Abstract
Risk assessment forms the basis for both occupational health decision-making and the development of occupational exposure limits (OELs). Although genetic and epigenetic data have not been widely used in risk assessment and ultimately, standard setting, it is possible to envision such uses. A growing body of literature demonstrates that genetic and epigenetic factors condition biological responses to occupational and environmental hazards or serve as targets of them. This presentation addresses the considerations for using genetic and epigenetic information in risk assessments, provides guidance on using this information within the classic risk assessment paradigm, and describes a framework to organize thinking about such uses. The framework is a 4 × 4 matrix involving the risk assessment functions (hazard identification, dose-response modeling, exposure assessment, and risk characterization) on one axis and inherited and acquired genetic and epigenetic data on the other axis. The cells in the matrix identify how genetic and epigenetic data can be used for each risk assessment function. Generally, genetic and epigenetic data might be used as endpoints in hazard identification, as indicators of exposure, as effect modifiers in exposure assessment and dose-response modeling, as descriptors of mode of action, and to characterize toxicity pathways. Vast amounts of genetic and epigenetic data may be generated by high-throughput technologies. These data can be useful for assessing variability and reducing uncertainty in extrapolations, and they may serve as the foundation upon which identification of biological perturbations would lead to a new paradigm of toxicity pathway-based risk assessments.
INTRODUCTION
A growing body of research and information demonstrates that genetic and epigenetic factors condition biological response to occupational and environmental hazards or serve as targets of them.(Citation1–Citation19) These factors can be biomarkers of susceptibility, exposure, or effect depending on how they are used. Critical in using genetic or epigenetic biomarkers in research and risk assessment is the extent to which they are validated for a specific use.(Citation20,Citation21) Incorporating genetic and epigenetic information into risk assessments can provide a range of benefits leading to the development of more precise occupational exposure limits (OELs). The Environmental Protection Agency (EPA) has described the use of genetic information in environmental risk assessment as an advanced approach in their Next Generation Risk Assessment Report.(Citation22) At the most fundamental level, genetic and epigenetic information may be useful in addressing uncertainty and inter-individual variability, two major issues in risk assessment. Uncertainty can be seen in the four components (hazard identification, dose-response modeling, exposure assessment, and risk characterization) of the common model of the risk assessment process.(Citation23) Generally, “any collection of observations describing response to hazardous agents will include uncertainty and variability from a variety of sources.”(Citation24) Uncertainty has been defined as lack of precise knowledge about the state of nature.(Citation23) “Uncertainty in risk assessment is commonly associated with issues such as the selection of concentration-response models and extrapolating across exposure conditions, species, or units of exposure.”(Citation23) In contrast, the concept of variability usually pertains to a differential response of individual people or animals to hazardous exposures. Differential response to occupational hazards may arise from several sources including variability in exposure, biologic response, and methodology.(Citation24) Genetic and epigenetic factors may be a major cause of variability in response in similarly exposed individuals.(Citation25)
A genetic mutation or epigenetic change can be the result of an occupational or environmental exposure and be passed down to future generations through inheritance; however, there is controversy over the extent to which an epigenetic mechanism is responsible for transgenerational effects.(Citation12,Citation26,Citation27) Genetic and epigenetic characteristics can also serve to modify the effect of an occupational/environmental exposure and disease. Possibly even more powerful will be the use of genetic and epigenetic information to help develop a new paradigm of toxicity-pathway-based risk assessment.(Citation28,Citation29) This is where important biological perturbations in toxicity pathways could be used to describe previous exposures, modes of action, and pathologic endpoints for use in conducting risk assessments on “tens of thousands of chemicals and substances on which toxicity information is incomplete and emerging chemicals and substances that will need risk assessment and possible regulation.”(Citation29) Much of this may be possible because of the use of high-throughput technologies and computational strategies that allow for rapid generation of sequence data and information on the expression and regulation of a vast number of genes.(30–32) To realize these benefits for risk assessment, it may be of value to have a framework for considering genetic and epigenetic information in each step of risk assessment. We previously proposed a model for examining genetic susceptibility to inhaled manganese(Citation33) which we have updated to consider other occupational exposures (). In this article, a framework is proposed to cover the wide range of occupational exposures. This framework is based on previous considerations of genetic information in occupational health by Schulte(Citation34) and on the conceptual work of Bollati and Baccarelli,(Citation12) as shown in .
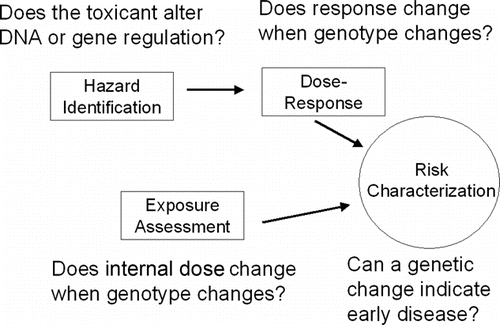
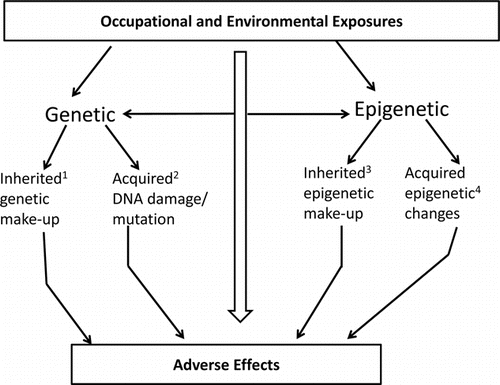
In order to fully appreciate the proposed framework, the nature of genetic and epigenetic information needs to be considered. There are two levels of thinking about genetic and epigenetic information in occupational health risk assessment. The first involves the inclusion during in vitro, animal, and epidemiologic research studies of genetic/epigenetic factors that will be of use in risk assessment, and the second is the use of the data from those studies in quantitative risk assessments. To date, however, despite great potential, there has been little use of genetic or epigenetic information in quantitative risk assessments for occupational or environmental exposures. This is because, thus far, the evidence base for risk assessors to use has been limited, but the number of studies with such information is growing.(1,2,8,9,12–14,31,35–43)
Key points of emphasis covered in this article include the following.
There is a growing but limited amount of genetic and epigenetic data that can be used for hazard identification; dose-response modeling, exposure assessment, and risk characterization.
Additional research is required to identify modes of action and adverse outcome pathways before the risk of genetic and epigenetic variation can be quantified.
Assessing gene-environment interaction is the foundation on which genetic and epigenetic data can be useful in risk assessment.
Animal and in vitro data should complement human data collected in molecular epidemiologic studies when occupational health risk assessments are performed
Studies should be designed to maximize information on both genetic and epigenetic variation and their physiological relevance to adverse outcome pathways.
Ultimately, if genetic and epigenetic data are to be useful in occupational health risk assessments, attention must be paid to the ethical, legal, societal, and political implications.
GENETIC RESEARCH
while there is at least a 150-year history of scientific assessment of genetics, it is only in the last 20 years that the sequence of the human genome has been mapped. Understanding of the inherited genetic component of disease and knowledge about the interaction of genetic and environmental factors have increased, but there are still many questions about the function of a large proportion of genes, the entirety of the sequences, and the involvement of multiple genes in disease processes. Moreover, the reductionist approach of focusing on one or a few genes as instrumental in an exposure-disease relationship may not be supportable for many occupational exposures. This means there will be a need for systems-based approaches to interpret genetic data.(Citation7,Citation11,Citation30,Citation44) For example, the amount of genetic information on Phase I and Phase II metabolism of industrial chemicals has grown considerably in recent years. Understanding which genotypes increase susceptibility or resistance to occupational exposures could allow risk assessors to reduce the typical uncertainty factor for inter-individual variability from 10 to a lower, data-derived number. Pohl and Scinicariello(Citation45) attempted to do this for solvent metabolism by CYP2E1 incorporating multiple reported allelic variants affecting catalytic activity and gene expression; however, they acknowledged this approach is probably limited to single chemicals and would be more challenging for exposure to mixtures.
There is a rich history of using a small number of mutations in research and risk assessments for radiation and chemical carcinogens.(41,46–51) A classic example is benzene toxicity where polymorphisms in NQO1 can increase the risk of adverse health effects whereas mutations in GSTM1, GSTT1, and GSTA1 can alter metabolism and internal dose.(Citation51) The U.S. EPA cancer risk assessment guidelines are based on defining the mode of action.(Citation50) Two key events, mutation and cell proliferation, are critical in defining the mode of action for a particular carcinogen.(Citation41,Citation52) The use of genetic information for “improving current risk assessment practices is based on the premise that the frequency of somatic mutation is of critical importance in understanding and modeling carcinogenesis. Ultimately, genotypic selection will have the greatest impact on risk assessment if measurement of spontaneous mutations is possible.”(Citation36) Importantly, numerous assays have been used routinely to screen for DNA damage, which have proven effective at identifying new carcinogens.(Citation2,Citation9,Citation53)
Gene-Environment Interactions
The complexities of gene-environment interactions are important to recognize if data from these studies and data sets are to be useful in quantitative risk assessment. Gene-environment interactions have been simplistically interpreted so that genes are equated as causal factors inside the body and environments as causal factors outside the body.(54) However, the term “gene-environment” interaction can involve a range of interpretations of joint effects, including the risk of a single genotype across a range of environmental exposures, or the risk of exposure across a range of genotypes. The complexity of these interactions will influence how they may be used or interpreted in risk assessments.(Citation54)
Animal or epidemiological research involving genetic factors will be useful for occupational health quantitative risk assessments. The multitude of genetically modified and highly inbred animal models allow testing of specific gene x environment interactions when epidemiology data suggests strong correlations between allelic differences and increased risk.(Citation55) Ultimately, evidence from epidemiologic studies involving assessment of gene-environment interactions may be the most desirable input for quantitative risk assessments.(Citation56) The phrase “gene-environment” interaction infers that the direction and magnitude of the clinical and exposure-response effect can vary with different genetic polymorphisms.(Citation15,Citation35,Citation44,Citation57)
Multiple types of research designs have been used to detect and assess the magnitude of gene-environment interactions.(56–59) In the past, the relationship between genes and the environment has been hampered by limited knowledge of the human genome, but this has changed with the emergence of such efforts as the Human Genome Project, the Hap Map Project, and the Environmental Genome Project, and the ability to directly assess DNA sequence variability primarily in the form of single nucleotide polymorphisms (SNP) as well as the ability to assess gene expression with microarrays.(6,7,60,61,63) Studies of gene-environment interactions require information on both elements in the relationship.(Citation63)
While animal and in vitro studies will contribute to future risk assessments, molecular epidemiological studies also should be of great importance.(Citation64,65) The study of gene-environ-ment interaction in worker populations using molecular epidemiologic methods is increasing.(9,14,64–66) Thomas(Citation67,Citation68) has reviewed the epidemiological and statistical issues involved in the investigation of gene-environment interactions and provided extensive guidance that is useful as the foundation for conducting such research and considering it in quantitative risk assessments. Clearly, in addition to genetic data, valid and adequate exposure data are critical. (This will be discussed later in the article.)
Sample size, statistical power, and multiple comparisons are other major factors that need to be considered in evaluating gene-environment studies.(Citation19,Citation20,Citation68) Ioannidis et al.(Citation69) found that sample size requirements can be very large in studies of gene associations in complex diseases and even larger if there are synergistic gene-environment interactions. To date, there are four major approaches to the analysis of interactions between genetic and environmental factors. These include interactions with single genes, pathway-driven approaches, and systems biology, and genome-wide association studies.(Citation68)
However, since multiple genes are generally involved in response to an exposure, there is need for a comprehensive model for a complex disease involving multiple genes and multiple environmental risk factors.(Citation68) In some cases, there will also be gene-gene interactions. A widely used tool to analyze high-dimensional interaction has been Multifactor Dimension Reduction (MDR)(Citation70,Citation71) “… which searches across all possible partitions of the cells of the multi-pathway contingency table for the best classifier of disease risk on multiple training sets and tests, [and assesses] their predictions on the remaining data.”(Citation68) The pathway-driven approach is based in establishing hypotheses about causal pathways. This could involve regression modeling tools and Bayesian modeling. In Bayesian modeling, the prior covariates can be derived from various ontology data bases such as the Kyoto Encyclopedia of Genes and Genomes, Gene Ontology, Ingenuity Pathway Analysis and various others.(Citation68)
Another aspect of gene-environment interactions involves expanding the environmental exposure evidence base and integrating population scale and molecular scale data. Patel et al.(Citation72) described a framework for an environment-wide association studies (EWAS) analog to genome-wide association studies (GWAS). EWAS allows for the evaluation of multiple environmental factors to address the complex nature of exposure in relation to disease. Since GWAS and EWAS operate on the population scale, “… there is need to integrate molecular-scale toxicological evidence—such as how an environmental factor might modulate a biological process—between exposure and genes.”(Citation19) The approach that was used involved deriving lists of candidate interacting genetic and environmental factors by integrating findings from GWAS and EWAS then searching for evidence of toxicological relationships between those factors that could have an etiological role in the disease.(Citation19)
Today, with the “introduction of array-based genotyping techniques allowing simultaneous assessment of up to 1 million single nucleotide polymorphisms (SNPs) in a single assay, it has become possible to cover with varying resolutions, the entire genome in what are now commonly referred to as genome-wide association studies (GWAS).”(Citation42) With the advent of GWAS, a different approach has been utilized, based on “agnostic” searches with no prior hypotheses.(Citation67) In the current “post GWAS” era, the focus is on integrating findings from a vast body of data including genomics, proteomics, metabolomics, and transcriptomics. Early SNP-based studies often assessed a few SNPs in a limited set of candidate genes. However, the current practice is to examine vast numbers of genes and gene products. Currently, more than 9 million SNPs have been identified and listed in public data bases.(Citation42,Citation73) This is one basis for establishing a mode of action information base for use in risk assessment.(Citation29,Citation74)
EXPOSURE
In an occupational setting, gene-environment interactions must be carefully defined to include exposures that are unique to the workplace. For the last quarter century, the emphasis has focused more on the genetic than the environmental component.(Citation75) This was spurred on by the promise of the Human Genome efforts, sequencing, mapping, and storing of large numbers of biological specimens. Partially, as a consequence of the emphasis on genotyping, the accurate measurement of many environmental and occupational exposures remains an outstanding and largely unmet challenge in epidemiology. There is a strong need to develop methods with the same precision for an individual's environmental exposure as there is for the individual's genome.(Citation75) Clearly, for most diseases, particularly occupational ones, environment rather than genetics is an equal or more important risk factor. Moreover, the variability in response to chemicals, which can be conditioned by genetics, has long been known to also be linked to exposure variability. “Within-person and between-person sources of variability in exposure levels were recognized as early as 1952 when Oldham and Roach applied ANOVA models to breathing zone samples of dust in British coal miners.”(Citation76) Variability in exposure can be the result of multiplicative effects of several variables (jobs, time, locations, sources of contamination, activities, equipment, worker/source mobility, and environmental conditions). Historically, exposure data in epidemiologic studies was rather sparse. In a review by Armstrong et al., only 13% of epidemiologic studies used quantitative exposure measurements.(Citation77) The amount and quality of exposure data has been increasing and new technology (e.g., nanosensors), new regulations, practices, and new concepts (e.g., biomarkers and the exposome), may promote “putting the E into “G x E” interaction studies.”(Citation78,Citation79) Promising analytical approaches for more precisely measuring environmental/occupational substances are in the developmental stage, including microfluidics, nanotechnologies, and mass and Raman spectrometry.(80–82) Simple inexpensive direct reading exposure measurement (DREM) techniques should allow for a broader and more comprehensive exposure assessment in epidemiological studies and in risk characterization.(Citation78,Citation83)
One of the critical issues in exposure assessment is to consider both the totality of exposure that might be related to an adverse effect as well as the particular contribution of the occupational component.(Citation84,Citation85) An approach known as meet-in-the-middle (MITM) has been devised. It involves a combination of efforts within a prospective population study of a search for intermediate biomarkers which are elevated in subjects who eventually develop a disease and a retrospective search for links of such biomarkers to past exposures.(Citation86,Citation87) The approach uses various omics-based biomarkers to assess exposure and is likely to be a useful tool to enhance exposure assessment.(Citation64) Many studies that assess such biomarkers, may use surrogate as well as target tissues.(Citation20,63,64)
RISK ASSESSMENT INVOLVING INHERITED GENETIC DATA
Genetic data can be classified as inherited or acquired.(Citation82) Inherited genetic information is passed on through the process of meiosis to succeeding generations of organisms. Acquired genetic information is passed on to generations of somatic cells within the same organism. Both types of genetic information can be used in risk assessment, but we will discuss inherited genetic data first. Hereditary changes such as polymorphisms in metabolic enzymes are useful for stratification of main effects.
The use of genetic information in quantitative risk assessment (QRA) has been rare and practically no OELs are based on such information except for internal OELs in the pharmaceutical industry.(Citation38,Citation89) Naumann et al.(Citation38) incorporated genetic data (expression of CYP2D6) to develop an OEL for timololal maleate in the pharmaceutical industry. A polymorphism in CYP2D6 influences metabolism of timololol maleate. Chemical-specific adjustment factors using the CYP2D6 expression data were developed and used to replace the default uncertainty factor for inter-individual variability. Nonetheless, the potential benefit of such use has been identified.(Citation90,Citation91) This includes contributing to the knowledge base by improving the understanding of the mechanism of action, clarifying the extrapolation from animals to humans, and explaining variability in response to exposures. Beyond QRA, genetic information may help to identify groups at high risk of occupational disease given a particular exposure whether it is due to allelic differences in DNA repair enzymes,(Citation92) differential transport of metal ions(Citation93) or a mismatch between a highly active Phase I enzyme and a low-activity Phase II enzyme.(Citation94) “Although it has long been recognized that genetic polymorphism plays an important role in driving variability in xenobiotic metabolism, this awareness typically has not translated into the use of these data in a quantitative sense for risk assessment.”(Citation95) However, there is a growing literature that shows that polymorphisms can influence the risk of toxic effects on animals and people and such influence can be quantitated.(1,9,14,64,95,96) For example, Mörk et al.(97) used Monte Carlo simulations and PBPK modeling to develop chemical specific adjustment factors for toluene, styrene, and methyl chloride that could account for known variability in the human population.(Citation97)
Addressing variability is a critical aspect of risk assessment. Integrating data on polymorphisms in enzymes with physiologically-based pharmacokinetic modeling is one promising approach to addressing variability. Haber et al.(Citation95) evaluated the role of polymorphisms in enzymes modulating the disposition of four diverse compounds: methylene chloride, warfarin, parathion, and dichloroacetic acid. They used the analysis to identify key uncertainties in using polymorphism data and highlighted potential simplifying assumptions that might be needed to test the hypothesis that the genetic factors are a substantive source of human variability in susceptibility to occupational or environmental toxicants. Of highest interest to those trying to incorporate genetic information into risk assessment are the following issues:
how to assess the relative contribution of different enzyme systems;
reconciling differences between in vitro and in vivo data;
the lack of toxicokinetic data for many allelic variants; and
uncertainties regarding the effect of co-exposures which could lead to either induction or inhibition.(95)
Another evaluation of the role of polymorphisms in accounting for inter-individual variability identified further uncertainties that need consideration in risk assessment. A Monte Carlo simulation analysis of various enzymes (cytochrome P-450 CYP2D6, CYP2E1, aldehyde dehydrogenase-2, paraoxonase, GSTM1, GSTT1, GSTP, NAT1, and NAT2) showed large inter-individual variability in enzyme function and the need to consider other factors such as blood flow to the liver and compensating pathways for clearance that affect how a specific polymorphism will alter internal dose and toxicity.(Citation10) Such information on genetic polymorphisms and related data can be used to help refine risk assessments by more accurately defining the Point of Departure, for example, and more accurately identifying the most susceptible individuals.(Citation94)
RISK ASSESSMENT INVOLVING ACQUIRED GENETIC DATA
Somatic genetic changes or induced changes in gene regulation can be interpreted as genotoxic effects or “toxicity pathway perturbations” and ultimately contribute to augmentation of the weight of evidence for the mode of action determination.(Citation74) There is a rich history of research on genotoxic somatic cell changes associated with occupational and environmental agents.(64,98–104) Genotoxic effects have been considered as predictors of disease as well as dependent (outcome) variables in research(Citation21,Citation100) or targets of monitoring in exposed populations.(Citation99) Genotoxic effects could be considered in risk assessments if the link between these endpoints and a disease was validated.(Citation106) Additionally, such genotoxic effects could indicate toxic exposure in research or risk assessments.(105–107) The acquired genomic data can also be used in the weight of evidence evaluation of particular hypotheses that affect decisions made in developing quantitative toxicity values (e.g., human relevance, critical effect, and/or response level selection and low-dose extrapolation approach.(Citation13)
In low-dose extrapolation, toxicogenomic data are sometimes used to buttress a particular decision on mode of action. A more direct approach for using toxicogenomic data in QRA is to use alternative methods such as in vivo, in vitro, or in silico methods to predict in vivo experimental animal toxicity endpoints.(Citation13) Thus, data on acquired transcription or other toxicogenomic changes observed in vitro would be considered as a new endpoint linked to in vivo toxicity. For example, “as an alternative to a NOAEL, gene expression could be used to define a “no observed transcriptional level effect” (NOTLE) as a point of departure (POD) either for deriving a reference value after application of uncertainty or safety factors for benchmark dose modeling of gene expression or pathway activity.(Citation11,13,Citation108)
Increasingly, acquired genetic data are in the form of outputs from high-throughput technologies such as microarrays that can show the level of expression of thousands of genes after exposure to toxicants.(Citation7,Citation109,Citation110) How these data are interpreted is critical. Various approaches have been explored. Gene Ontology (GO) and pathway mapping have been shown to be powerful approaches to assess microarray outputs.(Citation7,Citation111) Gene Ontology is an initiative in the bioinformatics community to develop a controlled vocabulary of gene and gene product attributes across all species. A quantitative dimension, GO-Quant, was developed by Yu et al.(Citation7) in calculating the corresponding ED50 for each specific functional GO term found useful in risk assessment. This approach allows for the identification of a response pathway from toxicant exposure and can be used for assessing the mechanistic response across various species. The advent of “humanized mice” expressing human version of key metabolic enzymes,(Citation55) transporters, or other key pathway proteins will greatly improve the quality of data derived from animal experimentation for application in risk assessment.
EPIGENETIC RESEARCH
Although the concept is of ancient origin, the first use of the term “epigenetics” is attributable to Waddington in 1942 as the study of processes by which the genotype gives rise to the phenotype,(Citation112,Citation113) linking environmental and genetic influences on the same individual.(Citation26) Epigenetics manifests, not as changes in the DNA sequence, but in the instructions and timing of gene products. Regulation of gene expression is a complex process that can have dramatic effects on the development and characteristics of an individual. Epigenetic changes are durable and heritable. Each epigenetic change or modification is referred to as a “mark” or “tag” and the total complement of epigenetic marks in an individual is referred to as the epigenome.(Citation26) Epigenetics is the “modification of DNA or associated proteins other than DNA sequence variation that carry information content during cell division and these modifications are mitotically or meiotically heritable chemical/structural changes that regulate gene activity in the absence of underlying changes to DNA sequence.”(Citation14,Citation115) Hence, they can be modifiers of gene-environment interactions.
With respect to environmental chemicals, several epigenetic mechanisms including DNA methylation, histone modifications, and microRNA (miRNA expression) can change gene expression and physiological function.(Citation12) Various classes of chemicals can cause epigenetic modifications. These include metals (e.g. arsenic, cadmium, chromium, methylmercury, nickel), peroxisome proliferators (trichloroethylene, dichloroacetic acid, trichloroacetic acid), air pollutants (particulate matter, black carbon, benzene), and endocrine-disrupting/reproductive toxicants (diethylstilbestrol, Bisphenol A, persistent organic pollutants, dioxin).(Citation12,Citation116) Epigenetic modifications are not necessarily adverse, so caution must be taken when incorporating epigenetic data into risk assessment to insure the change represents an actual hazard ().
Epigenetics can contribute to understanding the relationship between an individual's genetic background, the environment, age, and disease.(Citation114,Citation117) It may be that similar questions about disease risk and modification effects of occupational exposure using genetic variability can now be asked using epigenetic variability.(Citation118) Since some epigenetic effects may be hereditary and some acquired through life, it will be necessary to sort out the contribution from these sources and determine whether they are independent or interactive risk factors. Additionally, it will be necessary to determine whether an epigenetic effect is on the causal pathway to a disease, modifies a pathway or is only associated with a causal pathway.(115.119) There is some disagreement in the literature whether the Mendelian randomization approach can be extended to sorting out causal relationships between epigenetic patterns, phenotypes, and exposures.(Citation115,Citation119) Additionally, there is no consensus on how to model or measure methylation status(Citation120) (one of the most common types of epigenetic effects) or other epigenetic data.(Citation115) Foley et al.(Citation115) identified the following analytical issues.
The appropriate statistical model will depend on the scientific question of interest and knowledge about biological pathways.
Since methylated CpG sites often exist in clusters and may show correlated methylation changes, analytical methods for summarizing correlated data may be required.
Since most epigenetic data summarizes methylation at individual CpG sites as proportions, specific models for proportional data will be required.
Disentangling genetic and epigenetic effects could be a challenge.
It would also be important to factor in the location of the CpG islands, since methylation would have differing effects depending on the site (e.g., promoter sites vs. exons).(115,121)
Epigenetic research, that will be useful in QRA and ultimately as a basis for OELs, still appears to be a long way off. Technical issues need to be resolved.(Citation121) These include such issues as how to make sense out of epigenetic data since the level and pattern of epigenetic marks vary across different tissues and cells and their presence in easily accessible tissues may not reflect what would occur in harder to reach tissues of interest.(Citation115) Including epigenetics in epidemiologic studies of occupational disease may help explain the relationship between the genome and the work environment; however, other environmental exposures outside of work also will need to be addressed.(Citation27,Citation114)
One approach to advancing epidemiologic use of epigenetic data is to determine whether epigenetic marks are associated with complex diseases such as cancer and cardiovascular disease (CVD).(Citation115,Citation122) An important logistical and technical step in this approach is to determine if DNA from existing biological specimen banks (biobanks) could be used to provide specimens for epigenetic association studies. Considering this question, Talens et al.(Citation123) concluded that, provided they are carefully designed, epigenetic studies of complex diseases may be feasible using genomic data derived from banked specimens. Perhaps more important, the need for a larger database should prompt closer attention to study design, especially when it's possible to collect blood for future DNA and microRNA analysis. Studies in steelworkers(Citation124) and nickel refinery workers(Citation125) demonstrated the efficacy of using leukocytes to identify histone modifications associated with inhalation exposures while simultaneously collecting exposure data. This could be a model for similar future studies.
RISK ASSESSMENT USING EPIGENETIC DATA
To date, there appear to be no QRA using epigenetic data that could be the basis of OELs. In fact, there are many complex issues with epigenetic data that require investigation before there will be useful epigenetic studies that can be included in QRAs. Nonetheless, there is a growing body of information that illustrates how environmental exposures can be associated with altered epigenetic profiles.(Citation40,Citation115,Citation126) Much of this information pertains to impacts on offspring and not on environmental effects in exposed workers. Ray et al. recently summarized numerous epigenetic changes associated with occupational exposure to arsenic and four toxic metals that have strong potential to inform future risk assessments.(Citation126) Ultimately, as more data are gathered, epigenetic studies may help explain variable distribution of adverse effects in worker population groups. To achieve this utility, there is need for agreement on appropriate paradigms, approaches and methods for using epigenetic data. An interactive approach will be essential with risk assessors clearly communicating critical needs to researchers and, in turn, incorporating that new data into updated risk assessments.(Citation127) If proof-of-principle causal linkages can be established between epigenetic changes and apical endpoints, generation of full dose-response data useful in risk assessments will follow.(Citation128,Citation129)
There may be many benefits of integrating epigenetic data into the risk assessment process. Epigenetic data has the potential to inform both mechanism and modes of action and in combination with genome data may identify novel modes of action. “Epigenetic data may also be used to identify toxicodynamic (TD) and toxicokinetic (TK) data, inter-and-intraspecies differences in TD and TK, exposure assessments, and dose-response assessments.”(Citation126)
INCORPORATING GENETIC, EPIGENETIC, AND OCCUPATIONAL DATA IN THE SAME ANALYSES
Ultimately, genetic, epigenetic, and environmental information may be included in the same analysis.(Citation130) This may help identify key regulatory pathways and allow efficient screening of large numbers of occupational (and environmental) factors to guide further research, risk assessment, and occupational health decisions.(Citation11) For example, Gohlke et al.(Citation11) used network theory(Citation131) to explore how genetic and environmental factors interact in complex diseases like metabolic syndrome and neuropsychiatric disorders. These researchers integrated gene-centered knowledge from epidemiological and mechanistic environmental research to identify pathways that define disease phenotypes. Basu et al. explored the feasibility of combining SNP data with epigenetic changes at specific loci to improve the risk assessment of mercury, noting that global DNA methylation studies were more variable and less useful.(Citation132) These approaches make possible the development of new hypotheses in studying the impact of genetic and environmental factors on disease. Ultimately, epigenetic factors could be included, and this approach could identify how different levels of environmental exposures to a target chemical can modulate the underlying disease pathways. This type of data could be the basis for an occupational health risk assessment, so we have built upon our previous work with inhaled manganese(Citation33) to provide a guide for risk assessors (). The guide provides key questions that should be addressed when using genetic and epigenetic data in risk assessment and the development of OELs.
Table 1 Guide to Assessing Genetic and Epigenetic Data for Risk Assessment
FRAMEWORK FOR USE OF GENETIC AND EPIGENETIC DATA IN OCCUPATIONAL RISK ASSESSMENT
The utility of genetic and epigenetic data in occupational risk assessment can be broadly seen in the framework shown in . The framework represents a 4 × 4 matrix with the rows showing the risk assessment functions (hazard identification, dose-response modeling, exposure assessment, and risk characterization). In the columns, genetic and epigenetic data, each subdivided by “inherited” or “acquired,” are listed. The distinction between inherited and acquired for genetic and epigenetic is described in . The concept of inherited epigenetic effects is meant to be used figuratively since the epigenome undergoes constant reconfiguration during zygote development and maturation of the individual and it is difficult to identify a single epigenomic configuration and define it as “inherited.”
Table 2 Framework for use of genetic and epigenetic data in occupational and environmental risk assessment
In hazard identification, genetic and epigenetic changes that are associated with adverse effects can serve as indicators of hazard, as well as shed light on mode of action. Additionally, genetic and epigenetic analyses can help in the screening of large numbers of chemicals to develop risk categories or priorities for in-depth toxicological testing. Genetic and epigenetic changes can be indicators of exposure in exposure assessment, either alone or in combination with environmental measurements or job scenario classifications. Genetic and epigenetic data also can serve as effect modifiers of exposure-disease relationships, and epigenetic data can serve as effect modifiers of exposure-gene relationships as well. Genetic and epigenetic data can be used in deriving uncertainty factors useful in setting occupational exposure limits.(Citation89) Finally, the prevalence and distribution of genetic or epigenetic factors in populations can be used to characterize risks in exposed populations.
QRA AND OCCUPATIONAL EXPOSURE LIMITS
QRA is the foundation on which occupational exposure limits are developed in the United States.(Citation91,Citation133) However, there have been no OELs that have been based on genetic or epigenetic data thus far. When to use genetic or epigenetic data is a question that has been considered by EPA for environmental risk assessments.(Citation25,Citation134,Citation135) Kramer et al.(Citation90) developed the following criteria useful for considering genetic (and possibly epigenetic) information in risk assessment and development of OELs.
The gene product must be relevant to the pathophysiology of a clearly defined and consistent phenotype.
Gene function must be associated with exposure to a regulated-pollutant or, at the very least, to a disease-progression process known to be associated with exposure to the chosen regulated pollutant.
The mutation must be functionally relevant.
The magnitude or frequency of occurrence in the population must be measured, and variation across populations (e.g., geography, race) must be considered.
There must be a high magnitude of association (i.e., preferably a relative risk >1.5) between the phenotype of interest and an adverse health effect.
Although no OELs have been developed making direct use of genetic data, one example where the potential impact on OEL setting can be demonstrated is methylene chloride (dichloromethane). The available information meets the five criteria above. The metabolism of methylene chloride in mice and humans has been worked out and there is general acceptance regarding the metabolic pathway leading to carcinogenesis. A key component, distributional information on genetic polymorphisms of the key enzyme involved in carcinogenesis, glutathione S-transferase T1 (GSTT1), is also available. Jonsson and Johanson(Citation4) used a Bayesian approach and built on earlier work of El-Masri et al.(Citation136) to consider how the risks of methylene chloride exposure would differ when polymorphisms in GSTT1 were estimated across the population. From their work and follow-up work by David et al.(Citation137), it is possible to see the impact genetic information could have on OEL setting. The issue of who is protected by the OEL becomes critically important—whether it is the “average” worker (which would include invidivuals lacking GSTT1 and presumably at zero risk) or the most sensitive subpopulation (+/+) GSTT1). Their work demonstrates how the use of this information and these techniques can help to reduce the uncertainties in the QRA and support OEL-setting.
Ultimately, before genetic and epigenetic data are used for occupational health risk assessment and OEL development, published studies must demonstrate that these changes influence the relationship between an occupational exposure and adverse effect. Such studies will need to be of the size and statistical power to be robust, and the data outputs of the studies need to be useful for statistical modeling. Guidance such as STrengthening the Reporting of OBservational studies in Epidemiology (STROBE), and the molecular epidemiologic variant of it, STROBE-ME, as well as Strength and Reporting of Genetic Associations (STREGA) and other guidelines will help assure that genetic and epigenetic studies are useful for occupational health risk assessment.(138–141) Increasingly, Computation Biology and the use of Adverse Outcome Pathways allow the extraction of more signal from noise by focusing on physiologically relevant changes that are consistent across similar groups of toxicants.(Citation142,Citation143)
CONCLUSION
Understanding the role that genetic and epigenetic factors play in occupational disease can improve risk assessments and ultimately lead to better worker protection through the development of more targeted occupational exposure limits. These improved risk assessments are still on the horizon, but they can be envisioned. In this article, the functions of inherited and contemporary genetic and epigenetic information are identified for each element in the risk assessment process. There is still much work that needs to be accomplished to validate genetic and epigenetic markers for the various functions. Ultimately, risk assessments based on robust descriptions of mode of action and evidence-based extrapolations across species, from in vitro to in vivo, may provide a mechanistic basis for describing the susceptibility of certain subpopulations.(Citation74)
In addition to the need for further scientific and technical methods development, there are also ethical, legal, social, and political considerations.(3,14,91,144-148) While these issues are beyond the scope of this article, their importance cannot be underestimated. It is not far-fetched that a worker's “Right to Know” might someday extend to the worker's right to know their genetic susceptibility to workplace toxicants. Therefore, attention to these issues is imperative in order to realize the potential of genetic and epigenetic technologies to enhance risk assessments and protect workers.
ACKNOWLEDGMENTS
The authors thank the following for comments on earlier drafts: Gayle DeBord, Patricia Sullivan, Paolo Vineis, Muin Khoury, and Ila Cote.
FUNDING
Christine Perdan Curran's participation was partially supported by NIH grants ES020053 and GM103436.
DISCLAIMER
The findings and conclusions in this report are those of the author(s) and do not necessarily represent the views of the National Institute for Occupational Safety and Health. Mention of any company or product does not constitute endorsement by the National Institute for Occupational Safety and Health.
REFERENCES
- Bois, F.Y., G. Krowech, and L. Zeise: Modeling human interindividual variability in metabolism and risk: the example of 4-aminobiphenyl. Risk Anal. 15(2):205–213 (1995).
- Ingelman-Sundberg, M.: Genetic variability in susceptibility and response to toxicants. Toxicol Lett. 120(1–3):259–268 (2001).
- Henry, C.J., R. Phillips, F. Carpanini, et al.: Use of genomics in toxicology and epidemiology: findings and recommendations of a workshop. Environ. Health Perspect. 110(10):1047–1050 (2002).
- Jonsson, F., and G. Johanson: A Bayesian analysis of the influence of GSTT1 polymorphism on the cancer risk estimate for dichloromethane. Toxicol. Appl. Pharmacol. 174(2):99–112 (2001).
- Nebert, D.W.: Inter-individual susceptibility to environmental toxicants–a current assessment. Toxicol. Appl. Pharmacol. 207(2 Suppl):34–42 (2005).
- Faustman E.M., and Omenn, G.: Risk Assessment and the Impact of Ecogenetics. In Gene-environment Interactions: Fundamentals of Ecogenetics, L.G. Costa and D.l. Eaton (eds.). Hoboken, NJ: John Wiley & Sons, 2006. pp. 427–450.
- Yu, X., W.C. Griffith, K. Hanspers et al.: A system-based approach to interpret dose- and time-dependent microarray data: Quantitative integration of gene ontology analysis for risk assessment. Toxicol. Sci. 92(2):560–577 (2006).
- Kuppsamy, S.P., J.P. Kaiser, and S.C. Wesselkamper: Epigenetic regulation in environmental chemical carcinogenesis and its applicability in human health risk assessment. Int. J. Toxicol. 34(5):384–392 (2015).
- Christiani, D.C., A.J. Mehta, and C.L. Yu: Genetic susceptibility to occupational exposures. Occup. Environ. Med. 65(6):430–436 (2008).
- Ginsberg, G., S. Smolenski, P. Neafsey et al.: The influence of genetic polymorphisms on population variability in six xenobiotic-metabolizing enzymes. J. Toxicol. Environ. Health Part B Crit. Rev. 12(5–6):307–333 (2009).
- Gohlke, J.M., R. Thomas, Y. Zhang et al.: Genetic and environmental pathways to complex diseases. BMC Syst. Biol. 3(2009).
- Bollati, V., and A. Baccarelli: Environmental epigenetics. Heredity 105(1):105–112 (2010).
- Chiu, W.A., S.Y. Euling, C.S. Scott, and R.P. Subramaniam: Approaches to advancing quantitative human health risk assessment of environmental chemicals in the post-genomic era. Toxicol. Appl. Pharmacol. (2010).
- U.S. Department of Health and Human Services: Genetics in the Workplace: Implications for Occupational Safety and Health. Cincinnati, OH: Centers for Disease Control and Prevention, National Institute for Occupational Safety and Health, 2009.
- Bernstein, D.I., G.E. Kissling, G.K. Hershey et al.: Hexamethylene diisocyanate asthma is associated with genetic polymorphisms of CD14, IL-13, and IL-4 receptor alpha. J. Allergy Clin. Immunol. 128(2):418–420 (2011).
- Ginsberg, G., K. Angle, K. Guyton, and B. Sonawane: Polymorphism in the DNA repair enzyme XRCC1: Utility of current database and implications for human health risk assessment. Mutat. Res. Rev. Mutat. Res. 727(1–2):1–15 (2011).
- Mansi, A., R. Bruni, P. Capone et al.: Low occupational exposure to benzene in a petrochemical plant: Modulating effect of genetic polymorphisms and smoking habit on the urinary t,t-MA/SPMA ratio. Toxicol. Lett. 213(1):57–62 (2011).
- McHale, C.M., L. Zhang, and M.T. Smith: Current understanding of the mechanism of benzene-induced leukemia in humans: implications for risk assessment. Carcinogenesis 33(2):240–252 (2012).
- Patel, C.J., R. Chen, and A.J. Butte: Data-driven integration of epidemiological and toxicological data to select candidate interacting genes and environmental factors in association with disease. Bioinformatics 28(12):I121–I126 (2012).
- Owen, R., T.S. Galloway, J.A. Hagger, M.B. Jones, and M.H. Depledge: Biomarkers and environmental risk assessment: Guiding principles from the human health field. Mar. Pollut. Bull. 56(4):613–619 (2008).
- Schulte, P.A., and J.E. Hauser: The use of biomarkers in occupational health research, practice, and policy. Toxicol. Lett. 213(1):91–99 (2012).
- U.S. EPA: Next Generation Risk Assessment: Incorporation of Recent Advances in Molecular, Computational, and Systems Biology (Final Report). Washington, D.C.: U.S. Environmental Protection Agency, EPA/600/R-14/004, 2014.
- Bailar, J.C., and A.J. Bailer: Risk assessment - the mother of all uncertainties - Disciplinary perspectives on uncertainty in risk assessment. In Uncertainty in the Risk Assessment of Environmental and Occupational Hazards, A.J. Bailer, C. Maltoni, J.C. Bailar (eds.), 1999. pp. 273–285.
- Neumann, D.A., and C.A. Kimmel: Human Variability in Response to Chemical Exposures, Measures, Modeling, and Risk Assessment. Boca Raton, FL:CRC Press, 1998.
- Schwartz, J., D. Bellinger, and T. Glass: Expanding the scope of risk assessment: methods of studying differential vulnerability and susceptibility. Am. J. Public Health 101:S102–S109 (2011).
- Rothstein, M.A., Y. Cai, and G.E. Marchant: The ghost in our genes: legal and ethical implications of epigenetics. Health Matrix Clevel. 19(1):1–62 (2009).
- Cortessis, V.K., D.C. Thomas, A.J. Levine et al.: Environmental epigenetics: prospects for studying epigenetic mediation of exposure-response relationships. Hum. Genet. 131(10):1565–1589 (2012).
- National Research Council (NRC): Toxicity Testing in the 21st Century: A Vision and a Strategy. Washington, D.C.: National Academies Press, 2007.
- National Research Council (NRC): Toxicity-Pathway-based Risk Assessment: Preparing for a Paradigm Change. Washington, D.C.: National Academies Press, 2010.
- U.S. Environmental Protection Agency: Advancing the Next Generation (NextGen) of Risk Assessment: Public Dialogue Conference, 2011.
- Zeise, L., F.Y. Bois, W.A. Chiu, D. Hattis, I. Rusyn, and K.Z. Guyton: Addressing human variability in next-generation human health risk assessments of environmental chemicals. Environ. Health Perspect. 121:23–31 (2013).
- Bush, W.S., J. Boston, S.A. Pendergrass et al.: Enabling high-throughput genotype-phenotype associations in the epidemiologic architecture for genes linked to environment (eagle) project as part of the population architecture using genomics and epidemiology (page) study. Pacific Symposium on Biocomputing. Pacific Symposium on Biocomputing, 373–384 (2013).
- Curran, C.P., R.M. Park, S.M. Ho, and E.N. Haynes: Incorporating genetics and genomics in risk assessment for inhaled manganese: From data to policy. Neurotoxicology 30(5): 754–760 (2009).
- Schulte, P.A.: Framework for considering genetics in the workplace. Med. Lav. 97(2): 339–347 (2006).
- Knudsen, L.E., S.H. Loft, and H. Autrup: Risk assessment: the importance of genetic polymorphisms in man. Mutat. Res. Fund. Molec. Mechan. Mutagen. 482(1–2):83–88 (2001).
- McKinzie, P.B., R.R. Delongchamp, R.H. Heflich, and B.L. Parsons: Prospects for applying genotypic selection of somatic oncomutation to chemical risk assessment. Mutat. Res. Rev. Mutat. Res. 489(1):47–78 (2001).
- McCanlies, E., D.P. Landsittel, B. Yucesoy, V. Vallyathan, M.L. Luster, and D.S. Sharp: Significance of genetic information in risk assessment and individual classification using silicosis as a case model. Ann. Occup. Hyg. 46(4):375–381 (2002).
- Naumann, B.D., D.G. Dolan, and E.V. Sargent: Rationale for the chemical-specific adjustment factors used to derive an occupational exposure limit for timolol maleate. Hum. Ecol. Risk Assess. 10(1): 99–111 (2004).
- Demchuk, E., B. Yucesoy, V.J. Johnson et al.: A statistical model for assessing genetic susceptibility as a risk factor in multifactorial diseases: Lessons from occupational asthma. Environ. Health Perspect. 115(2):231–234 (2007).
- Herceg, Z.: Epigenetics and cancer: towards an evaluation of the impact of environmental and dietary factors. Mutagen 22(2):91–103 (2007).
- Moore, M.M., R.H. Heflich, L.T. Haber, B.C. Allen, A.M. Shipp, and R.L. Kodell: Analysis of in vivo mutation data can inform cancer risk assessment. Regul. Toxicol. Pharmacol. 51(2):151–161 (2008).
- Vlaanderen, J., L.E. Moore, M.T. Smith et al.: Application of omics technologies in occupational and environmental health research: current status projections. Occup. Environ. Med. 67(2):136–143 (2010).
- Bhattacharya, S., Q. Zhang, P.L. Carmichael, K. Boekelheide, and M.E. Andersen: Toxicity testing in the 21(st) century: defining new risk assessment approaches based on perturbation of intracellular toxicity pathways. PLoS One 6(6):(2011).
- Vineis, P., and D. Kriebel: Causal models in epidemiology: past inheritance and genetic future. Environ. Health 5:21 (2006).
- Pohl, H.R., and F. Scinicariello: The impact of CYP2E1 genetic variability on risk assessment of VOC mixtures. Regul. Toxicol. Pharmacol. 59(3):364–374 (2011).
- National Academy of Science (NAS): Identifying and estimating the genetic impact of chemical mutagens. In Committee on Environmental Mutagens. Washington, D.C.: National Academy Press, 1962.
- National Research Council (NRC): The effects on population of exposure to low levels of ionizing radiation (BEIR 1). In Advisory Committee on the Biological Effects of Ionizing Radiation. Washington, D.C.: National Academy of Sciences, 1972.
- Ehling, U.H., and A. Neuhauser: Procarbazine-induced specific-locus mutations in male mice. Mutat. Res. 59(2):245–256 (1979).
- National Research Council (NRC): Considerations of health benefit-cost analysis for activities involving ionizing radiation exposure and alternatives (BEIR II). In Advisory Committee on Biological Effects of Ionizing Radiations. Washingtion, D.C.: National Academy of Sciences, 1977.
- U.S. EPA: Guidelines for carcinogen risk assessment. EPA/630/P-03/001B, March 2005 (2005).
- Palma, G.D., and M. Manno: Metabolic polymorphisms and biomarkers of effect in the biomonitoring of occupational exposure to low-levels of benzene: State of the art. Toxicol. Lett. 231(2):194–204 (2014).
- Meek, M.E., J.R. Bucher, S.M. Cohen et al.: A framework for human relevance analysis of information on carcinogenic modes of action. Crit. Rev. Toxicol. 33(6):591–653 (2003).
- Toraason, M., D.W. Lynch, D.G. DeBord et al.: DNA damage in leukocytes of workers occupationally exposed to 1-bromopropane. Mutat. Res. Genet. Toxicol. Environ. Mutagen. 603(1): 1–14 (2006).
- Grigorenko, E.L.: The inherent complexities of gene-environment interactions. J. Gerontol. Ser. B Psychol. Sci. Soc. Sci. 60:53–64 (2005).
- Derkenne, S., C.P. Curran, H.G. Shertzer, T.P. Dalton, N. Dragin, and D.W. Nebert: Theophylline pharmacokinetics: comparison of Cyp1a1(-/-) and Cyp1a2(-/-) knockout mice, humanized hCYP1A1_1A2 knock-in mice lacking either the mouse Cyp1a1 or Cyp1a2 gene, and Cyp1(+/+) wild-type mice. Pharmacogene Genom. 15(7):503–511 (2005).
- Barry, K.H., Y. Zhang, Q. Lan et al.: Genetic variation in metabolic genes, occupational solvent exposure, and risk of non-hodgkin lymphoma. Am. J. Epidemiol. 173(4):404–413 (2011).
- Ramos, G., and K. Olden: Gene-environment interactions in the development of complex disease phenotypes. Int. J. Environ. Res. Public Health 5(1):4–11 (2008).
- Patel, C.J., R. Chen, K. Kodama, J.P.A. Ioannidis, and A.J. Butte: Systematic identification of interaction effects between genome- and environment-wide associations in type 2 diabetes mellitus. Hum. Genet. 132:495–508 (2013).
- Kraft, P., Y.C. Yen, D.O. Stram, J. Morrison, and W.J. Gauderman: Exploiting gene-environment interaction to detect genetic associations. Hum. Hered. 63(2):111–119 (2007).
- Guengerich, F.P.: The Environmental Genome Project: Functional analysis of polymorphisms—Commentary. Environ. Health Perspect. 106(7):365–368 (1998).
- Collins, F.S., E.D. Green, A.E. Guttmacher, M.S. Guyer, and US National Human Genome Research Institute: A vision for the future of genomics research. Nature 422(6934):835–847 (2003).
- International HapMap Project: “International HapMap Project Homepage.” [Online] Available at http://hapmap.ncbi.nlm.nih.gov/. 2005).
- Hunter, D.J.: Gene-environment interactions in human diseases. Nat. Rev. Genet. 6(4):287–298 (2005).
- Rothman, N., P. Hainaut, P. Schulte, M. Smith, P. Boffetta, and P.M. Perea: Molecular Epidemiology Principles and Practices. Lyon: International Agency for Research on Cancer, 2012.
- Schulte, P.A., and F.P. Perea: Molecular Epidemiology: Principles and Practices. San Diego, CA: Academic Press Inc., 1993.
- Garte, S.: Individual susceptibility and gene environment interaction. In Molecular Epidemiology of Chronic Diseases, C.P. Wild, P. Vineis, and S. Garte (eds.). West Sussex, UK: John Wiley and Sons, Ltd., 2008.
- Thomas, D.: Gene-environment-wide association studies: emerging approaches. Nat. Rev. Genet. 11(4):259–272 (2010).
- Thomas, D: Methods for investigating gene-environment interactions in candidate pathway and genome-wide association studies. In Annual Review of Public Health, J.E. Fielding, R.C. Brownson, and L.W. Green (eds.). Palo Alto: Annual Reviews, 2010. pp. 21–36.
- Ioannidis, J.P.A., T.A. Trikalinos, and M.J. Khoury: Implications of small effect sizes of individual genetic variants on the design and interpretation of genetic association studies of complex diseases. Am. J. Epidemiol. 164(7):609–614 (2006).
- Cordell, H.J.: Detecting gene-gene interactions that underlie human diseases. Nat. Rev. Genet. 10(6):392–404 (2009).
- Edwards, T.L., K. Lewis, D.R. Velez, S. Dudek, and M.D. Ritchie: Exploring the performance of Multifactor Dimensionality Reduction in large scale SNP studies and in the presence of genetic heterogeneity among epistatic disease models. Hum. Hered. 67(3):183–192 (2009).
- Patel, C.J., J. Bhattacharya, and A.J. Butte: An environment-wide association study (EWAS) on Type 2 diabetes mellitus. PLoS One 5(5):(2010).
- Sachidanandam, R., D. Weissman, S.C. Schmidt et al.: A map of human genome sequence variation containing 1.42 million single nucleotide polymorphisms. Nature 409(6822): 928–933 (2001).
- Edwards, S.W., and R.J. Preston: Systems biology and mode of action based risk assessment. Toxicol. Sci. 106(2):312–318 (2008).
- Wild, C.P.: Complementing the genome with an “exposome”: The outstanding challenge of environmental exposure measurement in molecular epidemiology. Cancer Epidemiol. Biomark. Prev. 14(8):1847–1850 (2005).
- Rappaport, S., and L.L. Kupper: Quantitative Exposure Assessment. El Cerrito, CA: Lulu Press, 2008.
- Armstrong, B.K., E. White, and R. Saracci: Principles of Exposure Measurement in Epidemiology. New York: Oxford University Press 1992.
- Smith, M.T., and S.M. Rappaport: Building Exposure Biology Centers to Put the E into “G x E” Interaction Studies. Environ. Health Perspect. 117(8):A334–A335 (2009).
- Rappaport, S.M.: Implications of the exposome for exposure science. J. Exposure Sci. Environ. Epidemiol. 21(1):5–9 (2011).
- Rubino, F.M., M. Pitton, D. Di Fabio, and A. Colombi: Toward an “omic” physiopathology of reactive chemicals: thirty years of mass spectrometric study of the protein adducts with endogenous and xenobiotic compounds. Mass Spect. Rev. 28(5):725–784 (2009).
- Stockton, A.M., T.N. Chiesl, J.R. Scherer, and R.A. Mathies: Polycyclic aromatic hydrocarbon analysis with the Mars organic analyzer microchip capillary electrophoresis system. Anal. Chem. 81(2):790–796 (2009).
- Soltow, Q.A.: High-performance metabolic profiling with dual chromatography-Fourier-transform mass spectrometry (DC-FTMS) for study of the exposome. Metabolomics 9(0):132–143 (2013).
- Weis, B.K., D. Balshawl, J.R. Barr et al.: Personalized exposure assessment: Promising approaches for human environmental health research. Environ. Health Perspect. 113(7):840–848 (2005).
- Brunekreef, B.: Exposure science, the exposome, and public health. Environ. Molec. Mutagen. 00: n/a-n/a (2013).
- Lioy, P.J., and Smith, K.R.: A discussion of exposure science in the 21st century: a vision and strategy. Environ. Health Perspect. 121:405–409 (2013).
- Chadeau-Hyam, M., T.J. Athersuch, H.C. Keun et al.: Meeting-in-the-middle using metabolic profiling—a strategy for the identification of intermediate biomarkers in cohort studies. Biomarkers 16(1):83–88 (2011).
- Vineis, P., K. van Veldhoonk, M. Chadeau-Hyam, and T. Athersuch: Advancing application of omics-based biomarkers in environmental epidemiology. Environ. Molec. Mutagen. 54(7):461–467 (2013).
- Schulte, P.A., and G. DeBord: Public health assessment of genetic information in the occupational setting. In Genetics and Public Health in the 21st Century, M.J. Khoury, W. Burke, and T. EJ (eds.), New York: Oxford University Press, 2000. pp. 203–219.
- Dankovic, D.A., B.D. Naumann, M.L. Dourson, A.M. Maier, and L. Levy: The scientific basis of uncertainty factors used in setting occupational exposure limits. J. Occup. Environ. Hyg. 12(0):S55–S68 (2015).
- Kramer, C.B., A.C. Cullen, and E.M. Faustman: Policy implications of genetic information on regulation under the Clean Air Act: The case of particulate matter and asthmatics. Environ. Health Perspect. 114(3):313–319 (2006).
- Schulte, P., and J. Howard: Genetic Susceptibility and the Setting of Occupational Health Standards. In Annual Review of Public Health, Vol 32, J.E. Fielding, R.C. Brownson, and L.W. Green (eds.), Palo Alto, Annual Reviews: 2011. pp. 149–159.
- O’Driscoll, M.: Diseases Associated with Defective Responses to DNA Damage. Cold Spring Harbor Perspect. Biol. 4(12), (2012).
- Jenkitkasemwong, S., C.Y. Wang, B. Mackenzie, and M.D. Knutson: Physiologic implications of metal-ion transport by ZIP14 and ZIP8. Biometals 25(4):643–655 (2012).
- Nebert, D.W.: Extreme discordant phenotype methodology: an intuitive approach to clinical pharmacogenetics. Eur. J. Pharmacol. 410(2–3):107–120 (2000).
- Haber, L.T., A. Maier, P.R. Gentry, H.J. Clewell, and M.L. Dourson: Genetic Polymorphisms in assessing interindividual variability in delivered dose. Regul. Toxicol. Pharmacol. 35(2):177–197 (2002).
- Shen, M., L. Zhang, K.-M. Lee et al.: Polymorphisms in genes involved in innate immunity and susceptibility to benzene-induced hematotoxicity. Exper. Molec. Med. 43(6): 374–378 (2011).
- Mörk, A.K., F. Jonsson, and G. Johanson: Adjustment factors for toluene, styrene and methyl chloride by population modeling of toxicokinetic variability. Regul. Toxicol. Pharmacol. 69(1):78–90 (2014).
- Berg, K: Inherited Variation in Susceptibility and Resistance to Environmental Agents. San Diego, CA: Academic Press, 1979.
- Albertini, R.J.: Developing sustainble studies on environmental health. Mutat. Res. 480(317–331):(2000).
- Jones, I.M., H. Galick, P. Kato et al.: Three somatic genetic biomarkers and covariates in radiation-exposed Russian cleanup workers of the Chernobyl nuclear reactor 6-13 years after exposure. Rad. Res. 158(4):424–442 (2002).
- Bonassi, S., A. Znaor, H. Norppa, and L. Hagmar: Chromosomal aberrations and risk of cancer in humans: an epidemiologic perspective. Cytogen. Genome Res. 104(1–4):376–382 (2004).
- Hagmar, L., U. Stromberg, S. Bonassi et al.: Impact of types of lymphocyte chromosomal aberrations on human cancer risk: Results from Nordic and Italian cohorts. Cancer Res. 64(6):2258–2263 (2004).
- Lan, Q., R. Cawthon, M. Shen et al.: A prospective study of telomere length measured by monochrome multiplex quantitative PCR and Risk of non-Hodgkin lymphoma. Clin. Cancer Res. 15(23): 7429–7433 (2009).
- Cassini, C., C. Calloni, G. Bortolini et al.: Occupational risk assessment of oxidative stress and genotoxicity in workers exposed to paints during a working week. Int. J. Occup. Med. Environ. Health 24(3):308–319 (2011).
- Zeiger, E., B. Gollapudi, M.J. Aardema et al.: Opportunities to integrate new approaches in genetic toxicology: An ILSI‐HESI workshop report. Environ. Molec. Mutagen. 56:277–285 (2015).
- International Programme on Chemical Safety (IPCS): Biomarkers in Risk Assessment: Validity and Validation. Geneva: World Health Organization, 2001.
- Dary, C.C., J.J. Quachenboss, C.H. Nauman, and S.C. Hein: Relationship of biomarkers of exposure to risk assessment and risk management. In Biomarkers for Agrochemicals and Toxic Substances, J.W. Blancato, R.N. Brown, C.C. Dary, and M.A. Saleh (eds.). ACS Symposium Series 643, Washington, DC: ACS, 1990. pp. 2–23.
- Lobenhofer, E.K., X. Cui, L. Bennett et al.: Exploration of low-dose estrogen effects: identification of No Observed Transcriptional Effect Level (NOTEL). Toxicol. Pathol. 32(4): 482–492 (2004).
- Judson, R.S., R.J. Kavlock, R.W. Setzer et al.: Estimating toxicity-related biological pathway altering doses for high-throughput chemical risk assessment. Chem. Res. Toxicol. 24(4): 451–462 (2011).
- Judson, R., R. Kavlock, M. Martin et al.: Perspectives on validation of high-throughput assays supporting 21st century toxicity testing. Altex-Altern Exper. 30(1):51–66 (2013).
- Wang, I.M., B. Zhang, X. Yang et al.: Systems analysis of eleven rodent disease models reveals an inflammatome signature and key drivers. Molec. Syst. Biol. 8(2012).
- Waddington, C.H.: The epigenotype. Endeavour 18(18–20),(1942).
- Morange, M.: The relations between genetics and epigenetics - A historical point of view. Ann. NY Acad. Sci. 981: 50–60 (2002).
- Feinberg, A.P.: Epigenetics at the epicenter of modern medicine. J. Am. Med. Assoc. 299(11):1345–1350 (2008).
- Foley, D.L., J.M. Craig, R. Morley et al.: Prospects for epigenetic epidemiology. Amer. J. Epidemiol. 169(4):389–400 (2009).
- Stein, R.A.: Epigenetics and environmental exposures. J. Epidemiol. Comm. Health 66(1):8–13 (2012).
- Michels, K.B.: Epigenetic Epidemiology. Dodrecht: Springer, 2012.
- Flanagan, J.M.: Human methylome variation and the rise of epigenetic epidemiology. Curr. Pharmacogen. Personalized Med. 8:89–91 (2010).
- Relton, C.L., and G.D. Smith: Epigenetic epidemiology of common complex disease: prospects for prediction, prevention, and treatment. Plos Med. 7(10), (2010).
- Ho, S.M., and W.Y. Tang: Techniques used in studies of epigenome dysregulation due to aberrant DNA methylation: An emphasis on fetal-based adult diseases. Reprod. Toxicol. 23(3):267–282 (2007).
- Goodman, J.I., K.A. Augustine, M.L. Cunnningham et al.: What do we need to know prior to thinking about incorporating an epigenetic evaluation into safety assessments? Toxicol. Sci. 116(2):375–381 (2010).
- Jablonka, E.: Epigenetic epidemiology. Int. J. Epidemiol. 33(5):929–935 (2004).
- Talens, R.P., D.I. Boomsma, E.W. Tobi et al.: Variation, patterns, and temporal stability of DNA methylation: considerations for epigenetic epidemiology. Faseb J. 24(9):3135–3144 (2010).
- Cantone, L., F. Nordio, L. Hou et al.: Inhalable metal-rich air particles and histone H3K4 dimethylation and H3K9 acetylation in a cross-sectional study of steel workers. Environ. Health Perspect. 119(7):964–969 (2011).
- Arita, A., J. Niu, Q. Qu et al.: Global levels of histone modifications in peripheral blood mononuclear cells of subjects with exposure to nickel. Environ. Health Perspect. 120(2):198 (2012).
- Ray, P.D., A. Yosim, and R.C. Fry: Incorporating epigenetic data into the risk assessment process for the toxic metals arsenic, cadmium, chromium, lead, and mercury: strategies and challenges. Front. Genet. 5, (2014).
- Rozek, L.S., D.C. Dolinoy, M.A. Sartor, and G.S. Omenn: Epigenetics: relevance and implications for public health. Ann. Rev. Public Health 35:105–122 (2014).
- Boekelheide, K., B. Blumberg, R.E. Chapin et al.: Predicting later-life outcomes of early-life exposures. Environ. Health Perspect. 120(10):1353–1361 (2012).
- Rasoulpour, R.J., M.J. LeBaron, R.G. Ellis-Hutchings, J. Klapacz, and B.B. Gollapudi: Epigenetic screening in product safety assessment: are we there yet? Toxicol. Mech. Meth. 21(4):298–311 (2011).
- Ryan, P.B., T.A. Burke, E.A.C. Hubal, J.J. Cura, and T.E. McKone: Using biomarkers to inform cumulative risk assessment. Environ. Health Perspect. 115(5):833–840 (2007).
- Butte, A.J., and I.S. Kohane: Creation and implications of a phenome-genome network. Nat. Biotechnol. 24(1): 55–62 (2006).
- Basu, N., J.M. Goodrich, and J. Head: Ecogenetics of mercury: From genetic polymorphisms and epigenetics to risk assessment and decision‐making. Environ. Toxicol. Chem. 33(6):1248–1258 (2014).
- National Research Council (NRC): Science and Decisions: Advancing Risk Assessment. Washington, D.C.: National Academies Press, 2009.
- EPA: Interim Policy on Genomics. Washington, D.C.: U.S. EPA, 2002.
- EPA: Potential implications of genomics for regulatory and risk assessment applications at EPA. Genomics Task Force Workgroup EPA/100/B-04/002 (2004).
- El-Masri, H.A., D.A. Bell, and C.J. Portier: Effects of glutathione transferase theta polymorphism on the risk estimates of dichloromethane to humans. Toxicol. Appl. Pharmacol. 158(3):221–230 (1999).
- David, R.M., H.J. Clewell, P.R. Gentry, T.R. Covington, D.A. Morgott, and D.J. Marino: Revised assessment of cancer risk to dichloromethane II. Application of probabilistic methods to cancer risk determinations. Regul. Toxicol. Pharmacol. 45(1):55–65 (2006).
- Ioannidis, J.P., P. Boffetta, J. Little et al.: Assessment of cumulative evidence on genetic associations: interim guidelines. Int. J. Epidemiol. 37(1):120–132 (2008).
- von Elm, E., D.G. Altman, M. Egger, S.J. Pocock, P.C. Gotzsche, and J.P. Vandenbroucke: The Strengthening the Reporting of Observational Studies in Epidemiology (STROBE) statement: guidelines for reporting observational studies. Lancet 370(9596):1453–1457 (2007).
- Little, J., J.P.T. Higgins, J.P.A. Ioannidis et al.: STrengthening the REporting of Genetic Association Studies (STREGA) - An Extension of the STROBE Statement. Plos Med. 6(2):151–163 (2009).
- Gallo, V., M. Egger, V. McCormack et al.: STrengthening the Reporting of OBservational studies in Epidemiology–Molecular Epidemiology STROBE-ME: an extension of the STROBE statement. J. Clin. Epidemiol. 64(12):1350–1363 (2011).
- Jennings, P.: Stress response pathways, toxicity pathways and adverse outcome pathways. Arch. Toxicol. 87(1):13–14 (2013).
- MacKay, C., M. Davies, V. Summerfield, and G. Maxwell: From Pathways to People: Applying the Adverse Outcome Pathway (AOP) for skin sensitization to risk assessment. Altex-Alter. Animal Exper. 30(4):473–486 (2013).
- Bergeson LL: Toxicogenomics and the workplace: what you need to know. Manufact. Today Jan/Feb: 8–9 (2003).
- Samuels, S.W.: Occupational medicine and its moral discontents. J. Occup. Environ. Med. 45(12):1226–1233 (2003).
- Hansson, S.O.: Should we protect the most sensitive people? J. Radiol. Protect. 29(2):211–218 (2009).
- Grodsky, J.A.: Genetics and environmental law: redefining public health. Calif. Law Rev. 93(1):171–270 (2005).
- Rothstein, M.A.: Legal conceptions of equality in the genomic age. Law Inequal. 25(2):429 (2007).