it is necessary to include all relevant words derived from a particular word root. In the mentioned example, instead of only taking into account ‘rage’, the words ‘raged’ and ‘rages’ also have to be included separately in the respective keyword dictionary. Word roots with three or fewer mentions in the 720 articles are excluded to keep our dictionaries to manageable size, and without any measurable impact on our subsequent empirical results.
We also remove words that might be used in a different context or have another meaning in the majority of the articles from our dictionaries. For example, the word ‘terror’ needed to be excluded as following the terrorist attacks of the 11th of September 2001 it is overwhelmingly used in this context in articles.Footnote9
Some words such as ‘bear’ and ‘gamble’ often appear in word combinations which are not appropriate for our analysis. ‘Bear’ is often used in combination with Stearns (Bear Stearns), and ‘gamble’ in combination with Procter (Procter & Gamble). In such cases both the word combination as well as the single word are searched for and the total count of the word combination is subtracted from the single word count to ensure that only appearances of the word in the intended context are used in analysis. Lastly, to ensure potentially important emotion words are not missed, other possibly relevant words are added from the Book of Human Emotions (Smith, Citation2015) and then checked for their appropriateness in the context of the dot.com bubble using KWIC analysis.
This whole dictionary building process generates 251 distinct word roots with clear emotional content and relevance for our purposes. Taking into account all their different appropriate word forms results in the total of 835 keywords we employ in our textual analysis. These are provided in the on-line internet Appendix A to this paper.
Finally, our root keywords are allocated to our emotion categories: excitement, mania, anxiety, panic, revulsion/blame, denial, and guilt. To ensure robust and valid keyword dictionary construction each of the three authors of this paper first independently classified all the 251 emotion word roots to one of our seven emotion word categories. Then, when the initial assignments differed, these differences were resolved via discussion, and reference back to the keyword-in-context analysis. lists the five most common words in each of the seven emotion keyword dictionaries.
Table 1. Five most common words in each key word emotion category.
3.2. Article analysis
Since the driving forces behind the three crisis periods we explore are distinct, articles for our main textual analysis are identified for our three periods separately. For the dot.com period, we use the same criteria as those employed for dictionary building purposes.Footnote10 However, for the Global Financial Crisis period, we use Lexis/Nexis and ABI/Inform, and limit our article search to the following keywords: “S&P”, “S&P 500”, “Standard and Poor”, “Standard and Poor 500”, and “Stock market” and only work with articles with relevance score > 80%.Footnote11 For the COVID-19 pandemic we employ Factiva again using the same keywords as for the GFC and inspect all headlines and, if necessary, the articles themselves for relevance.Footnote12
3.3. Variable creation
To measure the emotional content of financial media narratives during our three market crisis periods, the salience of each investor emotion each month is computed as follows:Footnote13
(1)
(1)
We test our propositions by exploring the emotional impact of economic narratives on investor behavior in terms of both market return and market uncertainty, the latter we proxy for with a number of alternative variables. These are market volatility, trading volume, and the economic policy uncertainty (EPU) index of Baker et al. (Citation2016). Although trading volume is conventionally viewed as a measure of trading activity (e.g., Chordia et al., Citation2001), or liquidity (e.g., Avramov & Chordia, Citation2006), Banerjee and Kremer (Citation2010) show theoretically how disagreement between investors leads to higher volatility, and increased trading volume. Barinov (Citation2014) shows empirically how trading volume actually proxies for uncertainty which he measures in a number of different ways. In parallel, Carlin et al. (Citation2014) show how asset pricing disagreements lead to increased return volatility and greater trading volume, although volatility, itself, does not result in higher trading activity save in the case of increased levels of uncertainty/disagreement. We scale dollar trading volume by total market capitalization to control for the impact of price increases or decreases. The Baker et al. (Citation2016) EPU index is financial media news-based and measures economic policy uncertainty directly by virtue of its construction. It is computed as the number of articles in 10 leading newspapers containing terms pertaining to uncertainty, economy, and policy, scaled by total number of articles in that month, normalized and standardized. Our main uncertainty analysis is conducted employing this time series in preference to the macro-economic focused uncertainty estimates of Jurado et al. (Citation2015) as this latter is derived from a large number of macro-economic and financial indicators, not narratives.Footnote14 In the case of the internet bubble, we measure market return using the Dow Jones Internet index (DJII). Stock volatility is measured by the standard deviation of daily returns of the DJII in month t. Trading volume is calculated as the average of the daily total value of shares traded divided by end of day market capitalization of the DJII over month t.
With the Global Financial Crisis and the COVID-19 pandemic events, which were economy-wide, we work with return on the S&P 500 and, in parallel, proxy uncertainty using three variables. These are VIX (the CBOE S&P 500 options-based volatility index, which is also colloquially known by other names such as 'fear gauge’ or 'fear index’), trading volume measured as above save that we use the S&P 500 index instead of the DJII, and EPU.
We obtain the index data to calculate returns and standard deviation, and values of VIX from Datastream and Bloomberg. GDP data is from the Federal Reserve Bank of St. Louis website (https://fred.stlouisfed.org), and the EPU data is taken from https://www.policyuncertainty.com/us_monthly.html.
3.3.1. Event periods examined
We define the duration of the internet bubble period as covering the 48 months from October 1998 to September 2002. During its up phase between October 1998 and February 2000, the Dow Jones Internet index rose by 460%, reaching its peak in early March 2000. It then lost 94.0% of its value by the end of September 2002 (our down phase).
In the case of the Global Financial Crisis, we delineate this as lasting 45 months, from October 2007 to June 2011. Post the end of the internet bubble, and the concurrent collapse in the S&P 500, the index recovered strongly in 2003, and was then relatively flat during 2004-05 registering a gain of only 14% over this two-year period, in line with its long-term average annual return. However, it started rising strongly again from the beginning of 2006, gaining 22% over the next 21 months, even as house prices started to decline. The S&P 500 broadly peaked in October 2007 and then fell by 57% reaching its lowest point in early March of 2009, accompanied by further collapse in house prices, and the “Great Recession”. We classify this period, October 2007 to February 2009, as the down phase of the GFC. Finally, the market started to recover and, by the end of June 2011, the S&P 500 had gone up over 90%. We categorize this period of market recovery as our up phase.
COVID-19, our third event period, runs for 29 months from February 2020 to June 2022. The S&P 500 peaked on 19 February 2020 and then fell by a third over the following month reaching a low on 23 March 2020 when governments around the world started imposing lock-downs in an attempt to limit the spread of COVID-19. The market then started to recover as governments took concerted action to reduce the impact of the pandemic on the economy and was up by two-thirds from the March low by the end of 2020. It continued its upward trajectory peaking at 4,796 on 3 January 2022, more than double its March 2020 level. It then fell by 21% over the next six months as concerns about the overall state of the economy started to emerge. We categorize the 8 months February and March 2020 and January-June 2022 as our down phases with the 21 months from April 2020 to December 2021 as our up phase.
3.4. Method
While we would like to be able to establish a direct causal relationship between emotions on the one hand, and market returns and uncertainty on the other, this is not possible to do for several reasons. Even if causality exists it inevitably can go both ways and, in any case, is impossible to disentangle, as emotional responses to new stimuli occur effectively immediately. As Shiller (Citation2019) points out “…new contagious narratives cause economic events, and economic events cause changed narratives” (p. 71) – illustrates. In line with this, Griffith et al. (Citation2020) show that the MarketPsych sentiment daily data has only a mixed and very short-term impact on S&P 500 returns, and these effects are bi-directional. Therefore, as with Shen et al. (Citation2023a), who also work with the same data set on a contemporaneous basis, we limit our analysis to exploring the synchronous relationships between market prices and crisis events as being of intrinsic interest in their own right. We argue that if we want to enhance our ability to anticipate and manage major economic and market events, understanding the manner in which a powerful confluence of stories is associated with the emotions which drive investor behavior is crucial.
To test our first two propositions about the relationship between emotions and market dynamics, we employ contemporaneous regressions of the form:
(2)
(2)
and
(3)
(3)
Where:
Returnt is the return on the market index (Dow Jones Internet Index for the internet bubble period, and S&P 500 Index for the Global Financial Crisis and COVID-19 periods) during month t,
Emotiont is the standardized value (using Equationequation (1)(1)
(1) ) of one of our seven emotions, depending upon the specification, all measured over month t.
Uncertaintyt is measured in three ways: (1) The standard deviation of daily returns on the Dow Jones Internet Index during month t for the internet bubble period (StDevt), or the value of the VIX index at the end of month t for the GFC and COVID-19 periods (VIXt), (2) Trading volume (Volumet) calculated as the average of daily total value of shares traded divided by end of day market capitalization of the DJII (dot.com), or S&P500 (GFC and COVID-19) index over month t, and (3) The news-based Economic Policy Uncertainty Index value (EPUt) at the end of month t, obtained from www.policyuncertainty.com (based on Baker et al., Citation2016).
We employ the standard deviation of the DJII,Footnote15 and its trading volume, for the first economic event we study because the phenomenon was largely restricted to internet stocks with the wider economy not directly affected in terms of overall GDP growth rate, as illustrates. Since the effects of the Global Financial Crisis and COVID-19 Pandemic were much more pervasive, we use S&P 500 returns, VIX, and S&P 500 trading volume in these periods.Footnote16
Our third proposition relates to differences in emotions in the up and down phases of our three event periods as defined in the previous section.
4. Results
This section provides descriptive statistics for our data and then tests each of our three propositions relating to how economic narratives reflect and drive market events through the agency of investor emotions.
4.1. Descriptives
In order to examine whether our emotion variables derived from our economic narratives ([ii] in ), which are determined independent of the market, are measuring underlying investor constructs, we explore their associations with market returns and uncertainty ([iii] in ). presents the simple correlations between emotions, market returns, and uncertainty (proxied by volatility, trading volume, and economic policy uncertainty).
Table 2. Correlation matrix: Emotions, market returns, and uncertainty – Internet bubble.
In the case of the dot.com bubble period (October 1998 to September 2002), shows that exciting emotions (excitement and mania) are positively correlated (r = 0.43) with each other, as well as with concurrent Dow Jones Internet Index monthly returns (r = 0.46 and 0.29 respectively). In parallel, anxious emotions (anxiety, panic, revulsion/blame, denial, and guilt) are all strongly positively correlated with each other (at between 0.56 and 0.78), and also have strong negative correlations with contemporaneous market returns (ranging from −0.21 to −0.49). These relationships provide preliminary evidence investor emotions and market returns are closely related.
similarly provides clear evidence of a strong relationship between investor emotions and market uncertainty, as measured by standard deviation of DJII returns, although only significant for the strongest anxious emotions anxiety and panic (r = 0.37; 0.30). In parallel, anxious emotions are also highly correlated with news-based economic policy uncertainty (EPU) (ranging from 0.41 to 0.76), while exciting emotions are uncorrelated with EPU. Unlike the other uncertainty proxies, trading volumes are higher when exciting emotions dominate. The limited nature of the dot.com phenomenon with respect to the broader economy is shown by the low correlation of DJII volatility and trading volume with the other uncertainty measures in the table.
Broadly speaking, provides similar results for our Global Financial Crisis period (January 2006 to June 2011) although the correlation of mania with returns is insignificant as the market up phases were relatively gentle in comparison. The relationship between anxious emotions and volatility (measured by VIX) is much stronger in this period, with most correlations exceeding 0.45. In contrast to the new economy period, all three uncertainty proxies (VIX, trading volume, and EPU) are positively and highly correlated with each other (minimum r = 0.72) showing the more pervasive market-wide nature of the Global Financial Crisis.
Table 3. Correlation matrix: Emotions, market returns, and uncertainty – Global Financial Crisis.
In the case of the COVID epidemic, provides a broadly parallel picture to for the GFC. Excitement and mania are positively correlated with r = 0.41; also most of the anxious emotions are significantly intercorrelated. Anxiety and panic are strongly negatively correlated with contemporaneous market returns and, interestingly, our five anxious emotions are highly correlated with each of our three market uncertainty measures, VIX, trading volume and EPU, with correlation coefficients significant at p < 0.01 in most cases.
Table 4. Correlation matrix: Emotions, market returns, and uncertainty – COVID-19 pandemic.
Our correlation analyses thus provide clear evidence consistent with our main premise that media narrative-derived investor emotions, measured independently, are closely associated with both market returns and different measures of market uncertainty.
To explore our main proposition that there is a relationship between economic narratives and market behavior mediated by the emotions they reflect, we test the extent to which our seven salient emotions taken together help explain market pricing. presents the results of regressing market returns, volatility, trading volume, and EPU separately against our seven emotion variables jointly. Panel A reports respective adjusted R2s for each regression for the internet bubble period, and Panels B and C the equivalents for the Global Financial Crisis and COVID-19 epidemic period. All adjusted R2s (except for EPU in the GFC period) are significant at p < 0.01, and 10 out of the 12 in the three panels are 40% or above. In the case of the internet bubble and the COVID-19 pandemic, our emotion variables derived from media stories explain 40% of market returns, and for the GFC, 52%. Both these results are consistent with our main thesis.
Table 5. Contemporaneous regression: Emotions, market returns, and uncertainty during the internet bubble, the Global Financial Crisis, and the COVID-19 pandemic.
Perhaps of equal interest is that regressions of our measures of uncertainty on investor emotions have an average adjusted R2 of 49%.
4.2. Relationship between economic narratives and market dynamics
How are different media stories linked to market movements? To explore their association with market returns and market uncertainty in our three crisis periods reports the emotion variable coefficients in regressions (2) and (3).Footnote17 Panel A presents our results for the new economy bubble period, Panel B for the Global Financial Crisis, and Panel C for the COVID-19 pandemic. Panel A row (1) shows that exciting emotions have a strong positive association with contemporaneous returns (t = 3.5 and 2.0 for excitement and mania respectively) even after controlling for level of uncertainty as measured by the standard deviation of DJII returns (market volatility). In parallel, anxious emotions (with the exception of denial) have a strong negative relationship with returns. Row (2) shows only anxiety (t = 3.9), panic (t = 2.3), and denial (t = 2.3) are associated with the standard deviation of DJII returns, while row (3) shows only excitement (t = 3.5) and guilt (t = −2.2) have a significant relationship with trading volume. Finally, row (4) shows a strong relationship between anxious emotions and the policy uncertainty measure EPU.
Table 6. Contemporaneous regressions: Emotions, market returns, and uncertainty.
In the case of the GFC, Panel B row (1) shows a weaker relationship between emotions and returns. While excitement is strongly associated with returns (t = 3.2), mania is not. For the anxious emotions, we find panic is strongly and negatively associated with returns (t = 4.8), while denial is positively associated. Row (2) presents parallel results regressing VIX against investor emotion variables. This shows that while exciting emotions are not related with VIX, anxious emotions (except guilt) are strongly, and positively, associated with uncertainty demonstrating the powerful anxious emotions salient during periods of high market volatility. Row (3) shows similar results for trading volume. The relationship between emotions and EPU is weak as is seen in Row (4).
As regards the COVID-19 pandemic, , Panel C shows mania to be associated with market returns (t = 2.0). Anxiety, denial and guilt are now strongly associated with market movements (t = -2.0; 3.8; 2.5). Turning to Rows (2) to (4) showing the relationships between investor emotions and market uncertainty, although mania and VIX are weakly related (t = 1.8), much more interesting are how our five anxious emotions: panic, revulsion/blame, denial and guilt, are all (save anxiety) significantly associated with levels of VIX, trading volume and EPU; mainly with very high t-stats. Anxiety is only associated with trading volume (t = 2.9).
Consistent with our first proposition, demonstrates how different economic narratives are important in explaining market behavior, at least in the crisis periods we examine. Specifically, we show that exciting stories have a positive relationship with returns, while anxiety-generating ones have a negative relationship. Turning to our second proposition, also confirms our expectation that investor emotions as reflected in economic narratives are particularly salient in conditions of market uncertainty measured in terms of market volatility, trading volume and EPU, particularly the anxious emotions of anxiety, panic, denial, revulsion/blame, and guilt.
4.3. Economic narratives in the up and down phases of market crises
In this sub-section, we compare the nature of economic narratives during extreme market conditions to test our third proposition that positive (negative) media-derived emotions are more (less) salient when markets go up, and less (more) salient as they collapse. compares investor emotions, and level of uncertainty, across the growth and contraction phases of our three market crisis periods. In the case of the internet bubble, column (1) of Panel A clearly shows, not surprisingly, excitement is much higher as new economy stock prices shoot up, while columns (3) to (7) that similarly anxious emotions predominate after the bubble bursts. With regard to our uncertainty measures, columns (8) to (10) show that while volatility (standard deviation of the Dow Jones Internet Index) does not differ in the up and down phases of the new economy bubble, trading volumes are much higher in the up phase, consistent with market participants becoming caught up in the excitement. Needless to say, economic policy uncertainty is much more manifest after the bubble bursts.
Table 7. Tests of differences in mean emotions and uncertainty in the up and down phases of our crisis periods.
With respect to the Global Financial Crisis, Panel B of , column (1), again shows how investor excitement is much greater as the market recovers from its nadir (March 2009 to June 2011) compared with its contraction phase (October 2007 to February 2009). Not surprisingly, anxiety, panic, and revulsion/blame (columns (3) to (5)) are much stronger as the market falls. However, uncertainty measures (columns (8) to (10)) present a different picture to those for the new economy bubble. In this case, volatility (as measured by VIX) is much higher during the down phase of the Crisis than the recovery phase, highlighting how uncertainty and anxious emotions are intimately linked. Similarly, trading volume is greater as the market collapses, possibly reflecting panic selling.Footnote18
For the COVID pandemic, Panel C is virtually identical to Panel B for the GFC. Both the exciting emotions, excitement and mania differ significantly in the up market phase compared to its down phase and the anxious emotions of anxiety, panic and revulsion/blame are all much higher again when the market collapses. VIX is similarly significantly higher during the market collapse periods and trading volume likewise, possibly again for similar reasons of panic selling, as during the GFC, in February-March 2020.
clearly supports our third proposition in showing that exciting media stories are particularly associated with the up phases of extreme market events and anxious ones with the down phases. Overall, our empirical findings are again consistent with our main thesis that economic narratives reflect investor emotions, and these are an integral part of market activity. show how anxious investor emotions are closely associated with market uncertainty. provides further direct evidence of this in terms of how the negative investor emotions reflected in media narratives are much stronger when market uncertainty is more salient in down market conditions.
5. Discussion and conclusion
In his book Narrative Economics, Shiller (Citation2019) describes the key role economic narratives play in helping to explain important economic events. However, he cautions against assuming that economic events always drive economic narratives, and argues (p. 75) “contagious stories are largely creative and innovative, not simply a logical reaction to economic events”. We argue it is the power of investor emotions, as reflected in economic narratives, which provokes spontaneous and powerful responses in line with System 1 intuitive and reflexive mental processes (e.g., Kahneman, Citation2011).
Our empirical analysis demonstrates how in seeking to understand the etiology of, inter alia, asset pricing bubbles, as well as market crises more generally, and manage these more effectively, we need to explore more formally the underlying emotional processes at work, and their drivers. In particular, focusing on three episodes of extreme market movements, the internet bubble, the Global Financial Crisis, and the COVID-19 pandemic, we show how popular narratives published in the media reflect powerful emotions which help explain investor behavior, and consequently market dynamics.
Specifically, we adopt formal textual analysis of financial media narratives to measure the relative strengths of a range of different market emotions derived from psychological theory as they change dynamically during our crisis events. We provide evidence consistent with our main thesis that economic narratives, investor emotions, and market prices are intimately linked. We demonstrate the power emotions reflected in popular stories have to explain contemporaneous market returns, and market uncertainty. Media-derived measures of investor emotions together can explain up to half of monthly market returns and around two thirds of market uncertainty. As expected, narratives that reflect exciting emotions dominate in the up phase of a bubble and recovery from a market crisis, and anxious ones after the bubble bursts and during the crisis.
Importantly, we show that the relationship of the key investor emotions we measure with market behavior is similar in our three crisis periods, although the individual relationships of different emotions with market return and uncertainty measures are subtly different in some cases. In this context, it would be interesting to explore how economic narratives and associated investor emotions change over time as our different market crisis events evolve in the manner of Taffler et al. (Citation2022) in the case of the Chinese stock market bubbles.
This paper adds to the nascent empirical literature on narrative economics, as well as seeking to increase understanding of the morphology of financial crises in general. First, it suggests that in seeking to explain asset pricing bubbles formal models could usefully take into account the emotions stories propagated, inter alia in the financial media reflect. Our empirical evidence clearly suggests these closely mirror market behavior, at least during such extreme market events. Second, methodologically we demonstrate how it is possible to measure different market emotions directly through systematic analysis of economic narratives. Third, we construct original context-dependent emotion keyword dictionaries which are novel in the literature and have the potential for wider application, and show how such simple bag-of-words approaches can be powerful in the analysis of market stories. We also demonstrate how our emotion keyword dictionaries constructed using one major market bubble work equally well when used to analyze very different market crisis episodes up to two decades later. Finally, we show how high levels of anxiety, panic, and related emotions are associated with market uncertainty and that this can be empirically measured.
Our results open up a number of interesting areas for further research. These include analyzing other stock market bubbles such as the two recent Chinese episodes, in a parallel way, property bubbles such as those in Ireland and Japan, and other types of extreme market events, and the market prices of highly speculative assets (e.g., cryptocurrencies and ‘meme’ stocks). Andrade et al. (Citation2016) and Breaban and Noussair (Citation2018) create experimental asset markets designed to generate asset pricing bubbles and confirm how emotions and market dynamics are closely related. Our findings suggest the opportunity for developing experimental markets with greater verisimilitude which might include introducing economic narratives into the experimental design to allow more detailed investigation of the joint role played by stories and emotions in determining investor behavior. Likewise, how investor emotions impact the market pricing of individual stocks (Bin Hasan, Kumar and Taffler, Citation2023).
In line with Shiller (Citation2019), we acknowledge how individual economic narratives may be highly entertaining and of intrinsic interest in their own right. However, in seeking to understand the role played by potentially different and conflicting stories in major economic events, it is not necessary to have to employ a large number of carefully trained research assistants to analyze and classify these directly, as Shiller suggests in his final chapter. We demonstrate directly how it is possible to disentangle the relationship between a constellation of narratives and market dynamics by explicitly focusing on, and formally measuring, the different emotions associated with the often complex and contradictory web of stories.
Disclosure statement
No potential conflict of interest was reported by the authors.
Notes
1 For example, Shiller (Citation2017) lists the following illustrative narratives for the Great Depression: the stock market drop on October 28, 1929, moralizing about the excesses of the “Roaring Twenties”, a repeat of 1920-1921, the shopping behavior of housewives, the rising leftist or Communist movement and associated conspiracy theories, the frightening narratives coming out of Europe and Asia including the Japanese occupation of Manchuria, the genocide of ethnic Ukrainians by Stalin, the rise of Hitler, and stories about business people committing suicide.
2 In this paper we focus on the key role ‘integral’ or fundamental emotions such as excitement, anxiety, denial and guilt (Loewenstein & Lerner, Citation2003) which are often unconscious and at sufficient levels of intensity can strongly impact investment decisions. This is in contrast to ‘incidental’ emotions such as mood and sentiment (e.g., Hirshleifer & Shumway, Citation2003; Edmans et al., Citation2007; Hirshleifer et al., Citation2020; Edmans et al., Citation2021) which have a more general and short-lived effect on decision making.
3 These are likely to be equally caught up emotionally by the same contagious stories and dramatic market movements which will be reflected in what they write, again reinforcing the different feedback loops in Figure 1.
4 “Sense making is a search for plausibility and coherence that is reasonable and memorable, which embodies past experience and expectations, and maintains a self while resonating with others. It can be constructed retrospectively, yet used prospectively, and captures thoughts and emotions… [I]t renders the subjective something more tangible.” (Weick, Citation1995, p. 14).
5 Shiller (Citation2019, p. 280) also points out how psychoanalysis can help economists understand “people, their behavior, and their thinking” and how “the study of narratives naturally leads to psychoanalysis” (p. 16).
6 Although specially designed dictionaries are, as Loughran and McDonald (Citation2016) point out, often called lexicons we continue to use the term dictionaries to avoid confusion.
7 We employ a minimum of 500 words as we found it takes roughly these many words to get a sense of what the overall text is saying.
9 Other examples include ‘revolt’ (primarily used in the context of Venezuelan politics around this time), ‘wonder’ (used in both question and excitement contexts), and ‘concern’ (used both to indicate anxiety and as a company designation).
10 To reduce any potential small sample bias in our empirical results, the 150 most ‘relevant’ articles were content analyzed each month.
11 In the case of the GFC period, we are grateful to Mohammad Shehub Bin Hasan for providing our article corpus drawing on 21 different US newspapers including USA Today but excluding The Financial Times. Dow Jones Newswires, Barron’s and Forbes are also not accessed. In total we work with 18,453 articles from January 2004 to June 2011.
12 Again, we are grateful to Dr. Bin Hasan for assisting with this article extraction process. We broadly restrict ourselves to a similar range of newspaper sources to those we use in our Global Financial Crisis period analysis for consistency purposes.
13 We standardize by the total number of words since these vary significantly month-by-month. A higher frequency of the number of counted words in a certain category can be either due to higher emotional content or simply because more words are available in that month.
14 However, we rerun all our analyses separately substituting Jurado et al.'s (Citation2015) financial uncertainty, macro-economic uncertainty, and real uncertainty variables for EPU, and find similar empirical results. These are available on request.
15 We are grateful to Standard & Poor’s for providing this data.
16 We do, however, run all our tests with VIX in the dot.com period, and standard deviation of S&P 500 returns in the GFC and COVID-19 periods, and find the tenor of our conclusions remains unchanged.
17 Intercepts and the control variable coefficients are not reported for parsimony reasons.
18 We speculate that the differences between the two crises might be reflecting that in the dot.com case there were no buyers as many of these stocks were effectively worthless, whereas in the case of the GFC, stocks were fundamentally viable in most cases, but simply ‘worth less’.
References
- Adämmer, Philipp, and Rainer A. Schüssler. 2020. “Forecasting the Equity Premium: Mind the News!.” Review of Finance 24 (6):1313–55. 10.1093/rof/rfaa007.
- Aggarwal, Rajesh, Sanjai Bhagat, and Srinivasan Rangan. 2009. “The Impact of Fundamentals on IPO Valuation.” Financial Management 38 (2):253–84. 10.1111/j.1755-053X.2009.01035.x.
- Aliber, Robert Z., and Charles P. Kindleberger. 2015. Manias, Panics and Crashes: A History of Financial Crises. 7th ed. Basingstoke, Hants: Palgrave Macmillan.
- Andrade, Eduardo B., Terrance Odean, and Shengle Lin. 2016. “Bubbling with Excitement: An Experiment.” Review of Finance 20 (2):447–66. 10.1093/rof/rfv016.
- Avramov, Doron, and Tarun Chordia. 2006. “Asset Pricing Models and Financial Market Anomalies.” Review of Financial Studies 19 (3):1001–40. 10.1093/rfs/hhj025.
- Baker, Scott, Nicholas Bloom, and Steven Davis. 2016. “Measuring Economic Policy Uncertainty.” The Quarterly Journal of Economics 131 (4):1593–636. 10.1080/01422419908228843. 10.1093/qje/qjw024
- Banerjee, Snehal, and Ilan Kremer. 2010. “Disagreement and Learning: Dynamic Patterns of Trade.” The Journal of Finance 65 (4):1269–302. 10.1111/j.1540-6261.2010.01570.x.
- Barinov, Alexander. 2014. “Turnover: Liquidity or Uncertainty?” Management Science 60 (10):2478–95. 10.1287/mnsc.2014.1913.
- Bhattacharya, Utpal, Neal Galpin, Rina Ray, and Xiaoyun Yu. 2009. “The Role of the Media in the Internet IPO Bubble.” Journal of Financial and Quantitative Analysis 44 (3):657–82. 10.1017/S0022109009990056.
- Bin Hasan, Shehub, Alok Kumar, and Richard Taffler . 2023. Anxiety, Excitement, and Asset Prices. University of Miami Business School Research Paper No. 3902654. 10.2139/ssrn.3902654
- Bird, Ron, David R. Gallagher, Ahmed Khan, and Danny Yeung. 2023. “Do Emotions Influence Investor Behavior?” Journal of Behavioral Finance:1–22. 10.1080/15427560.2023.2282966.
- Breaban, Adriana, and Charles N. Noussair. 2018. “Emotional State and Market Behavior.” Review of Finance 22 (1):279–309. 10.1093/rof/rfx022.
- Call, Andrew C., Scott A. Emett, Eldar Maksymov, and Nathan Y. Sharp. 2022. “Meet the Press: Survey Evidence on Financial Journalists as Information Intermediaries.” Journal of Accounting and Economics 73 (2–3):101455. 10.1016/j.jacceco.2021.101455.
- Carlin, Bruce I., Francis A. Longstaff, and Kyle Matoba. 2014. “Disagreement and Asset Prices.” Journal of Financial Economics 114 (2):226–38. 10.1016/j.jfineco.2014.06.007.
- Cassidy, John. 2002. Dot. Con: The Greatest Story Ever Sold. New York: Harper Collins.
- Chordia, Tarun, Richard Roll, and Avanidhar Subrahmanyam. 2001. “Market Liquidity and Trading Activity.” The Journal of Finance 56 (2):501–30. 10.1111/0022-1082.00335.
- Currie, Mark. 1998. Postmodern Narrative Theory. New York: St. Martin’s Press.
- Dougal, Casey, Joseph Engelberg, Diego García, and Christopher A. Parsons. 2012. “Journalists and the Stock Market.” Review of Financial Studies 25 (3):639–79. 10.1093/rfs/hhr133.
- Edmans, Alex, Adrian Fernandez-Perez, Alexandre Garel, and Ivan Indriawan. 2021. “Music Sentiment and Stock Returns around the World.” Journal of Financial Economics 145 (2):234–54. 10.1016/j.jfineco.2021.08.014.
- Edmans, Alex, Diego García, and Øyvind Norli. 2007. “Sports Sentiment and Stock Returns.” The Journal of Finance 62 (4):1967–98. 10.1111/j.1540-6261.2007.01262.x.
- El-Haj, Mahmoud, Paul Rayson, Martin Walker, Steven Young, and Vasiliki Simaki. 2019. “In Search of Meaning: Lessons, Resources and Next Steps for Computational Analysis of Financial Discourse.” Journal of Business Finance & Accounting 46 (3–4):265–306. 10.1111/jbfa.12378.
- Engelberg, Joseph E., and Christopher A. Parsons. 2011. “The Causal Impact of Media in Financial Markets.” The Journal of Finance 66 (1):67–97. 10.1111/j.1540-6261.2010.01626.x.
- Fisher, Walter R. 1984. “Narration as a Human Communication Paradigm: The Case of Public Moral Argument.” Communication Monographs 51 (1):1–22. 10.1080/03637758409390180.
- Galbraith, John K. 1993. A Short History of Financial Euphoria. New York, NY: Penguin Books.
- Gamson, William A., David Croteau, William Hoynes, and Theodore Sasson. 1992. “Media and the Social Construction of Reality.” Annual Review in Sociology 18:373–93. 10.1177/009365084011003001.
- Griffith, John, Mohammad Najand, and Jiancheng Shen. 2020. “Emotions in the Stock Market.” Journal of Behavioral Finance 21 (1):42–56. 10.1080/15427560.2019.1588275.
- Henry, Elaine, and J. Andrew Leone. 2016. “Measuring Qualitative Information in Capital Markets Research: Comparison of Alternative Methodologies to Measure Disclosure Tone.” The Accounting Review 91 (1):153–78. 10.2308/accr-51161.
- Hirshleifer, David, and Tyler Shumway. 2003. “Good Day Sunshine: Stock Returns and the Weather.” The Journal of Finance 58 (3):1009–32. 10.1111/1540-6261.00556.
- Hirshleifer, David, Danling Jiang, and Yuting Meng DiGiovanni. 2020. “Mood Beta and Seasonalities in Stock Returns.” Journal of Financial Economics 137 (1):272–95. 10.1016/j.jfineco.2020.02.003.
- Jurado, Kyle, Sydney Ludvigson, and Serena Ng. 2015. “Measuring Uncertainty.” American Economic Review 105 (3):1177–1216. 10.1016/0305-0483(75)90069-9. 10.1257/aer.20131193
- Kahneman, Daniel. 2011. Thinking, Fast and Slow. New York, NY: Farrar, Strauss & Giroux.
- Knight, Frank H. 1921. “Cost of Production and Price over Long and Short Periods.” Journal of Political Economy 29 (4):304–35. 10.2307/1885951. 10.1086/253349
- Kury, Kenneth. 2014. “You Paid Too Much for That Dotcom Stock?: Sensemaking during Ecological Change.” Economics, Management, and Financial Markets 9 (2):66–94.
- Loewenstein, George, and Jennifer Lerner. 2003. “The Role of Affect in Decision Making.” In Handbook of Affective Sciences, edited by R. J. Davidson, K. R. Scherer, and H. H. Goldsmith, 619–42. Oxford, UK: Oxford University Press.
- Loughran, Tim, and Bill McDonald. 2011. “When is a Liability Not a Liability? Textual Analysis, Dictionaries, and 10-Ks.” The Journal of Finance 66 (1):35–65. 10.1111/j.1540-6261.2010.01625.x.
- Loughran, Tim, and Bill McDonald. 2016. “Textual Analysis in Accounting and Finance: A Survey.” Journal of Accounting Research 54 (4):1187–230. 10.1111/1475-679X.12123.
- Loughran, Tim, and Bill McDonald. 2020. “Textual Analysis in Finance.” Annual Review of Financial Economics 12 (1):357–75. 10.1146/annurev-financial-012820-032249.
- Mackay, Charles. 1852. Extraordinary Popular Delusions and the Madness of Crowds. 2nd ed. London: Office of the National Illustrated Library. Reprinted 1995. Ware, Herts: Wordsworth Editions.
- Mangee, Nicholas. 2021. How Novelty and Narratives Drive the Stock Market: Black Swans, Animal Spirits and Scapegoats. Cambridge, UK: Cambridge University Press.
- Ofek, Eli, and Matthew Richardson. 2003. “DotCom Mania: The Rise and Fall of Internet Stock Prices.” The Journal of Finance 58 (3):1113–37. 10.1111/1540-6261.00560.
- Peress, Joel. 2014. “The Media and the Diffusion of Information in Financial Markets: Evidence from Newspaper Strikes.” The Journal of Finance 69 (5):2007–43. 10.1111/jofi.12179.
- Polkinghorne, Donald E. 1988. Narrative Knowing and the Human Sciences. Albany: State University of New York Press.
- Quinn, William, and John D. Turner. 2020. Boom and Bust: A Global History of Financial Bubbles. Cambridge, UK: Cambridge University Press.
- Shen, Jiancheng, John Griffith, Mohammad Najand, and Licheng Sun. 2023a. “Predicting Stock and Bond Market Returns with Emotions: Evidence from Futures Markets.” Journal of Behavioral Finance 24 (3):333–44. 10.1080/15427560.2021.1975717.
- Shen, Jiancheng, Mohammad Najand, and Chen Chen. 2023b. “The Influence of Emotions on Cross-Section Returns: Tests for Cognitive Appraisal Theory.” Journal of Behavioral Finance:1–16. 10.1080/15427560.2023.2200187.
- Shiller, Robert J. 2014. “Speculative Asset Prices.” American Economic Review 104 (6):1486–517. 10.1257/aer.104.6.1486.
- Shiller, Robert J. 2017. “Narrative Economics.” American Economic Review 107 (4):967–1004. 10.1257/aer.107.4.967.
- Shiller, Robert J. 2019. Narrative Economics: How Stories Go Viral & Drive Major Economic Events. Princeton: Princeton University Press.
- Smith, Tiffany W. 2015. The Book of Human Emotions. London, UK: Wellcome Collection Profile Books.
- Taffler, Richard J, Xijuan Bellotti, Vineet Agarwal, and Linglu Li. 2022. “Investor Emotions and the Psychodynamics of Asset Pricing Bubbles: A Chinese Perspective.” Journal of Behavioral Finance :1–25. 10.1080/15427560.2022.2138391.
- Tetlock, Paul C. 2011. “All the News That’s Fit to Reprint: Do Investors React to Stale Information?” Review of Financial Studies 24 (5):1481–512. 10.1093/rfs/hhq141.
- Tuckett, David, and Richard Taffler. 2008. “Phantastic Objects and the Financial Market’s Sense of Reality: A Psychoanalytic Contribution to the Understanding of Stock Market Instability.” The International Journal of Psycho-Analysis 89 (2):389–412. 10.1111/j.1745-8315.2008.00040.x.
- Weick, Karl E. 1995. Sensemaking in Organizations. Thousand Oaks, CA: Sage.
Appendix A:
emotion dictionary key word lists
1. Excitement
Appetite, Appetizer, Appetizing, Awesome, Awesomeness, Boost, Boosted, Booster, Boosting, Boosts, Brilliance, Brilliant, Brilliantly, Bull, Bullish, Bulls, Celebrate, Celebrated, Celebrating, Celebration, Celebrity, Climb, Climbed, Climber, Climbing, Climbs, Comfort, Comfortable, Comforting, Confidence, Confident, Confidently, Curiosity, Curious, Delight, Delighted, Delightful, Desirable, Desirability, Desire, Desired, Desires, Desiring, Double, Doubled, Doubles, Doubling, Eager, Eagerly, Eagerness, Enthuse, Enthusiasm, Enthusiast, Enthusiastic, Enthusiastically, Excite, Excited, Excitement, Excites, Expand, Expanded, Expanding, Expands, Expansion, Fantastic, Fantastically, Ferocious, Ferociously, Flourish, Flourished, Flourishes, Flourishing, Gamble, Gambles, Gambling, Glorious, Glory, Happiness, Happy, Jump, Jumped, Jumping, Jumps, New high, New low, Optimism, Optimist, Optimistic, Optimistically, Popular, Popularity, Popularize, Popularly, Pride, Proud, Ramp up, Reliability, Reliable, Rise, Risen, Rises, Run up, Run-up, Satisfaction, Satisfied, Satisfy, Sensation, Sensational, Sensationally, Sexy, Shoot, Shooter, Shooting, Shoots, Shot, Speculate, Speculated, Speculates, Speculating, Speculation, Speculations, Steal, Stealing, Steals, Stole, Stolen, Success, Successful, Successfully, Superior, Superiority, Surprise, Surprised, Surprises, Surprising, Surprisingly, Triple, Tripled, Triplet, Unprecedented, Winner
2. Mania
Amaze, Amazed, Amazement, Amazes, Amazing, Amazingly, Astonish, Astonished, Astonishes, Astonishing, Astonishment, Balloon, Balloons, Bandwagon, Boom, Boomed, Booming, Booms, Bubble, Bubbling, Bubbly, Crazy, Cried, Cries, Delusion, Delusions, Ecstatic, Enormous, Enormously, Euphoria, Euphoric, Exotic, Explode, Exploded, Explodes, Exploding, Explosion, Explosive, Exuberance, Exuberant, Exuberantly, Fantasy, Fever, Feverish, Feverishly, Frantic, Frantically, Frenzied, Frenziedly, Frenzy, Furious, Giant, Gigantic, Gigantically, Gold rush, Huge, Hype, Hyped, Hyper, Hypes, Hyping, Hysteria, Hysterical, Illusion, Illusions, Incredible, Incredibly, Infatuation, Love, Loving, Lunacy, Lunatic, Magic, Magical, Mania, Maniac, Maniacal, Manic, Obsessed, Obsession, Obsessional, Obsessive, Obsessively, Obsessiveness, Overwhelm, Overwhelmed, Overwhelming, Overwhelmingly, Passion, Passionate, Phenomena, Phenomenal, Phenomenally, Phenomenon, Rage, Rages, Revolution, Revolutionary, Revolutionize, Revolutionized, Rocket, Rocketed, Rocketing, Self-Delusion, Skyrocket, Skyrocket, Skyrocketed, Skyrocketing, Stratosphere, Stratospheric, Triumph, Triumphant, Vast, Vastly, Wave, Waves, Zeal
3. Anxiety
Afraid, Anxiety, Anxious, Anxiously, Anxiousness, Avoid, Avoidance, Avoided, Avoiding, Avoids, Bear, Bearish, Bears, Calm, Caution, Cautionary, Cautioned, Cautioning, Cautions, Cautious, Confuse, Confused, Confuses, Confusing, Confusingly, Confusion, Cool, Cooled, Cooler, Cooling, Cooling off, Cooling-off, Cools, Cools off, Danger, Dangerous, Dangerously, Dangers, Dangers, Dead, Deadly, Death, Deathly, Depress, Depressed, Depressing, Depression, Die, Dies, Difficult, Difficulties, Difficultly, Dislike, Dislikes, Distress, Distressed, Distressing, Downfall, Dying, Endangers, Endangers, Excess, Excesses, Excessive, Excessively, Fall, Fallen, Falls, Falter, Faltered, Faltering, Falters, Fear, Feared, Fearful, Fearing, Fears, Fearsome, Flameout, Flame-out, Fright, Frighten, Frightened, Frighteningly, Frightens, Hazard, Hazardous, Jitter, Jitters, Jittery, Liable, Nerve, Nerves, Nerviness, Nervous, Nervously, Nervousness, Nervy, Overheated, Overhyped, Over-hyped, Pessimism, Pessimist, Pessimistic, Pessimistically, Pressure, Pressured, Pressures, Pressuring, Reluctance, Reluctant, Risk, Risked, Riskier, Riskiness, Risks, Risky, Scare, Scared, Scares, Scaring, Scary, Shake out, Shakeout, Shake-out, Shrink, Shrinkage, Shrinking, Shrinks, Stress, Stressed, Stresses, Stressful, Stressing, Struggle, Struggled, Struggles, Struggling, Threat, Threaten, Threatened, Threatening, Threatens, Tumble, Tumbled, Tumbles, Tumbling, Uncertain, Uncertainly, Uncertainty, Uncomfortable, Uncomfortably, Unease, Uneasily, Uneasiness, Uneasy, Unreliability, Unreliable, Volatile, Volatility, Vulnerability, Vulnerable, Wonderful, Wonderfully, Wondrous, Worried, Worries, Worry, Worrying
4. Panic
Bewildered, Bomb, Bombed, Bombing, Bombing, Bombs, Burst, Bursted, Bursting, Bursts, Chaos, Chaotic, Chaotically, Collapse, Collapsed, Collapses, Collapsing, Contagion, Contagious, Crash, Crashed, Crashes, Crashing, Crater, Craters, Craze, Crazed, Crazily, Craziness, Crisis, Crush, Crushed, Crushing, Dark, Darken, Darkest, Darkness, Desperate, Desperately, Disaster, Disastrous, Disastrously, Doom, Doomed, Dooming, Dooms, Drama, Dramatic, Dramatically, Drop, Dropped, Dropping, Drops, Earthquake, Free fall, Free-fall, Gloom, Gloomy, Hurricane, Implode, Implodes, Imploding, Implosion, Liquidate, Liquidated, Liquidates, Liquidating, Liquidation, Meltdown, Nightmare, Nightmarish, Out of control, Panic, Panicked, Panicking, Panicky, Panics, Paranoia, Paranoid, Plummet, Plummeted, Plummeting, Plummets, Plunge, Plunged, Plunges, Plunging, Shock, Shocked, Shocker, Shocking, Shocks, Slump, Slumped, Slumping, Slumps, Terrifying, Trouble, Troubled, Troublesome, Troubling, Turmoil
5. Revulsion/blame
Absurd, Absurdity, Absurdly, Accusation, Accuse, Accused, Accusing, Anger, Angrily, Angry, Apathy, Awful, Awfully, Bankrupt, Bankruptcies, Bankruptcy, Bankrupted, Blame, Blamed, Blameless, Blames, Blaming, Bore, Bored, Boredom, Boring, Condemn, Condemnation, Condemned, Condemns, Contempt, Crime, Criminal, Crises, Critic, Critical, Critically, Criticize, Criticism, Criticize, Criticized, Criticizes, Criticizing, Despair, Destroy, Destroyed, Destroying, Destroys, Destruction, Disappoint, Disappointed, Disappointing, Disappointment, Disappoints, Disillusion, Disillusioned, Disillusioning, Disillusionment, Egregious, Egregiously, Envy, Error, Errors, Fail, Failed, Failing, Fails, Failure, Fault, Faulty, Fraud, Fraudful, Fraudulent, Frustrate, Frustrated, Frustrates, Frustrating, Frustration, Greed, Greediness, Greedy, Horrible, Horribly, Horrific, Horrify, Horror, Hurt, Hurtful, Hurting, Hurts, Insolvency, Insolvent, Ludicrous, Ludicrously, Miserable, Miserably, Misery, Mislead, Misleading, Misleads, Misled, Mistake, Mistaken, Mistakenly, Mistakes, Pain, Pained, Painful, Painfully, Painless, Perverse, Perversely, Perverseness, Perversion, Perversity, Perverted, Punish, Punished, Punishing, Punishment, Recession, Recessionary, Recessions, Resentment, Responsibility, Responsible, Ridicule, Ridiculous, Ridiculously, Sad, Sadly, Scandal, Scandals, Scorn, Scorned, Scorning, Scorns, Suffer, Suffered, Suffering, Suffers, Taint, Tainted, Terrible, Terribly, Tragedy, Tragic, Uglier, Ugliest, Ugliness, Ugly, Upset, Upsets, Upsetting, Woe, Woeful, Woefully, Woefulness, Woes, Wrong, Wrongs
6. Denial
Annoy, Annoyance, Annoyed, Annoying, Annoyingly, Arrogance, Arrogant, Arrogantly, Contradict, Contradicted, Contradicting, Contradiction, Contradictions, Contradicts, Contradictory, Defence, Defenseless, Defend, Defended, Defending, Defends, Defense, Defenseless, Defensive, Defensively, Defiance, Defied, Defies, Defy, Denial, Denied, Denies, Deny, Disagree, Disagreed, Disagreeing, Disagreeing, Disagreement, Disagrees, Disbelief, Disbeliefs, Disbelieved, Disbelieving, Hope, Hoped, Hopeful, Hopefully, Hopes, Hoping, Hubris, Hubristic, Ignorance, Ignorant, Ignore, Ignored, Ignores, Ignoring, Impatience, Impatient, Overconfidence, Overconfident, Refusal, Refuse, Refused, Refuses, Refusing, Reject, Rejected, Rejecting, Rejection, Rejects, Resist, Resistance, Resistant, Resisted, Resisting, Resists, Shrug, Shrug off, Shrugging, Shrugs, Shrunken, Storm, Stormy, Unstoppable, Victim, Victimized, Victims, Warn, Warned, Warning, Warns
7. Guilt
Apologize, Apologized, Ashamed, Burn, Burned, Burner, Burning, Burns, Burnt, Confess, Confessed, Confesses, Confessing, Confession, Confessor, Cry, Cry out, Crying, Damage, Damaged, Damages, Damaging, Discomfort, Discomforted, Discomforting, Discomforts, Dismay, Dismayed, Embarrass, Embarrassed, Embarrassing, Embarrassment, Excuse, Excused, Excuses, Grief, Grieve, Guilt, Guilty, Regret, Regrets, Regrettably, Remorse, Resenting, Shame, Shameful, Shamefully, Sorry.