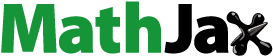
ABSTRACT
Natural fiber composites are being increasingly used in several fields, owing to their considerable cost, weight, and environmental benefits. The objective of this research is to study the effect of water absorption on the behavior of laminated biocomposites using flax (F) and sisal (S) fiber biocomposites with different stacking sequences (3S and 3F) and fiber orientation at 90° (F090s and S090s), as well as hybridizations (F/4S90/F and S/4F90/S) to reinforce an epoxy matrix. The kinetics of water diffusion within the proposed biocomposites was monitored and analyzed through a model-based optimization approach using artificial neural network (ANN) method. The results of this study showed that the amount of water absorbed by different types of biocomposites increased with time according to Fick’s law. Non-hybrid flax and sisal fiber composites (UD and (0/90°)s oriented) had lower water absorption rates than the hybrid orientation. It was also observed that sisal fiber was most sensitive to water absorption than flax. A high correlation of experimental data with the model was revealed which denoted that the ANN learning process was perfectly performed. Therefore, it was inferred that the ANN approach can accurately predict biocomposites water absorption.
摘要
天然纤维复合材料由于其相当大的成本、重量和环境效益,越来越多地应用于多个领域. 本研究的目的是研究吸水性对使用不同堆叠顺序(3S和3F)的亚麻(F)和剑麻(S)纤维生物复合材料(纤维取向为90°(F090和S090))以及杂交(F/4S90/F和S/4F90/S)增强环氧基料的层压生物复合材料性能的影响. 通过使用人工神经网络(ANN)方法的基于模型的优化方法,监测和分析所提议的生物复合材料中的水扩散动力学. 本研究结果表明,根据菲克定律,不同类型的生物复合材料吸收的水量随时间增加. 非杂交亚麻和剑麻纤维复合材料(UD和(0/90°)s取向)的吸水率低于杂交取向. 还观察到剑麻纤维比亚麻对吸水最敏感. 实验数据与模型具有很高的相关性,这表明ANN学习过程是完美的. 因此,可以推断ANN方法可以准确预测生物复合材料的吸水率.
Introduction
In recent years, innovations in the usage of biocomposite materials have been on the rise (El Hajj et al. Citation2012), as these materials offer considerable environmental benefits in addition to their remarkable low cost and weight. They are annually renewable, biodegradable, and recyclable (Das et al. Citation2022; Sekar et al. Citation2022). The development of natural fibers as reinforcing materials in biocomposites has been a matter of high interest to various industries and created a new durable biocomposite material for industry applications (Sanjay et al. Citation2019). Plant fiber applications are increasingly being developed mainly in the transport and leisure industries (Boumaaza et al. Citation2022; Rangappa et al. Citation2022). These fibers can be derived from wood stems such as flax, jute, and hemp (Boumaaza, Belaadi, and Bourchak Citation2021a), leaves such as sisal, pineapple, and banana (Chen, Miao, and Ding Citation2009), and fruits such as cotton, and coconut (Kazi, Eljack, and Mahdi Citation2020). Moreover, vegetable fibers are increasingly being used in place of glass fibers as reinforcement for composite materials. These fibers offer a judicious alternative from an environmental and mechanical point of view (Belaadi, Laouici, and Bourchak Citation2020; Benzannache et al. Citation2021; Tabet et al. Citation2021; Vigneshwaran et al. Citation2020).
Owing to the hydrophilic nature of plant fiber, the use of plant fiber biocomposites in exterior structures requires knowledge about their behavior in a humid environment (Liu et al. Citation2019). Prolonged exposure of plant fiber biocomposites to moisture and temperature can adversely affect their mechanical properties (Sekar et al. Citation2021). Indeed, these fibers are particularly sensitive to climatic conditions and the environment to which they are operating at Moisture is an important parameter to be taken into consideration, as it can cause serious structural failures and call into question the choice of plant fibers over synthetic fibers (Coniglio et al. Citation2013).
Several recent studies have investigated the water absorption kinetics of plant fiber biocomposites and its consequences. Scida et al. (Citation2013) studied the effect of moisture on the mechanical properties of epoxy matrix and flax fiber biocomposites by comparing two different types of architectures: unidirectional and twill. The results showed that both materials follow a Fickian absorption behavior. However, the authors noted that the biocomposite with twill reinforcement showed greater absorption kinetics than the one with unidirectional reinforcement. Their study also revealed that the saturation water absorption was greater for this type of architecture. Some studies investigated the effect of glass fiber-natural fiber hybridization on water absorption of composite materials to infer the influence of hybridization on water absorption. Panthapulakkal and Sain (Citation2007) evaluated the effect of hemp-glass hybridization on water absorption, thermal and mechanical properties of polypropylene (PP) matrix short-fiber hybrid composites. They found that hemp-glass hybridization significantly increased the resistance of the composites to water absorption. Yorseng et al. (Citation2020) developed a fully biobased hybrid bioepoxy composite reinforced with kenaf/sisal fibers to fabricate biobased hybrid composites and composite laminates. Their findings showed that the all-biobased hybrid bioepoxy composites reinforced with kenaf/sisal fibers exhibited moderate properties in comparison to the pure bioepoxy composite before and after environmental conditions. Vinod et al. conducted another study on hybrid hemp/jute/hemp composite (Vinod et al. Citation2022). The authors revealed that hybridization with hemp fiber as a skin layer and jute fiber as a core layer can improve the tensile and flexural strength, but it decreases the inter laminar shear strength. Another study was conducted by Boopalan, Niranjanaa, and Umapathy (Citation2013) to evaluate the mechanical and thermal properties of hybrid epoxy biocomposites reinforced with jute and banana fibers. For this purpose, jute and banana fibers with different weight ratios (100/0, 75/25, 50/50, 25/75, and 0/100) were prepared and then incorporated into an epoxy matrix using an injection molding technique to form biocomposites. The study results showed that the addition of banana fibers in jute/epoxy composites up to 50% by weight increased the mechanical and thermal properties and decreased the moisture absorption. The water absorption behavior of the hybrid sisal/polyester composite is investigated with the numerical model of Fick’s diffusion law (Vigneshwaran et al. Citation2020).
The water absorption behavior of the flax/epoxy biocomposites was initially linear (Fickian diffusion), then slowed down until the moisture content approached saturation level; the water absorption rate at this point was about 13.5% for each sample. Watanabe et al. (Citation2013) investigated several artificial neural network (ANN) models to evaluate and predict the final moisture content of individual Sugi (Cryptomeria japonica) samples during air drying. They found that the proposed ANNs could create highly nonlinear relationships between inherent wood properties as well as predict MCF with higher accuracy than the model. Ozsahin and Aydin (Citation2014) also used the ANN method to predict the optimum drying temperature of varnish for achieving good bonding in the manufacture of plywood sheets from beech and spruce varnishes, dried at temperatures of 20°C, 110°C, 150°C, and 180°C, and tested in shear. The researchers concluded that high drying temperatures could alter some physical, mechanical and, chemical characteristics of wood and cause drying-related defects.
In this investigation, the ANN model was used to establish nonlinear dependencies among the process input parameters (distilled water immersion test time and sisal/flax fibers filling content) and the response (moisture content absorbed by the biocomposite). Modeling the water uptake of an epoxy matrix loaded with non-hybrid and hybrid sisal/flax fibres has not previously been conducted in literature, thus justifying the topic selection for this paper. Consequently, the novelty of this study is to develop a numerical tool using the Fick’s model and a numerical model using ANN method, to predict and model the water absorption kinetics of plant fibers reinforced biocomposites. The tests were carried out on laminated biocomposites of flax and sisal fibers on one hand and hybrids on the other hand with a volume fraction of 40%, using an epoxy resin.
Materials and methods
Materials and manufacturing process
The reinforcement materials investigated in this study were flax and sisal fibers (). These fibers present a very interesting alternative, as they have the advantages of having highly competitive mechanical characteristics and being easily accessible. The constituents for this study were provided by the natural fiber cable and cord manufacturing plant in Blida, Algeria. The characteristics of these utilized materials are described in . The epoxy resin type MEDAPOXY STR with a density of 1.110 kg/m3 was used with an HY951 hardener to form the matrix; both these ingredients were provided by a company called GRANITEX Alger (Algeria) (Belaadi et al. Citation2020; Cherief et al. Citation2020, Citation2021). These laminates were prepared at room temperature (25°C) through contact molding and the setup was retained in the mold for 24 hours to ensure complete polymerization of the resin. They were produced in the form of 300 mm by 300 mm plates, and oriented as defined in (Belaadi, Amroune, and Bourchak Citation2019) as follows: 3 unidirectional (UD) plies with cross (0/90°) orientations and 6 plies for hybrid laminates placed as S/4F90/S and F/4S90/F. The obtained plates were left exposed to air for 7 days, and then cut into samples of dimension 30 mm by 30 mm using a diamond disk. Finally, the cutout samples were post-cured in an oven at a temperature of 70°C for 4 hours.
Figure 1. (a) Unidirectional sisal fibers, (b) unidirectional flax fibers, (c) epoxy resin used in this work and (d) example of biocomposites elaborated in this study.
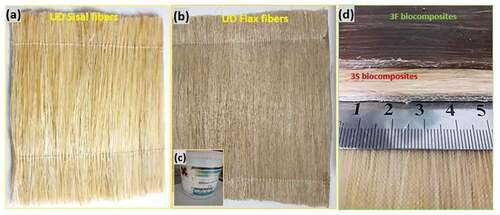
Table 1. Properties of the sisal, flax fibers and matrix used in the present work.
Table 2. Fiber lay-up and volume fraction of the various considered hybrid biocomposites.
Water absorption tests
Several methods can be used to estimate the amount of moisture absorbed by composite materials. The most widely used method is the gravimetric method, which involves testing a mass of the sample before and after aging (Dhakal, Zhang, and Richardson Citation2007). In this method, the evolution of the sample mass is observed in regular intervals over a total period exceeding 600 hours. For this purpose, a series of sample laminates of different thicknesses were taken from each biocomposite plate while their kinetics of water absorption was measured. The samples were weighed and immersed in distilled water, then removed from the bath and lightly wiped with absorbent paper to remove any water film present on the surface. The weighed samples were then immersed in water again and the process was repeated until complete saturation was reached. These tests were carried out according to ASTM standard methods (D1037–99, ASTM, 1999) at an ambient temperature of 23°C and a relative humidity of about 49%. The mass samples were taken on a KERN PF Bis balance with a precision of 0.0001 mg. The absorption rate of the composite material was calculated by finding the difference between the mass of the sample exposed to water and its mass in the unaged state.
Fick’s model
Moisture sensitivity of natural fiber composites is a major problem in various sectors, including automotive and marine industries (Gourier et al. Citation2014; Mokhothu and John Citation2015). This is because the presence of water molecules in these materials can affect their mechanical properties, thereby limiting their use. Many researchers have studied the water absorption kinetics of natural fiber biocomposites extensively (Cherif et al. Citation2016). Although the kinetics of water diffusion in a material can be modeled using different laws, the most widely used law is Fick’s law (Fick Citation1995). This law is widely used in the literature mainly due to its simplicity. Thus, after testing different mass samples over time, the characteristics related to the water absorption of the biocomposite were determined by finding out the mass percentage of water absorbed, Mt defined by equation 1,
where w0 is the mass of the unmerged sample at t = 0 and wt is the mass of the samples after a specific immersion time.
The absorption of water within a material is governed by the maximum absorption capacity (Mt) that corresponds to the maximum mass of water absorbable by the material and the diffusion coefficient (D) that expresses the speed of penetration into the environment considered. D is determined from the linear part of the gravimetric water absorption curve by the EquationEquation (2)(2)
(2) . Where, (k) is the slope of the linear part of the curve representing
.
ANN modeling
Artificial neural networks (ANNs) provide a model of computation based on biological neural networks. ANNs are composed of many interconnected parallel neurons that can optimally interact with each other, owing to their high precision and optimization. An ANN is generally made up of three layers (input layer, output layer, and hidden layer). Input layers: These layers contain the neurons that receive the input data (Adda et al. Citation2021; Safa and Samarasinghe Citation2011) where the number of neurons is directly related to the number of input variables. Then there is the output layer which is the layer that gives the results of the problem and between these two compartments are the hidden layers which are made up of neurons defining the internal activity of the network (Ighalo et al. Citation2021; Wang et al. Citation2020). ANNs are classified into two large families and based on the chosen interconnection; the first illustrates static networks (not looped), and the second represents so-called looped dynamic networks. Synapses interconnect neurons and allow distributed parallel processing. These synapses have the ability to establish complex relationships between numerical variables without requiring a preconceived model (Macedo et al. Citation2015). The ANN learning algorithm is more efficient when the sum of the squared errors is close to zero (Boumaaza, Belaadi, and Bourchak Citation2021b). The accuracy of the model given by ANN can be checked using the values of the correlation coefficient (R2) and the mean squared error (MSE). The lower the values of the MSE, the more precise the ANN model is in the prediction. The two parameters are given by equations 3 and 4 (Adeniyi, Ighalo, and Marques Citation2021).
Analysis of variance (ANOVA)
The results of the experiments were assessed using statistical analysis. One-way analysis of variance (ANOVA) was used to determine if there were any significant differences between the groups with respect to the architectures of the investigated biocomposites. The effectiveness of the ANOVA approach can be evaluated using different statistical parameters such as interval of confidence (CI), F-Fisher test, and probability. The F-test was utilized at a 0.05 level of significance, which represents a 95% confidence level.
Results and discussion
Water absorption
The water absorption rates recorded for the different biocomposites that were examined are presented in . In this figure, the increased of water absorption rates of flax and sisal fibers at different architectures is shown. It can be observed that the absorption curves of each biocomposite showed two different absorption areas. In the first area, the water absorption increases proportionally to the square root of the immersion time expressed in hours. This area is almost linear for water absorption values below 3%. This linear part of the curve gives information about the diffusivity of the water molecules, which depends on the speed at which the water penetrates the material. The second part is non-linear until saturation, i.e., the mass absorption increases progressively until the appearance of an equilibrium level called the saturation level. This bearing indicates the water mass absorbed by the material when the immersion time was up to 1400 h0.5 of immersion.The saturation weight gain Mm and the diffusion coefficient D are shown in . The analysis also shows that the 3S and 3F architectures are the least sensitive to water absorption, as the unidirectional layers exhibit good performance in the fiber direction. The 3S architecture has a lower percentage of absorption than the 3F architecture. This is due to the fact that the chains of the sisal fiber are connected by regularly reproduced hydrogen bonds between the groups, so their absorption is minimal (Célino et al. Citation2014; Komuraiah, Kumar, and Prasad Citation2014). The results obtained are very close to those reported by various other authors (Couture, Lebrun, and Laperrière Citation2016; Monti et al. Citation2017). It was also found that the orientation of the fibers had a significant impact on the water absorption: the S090s biocomposite had an absorption rate of 3.15%, while for the F090s biocomposite, this rate was 3.56%. Similarly, for hybrid reinforcements, the amount of water absorbed by the F/4S90/F biocomposite was higher than that of the S/4F90/S reinforcement; they were about 4.7% and 4.4%, respectively.
Table 3. Diffusion parameters for biocomposites laminate elaborated: Slope (k), diffusion coefficient (D) and water content at saturation (Mm).
ANN modeling
The ANN network was structured as a multilayer network with artificially generated neurons in each layer. The ANN is a multilayer perceptron (MLP) consisting of three layers. The first layer (input layer) consists of one neuron representing the independent variable that was already used in the Fick’s model. The second layer is a hidden layer. The last layer (output layer) is the response layer consisting of six neurons. The number of neurons required in the hidden layer was chosen to minimize the prediction error and also to reduce the number of neurons. A hidden layer with 10 neurons was selected to create robust ANN models where time was considered as an input parameter and the different architectures realized were considered as output parameters. The architecture of the developed ANN model is shown in .
The samples were distributed among 28 individuals for training and 4 for testing and validation (). During this study, the Levenberg-Marquardt algorithm was selected for ANN model training to predict the water uptake of the elaborated biocomposites. The network was repeatedly configured for training once the input and target data collection were performed. The discrepancies between the experimental data and the outputs generated by the ANNs are illustrated in . It can be noted that the MSE values were elevated at the initial stage of the ANN formation, but then got reduced in the later stages of the ANN training phase. The training of the ANN network finished with the MSE value, reaching the least in the fourth time epoch and when the gradient recorded an optimized validation performance of 0.0045858 at the fifth iteration. The graph shows the position where the lines representing the training, validation, and testing phases join the perfect line (best), indicating that the ANN network formation phase ended successfully.
The performance data of ANN in the training operation is presented in . It was observed that the initial error in the training process of ANN network occurred at the sixth epoch, continued for 6 repetitions, and ended at the tenth epoch. In the validation check that was conducted over a total of 10 epochs, the occurrence and recurrence of errors after the sixth epoch indicated an ideal fit of the data. An important part of the performance analysis of the ANN training process is the analysis of the error percentages of the ANN estimates. The difference in the error rates between the target value and the output values generated in the network throughout the training, validation, and testing phases are shown by means of an error histogram, which is distributed over an interval of 20 cells (). The lowest error values were found on and around the zero-error line when looking at the graph. Small error levels are a further indication of successful ANN training phase completion with minimum error levels.
shows a comparison between the ANN modeling results and the experimental data for linen/sisal/epoxy biocomposites during formation, validation, and testing. The variability of the experimental data is illustrated by the coefficient of determination, R2, which indicates the degree of prediction of the model (Boumaaza, Belaadi, and Bourchak Citation2021b). In the formation process, the proximity of the data points to the zero-error line shown by the dashed line and the line of equality drawn in blue, as well as the R-value of 0.99967, suggest that the formation process of the ANN network was perfectly performed. It was noted that the data points were close to the equality line and zero-error line, and that the obtained R-value was 0.99801 in the ANN network validation process. This proves that the ANN network validation phase was performed with minimal error rate. The R-value for the test phase is 0.99942. The data obtained in the test phase was located near both lines. It can be clearly seen from the graph that the test phase of the ANN network was completed with a very low error rate. In addition, it can be observed that the data obtained in all three phases of the ANN were along the fit line and the zero-error line. However, the R-value for all the data was obtained at 0.9995. The results of the ANN predicted values were close to the parity threshold () and they are in perfect agreement with those obtained experimentally (Ighalo et al. Citation2021). Thus, the ANN method gives a more reliable prediction of the water absorption levels of biocomposites.
ANOVA analysis
presents the water absorption results of flax and sisal fibers biocomposites taken by varying fiber architectures and determining whether significant differences existed between biocomposites consisting of 3-ply unidirectional UD (3S and 3F), cross orientations (F090s and S090s), and 6-ply hybrid laminates (S/4F90/S and F/4S90/F) with CI = 95%. The statistical terms used for the ANOVA were between-group (BG), within-group (WG), sum of squares (SS), degree of freedom (DF), mean square (MS), F-test statistic (F), and critical value (Fcrit) in order to confirm the effect of biocomposite structure type on the responses. The F-test statistic was used to determine if significant BG differences occurred in the range of the different biocomposites that were tested. If F was found to be superior to Fcrit, the null hypothesis would be dismissed, resulting in a significant variation in the mean value. This would indicate that the architectures were not all equally successful. If the value of F was found to be less than Fcrit, then the null hypothesis cannot be dismissed, which indicates that the results do not show a significant variation in the mean values. The significance of the P-value = .000 (p < .05) is less than the significance level (0.05); also, F = 24.02 is superior to the critical F = 2.26. Therefore, the null hypothesis that the means are equivalent can be rejected. The ANOVA results showed that there was a significant difference in the mean values between the different biocomposite structures as P (probability) is less than 0.05 and the F-value is above the critical value (Ighalo et al. Citation2021). The results suggest that the flax and sisal fiber biocomposites with different structures incorporated in an epoxy bio-resin exhibit a statistically significant change in water absorption. Group comparison reveals that the variance for the flax biocomposites is significantly lower than for the other sisal biocomposites. Additionally, the results identified whether the type of fiber as well as their orientations were able to provide a statistical improvement in the water absorption of these biocomposites.
Table 4. Variance analysis of moisture absorption at 95% level of significance.
Conclusion
Although the use of plant fibers in place of synthetic fibers can provide several benefits, the hydrophilic nature of plant fibers necessitates one to have knowledge about their performance in a humid environment, before actually putting them to use. The current study investigated the effect of water absorption on the behavior of flax/sisal/epoxy biocomposites built in different architectures. The following are the inferences drawn from the study: (1) The water absorption rates of both non-hybrid flax and sisal fiber composites (UD and (0/90°)s oriented) was lower when compared to the hybrid orientation. (2) Sisal fibers have a significant influence on the water diffusion process. Their water absorption was found to be lower than that of flax. (3) The water absorption pattern of all developed biocomposites was in accordance with the Fickian model. (4) ANN predicted values were close to the parity threshold; they were in perfect agreement with those obtained experimentally. Therefore, it was concluded to be the most appropriate approach as it provided a reliable prediction of the water absorption kinetics of flax/sisal biocomposites with a tolerable error quotient. The ANOVA results indicate that the flax and sisal fiber composites built into different structures and incorporated into an epoxy bio-resin revealed a statistically significant change in water absorption.
Highlights
The sisal fibers have a significant influence on the water diffusion process.
The water absorption pattern of all developed biocomposites was in accordance with the Fickian model.
The non-hybrid flax and sisal fiber composites exhibited low water absorption over the hybrid composites.
The ANN model based n the LM algorithm has the highest performance.
Ethics approval
The work contains no libelous or unlawful statements, does not infringe on the rights of others, or contains material or instructions that might cause harm or injury.
Acknowledgment
This project was funded by the Deanship of Scientific Research (DSR), King Abdulaziz University, Jeddah, under grant No. (D-1001-135-1443). The authors, therefore, gratefully acknowledge DSR technical and financial support.
Disclosure statement
No potential conflict of interest was reported by the author(s).
Additional information
Funding
References
- Adda, B., A. Belaadi, M. Boumaaza, and M. Bourchak. 2021. Experimental investigation and optimization of delamination factors in the drilling of jute fiber–reinforced polymer biocomposites with multiple estimators. International Journal of Advanced Manufacturing Technology 116 (9):2885–14. doi:10.1007/s00170-021-07628-9.
- Adeniyi, A. G., J. O. Ighalo, and G. Marques. 2021. Utilisation of machine learning algorithms for the prediction of syngas composition from biomass bio-oil steam reforming. International Journal of Sustainable Energy 40 (4):310–25. doi:10.1080/14786451.2020.1803862.
- Belaadi, A., S. Amroune, and M. Bourchak. 2019. Effect of Eco-FRiendly chemical sodium bicarbonate treatment on the mechanical properties of flax fibres: Weibull statistics. International Journal of Advanced Manufacturing Technology 106 (5–6):1753–74. https://doi.org/10.1007/s00170-019-04628-8.
- A. Belaadi, A. Bezazi, M. Bourchak, F. Scarpa, Tensile static and fatigue behaviour of sisal fibres, Mater. Des. 46 (2013). https://doi.org/10.1016/j.matdes.2012.09.048.
- Belaadi, A., M. Boumaaza, S. Amroune, and M. Bourchak. 2020. Mechanical characterization and optimization of delamination factor in drilling bidirectional jute fibre-reinforced polymer biocomposites. International Journal of Advanced Manufacturing Technology 111 (7–8):2073–94. doi:10.1007/s00170-020-06217-6.
- Belaadi, A., H. Laouici, and M. Bourchak. 2020. Mechanical and drilling performance of short jute fibre-reinforced polymer biocomposites: Statistical approach. International Journal of Advanced Manufacturing Technology 106 (5–6):1–18. doi:https://doi.org/10.1007/s00170-019-04761-4.
- Benzannache, N., A. Belaadi, M. Boumaaza, and M. Bourchak. 2021. Improving the mechanical performance of biocomposite plaster/Washingtonian filifera fibres using the RSM method. Journal of Building Engineering 33:101840. doi:https://doi.org/10.1016/j.jobe.2020.101840.
- Boopalan, M., M. Niranjanaa, and M. J. Umapathy. 2013. Study on the mechanical properties and thermal properties of Jute and Banana fiber reinforced epoxy hybrid composites. Composites Part B: Engineering 51:54–57. doi:10.1016/j.compositesb.2013.02.033.
- Boumaaza, M., A. Belaadi, and M. Bourchak. 2021a. The effect of alkaline treatment on mechanical performance of natural fibers-reinforced plaster: Optimization using RSM. Journal of Natural Fibers 18 (12):2220–40. doi:10.1080/15440478.2020.1724236.
- Boumaaza, M., A. Belaadi, and M. Bourchak. 2021b. The effect of alkaline treatment on mechanical performance of natural fibers-reinforced plaster: Part II optimization comparison between ANN and RSM statistics. Journal of Natural Fibers: 1–16, August. doi:10.1080/15440478.2021.1964129.
- Boumaaza, M., A. Belaadi, M. Bourchak, M. Jawaid, and S. Hamid. 2022. Comparative study of flexural properties prediction of Washingtonia Filifera Rachis biochar bio-mortar by ANN and RSM models. Construction and Building Materials 318:125985. doi:10.1016/j.conbuildmat.2021.125985.
- Célino, A., S. Fréour, F. Jacquemin, and P. Casari. 2014. ThE hygroscopic behavior of plant fibers: A review. Frontiers in Chemistry 1. doi:https://doi.org/10.3389/fchem.2013.00043.
- Chen, H., M. Miao, and X. Ding. 2009. Influence of moisture absorption on the interfacial strength of Bamboo/Vinyl ester composites. Composites: Part A, Applied Science and Manufacturing 40 (12):2013–19. doi:10.1016/j.compositesa.2009.09.003.
- Cherief, M., A. Belaadi, M. Bouakba, M. Bourchak, and I. Meddour. 2020. Behaviour of lignocellulosic fibre-reinforced cellular core under low-velocity impact loading: Taguchi method. International Journal of Advanced Manufacturing Technology 108 (1–2):223–33. doi:https://doi.org/10.1007/s00170-020-05393-9.
- Cherief, M., A. Belaadi, M. Boumaaza, and M. Bourchak. 2021. The effect of geometry on the flexural properties of cellular structures reinforced with natural fibres: Statistical approach. Journal of Natural Fibers 1–15. doi:10.1080/15440478.2021.1964134.
- Cherif, Z. E., C. Poilâne, A. Vivet, B. Ben Doudou, and J. Chen. 2016. About optimal architecture of plant fibre textile composite for mechanical and sorption properties. Composite Structures 140:240–51. doi:10.1016/j.compstruct.2015.12.030.
- Coniglio, N., K. Nguyen, R. Kurji, and E. Gamboa. 2013. Characterizing water sorption in 100% solids epoxy coatings. Progress in Organic Coatings 76 (9):1168–77. doi:10.1016/j.porgcoat.2013.03.011.
- Couture, A., G. Lebrun, and L. Laperrière. 2016. Mechanical Properties of Polylactic Acid (PLA) composites reinforced with unidirectional flax and flax-paper layers. Composite Structures 154:286–95. doi:10.1016/j.compstruct.2016.07.069.
- Das, O., K. Babu, V. Shanmugam, K. Sykam, M. Tebyetekerwa, R. E. Neisiany, M. Försth, G. Sas, J. Gonzalez-Libreros, A. J. Capezza, et al. 2022. Natural and industrial wastes for sustainable and renewable polymer composites. Renewable and Sustainable Energy Reviews 158:112054. doi:https://doi.org/10.1016/j.rser.2021.112054.
- Dhakal, H. N., Z. Y. Zhang, and M. O. W. Richardson. 2007. Effect of water absorption on the mechanical properties of hemp fibre reinforced unsaturated polyester composites. Composites Science and Technology 67 (7–8):1674–83. doi:10.1016/j.compscitech.2006.06.019.
- El Hajj, N., R. M. Dheilly, A. Goullieux, Z. Aboura, M. L. Benzeggagh, and M. Quéneudec. 2012. Innovant Agromaterials Formulated with Flax Shaves and Proteinic Binder: Process and Characterization. Composites Part B: Engineering 43 (2):381–90. doi:10.1016/j.compositesb.2011.05.022.
- Fick, A. 1995. On liquid diffusion. Journal of Membrane Science 100 (1):33–38. doi:10.1016/0376-7388(94)00230-V.
- Gourier, C., A. Le Duigou, A. Bourmaud, and C. Baley. 2014. MechanicaL analysis of elementary flax fibre tensile properties after different thermal cycles. Composites: Part A, Applied Science and Manufacturing 64:159–66. doi:10.1016/j.compositesa.2014.05.006.
- Ighalo, J. O., C. A. Igwegbe, A. G. Adeniyi, and S. A. Abdulkareem. 2021. Artificial neural network modeling of the water absorption behavior of plantain peel and bamboo fibers reinforced polystyrene composites. Journal of Macromolecular Science, Part B 60 (7):472–84. doi:10.1080/00222348.2020.1866282.
- Kazi, M. K., F. Eljack, and E. Mahdi. 2020. Optimal filler content for cotton fiber/PP composite based on mechanical properties using artificial neural network. Composite Structures 251:112654. doi:10.1016/j.compstruct.2020.112654.
- Komuraiah, A., N. S. Kumar, and B. D. Prasad. 2014. Chemical composition of natural fibers and its influence on their mechanical properties. Mechanics of Composite Materials 50 (3):359–76. doi:10.1007/s11029-014-9422-2.
- Liu, W., T. Chen, M. Fei, R. Qiu, D. Yu, T. Fu, and J. Qiu. 2019. Properties of natural fiber-reinforced biobased thermoset biocomposites: Effects Of fiber type and resin composition. Composites Part B: Engineering 171:87–95. doi:10.1016/j.compositesb.2019.04.048.
- Macedo, M. N. Q., J. J. M. Galo, L. A. L. de Almeida, and A. C. de C Lima. 2015. Demand side management using artificial neural networks in a smart grid environment. Renewable and Sustainable Energy Reviews 41:128–33. doi:10.1016/j.rser.2014.08.035.
- Mokhothu, T. H., and M. J. John. 2015. Review on hygroscopic aging of cellulose fibres and their biocomposites. Carbohydrate Polymers 131:337–54. doi:10.1016/j.carbpol.2015.06.027.
- Monti, A., A. El Mahi, Z. Jendli, and L. Guillaumat. 2017. Experimental and finite elements analysis of the vibration behaviour of a bio-based composite Sandwich Beam. Composites Part B: Engineering 110:466–75. doi:10.1016/j.compositesb.2016.11.045.
- Ozsahin, S., and I. Aydin. 2014. Prediction of the optimum veneer drying temperature for good bonding in plywood manufacturing by means of artificial neural network. Wood Science and Technology 48 (1):59–70. doi:10.1007/s00226-013-0583-2.
- Panthapulakkal, S., and M. Sain. 2007. Studies on the water absorption properties of short hemp—glass fiber hybrid polypropylene composites. Journal of Composite Materials 41 (15):1871–83. doi:10.1177/0021998307069900.
- Rangappa, S. M., S. Siengchin, J. Parameswaranpillai, M. Jawaid, and T. Ozbakkaloglu. 2022. Lignocellulosic fiber reinforced composites: ProgresS, performance, properties, applications, and future perspectives. Polymer Composites 43 (2):645–91. doi:10.1002/pc.26413.
- Safa, M., and S. Samarasinghe. 2011. Determination and modelling of energy consumption in wheat production using neural networks: ‘A case study in Canterbury Province, New Zealand’. Energy 36 (8):5140–47. doi:10.1016/j.energy.2011.06.016.
- Sanjay, M. R., S. Siengchin, J. Parameswaranpillai, M. Jawaid, C. I. Pruncu, and A. Khan. 2019. A comprehensive review of techniques for natural fibers as reinforcement in composites: Preparation, processing and characterization. Carbohydrate Polymers 207:108–21. doi:10.1016/j.carbpol.2018.11.083.
- Scida, D., M. Assarar, C. Poilâne, and R. Ayad. 2013. Influence of hygrothermal ageing on the damage mechanisms of flax-fibre reinforced epoxy composite. Composites Part B: Engineering 48 (May):51–58. doi:10.1016/j.compositesb.2012.12.010.
- Sekar, S., S. Kumar, S. Vigneshwaran, and G. Velmurugan. 2022. Evaluation of mechanical and water absorption behavior of natural fiber-reinforced hybrid biocomposites. Journal of Natural Fibers 19 (5):1772–82. doi:10.1080/15440478.2020.1788487.
- Sekar, S., V. Shanmugam, S. Kumar, V. Ganesan, G. Sas, D. J. Johnson, and M. Shanmugam. 2021. Effects of water absorption on the mechanical properties of hybrid natural fibre/phenol formaldehyde composites. Scientific Reports 11 (1):13385. doi:10.1038/s41598-021-92457-9.
- Tabet, Z., A. Belaadi, M. Boumaaza, and M. Bourchak. 2021. Drilling of a bidirectional Jute fibre and cork-reinforced polymer biosandwich structure : ANN and RSM approaches for modelling and optimization. International Journal of Advanced Manufacturing Technology 117 (11–12):3819–39. doi:https://doi.org/10.1007/s00170-021-07679-y.
- Vigneshwaran, S., R. Sundarakannan, K. M. John, R. Deepak Joel Johnson, K. Arun Prasath, S. Ajith, V. Arumugaprabu, and M. Uthayakumar. 2020. Recent advancement in the natural fiber polymer composites: A comprehensive review. Journal of Cleaner Production 277:124109. doi:https://doi.org/10.1016/j.jclepro.2020.124109.
- Vinod, A., J. Tengsuthiwat, Y. Gowda, R. Vijay, M. R. Sanjay, S. Siengchin, and H. Nath Dhakal. 2022. Jute/Hemp bio-epoxy hybrid bio-composites: Influence of stacking sequence on adhesion of fiber-matrix. International Journal of Adhesion and Adhesives 113:103050. doi:10.1016/j.ijadhadh.2021.103050.
- Wang, X., Y. Li, Y. Yan, E. Wright, N. Gao, and G. Chen. 2020. Prediction on the viscosity and thermal conductivity of Hfc/Hfo Refrigerants with artificial neural network models. International Journal of Refrigeration 119:316–25. doi:10.1016/j.ijrefrig.2020.07.006.
- Watanabe, K., Y. Matsushita, I. Kobayashi, and N. Kuroda. 2013. Artificial neural network modeling for predicting final moisture content of individual Sugi (Cryptomeria Japonica) samples during air-drying. Journal of Wood Science 59 (2):112–18. doi:10.1007/s10086-012-1314-2.
- Yorseng, K., S. M. Rangappa, H. Pulikkalparambil, S. Siengchin, and J. Parameswaranpillai. 2020. Accelerated weathering studies of Kenaf/Sisal fiber fabric reinforced fully biobased hybrid bioepoxy composites for semi-structural applications: Morphology, thermo-mechanical, water absorption behavior and surface hydrophobicity. Construction and Building Materials 235:117464. doi:10.1016/j.conbuildmat.2019.117464.